As part of DeepMinds mission to solve intelligence, we created a system called AlphaCode that writes computer programs at a competitive level.Read More
The downside of machine learning in health care
While working toward her dissertation in computer science at MIT, Marzyeh Ghassemi wrote several papers on how machine-learning techniques from artificial intelligence could be applied to clinical data in order to predict patient outcomes. “It wasn’t until the end of my PhD work that one of my committee members asked: ‘Did you ever check to see how well your model worked across different groups of people?’”
That question was eye-opening for Ghassemi, who had previously assessed the performance of models in aggregate, across all patients. Upon a closer look, she saw that models often worked differently — specifically worse — for populations including Black women, a revelation that took her by surprise. “I hadn’t made the connection beforehand that health disparities would translate directly to model disparities,” she says. “And given that I am a visible minority woman-identifying computer scientist at MIT, I am reasonably certain that many others weren’t aware of this either.”
In a paper published Jan. 14 in the journal Patterns, Ghassemi — who earned her doctorate in 2017 and is now an assistant professor in the Department of Electrical Engineering and Computer Science and the MIT Institute for Medical Engineering and Science (IMES) — and her coauthor, Elaine Okanyene Nsoesie of Boston University, offer a cautionary note about the prospects for AI in medicine. “If used carefully, this technology could improve performance in health care and potentially reduce inequities,” Ghassemi says. “But if we’re not actually careful, technology could worsen care.”
It all comes down to data, given that the AI tools in question train themselves by processing and analyzing vast quantities of data. But the data they are given are produced by humans, who are fallible and whose judgments may be clouded by the fact that they interact differently with patients depending on their age, gender, and race, without even knowing it.
Furthermore, there is still great uncertainty about medical conditions themselves. “Doctors trained at the same medical school for 10 years can, and often do, disagree about a patient’s diagnosis,” Ghassemi says. That’s different from the applications where existing machine-learning algorithms excel — like object-recognition tasks — because practically everyone in the world will agree that a dog is, in fact, a dog.
Machine-learning algorithms have also fared well in mastering games like chess and Go, where both the rules and the “win conditions” are clearly defined. Physicians, however, don’t always concur on the rules for treating patients, and even the win condition of being “healthy” is not widely agreed upon. “Doctors know what it means to be sick,” Ghassemi explains, “and we have the most data for people when they are sickest. But we don’t get much data from people when they are healthy because they’re less likely to see doctors then.”
Even mechanical devices can contribute to flawed data and disparities in treatment. Pulse oximeters, for example, which have been calibrated predominately on light-skinned individuals, do not accurately measure blood oxygen levels for people with darker skin. And these deficiencies are most acute when oxygen levels are low — precisely when accurate readings are most urgent. Similarly, women face increased risks during “metal-on-metal” hip replacements, Ghassemi and Nsoesie write, “due in part to anatomic differences that aren’t taken into account in implant design.” Facts like these could be buried within the data fed to computer models whose output will be undermined as a result.
Coming from computers, the product of machine-learning algorithms offers “the sheen of objectivity,” according to Ghassemi. But that can be deceptive and dangerous, because it’s harder to ferret out the faulty data supplied en masse to a computer than it is to discount the recommendations of a single possibly inept (and maybe even racist) doctor. “The problem is not machine learning itself,” she insists. “It’s people. Human caregivers generate bad data sometimes because they are not perfect.”
Nevertheless, she still believes that machine learning can offer benefits in health care in terms of more efficient and fairer recommendations and practices. One key to realizing the promise of machine learning in health care is to improve the quality of data, which is no easy task. “Imagine if we could take data from doctors that have the best performance and share that with other doctors that have less training and experience,” Ghassemi says. “We really need to collect this data and audit it.”
The challenge here is that the collection of data is not incentivized or rewarded, she notes. “It’s not easy to get a grant for that, or ask students to spend time on it. And data providers might say, ‘Why should I give my data out for free when I can sell it to a company for millions?’ But researchers should be able to access data without having to deal with questions like: ‘What paper will I get my name on in exchange for giving you access to data that sits at my institution?’
“The only way to get better health care is to get better data,” Ghassemi says, “and the only way to get better data is to incentivize its release.”
It’s not only a question of collecting data. There’s also the matter of who will collect it and vet it. Ghassemi recommends assembling diverse groups of researchers — clinicians, statisticians, medical ethicists, and computer scientists — to first gather diverse patient data and then “focus on developing fair and equitable improvements in health care that can be deployed in not just one advanced medical setting, but in a wide range of medical settings.”
