A first-of-its-kind experiment to measure the impact of out-of-home advertising
By combining surveys with ads targeted to metro and commuter rail lines, Amazon researchers identify the fraction of residents of different neighborhoods exposed to the ads and measure ad effectiveness.
Economics
Egor AbramovMay 21, 02:47 PMMay 21, 02:47 PM
Amazon promotes its products and services through many advertising channels, and we naturally want to know how much bang we get for our buck. We thus try to measure the effectiveness of our ads through a combination of data modeling and, most importantly, experimentation.
In online environments, where the majority of ads live today, researchers can randomize ads and make use of tracking technologies like cookies to measure behavior. This approach provides high-quality data and statistically significant results. In contrast, more-conventional ads found outdoors, in malls and airports, and on transport, referred to as out-of-home (OOH) ads, dont offer the same opportunity for personalized behavior measurements and therefore havent lent themselves well to experiments.
To close this gap, our team at Amazon has developed and successfully implemented an experimental design that focuses on metro and commuter lines. Based on the knowledge of Amazon marketing teams and marketing agencies engaged by Amazon including several that specialize in OOH advertising this is the first experiment of its kind.
First, we randomized ads across commuter lines in a particular city (not So Paulo: the figure below is a hypothetical example). Second, we sent out an e-mail survey to residents, asking which lines they regularly use. From the survey, we calculated the fraction of residents who had the opportunity to see ads in various neighborhoods. We then used this data to directly measure the effectiveness of an ad campaign.
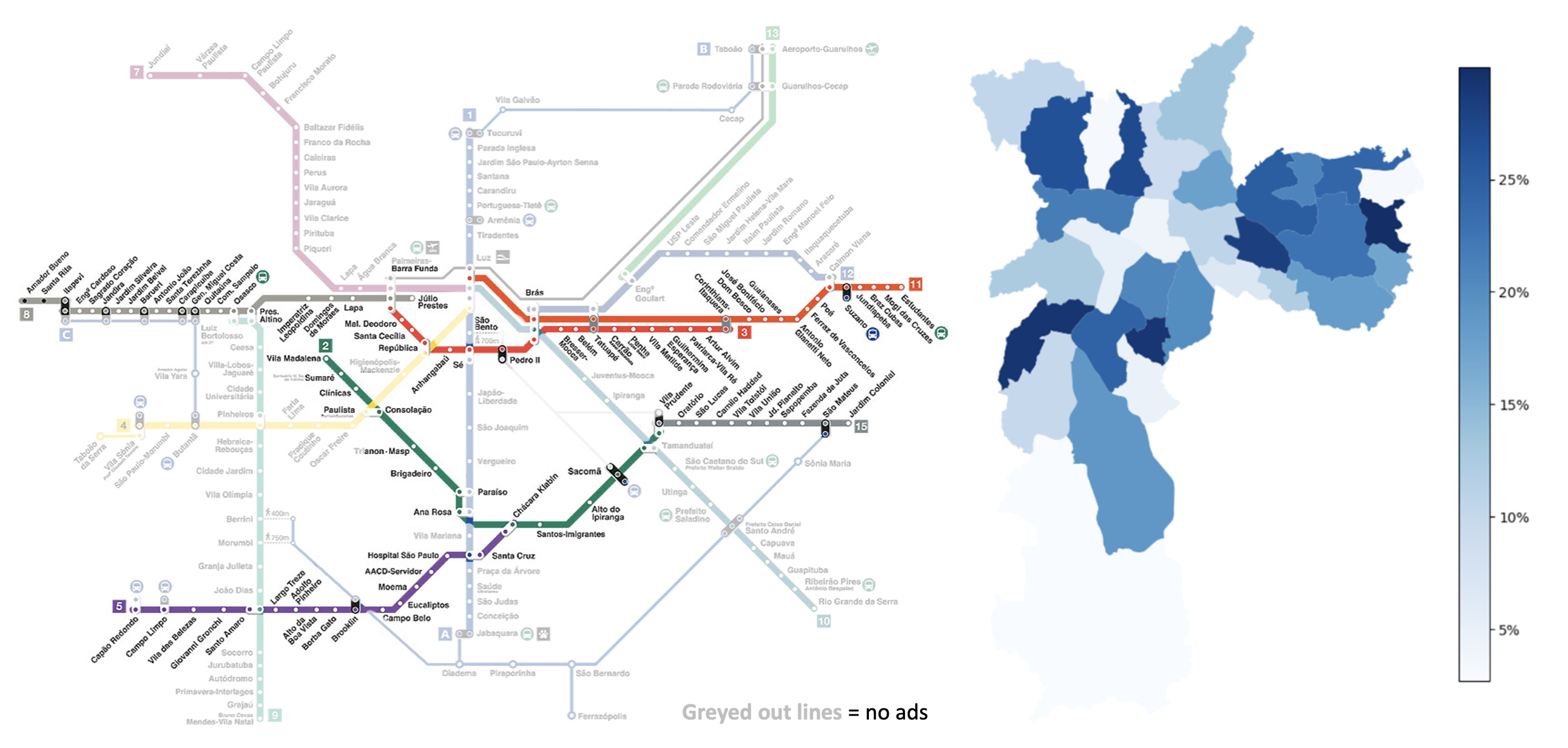
This radically simple approach produces strikingly clear results: over the course of a four-week ad campaign, the high-ad-exposure neighborhoods saw a measurable increase in sales versus neighborhoods with low exposure.
