Hirschberg explains why mastering empathetic speech is critical for successful dialogue systems.Read More
Amazon paper exposes biases in unreliable-news datasets
The paper, which received honorable mention at EACL, presents guidelines for better analysis and construction of datasets.Read More
A Grand Slam: GFN Thursday Scores 1,000th PC Game, Streaming Instantly on GeForce NOW
This GFN Thursday marks a new millennium for GeForce NOW.
By adding 13 games this week, our cloud game-streaming service now offers members instant access to 1,000 PC games.
That’s 1,000 games that members can stream instantly to underpowered PCs, Macs, Chromebooks, SHIELD TVs, Android devices, iPhones and iPads. Devices that otherwise wouldn’t dream of playing the latest PC hits now have access to 1,000 fully optimized games, streamed with GeForce performance.
The milestone marks an increase of more than 500 games since the service left beta less than 18 months ago.
And the best part? We’re just getting warmed up.
Playing with the Best
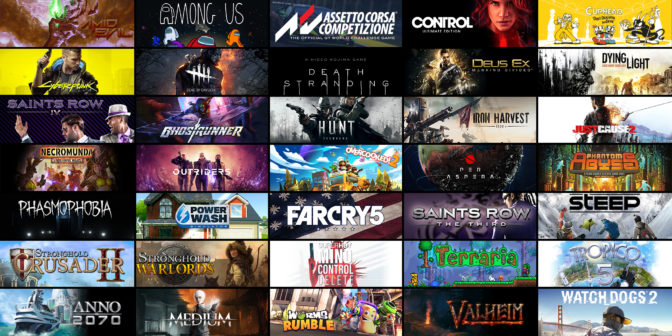
A grand milestone like this wouldn’t have been possible without the developers and publishers who opted in to stream their games on our open cloud-gaming service.
Publishers like Riot Games, Bungie, Paradox Interactive, Epic Games and more know that bringing their games to the cloud can be easy, and enables more gamers to play their titles. And partners like Square Enix have used GeForce NOW to make sure anyone and everyone can experience their new games, like Outriders, both as a demo and at launch.
These tremendous partners understand the value of making sure that members can play the PC games they already own across their devices. There are more than 300 of these partners who have shown how much they believe in our cloud gaming philosophy, with more joining every GFN Thursday.
Endless Choice
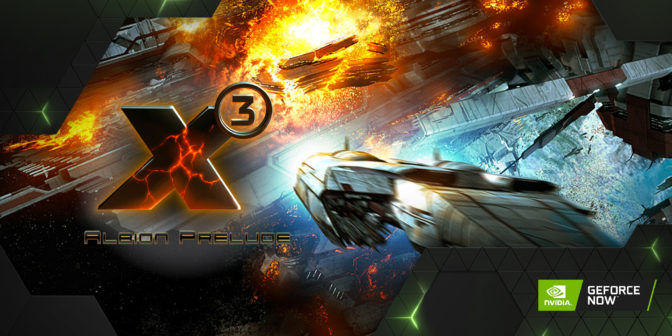
With 1,000 games in the GeForce NOW library, including nearly 100 free-to-play games that all members have access to, there’s a title for every type of PC gamer.
Want to become a hero in a strange new land? RPGs like The Witcher 3: Game of the Year Edition put you in the middle of fantasy epics, while exploration games like ASTRONEER challenge you to survive on a strange, brightly colored planet.
Looking for a little history lesson? Travel back to ancient Greece in Assassin’s Creed Odyssey, or rule over the Middle Ages in Crusader Kings III.
Members can meet up with their friends, playing cooperatively in games like Destiny 2, Valheim and OUTRIDERS, or competitively in Rocket League and Counter-Strike: Global Offensive. Or they can enjoy a stunning story in acclaimed titles like Death Stranding, Life is Strange 2, Alan Wake and more.
Want to experience real-time ray tracing for yourself? Priority and Founders members play Control, Shadow of the Tomb Raider, Cyberpunk 2077 and more with RTX ON.
