Generative AI is rapidly reshaping industries worldwide, empowering businesses to deliver exceptional customer experiences, streamline processes, and push innovation at an unprecedented scale. However, amidst the excitement, critical questions around the responsible use and implementation of such powerful technology have started to emerge.
Although responsible AI has been a key focus for the industry over the past decade, the increasing complexity of generative AI models brings unique challenges. Risks such as hallucinations, controllability, intellectual property breaches, and unintended harmful behaviors are real concerns that must be addressed proactively.
To harness the full potential of generative AI while reducing these risks, it’s essential to adopt mitigation techniques and controls as an integral part of the build process. Red teaming, an adversarial exploit simulation of a system used to identify vulnerabilities that might be exploited by a bad actor, is a crucial component of this effort.
At Data Reply and AWS, we are committed to helping organizations embrace the transformative opportunities generative AI presents, while fostering the safe, responsible, and trustworthy development of AI systems.
In this post, we explore how AWS services can be seamlessly integrated with open source tools to help establish a robust red teaming mechanism within your organization. Specifically, we discuss Data Reply’s red teaming solution, a comprehensive blueprint to enhance AI safety and responsible AI practices.
Understanding generative AI’s security challenges
Generative AI systems, though transformative, introduce unique security challenges that require specialized approaches to address them. These challenges manifest in two key ways: through inherent model vulnerabilities and adversarial threats.
The inherent vulnerabilities of these models include their potential of producing hallucinated responses (generating plausible but false information), their risk of generating inappropriate or harmful content, and their potential for unintended disclosure of sensitive training data.
These potential vulnerabilities could be exploited by adversaries through various threat vectors. Bad actors might employ techniques such as prompt injection to trick models into bypassing safety controls, intentionally altering training data to compromise model behavior, or systematically probing models to extract sensitive information embedded in their training data. For both types of vulnerabilities, red teaming is a useful mechanism to mitigate those challenges because it can help identify and measure inherent vulnerabilities through systematic testing, while also simulating real-world adversarial exploits to uncover potential exploitation paths.
What is red teaming?
Red teaming is a methodology used to test and evaluate systems by simulating real-world adversarial conditions. In the context of generative AI, it involves rigorously stress-testing models to identify weaknesses, evaluate resilience, and mitigate risks. This practice helps develop AI systems that are functional, safe, and trustworthy. By adopting red teaming as part of the AI development lifecycle, organizations can anticipate threats, implement robust safeguards, and promote trust in their AI solutions.
Red teaming is critical for uncovering vulnerabilities before they are exploited. Data Reply has partnered with AWS to offer support and best practices to help integrate responsible AI and red teaming into your workflows, helping you build secure AI models. This unlocks the following benefits:
- Mitigating unexpected risks – Generative AI systems can inadvertently produce harmful outputs, such as biased content or factually inaccurate information. With red teaming, Data Reply helps organizations test models for these weaknesses and identify vulnerabilities to adversarial exploitation, such as prompt injections or data poisoning.
- Compliance with AI regulation – As global regulations around AI continue to evolve, red teaming can help organizations by setting up mechanisms to systematically test their applications and make them more resilient, or serve as a tool to adhere to transparency and accountability requirements. Additionally, it maintains detailed audit trails and documentation of testing activities, which are critical artifacts that can be used as evidence for demonstrating compliance with standards and responding to regulatory inquiries.
- Reducing data leakage and malicious use – Although generative AI has the potential to be a force for good, models might also be exploited by adversaries looking to extract sensitive information or perform harmful actions. For instance, adversaries might craft prompts to extract private data from training sets or generate phishing emails and malicious code. Red teaming simulates such adversarial scenarios to identify vulnerabilities, enabling safeguards like prompt filtering, access controls, and output moderation.
The following chart outlines some of the common challenges in generative AI systems where red teaming can serve as a mitigation strategy.
