There is increasing interest in computer science and elsewhere to understand and improve peer review (see here for an overview). With this motivation, we conducted two experiments regarding peer review which we summarize in this blog post.
Pros and Cons of Posting Preprints Online
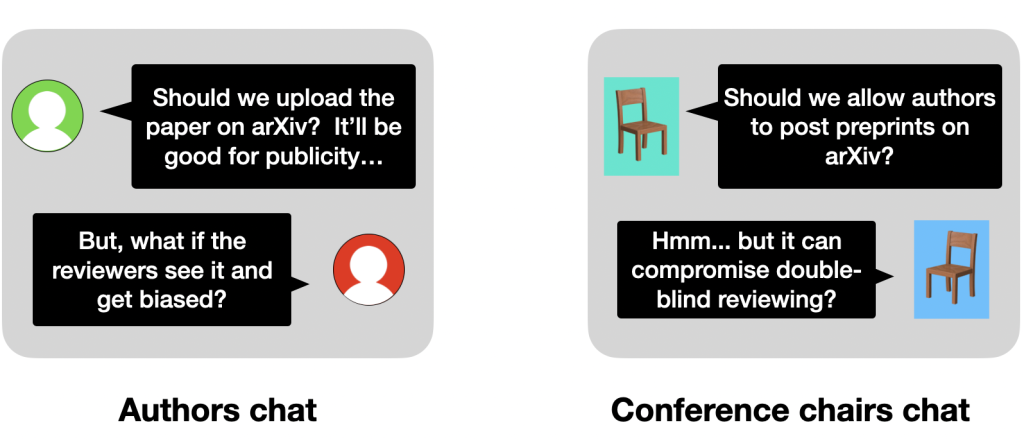
Motivation. Authors posting preprints online before review in double-blind peer-review is a widely debated issue of policy as well as authors’ personal choice. This choice is especially challenging for authors who perceive that they may be at a disadvantage in the review process if their identity is revealed. By posting their preprints online they stand to gain publicity but may lose benefits of double-blind review. We substantiate this debate quantitatively by addressing two research questions.
Research questions. We study the following research questions:
- (Q1) What fraction of reviewers deliberately search for their assigned paper on the Internet?
- (Q2) For preprints posted online, what is the relation between the papers’ visibility and the ranking of the authors’ affiliations?
Methods. We conduct survey-based experiments in the ICML 2021 and EC 2021 conferences.
- (Q1) We conduct an anonymized survey, where we ask each reviewer whether they deliberately searched online for any of their assigned papers.
- (Q2) We consider the papers submitted to ICML or EC which were also available online before review. We survey relevant reviewers to assess the visibility of these papers: we ask them whether they have seen these papers online outside of the reviewing context. Finally, we compute a correlation between papers’ visibility and associated authors’ affiliations’ ranking.
Key findings. We report two main insights:
- (Q1) More than a third of the respondents self-report searching online for their assigned paper in both ICML and EC.
- (Q2) We find a weak positive correlation: preprints from better-ranked affiliations enjoy a higher visibility. In ICML, the correlation coefficient is 0.06 and is statistically significant; in EC, the correlation coefficient is 0.05 and is not statistically significant. In particular, papers associated with the top-10-ranked affiliations had a visibility of about 11% in ICML and 22% in EC, whereas the remaining papers had a visibility of 7% and 18% respectively.
Implications. Conference organizers looking to design blinding policies and authors looking to post preprints online can use our findings to gauge the tradeoffs involved.
Details. https://arxiv.org/pdf/2203.17259.pdf
Citation Bias
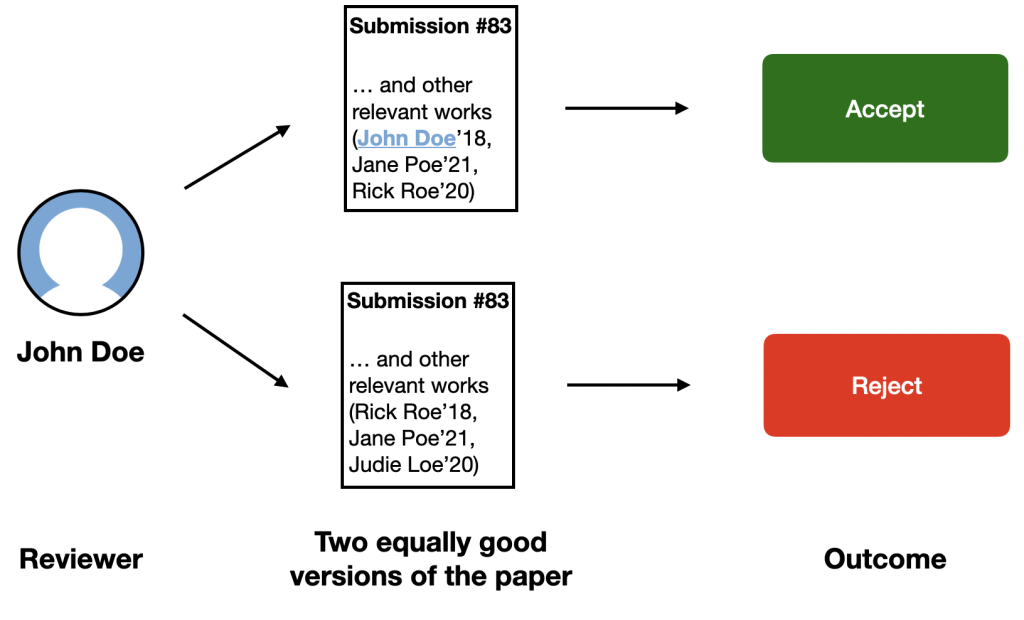
Motivation. Many anecdotes suggest that including citations to the works of potential reviewers is a good (albeit unethical) way to increase the acceptance chances of a manuscript.
Research question. Does the citation of a reviewer’s work in a submission cause the reviewer to be positively biased towards the submission, that is, cause a shift in reviewer’s evaluation that goes beyond the genuine change in the submission’s scientific merit?
Methods. We pair cited and uncited reviewers for each submitted paper and then carefully analyze the differences in their scores. Our analysis accounts for the many confounding factors that may exist. By pairing reviewers, we alleviate the confounding factor of “paper quality” as both cited and uncited reviewers review the same paper. We also control for confounders related to reviewer identities by accommodating various associated aspects such as the reviewers’ expertise and preferences in reviewing papers. Finally, we analyze reviews of uncited reviewers to exclude cases in which a reviewer genuinely decreases their evaluation of a paper because it fails to cite their own relevant past work.
Key findings. Our findings suggest that citation bias exists, and papers enjoy higher scores from a cited reviewer. Due to this bias, the expected increase in a cited reviewer’s score is 0.16 (on a 6 point scale) in ICML and 0.23 (on a 5 point scale) in EC. For reference, a one-point increase of a score by a single reviewer improves the position of a submission by 11% on average.
Implications. We detect and quantify the strength of citation bias in peer review, informing stakeholders of the presence of the bias. Our work also raises an important open problem of mitigating citation bias.
Details. https://arxiv.org/pdf/2203.17239.pdf
Acknowledgements
The post is based on joint works with Ivan Stelmakh, Ryan Liu, Xinwei Shen, Marina Meila, Shuchi Chawla, Federico Echenique, and Nihar B. Shah.