There’s a game for every mood. Feeling spooky? Try to survive in Dead by Daylight or Outlast. Feeling spooky and don’t want to be alone? Group up in Phasmophobia and shout at some ghosts. Maybe you’re only afraid of zombies? See how long you can survive in games like 7 Days to Die and Dying Light.
Feel like a collectable card game? Try out Legends of Runeterra or Magic The Gathering: Arena. Feel like a strategy game? How about a sci-fi 4X sim like Endless Space 2, or a historical take on tactics like Hearts of Iron II Complete? Every genre is playable instantly on GeForce NOW.
Looking for a Castaway moment, out in the middle of the ocean with only a shark and some distant dry land to keep you company? Check out Raft.
“Hang on, GeForce NOW,” you might say. “I want to actually play as the shark. Like, literally be a shark. Can you make that happen?” We’ve got that, too: Maneater is for you.
There’s always a new game to discover with a library this big, streaming instantly. And every GFN Thursday brings even more gaming goodness.
All of This and More
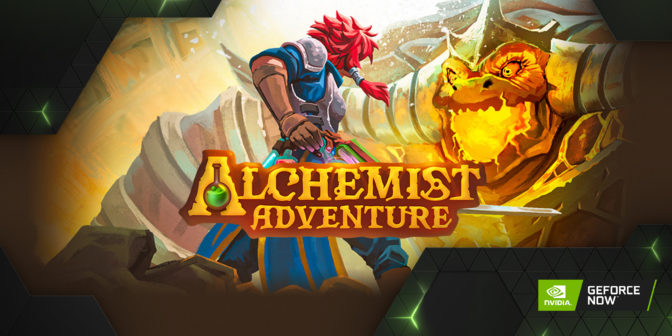
Over a dozen games released this GFN Thursday, bringing GeForce NOW to the grand milestone. This week’s new additions to the cloud library are:
- The Immortal Mayor (day-and-date release on Steam, July 15)
- Lost at Sea (day-and-date release on Steam, July 15)
- Obduction (Free on Epic Games Store until July 22)
- Alchemist Adventure (Steam)
- Retro Machina (Steam)
- Space Colony: Steam Edition (Steam)
- Tained Grail: Conquest (Steam)
- Unity of Command II (Steam)
- Warframe (Digital Extremes)
- Wildermyth (Steam)
- X3: Albion Prelude (Steam)
- Ys: Memories of Celceta (Steam)
- Ys Origin (Steam)
There’s a whole lot of games to play on GeForce NOW. What are you grinding this weekend? And what are some of your favorites among the 1,000-game library? Let us know on Twitter or in the comments below.
The post A Grand Slam: GFN Thursday Scores 1,000th PC Game, Streaming Instantly on GeForce NOW appeared first on The Official NVIDIA Blog.
From Vision to Language: Semi-supervised Learning in Action…at Scale
Posted by Thang Luong, Staff Research Scientist, Google Research and Jingcao Hu, Senior Staff Software Engineer, Google Search
Supervised learning, the machine learning task of training predictive models using data points with known outcomes (i.e., labeled data), is generally the preferred approach in industry because of its simplicity. However, supervised learning requires accurately labeled data, the collection of which is often labor intensive. In addition, as model efficiency improves with better architectures, algorithms, and hardware (GPUs / TPUs), training large models to achieve better quality becomes more accessible, which, in turn, requires even more labeled data for continued progress.
To mitigate such data acquisition challenges, semi-supervised learning, a machine learning paradigm that combines a small amount of labeled data with a large amount of unlabeled data, has recently seen success with methods such as UDA, SimCLR, and many others. In our previous work, we demonstrated for the first time that a semi-supervised learning approach, Noisy Student, can achieve state-of-the-art performance on ImageNet, a large-scale academic benchmark for image classification, by utilizing many more unlabeled examples.
Inspired by these results, today we are excited to present semi-supervised distillation (SSD), a simplified version of Noisy Student, and demonstrate its successful application to the language domain. We apply SSD to language understanding within the context of Google Search, resulting in high performance gains. This is the first successful instance of semi-supervised learning applied at such a large scale and demonstrates the potential impact of such approaches for production-scale systems.