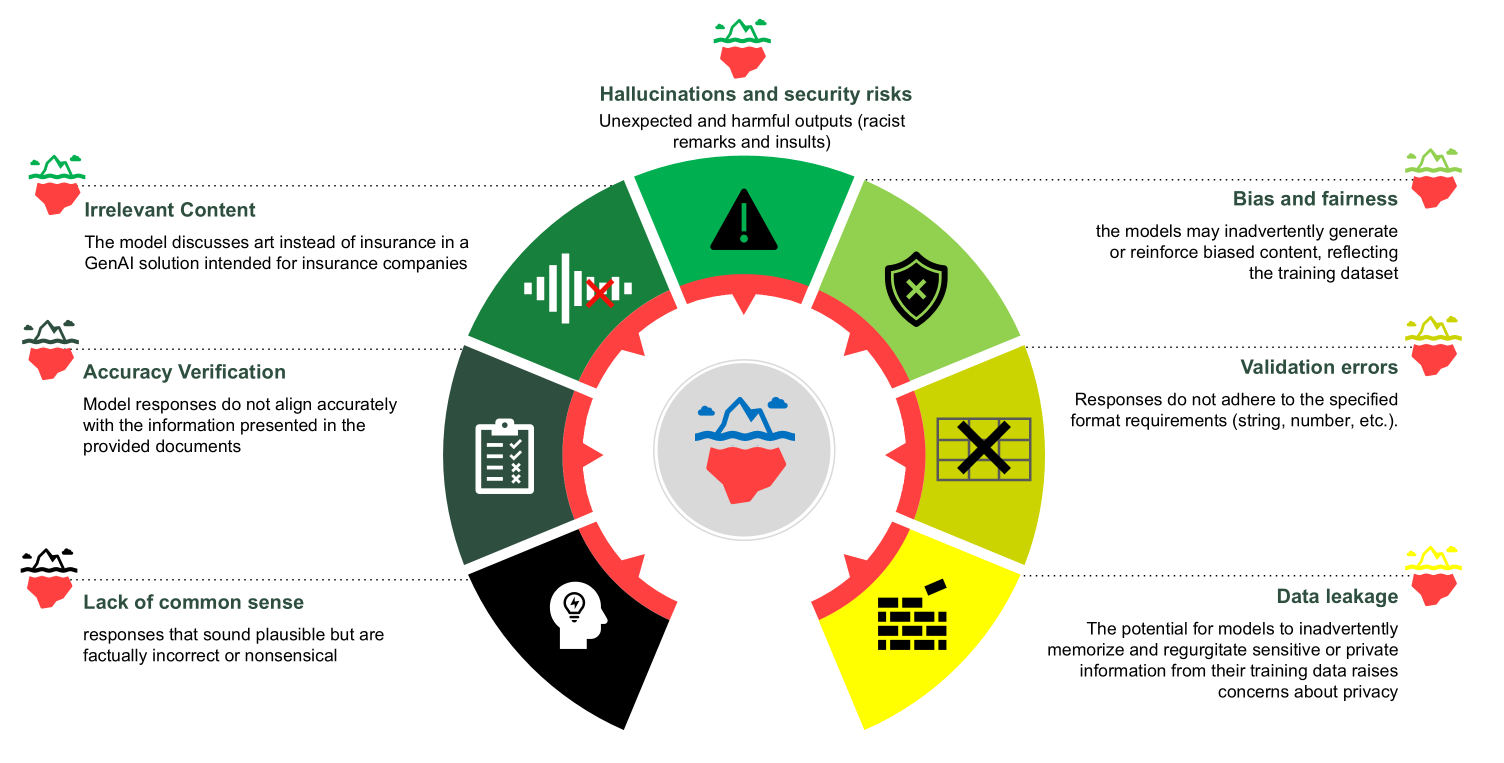
Before diving into specific threats, it’s important to acknowledge the value of having a systematic approach to AI security risk assessment for organizations deploying AI solutions. As an example, the OWASP Top 10 for LLMs can serve as a comprehensive framework for identifying and addressing critical AI vulnerabilities. This industry-standard framework categorizes key threats, including prompt injection, where malicious inputs manipulate model outputs; training data poisoning, which can compromise model integrity; and unauthorized disclosure of sensitive information embedded in model responses. It also addresses emerging risks such as insecure output handling and denial of service (DOS) that could disrupt AI operations. By using such frameworks alongside practical security testing approaches like red teaming exercises, organizations can implement targeted controls and monitoring to make sure their AI models remain secure, resilient, and align with regulatory requirements and responsible AI principles.