The objective of the Patterns paper is not to discourage technologists from bringing their expertise in machine learning to the medical world, she says. “They just need to be cognizant of the gaps that appear in treatment and other complexities that ought to be considered before giving their stamp of approval to a particular computer model.”
2021-22 Takeda Fellows: Leaning on AI to advance medicine for humans
In fall 2020, MIT’s School of Engineering and Takeda Pharmaceuticals Company Limited launched the MIT-Takeda Program, a collaboration to support members of the MIT community working at the intersection of artificial intelligence and human health. Housed at the Abdul Latif Jameel Clinic for Machine Learning in Health, the collaboration aims to use artificial intelligence to both benefit human health and aid in drug development. Combining technology with cutting-edge health research, the program’s participants hope to improve health outcomes across the world.
Thus far, the partnership has supported joint research efforts focused on topics such as automated inspection in sterile pharmaceutical manufacturing and machine learning for liver phenotyping.
Every year, the program also funds graduate fellowships to support students pursuing research on a broad range of issues tied to health and AI. This year’s Takeda fellows, described below, are working on research involving electronic health record algorithms, remote sensing data as it relates to environmental health risk, and neural networks for the development of antibiotics.
Monica Agrawal
Agrawal is a PhD student in the Department of Electrical Engineering and Computer Science (EECS). Her research focuses on the development of machine learning algorithms that could unlock the potential of electronic health records to power personalized, real-world studies of comparative effectiveness. She is tackling the issue from three interconnected angles: understanding the basic building blocks of clinical text, enabling the structuring of clinical timelines with only minimal labeled data, and redesigning clinical documentation to incentivize high-quality structured data at the time of creation. Agrawal earned both a BS and an MS in computer science from Stanford University.
Peng Cao
A PhD student in EECS, Peng Cao’s research is focused on developing a new approach to monitoring oxygen saturation by analyzing the radio frequency signals that bounce off a person’s body. To this end, she is extracting respiration signals from the radio signals and then training a neural network to infer oxygen levels from it. Peng earned a BS in computer science from Peking University in China.
Bianca Lepe
A PhD student in biological engineering, Bianca Lepe is working to benchmark existing and defining next-generation vaccine candidates for tuberculosis. She is using publicly available data combined with machine learning algorithms to identify the Mtb proteins that are well-suited as subunit vaccine antigens across the diversity of the human leukocyte antigen alleles. Lepe earned a BS in biological engineering and business from Caltech; an MS in systems and synthetic biology from the University of Edinburgh in Scotland; and an MPhil in technology policy from the University of Cambridge in England.
Caroline McCue
Caroline McCue is a PhD student in mechanical engineering who is developing a system that could simplify and speed up the process of cell passaging. More specifically, she is designing and testing a platform that triggers cell detachment in response to simple external stimuli, such as a change in voltage or in mechanical properties. She plans to test the efficacy of this platform by applying machine learning to quantify the adhesion of Chinese hamster ovary cells to these surfaces. McCue earned a BS in mechanical engineering from the University of Maryland.
Somesh Mohapatra
A PhD student in the Department of Materials Science and Engineering, Somesh Mohapatra is also pursuing an MBA at the MIT Sloan School of Management as part of the Leaders for Global Operations Program. His doctoral research, in close collaboration with experimentalists at MIT, focuses on designing biomacromolecules using interpretable machine learning and simulations. Specifically, Mohapatra leverages macromolecule graph representations to develop machine learning models for quantitative prediction, optimization, and attribution methods. He then applies these tools to elucidate design principles and to improve performance and synthetic accessibility of functionality macromolecules, ranging from peptides and glycans to electrolytes and thermosets. Mohapatra earned his BTech in metallurgical and materials engineering from the Indian Institute of Technology Roorkee in India.
Luke Murray
Luke Murray is a PhD student in EECS. He is developing MedKnowts, a system that combines machine learning and human computer interaction techniques to reduce the effort required to synthesize knowledge for medical decision-making, and author high-quality, structured, clinical documentation. MedKnowts unifies these two currently splintered workflows by providing a seamless interface that re-imagines documentation as a natural byproduct of clinical reasoning, rather than as a compliance requirement. Murray earned his BS in computer science from Brown University.