Noisy Student Training
Prior to our development of Noisy Student, there was a large body of research into semi-supervised learning. In spite of this extensive research, however, such systems typically worked well only in the low-data regime, e.g., CIFAR, SVHN, and 10% ImageNet. When labeled data were abundant, such models were unable to compete with fully supervised learning systems, which prevented semi-supervised approaches from being applied to important applications in production, such as search engines and self-driving cars. This shortcoming motivated our development of Noisy Student Training, a semi-supervised learning approach that worked well in the high-data regime, and at the time achieved state-of-the-art accuracy on ImageNet using 130M additional unlabeled images.
Noisy Student Training has 4 simple steps:
- Train a classifier (the teacher) on labeled data.
- The teacher then infers pseudo-labels on a much larger unlabeled dataset.
- Then, it trains a larger classifier on the combined labeled and pseudo-labeled data, while also adding noise (noisy student).
- (Optional) Going back to step 2, the student may be used as a new teacher.
![]() |
An illustration of Noisy Student Training through four simple steps. We use two types of noise: model noise (Dropout, Stochastic Depth) and input noise (data augmentation, such as RandAugment). |
One can view Noisy Student as a form of self-training, because the model generates pseudo-labels with which it retrains itself to improve performance. A surprising property of Noisy Student Training is that the trained models work extremely well on robustness test sets for which it was not optimized, including ImageNet-A, ImageNet-C, and ImageNet-P. We hypothesize that the noise added during training not only helps with the learning, but also makes the model more robust.
![]() |
Examples of images that are classified incorrectly by the baseline model, but correctly by Noisy Student. Left: An unmodified image from ImageNet-A. Middle and Right: Images with noise added, selected from ImageNet-C. For more examples including ImageNet-P, please see the paper. |
Connections to Knowledge Distillation
Noisy Student is similar to knowledge distillation, which is a process of transferring knowledge from a large model (i.e., the teacher) to a smaller model (the student). The goal of distillation is to improve speed in order to build a model that is fast to run in production without sacrificing much in quality compared to the teacher. The simplest setup for distillation involves a single teacher and uses the same data, but in practice, one can use multiple teachers or a separate dataset for the student.
![]() |
Simple illustrations of Noisy Student and knowledge distillation. |
Unlike Noisy Student, knowledge distillation does not add noise during training (e.g., data augmentation or model regularization) and typically involves a smaller student model. In contrast, one can think of Noisy Student as the process of “knowledge expansion”.
Semi-Supervised Distillation
Another strategy for training production models is to apply Noisy Student training twice: first to get a larger teacher model T’ and then to derive a smaller student S. This approach produces a model that is better than either training with supervised learning or with Noisy Student training alone. Specifically, when applied to the vision domain for a family of EfficientNet models, ranging from EfficientNet-B0 with 5.3M parameters to EfficientNet-B7 with 66M parameters, this strategy achieves much better performance for each given model size (see Table 9 of the Noisy Student paper for more details).
Noisy Student training needs data augmentation, e.g., RandAugment (for vision) or SpecAugment (for speech), to work well. But in certain applications, e.g., natural language processing, such types of input noise are not readily available. For those applications, Noisy Student Training can be simplified to have no noise. In that case, the above two-stage process becomes a simpler method, which we call Semi-Supervised Distillation (SSD). First, the teacher model infers pseudo-labels on the unlabeled dataset from which we then train a new teacher model (T’) that is of equal-or-larger size than the original teacher model. This step, which is essentially self-training, is then followed by knowledge distillation to produce a smaller student model for production.
