Academic medical centers worldwide are building new AI tools to battle COVID-19 — including at Mass General, where one center is adopting NVIDIA DGX A100 AI systems to accelerate its work.
Researchers at the hospital’s Athinoula A. Martinos Center for Biomedical Imaging are working on models to segment and align multiple chest scans, calculate lung disease severity from X-ray images, and combine radiology data with other clinical variables to predict outcomes in COVID patients.
Built and tested using Mass General Brigham data, these models, once validated, could be used together in a hospital setting during and beyond the pandemic to bring radiology insights closer to the clinicians tracking patient progress and making treatment decisions.
“While helping hospitalists on the COVID-19 inpatient service, I realized that there’s a lot of information in radiologic images that’s not readily available to the folks making clinical decisions,” said Matthew D. Li, a radiology resident at Mass General and member of the Martinos Center’s QTIM Lab. “Using deep learning, we developed an algorithm to extract a lung disease severity score from chest X-rays that’s reproducible and scalable — something clinicians can track over time, along with other lab values like vital signs, pulse oximetry data and blood test results.”
The Martinos Center uses a variety of NVIDIA AI systems, including NVIDIA DGX-1, to accelerate its research. This summer, the center will install NVIDIA DGX A100 systems, each built with eight NVIDIA A100 Tensor Core GPUs and delivering 5 petaflops of AI performance.
“When we started working on COVID model development, it was all hands on deck. The quicker we could develop a model, the more immediately useful it would be,” said Jayashree Kalpathy-Cramer, director of the QTIM lab and the Center for Machine Learning at the Martinos Center. “If we didn’t have access to the sufficient computational resources, it would’ve been impossible to do.”
Comparing Notes: AI for Chest Imaging
COVID patients often get imaging studies — usually CT scans in Europe, and X-rays in the U.S. — to check for the disease’s impact on the lungs. Comparing a patient’s initial study with follow-ups can be a useful way to understand whether a patient is getting better or worse.
But segmenting and lining up two scans that have been taken in different body positions or from different angles, with distracting elements like wires in the image, is no easy feat.
Bruce Fischl, director of the Martinos Center’s Laboratory for Computational Neuroimaging, and Adrian Dalca, assistant professor in radiology at Harvard Medical School, took the underlying technology behind Dalca’s MRI comparison AI and applied it to chest X-rays, training the model on an NVIDIA DGX system.
“Radiologists spend a lot of time assessing if there is change or no change between two studies. This general technique can help with that,” Fischl said. “Our model labels 20 structures in a high-resolution X-ray and aligns them between two studies, taking less than a second for inference.”
This tool can be used in concert with Li and Kalpathy-Cramer’s research: a risk assessment model that analyzes a chest X-ray to assign a score for lung disease severity. The model can provide clinicians, researchers and infectious disease experts with a consistent, quantitative metric for lung impact, which is described subjectively in typical radiology reports.
Trained on a public dataset of over 150,000 chest X-rays, as well as a few hundred COVID-positive X-rays from Mass General, the severity score AI is being used for testing by four research groups at the hospital using the NVIDIA Clara Deploy SDK. Beyond the pandemic, the team plans to expand the model’s use to more conditions, like pulmonary edema, or wet lung.
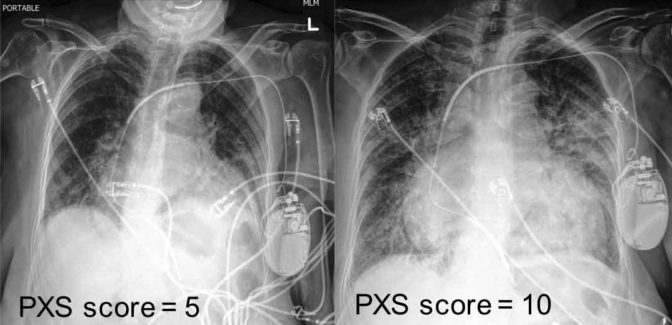
Foreseeing the Need for Ventilators
Chest imaging is just one variable in a COVID patient’s health. For the broader picture, the Martinos Center team is working with Brandon Westover, executive director of Mass General Brigham’s Clinical Data Animation Center.
Westover is developing AI models that predict clinical outcomes for both admitted patients and outpatient COVID cases, and Kalpathy-Cramer’s lung disease severity score could be integrated as one of the clinical variables for this tool.
The outpatient model analyzes 30 variables to create a risk score for each of hundreds of patients screened at the hospital network’s respiratory infection clinics — predicting the likelihood a patient will end up needing critical care or dying from COVID.
For patients already admitted to the hospital, a neural network predicts the hourly risk that a patient will require artificial breathing support in the next 12 hours, using variables including vital signs, age, pulse oximetry data and respiratory rate.
“These variables can be very subtle, but in combination can provide a pretty strong indication that a patient is getting worse,” Westover said. Running on an NVIDIA Quadro RTX 8000 GPU, the model is accessible through a front-end portal clinicians can use to see who’s most at risk, and which variables are contributing most to the risk score.
Better, Faster, Stronger: Research on NVIDIA DGX
Fischl says NVIDIA DGX systems help Martinos Center researchers more quickly iterate, experimenting with different ways to improve their AI algorithms. DGX A100, with NVIDIA A100 GPUs based on the NVIDIA Ampere architecture, will further speed the team’s work with third-generation Tensor Core technology.
“Quantitative differences make a qualitative difference,” he said. “I can imagine five ways to improve our algorithm, each of which would take seven hours of training. If I can turn those seven hours into just an hour, it makes the development cycle so much more efficient.”
The Martinos Center will use NVIDIA Mellanox switches and VAST Data storage infrastructure, enabling its developers to use NVIDIA GPUDirect technology to bypass the CPU and move data directly into or out of GPU memory, achieving better performance and faster AI training.
“Having access to this high-capacity, high-speed storage will allow us to to analyze raw multimodal data from our research MRI, PET and MEG scanners,” said Matthew Rosen, assistant professor in radiology at Harvard Medical School, who co-directs the Center for Machine Learning at the Martinos Center. “The VAST storage system, when linked with the new A100 GPUs, is going to offer an amazing opportunity to set a new standard for the future of intelligent imaging.”
To learn more about how AI and accelerated computing are helping healthcare institutions fight the pandemic, visit our COVID page.
Main image shows chest x-ray and corresponding heat map, highlighting areas with lung disease. Image from the researchers’ paper in Radiology: Artificial Intelligence, available under open access.
The post Mass General’s Martinos Center Adopts AI for COVID, Radiology Research appeared first on The Official NVIDIA Blog.