Ufuoma Ovienmhada
Ufuoma Ovienmhada SM ’20 is a PhD student in aeronautics and astronautics. Her research employs a mixed-methods approach (community-centered design, systems engineering, and machine learning) to satellite remote sensing data to create tools that evaluate how human health risk relates to environmental hazards. Ovienmhada earned her BS in mechanical engineering from Stanford University and her SM in media arts and sciences from MIT.
Lagnajit Pattanaik
Lagnajit “Lucky” Pattanaik is a PhD student in chemical engineering. He seeks to shift the paradigm of predictive organic chemistry from qualitative to quantitative. More specifically, his research is focused on the development of machine learning techniques for predicting 3D structures of molecules and reactions, including transition state geometries and the geometrical conformations that molecules take in solution. He earned a BS in chemical engineering from Ohio State University.
Na Sun
A PhD student in EECS, Na Sun is working in the emerging field of neuro-immuno-genomics. More specifically, she is developing machine learning methods to better understand the interactions between two extremely complex systems: the human brain and its dozens of cell types, and the human immune system and the dozens of biological processes that it integrates across cognition, pathogen response, diet-exercise-obesity, and synaptic pruning. Sun earned her BS in life sciences from Linyi University in China and an MS in developmental biology from the University of Chinese Academy of Sciences in China.
Jacqueline Valeri
Jacqueline Valeri is a PhD student in biological engineering who utilizes neural networks for antibiotics discovery. Her efforts include the recycling of compounds from existing compound libraries and the computationally assisted design of novel therapeutics. She is also excited by broader applications of machine learning and artificial intelligence in the fields of health care and biomedicine. Valeri earned her BSE and MSE in bioengineering from the University of Pennsylvania.
Clinton Wang
A PhD student in EECS, Clinton Wang SM ’20 has developed a new type of conditional generative adversarial network based on spatial-intensity transforms. It achieves high image fidelity, is robust to artifacts in training data, and generalizes to held-out clinical sites. Wang now aims to extend his model to even more challenging applications, including visualizing transformations of focal pathologies, such as lesions, where it could serve as a powerful tool for characterizing biomarkers of malignancy and treatment response. Wang earned a BS in biomedical engineering from Yale University and an SM in electrical engineering and computer science from MIT.
Advancing AI trustworthiness: Updates on responsible AI research

Editor’s note: This year in review is a sampling of responsible AI research compiled by Aether, a Microsoft cross-company initiative on AI Ethics and Effects in Engineering and Research, as outreach from their commitment to advancing the practice of human-centered responsible AI. Although each paper includes authors who are participants in Aether, the research presented here expands beyond, encompassing work from across Microsoft, as well as with collaborators in academia and industry.
Inflated expectations around the capabilities of AI technologies may lead people to believe that computers can’t be wrong. The truth is AI failures are not a matter of if but when. AI is a human endeavor that combines information about people and the physical world into mathematical constructs. Such technologies typically rely on statistical methods, with the possibility for errors throughout an AI system’s lifespan. As AI systems become more widely used across domains, especially in high-stakes scenarios where people’s safety and wellbeing can be affected, a critical question must be addressed: how trustworthy are AI systems, and how much and when should people trust AI?
-
Explore responsible AI resources
Designed to help you responsibly use AI at every stage of development.
As part of their ongoing commitment to building AI responsibly, research scientists and engineers at Microsoft are pursuing methods and technologies aimed at helping builders of AI systems cultivate appropriate trust—that is, building trustworthy models with reliable behaviors and clear communication that set proper expectations. When AI builders plan for failures, work to understand the nature of the failures, and implement ways to effectively mitigate potential harms, they help engender trust that can lead to a greater realization of AI’s benefits.
Pursuing trustworthiness across AI systems captures the intent of multiple projects on the responsible development and fielding of AI technologies. Numerous efforts at Microsoft have been nurtured by its Aether Committee, a coordinative cross-company council comprised of working groups focused on technical leadership at the frontiers of innovation in responsible AI. The effort is led by researchers and engineers at Microsoft Research and from across the company and is chaired by Chief Scientific Officer Eric Horvitz. Beyond research, Aether has advised Microsoft leadership on responsible AI challenges and opportunities since the committee’s inception in 2016.
-
Explore the HAX Toolkit
The Human-AI eXperience (HAX) Toolkit helps builders of AI systems create fluid, responsible human-AI experiences.