![]() |
An illustration of Semi-Supervised Distillation (SSD), a 2-stage process that self-trains an equal-or-larger teacher (T’) before distilling to a student (S). |
Improving Search
Having succeeded in the vision domain, an application in the language understanding domain, like Google Search, is a logical next step with broader user impact. In this case, we focus on an important ranking component in Search, which builds on BERT to better understand languages. This task turns out to be well-suited for SSD. Indeed, applying SSD to the ranking component to better understand the relevance of candidate search results to queries achieved one of the highest performance gains among top launches at Search in 2020. Below is an example of a query where the improved model demonstrates better language understanding.
![]() |
With the implementation of SSD, Search is able to find documents that are more relevant to user queries. |
Future Research & Challenges
We have presented a successful instance of semi-supervised distillation (SSD) in the production scale setting of Search. We believe SSD will continue changing the landscape of machine learning usage in the industry from predominantly supervised learning to semi-supervised learning. While our results are promising, there is still much research needed in how to efficiently utilize unlabeled examples in the real world, which is often noisy, and apply them to various domains.
Acknowledgements
Zhenshuai Ding, Yanping Huang, Elizabeth Tucker, Hai Qian, and Steve He contributed immensely to this successful launch. The project would not have succeeded without contributions from members of both the Brain and Search teams: Shuyuan Zhang, Rohan Anil, Zhifeng Chen, Rigel Swavely, Chris Waterson, Avinash Atreya. Thanks to Qizhe Xie and Zihang Dai for feedback on the work. Also, thanks to Quoc Le, Yonghui Wu, Sundeep Tirumalareddy, Alexander Grushetsky, Pandu Nayak for their leadership support.
Optimizing model accuracy and latency using Bayesian multi-objective neural architecture search
What the research is:
We propose a method for sample-efficient optimization of the trade-offs between model accuracy and on-device prediction latency in deep neural networks.
Neural architecture search (NAS) aims to provide an automated framework that identifies the optimal architecture for a deep neural network machine learning model given an evaluation criterion such as model accuracy. The continuing trend toward deploying models on end user devices such as mobile phones has led to increased interest in optimizing multiple competing objectives in order to achieve an optimal balance between predictive performance and computational complexity (e.g., total number of flops), memory footprint, and latency of the model.
Existing NAS methods that rely on reinforcement learning and/or evolutionary strategies can incur prohibitively high computational costs because they require training and evaluating a large number of architectures. Many other approaches require integrating the optimization framework into the training and evaluation workflows, making it difficult to generalize to different production use-cases. In our work, we bridge these gaps by providing a NAS methodology that requires zero code change to a user’s training flow and can thus easily leverage existing large-scale training infrastructure while providing highly sample-efficient optimization of multiple competing objectives.
We leverage recent advances in multi-objective and high-dimensional Bayesian optimization (BO), a popular method for black-box optimization of computationally expensive functions. We demonstrate the utility of our method by optimizing the architecture and hyperparameters of a real-world natural language understanding model used at Facebook.
How it works:
NLU Use-Case
We focus on the specific problem of tuning the architecture and hyperparameters of an on-device natural language understanding (NLU) model that is commonly used by conversational agents found in most mobile devices and smart speakers. The primary objective of the NLU model is to understand the user’s semantic expression and to convert it into a structured decoupled representation that can be understood by downstream programs. The NLU model shown in Figure 1 is an encoder-decoder non-autoregressive (NAR) architecture based on the state-of-the-art span pointer formulation.
Figure 1: Non-autoregressive model architecture of the NLU semantic parsing
The NLU model serves as the first stage in conversational assistants and high accuracy is crucial for a positive user experience. Conversational assistants operate over the user’s language, potentially in privacy-sensitive situations such as when sending a message. For this reason, they generally run “on-device,” which comes at the cost of limited computational resources. Moreover, it is important that the model also achieves short on-device inference time (latency) to ensure a responsive user experience. While we generally expect a complex NLU model with a large number of parameters to achieve better accuracy, complex models tend to have high latency. Hence, we are interested in exploring the trade-offs between accuracy and latency by optimizing a total of 24 hyperparameters so we can pick a model that offers an overall positive user experience by balancing quality and latency. Specifically, we optimize the 99th percentile of latency across repeated measurements and the accuracy on a held-out data set.