How Data Reply uses AWS services for responsible AI
Fairness is an essential component of responsible AI and, as such, part of the AWS core dimensions of responsible AI. To address potential fairness concerns, it can be helpful to evaluate disparities and imbalances in training data or outcomes. Amazon SageMaker Clarify helps identify potential biases during data preparation without requiring code. For example, you can specify input features such as gender or age, and SageMaker Clarify will run an analysis job to detect imbalances in those features. It generates a detailed visual report with metrics and measurements of potential bias, helping organizations understand and address imbalances.
During red teaming, SageMaker Clarify plays a key role by analyzing whether the model’s predictions and outputs treat all demographic groups equitably. If imbalances are identified, tools like Amazon SageMaker Data Wrangler can rebalance datasets using methods such as random undersampling, random oversampling, or Synthetic Minority Oversampling Technique (SMOTE). This supports the model’s fair and inclusive operation, even under adversarial testing conditions.
Veracity and robustness represent another critical dimension for responsible AI deployments. Tools like Amazon Bedrock provide comprehensive evaluation capabilities that enable organizations to assess model security and robustness through automated evaluation. These include specialized tasks such as question-answering assessments with adversarial inputs designed to probe model limitations. For instance, Amazon Bedrock can help you test model behavior across edge case scenarios by analyzing responses to carefully crafted inputs—from ambiguous queries to potentially misleading prompts—to evaluate if the models maintain reliability and accuracy even under challenging conditions.
Privacy and security go hand in hand when implementing responsible AI. Security at Amazon is “job zero” for all employees. Our strong security culture is reinforced from the top down with deep executive engagement and commitment, and from the bottom up with training, mentoring, and strong “see something, say something” as well as “when in doubt, escalate” and “no blame” principles. As an example of this commitment, Amazon Bedrock Guardrails provide organizations with a tool to incorporate robust content filtering mechanisms and protective measures against sensitive information disclosure.
Transparency is another best practice prescribed by industry standards, frameworks, and regulations, and is essential for building user trust in making informed decisions. LangFuse, an open source tool, plays a key role in providing transparency by keeping an audit trail of model decisions. This audit trail offers a way to trace model actions, helping organizations demonstrate accountability and adhere to evolving regulations.
Solution overview