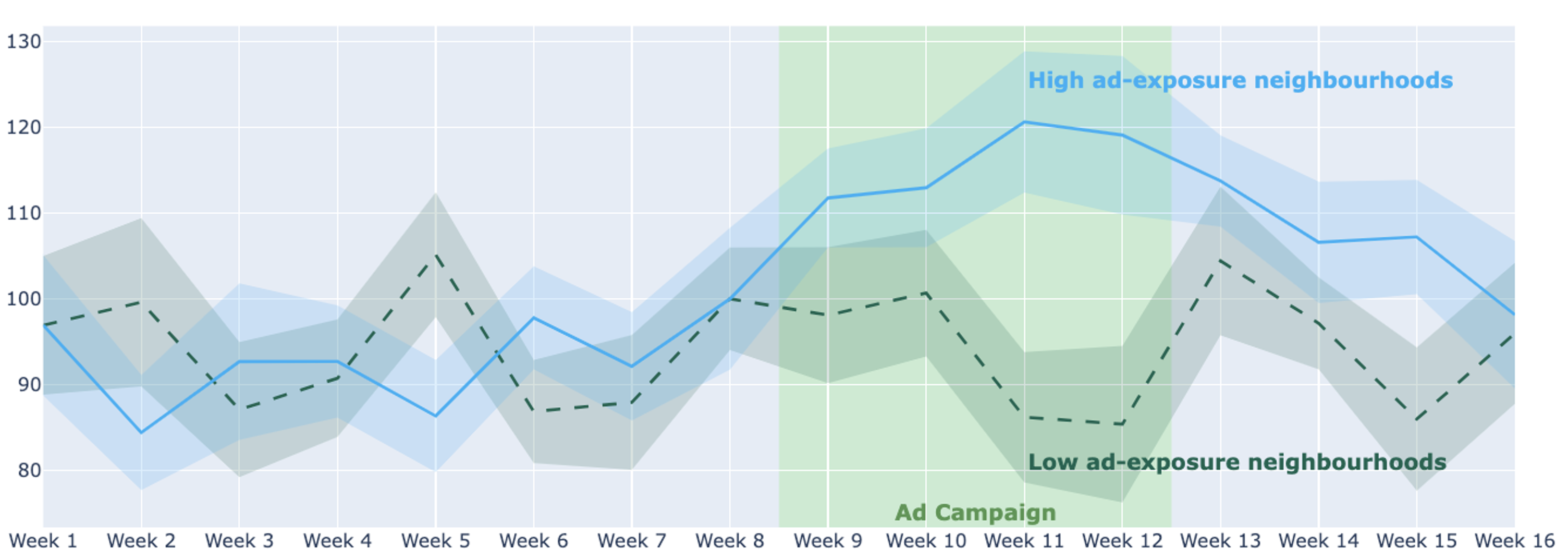
Our experimental design borrows concepts from a technique called geoexperimentation, an approach commonly used for online ads when individual user tracking or personalized ads arent available. Researchers deploying geoexperimentation take a given country and isolate geographical units states, regions, or metropolitan areas in which they randomly apply an ad campaign or not.
Geographic units are typically selected to be large enough that advertisements within a given unit are seen almost exclusively by people living there; that way, the campaigns impact is concentrated within the unit. To estimate the campaigns impact, researchers then compare sales numbers from different geographical units over time, with and without ads. Geoexperiments are effective because within any given country, there is usually a large enough number of relevant geographical units for the experiment to have sufficient statistical power.
There was a working assumption that OOH advertising wouldnt be a good candidate for a real-world implementation of geo experimentation, given how geographically concentrated OOH ad campaigns are. The typical geoexperiment requires dozens of geographic units to ensure sufficient statistical power, but OOH ads are generally deployed in only a handful of bigger cities or, in some cases, a single city within a country.
Using more-granular geographic units for example, city neighborhoods or districts is not an option either, as thered be no way to isolate or identify users who might see the ads. This, for instance, is the challenge posed by a billboard in one district that residents of other districts may drive past.
We realized, however, that these challenges can be met if you choose your OOH ad environments wisely. Targeting ads to metro and commuter rail lines lets us isolate and identify the users who have the opportunity to see them. Our survey asks which metro and commuter rail lines respondents use for commute and occasional travel, how often they so use them, and which part of the city they live in; this gives the fraction of residents exposed to our ads in each neighborhood. At the same time, the random ad placement creates random variation in ad exposure across the citys neighborhoods, giving us the number of geographic units we need for statistical power.
The idea, then, is to compare changes in sales in the treated and control regions, adjusted for historical sales trends. Many techniques used for analyzing geoexperiments apply here, too, including synthetic-control and difference-in-difference methods.
With this first-ever experimental design for OOH advertising, we believe weve opened the door for causal measurement of OOH ad effectiveness. To be sure, this implementation is specific to metro and commuter rail ads, which limits it to cities that support that kind of public transportation. However, we believe lessons learned from such experiments can be extrapolated to other types of OOH ad environments and can be combined with other, non-experiment-based measures of ad performance such as awareness surveys or multimedia models.
Research areas: Economics
Tags: Experimental design