Methods
BO is typically most effective on search spaces with less than 10 to 15 dimensions. To scale to the 24-dimensional search space in this work, we leverage recent work on high-dimensional BO [1]. Figure 2 shows that the model proposed by [1], which uses a sparse axis-aligned subspace (SAAS) prior and fully Bayesian inference, is crucial to achieving good model fits and outperforms a standard Gaussian process (GP) model with maximum a posteriori (MAP) inference on both accuracy and latency objective.
Figure 2: We illustrate the leave-one-out cross-validation performance for the accuracy and latency objectives. We observe that the SAAS model fits better than a standard GP using MAP.
To efficiently explore the trade-offs between multiple objectives, we use the parallel noisy expected hypervolume improvement (qNEHVI) acquisition function [2], which enables evaluating many architectures in parallel (we use a batch size of 16 in this work) and naturally handles the observation noise that is present in both latency and accuracy metrics: prediction latency is subject to measurement error and and accuracy is subject to randomness in NN training due to optimizing parameters using stochastic gradient methods.
Results
We compare the optimization performance of BO to Sobol (quasi-random) search. Figure 3 shows the results, where the objectives are normalized with respect to the production model, making the reference point equal to (1, 1). Using 240 evaluations, Sobol was only able to find two configurations that outperformed the reference point. On the other hand, our BO method was able to explore the trade-offs between the objectives and improve latency by more than 25% while at the same time improving model accuracy.
Figure 3: On the left, we see that Sobol (quasi-random) search is an inefficient approach that only finds two configurations that are better than the reference point (1,1). On the right, our BO method is much more sample-efficient and is able to explore the trade-offs between accuracy and latency.
Why it matters:
This new method has unlocked on-device deployment for this natural language understanding model as well as several other models at Facebook. Our method requires zero code changes to the existing training and evaluation workflows, making it easily generalizable to different architecture search use cases. We hope that machine learning researchers, practitioners, and engineers find this method useful in their applications and foundational for future research on NAS.
Read the full paper:
https://arxiv.org/abs/2106.11890
Citations:
[1] Eriksson, David, and Martin Jankowiak. “High-Dimensional Bayesian Optimization with Sparse Axis-Aligned Subspaces.” Conference on Uncertainty in Artificial Intelligence (UAI), 2021. [2] Daulton, Samuel, Maximilian Balandat, and Eytan Bakshy. “Parallel Bayesian Optimization of Multiple Noisy Objectives with Expected Hypervolume Improvement.” arXiv preprint arXiv:2105.08195, 2021.Try it yourself:
Check out our tutorial in Ax showing how to use the open-source implementation of integrated qNEHVI with GPs with SAAS priors to optimize two synthetic objectives.
The post Optimizing model accuracy and latency using Bayesian multi-objective neural architecture search appeared first on Facebook Research.
NVIDIA’s Liila Torabi Talks the New Era of Robotics Through Isaac Sim
Robots are not just limited to the assembly line. At NVIDIA, Liila Torabi works on making the next generation of robotics possible. Torabi is the senior product manager for Isaac Sim, a robotics and AI simulation platform powered by NVIDIA Omniverse.
Torabi spoke with NVIDIA AI Podcast host Noah Kravitz about the new era of robotics, one driven by making robots smarter through AI.
Isaac Sim is used to power photorealistic, physically accurate virtual environments to develop, test and manage AI-based robots.
Key Points From This Episode:
- To get to a point where robots and humans can interact and work together, developers need to train the robots and simulate their behavior ahead of time to ensure performance, safety and a variety of other factors. This is where Isaac Sim comes into play.
- For Torabi, the biggest technical hurdle is having the robot do more sophisticated jobs. Robot manipulation with different objects, shapes and environments is a challenge.