To achieve the goals mentioned in the previous section, Data Reply has developed the Red Teaming Playground, a testing environment that combines several open source tools—like Giskard, LangFuse, and AWS FMEval—to assess the vulnerabilities of AI models. This playground allows AI builders to explore scenarios, perform white hat hacking, and evaluate how models react under adversarial conditions. The following diagram illustrates the solution architecture.
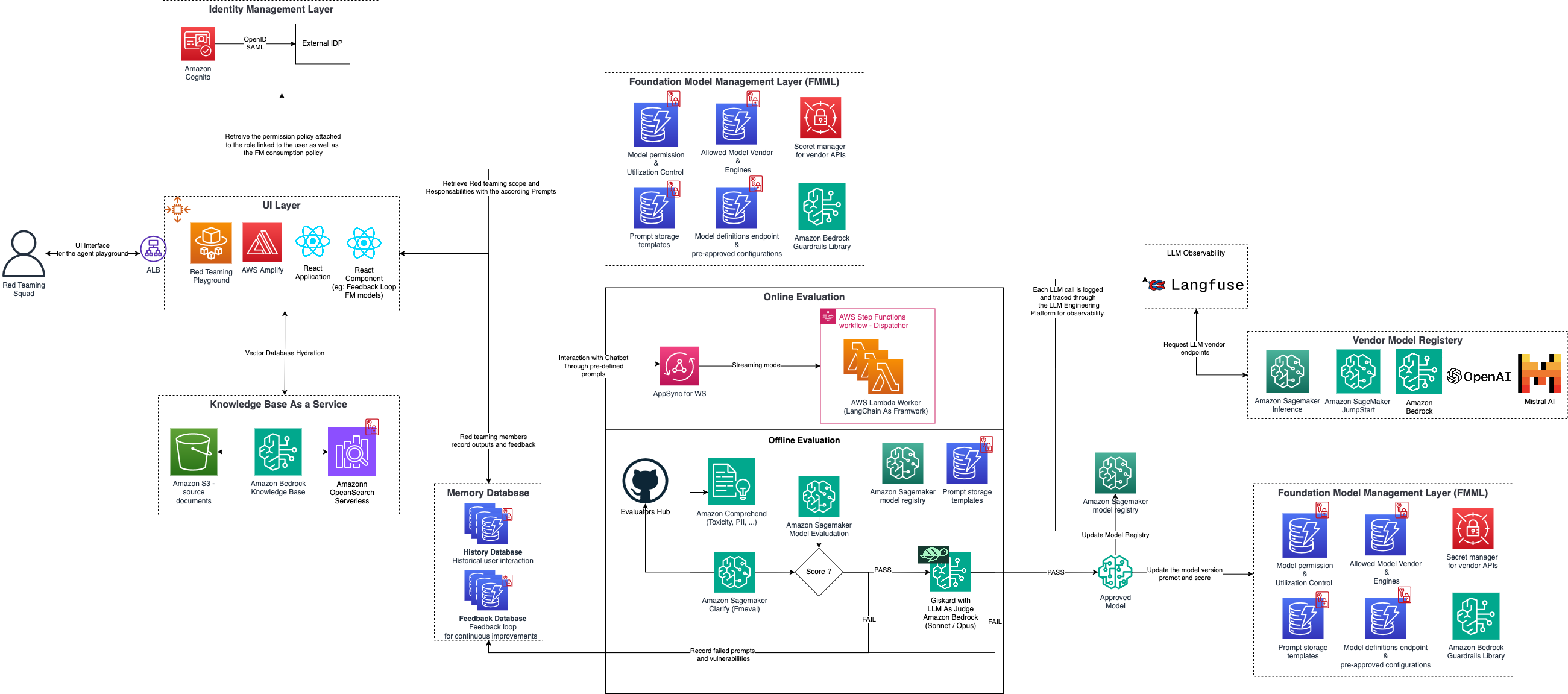
This playground is designed to help you responsibly develop and evaluate your generative AI systems, combining a robust multi-layered approach for authentication, user interaction, model management, and evaluation.
At the outset, the Identity Management Layer handles secure authentication, using Amazon Cognito and integration with external identity providers to help secure authorized access. Post-authentication, users access the UI Layer, a gateway to the Red Teaming Playground built on AWS Amplify and React. This UI directs traffic through an Application Load Balancer (ALB), facilitating seamless user interactions and allowing red team members to explore, interact, and stress-test models in real time. For knowledge retrieval, we use Amazon Bedrock Knowledge Bases, which integrates with Amazon Simple Storage Service (Amazon S3) for document storage, and Amazon OpenSearch Serverless for rapid and scalable search capabilities.
Central to this solution is the Foundation Model Management Layer, responsible for defining model policies and managing their deployment, using Amazon Bedrock Guardrails for safety, Amazon SageMaker services for model evaluation, and a vendor model registry comprising a range of foundation model (FM) options, including other vendor models, supporting model flexibility.
After the models are deployed, they go through online and offline evaluations to validate robustness.
Online evaluation uses AWS AppSync for WebSocket streaming to assess models in real time under adversarial conditions. A dedicated red teaming squad (authorized white hat testers) conducts evaluations focused on OWASP Top 10 for LLMs vulnerabilities, such as prompt injection, model theft, and attempts to alter model behavior. Online evaluation provides an interactive environment where human testers can pivot and respond dynamically to model answers, increasing the chances of identifying vulnerabilities or successfully jailbreaking the model.
Offline evaluation conducts a deeper analysis through services like SageMaker Clarify to check for biases and Amazon Comprehend to detect harmful content. The memory database captures interaction data, such as historical user prompts and model responses. LangFuse plays a vital role in maintaining an audit trail of model activities, allowing each model decision to be tracked for observability, accountability, and compliance. The offline evaluation pipeline uses tools like Giskard to detect performance, bias, and security issues in AI systems. It employs LLM-as-a-judge, where a large language model (LLM) evaluates AI responses for correctness, relevance, and adherence to responsible AI guidelines. Models are tested through offline evaluations first; if successful, they progress through online evaluation and ultimately move into the model registry.