-
Explore the Responsible AI Toolbox
Customizable dashboards that help builders of AI systems identify, diagnose, and mitigate model errors, as well as debug models and understand causal relationships in data.
The following is a sampling of research from the past year representing efforts across the Microsoft responsible AI ecosystem that highlight ways for creating appropriate trust in AI. Facilitating trustworthy measurement, improving human-AI collaboration, designing for natural language processing (NLP), advancing transparency and interpretability, and exploring the open questions around AI safety, security, and privacy are key considerations for developing AI responsibly. The goal of trustworthy AI requires a shift in perspective at every stage of the AI development and deployment life cycle. We’re actively developing a growing number of best practices and tools to help with the shift to make responsible AI more available to a broader base of users. Many open questions remain, but as innovators, we are committed to tackling these challenges with curiosity, enthusiasm, and humility.
Facilitating trustworthy measurement
AI technologies influence the world through the connection of machine learning models—that provide classifications, diagnoses, predictions, and recommendations—with larger systems that drive displays, guide controls, and activate effectors. But when we use AI to help us understand patterns in human behavior and complex societal phenomena, we need to be vigilant. By creating models for assessing or measuring human behavior, we’re participating in the very act of shaping society. Guidelines for ethically navigating technology’s impacts on society—guidance born out of considering technologies for COVID-19—prompt us to start by weighing a project’s risk of harm against its benefits. Sometimes an important step in the practice of responsible AI may be the decision to not build a particular model or application.
Human behavior and algorithms influence each other in feedback loops. In a recent Nature publication, Microsoft researchers and collaborators emphasize that existing methods for measuring social phenomena may not be up to the task of investigating societies where human behavior and algorithms affect each other. They offer five best practices for advancing computational social science. These include developing measurement models that are informed by social theory and that are fair, transparent, interpretable, and privacy preserving. For trustworthy measurement, it’s crucial to document and justify the model’s underlying assumptions, plus consider who is deciding what to measure and how those results will be used.

In line with these best practices, Microsoft researchers and collaborators have proposed measurement modeling as a framework for anticipating and mitigating fairness-related harms caused by AI systems. This framework can help identify mismatches between theoretical understandings of abstract concepts—for example, socioeconomic status—and how these concepts get translated into mathematics and code. Identifying mismatches helps AI practitioners to anticipate and mitigate fairness-related harms that reinforce societal biases and inequities. A study applying a measurement modeling lens to several benchmark datasets for surfacing stereotypes in NLP systems reveals considerable ambiguity and hidden assumptions, demonstrating (among other things) that datasets widely trusted for measuring the presence of stereotyping can, in fact, cause stereotyping harms.
-
VIDEO
Discovering and measuring harms in NLP
Examining pitfalls in state-of-the-art approaches to measuring computational harms in language technologies.
Flaws in datasets can lead to AI systems with unfair outcomes, such as poor quality of service or denial of opportunities and resources for different groups of people. AI practitioners need to understand how their systems are performing for factors like age, race, gender, and socioeconomic status so they can mitigate potential harms. In identifying the decisions that AI practitioners must make when evaluating an AI system’s performance for different groups of people, researchers highlight the importance of rigor in the construction of evaluation datasets.
Making sure that datasets are representative and inclusive means facilitating data collection from different groups of people, including people with disabilities. Mainstream AI systems are often non-inclusive. For example, speech recognition systems do not work for atypical speech, while input devices are not accessible for people with limited mobility. In pursuit of inclusive AI, a study proposes guidelines for designing an accessible online infrastructure for collecting data from people with disabilities, one that is built to respect, protect, and motivate those contributing data.
Related papers
- Responsible computing during COVID-19 and beyond
- Measuring algorithmically infused societies
- Measurement and fairness
- Stereotyping Norwegian salmon: An inventory of pitfalls in fairness benchmark datasets
- Understanding the representation and representativeness of age in AI data sets
- Designing disaggregated evaluations of AI systems: Choices, considerations, and tradeoffs
- Designing an online infrastructure for collecting AI data from People with Disabilities
Improving human-AI collaboration
When people and AI collaborate on solving problems, the benefits can be impressive. But current practice can be far from establishing a successful partnership between people and AI systems. A promising advance and direction of research is developing methods that learn about ideal ways to complement people with problem solving. In the approach, machine learning models are optimized to detect where people need the most help versus where people can solve problems well on their own. We can additionally train the AI systems to make decisions as to when a system should ask an individual for input and to combine the human and machine abilities to make a recommendation. In related work, studies have shown that people will too often accept an AI system’s outputs without question, relying on them even when they are wrong. Exploring how to facilitate appropriate trust in human-AI teamwork, experiments with real-world datasets for AI systems show that retraining a model with a human-centered approach can better optimize human-AI team performance. This means taking into account human accuracy, human effort, the cost of mistakes—and people’s mental models of the AI.