Tweetables:
“For robotics to get into the next era, we need it to be smarter, so we need the AI component to this.” — Liila Torabi [8:08]
“NVIDIA is well positioned for playing an important role in this next era of robotics because not only do we have the hardware for it, we know how to use this hardware to make this thing smarter. That’s why I’m very excited to see where we can go with Isaac Sim. ” — Liila Torabi [12:09]
You Might Also Like:
UC Berkeley’s Pieter Abbeel on How Deep Learning Will Help Robots Learn
Robots can do amazing things. Compare even the most advanced robots to a three-year-old, however, and they can come up short. UC Berkeley Professor Pieter Abbeel has pioneered the idea that deep learning could be the key to bridging that gap: creating robots that can learn how to move through the world more fluidly and naturally.
Sergey Levine on How Deep Learning Will Unleash a Robotics Revolution
What if robots could learn, teach themselves and pass on their knowledge to other robots? Where could that take machines and the notion of machine intelligence? And how fast could we get there? Sergey Levine, an assistant professor at UC Berkeley’s department of Electrical Engineering and Computer Sciences, explores these questions and more.
Jetson Interns Assemble! Interns Discuss Amazing AI Robots They’re Building
NVIDIA’s Jetson interns, recruited at top robotics competitions, discuss what they’re building with NVIDIA Jetson, including a delivery robot, a trash-disposing robot and a remote control car to aid in rescue missions.
Subscribe to the AI Podcast
Get the AI Podcast through iTunes, Google Podcasts, Google Play, Castbox, DoggCatcher, Overcast, PlayerFM, Pocket Casts, Podbay, PodBean, PodCruncher, PodKicker, Soundcloud, Spotify, Stitcher and TuneIn. If your favorite isn’t listed here, drop us a note.
Make the AI Podcast Better
Have a few minutes to spare? Fill out this listener survey. Your answers will help us make a better podcast.
The post NVIDIA’s Liila Torabi Talks the New Era of Robotics Through Isaac Sim appeared first on The Official NVIDIA Blog.
How AWS contributes to an earthquake safety system for the US West Coast
With the help of new machine learning techniques, a team at Caltech is upgrading their system to help scientists identify more earthquakes — and understand why they happen.Read More
The Surprising Effectiveness of PPO in Cooperative Multi-Agent Games
Recent years have demonstrated the potential of deep multi-agent reinforcement
learning (MARL) to train groups of AI agents that can collaborate to solve complex
tasks – for instance, AlphaStar achieved professional-level performance in the
Starcraft II video game, and OpenAI Five defeated the world champion in Dota2.
These successes, however, were powered by huge swaths of computational resources;
tens of thousands of CPUs, hundreds of GPUs, and even TPUs were used to collect and train on
a large volume of data. This has motivated the academic MARL community to develop
MARL methods which train more efficiently.
DeepMind’s AlphaStar attained professional level performance in StarCraft II, but required enormous amounts of
computational power to train.
Research in developing more efficient and effective MARL algorithms has focused on off-policy methods – which store and re-use data for multiple policy updates – rather than on-policy algorithms, which use newly collected training data before each update to the agents’ policies. This is largely due to the common belief that off-policy algorithms are much more sample-efficient than on-policy methods.
In this post, we outline our recent publication in which we re-examine many of these assumptions about on-policy algorithms. In particular, we analyze the performance of PPO, a popular single-agent on-policy RL algorithm, and demonstrate that with several simple modifications, PPO achieves strong performance in 3 popular MARL benchmarks while exhibiting a similar sample efficiency to popular off-policy algorithms in the majority of scenarios. We study the impact of these modifications through ablation studies and suggest concrete implementation and tuning practices which are critical for strong performance. We refer to PPO with these modifications as Multi-Agent PPO (MAPPO).
Extremism is bad for our business and what we are doing about it
A piece in today’s Washington Post alleges that it is not in Facebook’s financial interests to discourage extremism on our platform because research shows that outrage is what makes us more money. The research they cite did not even look at extremism. The opinion editorial is simply wrong.