The Red Teaming Playground is a dynamic environment designed to simulate scenarios and rigorously test models for vulnerabilities. Through a dedicated UI, the red team interacts with the model using a Q&A AI assistant (for instance, a Streamlit application), enabling real-time stress testing and evaluation. Team members can provide detailed feedback on model performance and log any issues or vulnerabilities encountered. This feedback is systematically integrated into the red teaming process, fostering continuous improvements and enhancing the model’s robustness and security.
Use case example: Mental health triage AI assistant
Imagine deploying a mental health triage AI assistant—an application that demands extra caution around sensitive topics like dosage information, health records, or judgement call questions. By defining a clear use case and establishing quality expectations, you can guide the model on when to answer, deflect, or provide a safe response:
- Answer – When the bot is confident that the question is within its domain and is able to retrieve a relevant response, it can provide a direct answer. For example, if asked “What are some common symptoms of anxiety?”, the bot can respond: “Common symptoms of anxiety include restlessness, fatigue, difficulty concentrating, and excessive worry. If you’re experiencing these, consider speaking to a healthcare professional.”
- Deflect – For questions outside the bot’s scope or purpose, the bot should deflect responsibility and guide the user toward appropriate human support. For instance, if asked “Why does life feel meaningless?”, the bot might reply: “It sounds like you’re going through a tough time. Would you like me to connect you to someone who can help?” This makes sure sensitive topics are handled carefully and responsibly.
- Safe response – When the question requires human validation or advice that the bot can’t provide, it should offer generalized, neutral suggestions to minimize risks. For example, in response to “How can I stop feeling anxious all the time?”, the bot might say: “Some people find practices like meditation, exercise, or journaling helpful, but I recommend consulting a healthcare provider for advice tailored to your needs.”
Red teaming results help refine model outputs by identifying risks and vulnerabilities. For example, consider a medical AI assistant developed by the fictional company AnyComp. By subjecting this assistant to a red teaming exercise, AnyComp can detect potential risks, such as the assistant generating unsolicited medical advice before deployment. With this insight, AnyComp can refine the assistant to either deflect such queries or provide a safe, appropriate response.
This structured approach—answer, deflect, and safe response—provides a comprehensive strategy for managing various types of questions and scenarios effectively. By clearly defining how to handle each category, you can make sure the AI assistant fulfills its purpose while maintaining safety and reliability. Red teaming further validates these strategies by rigorously testing interactions, making sure that the assistant remains useful and trustworthy in different situations.
Conclusion
Implementing responsible AI policies involves continuous improvement. Scaling solutions, like integrating SageMaker for model lifecycle monitoring or AWS CloudFormation for controlled deployments, helps organizations maintain robust AI governance as they grow.
Integrating responsible AI through red teaming is a crucial step to assess that generative AI systems operate responsibly, securely, and remain compliant. Data Reply collaborates with AWS to industrialize these efforts, from fairness checks to security stress tests, helping organizations stay ahead of emerging threats and evolving standards.
Data Reply has extensive expertise in helping customers adopt generative AI, especially with their GenAI Factory framework, which simplifies the transition from proof of concept to production, benefiting industries such as maintenance and customer service FAQs. The GenAI Factory initiative by Data Reply France is designed to overcome integration challenges and scale generative AI applications effectively, using AWS managed services like Amazon Bedrock and OpenSearch Serverless.
To learn more about Data Reply’s work, check out their specialized offerings for red teaming in generative AI and LLMOps.
About the authors
Cassandre Vandeputte is a Solutions Architect for AWS Public Sector based in Brussels. Since her first steps into the digital world, she has been passionate about harnessing technology to drive positive societal change. Beyond her work with intergovernmental organizations, she drives responsible AI practices across AWS EMEA customers.
Davide Gallitelli is a Senior Specialist Solutions Architect for AI/ML in the EMEA region. He is based in Brussels and works closely with customers throughout Benelux. He has been a developer since he was very young, starting to code at the age of 7. He started learning AI/ML at university, and has fallen in love with it since then.
Amine Aitelharraj is a seasoned cloud leader and ex-AWS Senior Consultant with over a decade of experience driving large-scale cloud, data, and AI transformations. Currently a Principal AWS Consultant and AWS Ambassador, he combines deep technical expertise with strategic leadership to deliver scalable, secure, and cost-efficient cloud solutions across sectors. Amine is passionate about GenAI, serverless architectures, and helping organizations unlock business value through modern data platforms.
Read More