In systems for healthcare and other high-stakes scenarios, a break with the user’s mental model can have severe impacts. An AI system can compromise trust when, after an update for better overall accuracy, it begins to underperform in some areas. For instance, an updated system for predicting cancerous skin moles may have an increase in accuracy overall but a significant decrease for facial moles. A physician using the system may either lose confidence in the benefits of the technology or, with more dire consequences, may not notice this drop in performance. Techniques for forcing an updated system to be compatible with a previous version produce tradeoffs in accuracy. But experiments demonstrate that personalizing objective functions can improve the performance-compatibility tradeoff for specific users by as much as 300 percent.
System updates can have grave consequences when it comes to algorithms used for prescribing recourse, such as how to fix a bad credit score to qualify for a loan. Updates can lead to people who have dutifully followed a prescribed recourse being denied their promised rights or services and damaging their trust in decision makers. Examining the impact of updates caused by changes in the data distribution, researchers expose previously unknown flaws in the current recourse-generation paradigm. This work points toward rethinking how to design these algorithms for robustness and reliability.
Complementarity in human-AI performance, where the human-AI team performs better together by compensating for each other’s weaknesses, is a goal for AI-assisted tasks. You might think that if a system provided an explanation of its output, this could help an individual identify and correct an AI failure, producing the best of human-AI teamwork. Surprisingly, and in contrast to prior work, a large-scale study shows that explanations may not significantly increase human-AI team performance. People often over-rely on recommendations even when the AI is incorrect. This is a call to action: we need to develop methods for communicating explanations that increase users’ understanding rather than to just persuade.
Related papers
- Learning to complement humans
- Is the most accurate AI the best teammate? Optimizing AI for teamwork
- Improving the performance-compatibility tradeoff with personalized objective functions
- Algorithmic recourse in the wild: Understanding the impact of data and model shift
- Does the whole exceed its parts? The effect of AI explanations on complementary team performance
- A Bayesian approach to identifying representational errors
Designing for natural language processing
The allure of natural language processing’s potential, including rash claims of human parity, raises questions of how we can employ NLP technologies in ways that are truly useful, as well as fair and inclusive. To further these and other goals, Microsoft researchers and collaborators hosted the first workshop on bridging human-computer interaction and natural language processing, considering novel questions and research directions for designing NLP systems to align with people’s demonstrated needs.
Language shapes minds and societies. Technology that wields this power requires scrutiny as to what harms may ensue. For example, does an NLP system exacerbate stereotyping? Does it exhibit the same quality of service for people who speak the same language in different ways? A survey of 146 papers analyzing “bias” in NLP observes rampant pitfalls of unstated assumptions and conceptualizations of bias. To avoid these pitfalls, the authors outline recommendations based on the recognition of relationships between language and social hierarchies as fundamentals for fairness in the context of NLP. We must be precise in how we articulate ideas about fairness if we are to identify, measure, and mitigate NLP systems’ potential for fairness-related harms.
-
Launch the HAX Playbook
A low-cost way to proactively identify, design for, and test human-AI interaction failures.
The open-ended nature of language—its inherent ambiguity, context-dependent meaning, and constant evolution—drives home the need to plan for failures when developing NLP systems. Planning for NLP failures with the AI Playbook introduces a new tool for AI practitioners to anticipate errors and plan human-AI interaction so that the user experience is not severely disrupted when errors inevitably occur.
Related papers
- Proceedings of the first workshop on bridging human-computer interaction and natural language processing
- Language (technology) is power: A critical survey of “bias” in NLP
- Planning for natural language failures with the AI Playbook
- Invariant language modeling
- On the relationships between the grammatical genders of inanimate nouns and their co-occurring adjectives and verbs
Improving transparency
To build AI systems that are reliable and fair—and to assess how much to trust them—practitioners and those using these systems need insight into their behavior. If we are to meet the goal of AI transparency, the AI/ML and human-computer interaction communities need to integrate efforts to create human-centered interpretability methods that yield explanations that can be clearly understood and are actionable by people using AI systems in real-world scenarios.