Polarizing and extremist content is not just bad for society, it’s also bad for our business. Our business only works when people choose to use our apps because they have a positive impact on their lives – and advertisers choose to run ads that are relevant to the folks that they are trying to reach. Polarizing and extremist content drives both of them away. That’s part of the reason why we have invested in technology to find and remove hate speech and other forms of extremism that violate our rules quickly and have also built a global team of more than 35,000 people to help keep our services safe for everyone who uses them.
The research cited in the Post uses data that only reflects a specific period of time, the months leading up to last year’s US elections. That was a time when the US was experiencing historically high levels of polarization and it’s unclear whether those results would translate into other periods of time and other nations. It’s also important to note that political content is only a narrow slice of all social media content – representing just 6% of what people saw on our services during the height of last year’s election cycle. It’s reasonable to assume that number is even lower today.
The piece also paints an overly simplistic – and limited – picture of what a substantial amount of research into polarization and the role that Twitter and Facebook play in driving it actually shows so far. For example, research from Stanford University in 2020 showed that in some countries polarization was on the rise before Facebook even existed and in others it has been decreasing while internet and Facebook use increased.
Research published this year by the US National Bureau of Economic Research found that the best explanation for levels of polarization across nine countries studied were the specific conditions in each country, as opposed to general trends like the rise of internet use. A 2017 study published in the US Proceedings of the National Academy of Sciences found that polarization in the United States has increased the most among the demographic groups least likely to use the internet and social media. And data published in 2019 from the EU suggests that whether you get your news from social media or elsewhere, levels of ideological polarization are similar. One recent paper even showed that stopping social media use actually increased polarization.
However, none of these studies provide a definitive answer to the question of what role social media plays in driving polarization. The questions of what drives polarization in our society – and what are the best ways to reduce it – are complex. Much more research is clearly needed. That’s why we have not only commissioned our own research into this topic but have asked respected academics, including some of our critics, to conduct their own research independent from us.
For example, we have undertaken a new research partnership with external academics to better understand the impact of Facebook and Instagram on key political attitudes and behaviors during the US 2020 elections, building on an initiative we launched in 2018. It will examine the impact of how people interact with our products, including content shared in News Feed and across Instagram, and the role of features like content ranking systems. Matthew Gentzkow, who previously authored a study on how Facebook increased affective polarization, is one of the collaborators.
But there is another important point that is missing from the analysis in the Washington Post. That is the fact that all social media platforms, including but not limited to ours, reflect what is happening in society and what’s on people’s minds at any given moment. This includes the good, the bad, and the ugly. For example, in the weeks leading up to the World Cup, posts about soccer will naturally increase – not because we have programmed our algorithms to show people content about soccer but because that’s what people are thinking about. And just like politics, soccer strikes a deep emotional chord with people. How they react – the good, the bad, and the ugly – will be reflected on social media.
It is helpful to see Facebook’s role in the 2020 elections through a similar lens. Last year’s elections were perhaps the most emotional and contested in American history. Politics was everywhere in our society last year – in bars and cafes (at least before the pandemic lockdowns), on cable news, at family gatherings, and yes on social media too. And of course some of those discussions were emotional and polarizing because our politics is emotional and polarizing. It would be strange if some of that wasn’t reflected on social media.
But we also need to be very clear that extremist content is not in fact fundamental to our business model. It is counterproductive to it, as last year’s Stop Hate for Profit advertising boycott showed. What drives polarization deserves a deeper examination. That’s exactly why we are working with the world’s most esteemed academics to study this issue seriously so we can take the right steps to address it.
The post Extremism is bad for our business and what we are doing about it appeared first on Facebook Research.
Melting Pot: an evaluation suite for multi-agent reinforcement learning
Here we introduce Melting Pot, a scalable evaluation suite for multi-agent reinforcement learning. Melting Pot assesses generalisation to novel social situations involving both familiar and unfamiliar individuals, and has been designed to test a broad range of social interactions such as: cooperation, competition, deception, reciprocation, trust, stubbornness and so on. Melting Pot offers researchers a set of 21 MARL “substrates” (multi-agent games) on which to train agents, and over 85 unique test scenarios on which to evaluate these trained agents.Read More