As a case in point, experiments investigating whether simple models that are thought to be interpretable achieve their intended effects rendered counterintuitive findings. When participants used an ML model considered to be interpretable to help them predict the selling prices of New York City apartments, they had difficulty detecting when the model was demonstrably wrong. Providing too many details of the model’s internals seemed to distract and cause information overload. Another recent study found that even when an explanation helps data scientists gain a more nuanced understanding of a model, they may be unwilling to make the effort to understand it if it slows down their workflow too much. As both studies show, testing with users is essential to see if people clearly understand and can use a model’s explanations to their benefit. User research is the only way to validate what is or is not interpretable by people using these systems.
Explanations that are meaningful to people using AI systems are key to the transparency and interpretability of black-box models. Introducing a weight-of-evidence approach to creating machine-generated explanations that are meaningful to people, Microsoft researchers and colleagues highlight the importance of designing explanations with people’s needs in mind and evaluating how people use interpretability tools and what their understanding is of the underlying concepts. The paper also underscores the need to provide well-designed tutorials.
Traceability and communication are also fundamental for demonstrating trustworthiness. Both AI practitioners and people using AI systems benefit from knowing the motivation and composition of datasets. Tools such as datasheets for datasets prompt AI dataset creators to carefully reflect on the process of creation, including any underlying assumptions they are making and potential risks or harms that might arise from the dataset’s use. And for dataset consumers, seeing the dataset creators’ documentation of goals and assumptions equips them to decide whether a dataset is suitable for the task they have in mind.
Related papers
- A human-centered agenda for intelligible machine learning
- Datasheets for datasets
- Manipulating and measuring model interpretability
- From human explanation to model interpretability: A framework based on weight of evidence
- Summarize with caution: Comparing global feature attributions
Advancing algorithms for interpretability
Interpretability is vital to debugging and mitigating the potentially harmful impacts of AI processes that so often take place in seemingly impenetrable black boxes—it is difficult (and in many settings, inappropriate) to trust an AI model if you can’t understand the model and correct it when it is wrong. Advanced glass-box learning algorithms can enable AI practitioners and stakeholders to see what’s “under the hood” and better understand the behavior of AI systems. And advanced user interfaces can make it easier for people using AI systems to understand these models and then edit the models when they find mistakes or bias in them. Interpretability is also important to improve human-AI collaboration—it is difficult for users to interact and collaborate with an AI model or system if they can’t understand it. At Microsoft, we have developed glass-box learning methods that are now as accurate as previous black-box methods but yield AI models that are fully interpretable and editable.
-
VIDEO
Editing GAMs with interactive visualization
Machine learning interpretability techniques reveal that many accurate models learn some problematic and dangerous patterns from the training data. GAM Changer helps address these issues.
Recent advances at Microsoft include a new neural GAM (generalized additive model) for interpretable deep learning, a method for using dropout rates to reduce spurious interaction, an efficient algorithm for recovering identifiable additive models, the development of glass-box models that are differentially private, and the creation of tools that make editing glass-box models easy for those using them so they can correct errors in the models and mitigate bias.
Related papers
- NODE-GAM: Neural generalized additive model for interpretable deep learning
- Dropout as a regularizer of interaction effects
- Purifying interaction effects with the Functional ANOVA: An efficient algorithm for recovering identifiable additive models
- Accuracy, interpretability, and differential privacy via explainable boosting
- GAM changer: Editing generalized additive models with interactive visualization
- Neural additive models: Interpretable machine learning with neural nets
- How interpretable and trustworthy are GAMs?
- Extracting clinician’s goals by What-if interpretable modeling
- Automated interpretable discovery of heterogeneous treatment effectiveness: A Covid-19 case study
Exploring open questions for safety, security, and privacy in AI
When considering how to shape appropriate trust in AI systems, there are many open questions about safety, security, and privacy. How do we stay a step ahead of attackers intent on subverting an AI system or harvesting its proprietary information? How can we avoid a system’s potential for inferring spurious correlations?
With autonomous systems, it is important to acknowledge that no system operating in the real world will ever be complete. It’s impossible to train a system for the many unknowns of the real world. Unintended outcomes can range from annoying to dangerous. For example, a self-driving car may splash pedestrians on a rainy day or erratically swerve to localize itself for lane-keeping. An overview of emerging research in avoiding negative side effects due to AI systems’ incomplete knowledge points to the importance of giving users the means to avoid or mitigate the undesired effects of an AI system’s outputs as essential to how the technology will be viewed or used.
When dealing with data about people and our physical world, privacy considerations take a vast leap in complexity. For example, it’s possible for a malicious actor to isolate and re-identify individuals from information in large, anonymized datasets or from their interactions with online apps when using personal devices. Developments in privacy-preserving techniques face challenges in usability and adoption because of the deeply theoretical nature of concepts like homomorphic encryption, secure multiparty computation, and differential privacy. Exploring the design and governance challenges of privacy-preserving computation, interviews with builders of AI systems, policymakers, and industry leaders reveal confidence that the technology is useful, but the challenge is to bridge the gap from theory to practice in real-world applications. Engaging the human-computer interaction community will be a critical component.
Related papers
Reliability and safety
- Avoiding negative side effects due to incomplete knowledge of AI systems
- Split-treatment analysis to rank heterogeneous causal effects for prospective interventions
- Out-of-distribution prediction with invariant risk minimization: The limitation and an effective fix
- Causal transfer random forest: Combining logged data and randomized experiments for robust prediction
- Understanding failures of deep networks via robust feature extraction
- DoWhy: Addressing challenges in expressing and validating causal assumptions
Privacy and security
- Exploring design and governance challenges in the development of privacy-preserving computation
- Accuracy, interpretability, and differential privacy via explainable boosting
- Labeled PSI from homomorphic encryption with reduced computation and communication
- EVA improved: Compiler and extension library for CKKS
- Aggregate measurement via oblivious shuffling
A call to personal action
AI is not an end-all, be-all solution; it’s a powerful, albeit fallible, set of technologies. The challenge is to maximize the benefits of AI while anticipating and minimizing potential harms.
Admittedly, the goal of appropriate trust is challenging. Developing measurement tools for assessing a world in which algorithms are shaping our behaviors, exposing how systems arrive at decisions, planning for AI failures, and engaging the people on the receiving end of AI systems are important pieces. But what we do know is change can happen today with each one of us as we pause and reflect on our work, asking: what could go wrong, and what can I do to prevent it?
The post Advancing AI trustworthiness: Updates on responsible AI research appeared first on Microsoft Research.
Applying Differential Privacy to Large Scale Image Classification
Machine learning (ML) models are becoming increasingly valuable for improved performance across a variety of consumer products, from recommendations to automatic image classification. However, despite aggregating large amounts of data, in theory it is possible for models to encode characteristics of individual entries from the training set. For example, experiments in controlled settings have shown that language models trained using email datasets may sometimes encode sensitive information included in the training data and may have the potential to reveal the presence of a particular user’s data in the training set. As such, it is important to prevent the encoding of such characteristics from individual training entries. To these ends, researchers are increasingly employing federated learning approaches.
Differential privacy (DP) provides a rigorous mathematical framework that allows researchers to quantify and understand the privacy guarantees of a system or an algorithm. Within the DP framework, privacy guarantees of a system are usually characterized by a positive parameter ε, called the privacy loss bound, with smaller ε corresponding to better privacy. One usually trains a model with DP guarantees using DP-SGD, a specialized training algorithm that provides DP guarantees for the trained model.
However training with DP-SGD typically has two major drawbacks. First, most existing implementations of DP-SGD are inefficient and slow, which makes it hard to use on large datasets. Second, DP-SGD training often significantly impacts utility (such as model accuracy) to the point that models trained with DP-SGD may become unusable in practice. As a result most DP research papers evaluate DP algorithms on very small datasets (MNIST, CIFAR-10, or UCI) and don’t even try to perform evaluation of larger datasets, such as ImageNet.
In “Toward Training at ImageNet Scale with Differential Privacy”, we share initial results from our ongoing effort to train a large image classification model on ImageNet using DP while maintaining high accuracy and minimizing computational cost. We show that the combination of various training techniques, such as careful choice of the model and hyperparameters, large batch training, and transfer learning from other datasets, can significantly boost accuracy of an ImageNet model trained with DP. To substantiate these discoveries and encourage follow-up research, we are also releasing the associated source code.
Testing Differential Privacy on ImageNet
We choose ImageNet classification as a demonstration of the practicality and efficacy of DP because: (1) it is an ambitious task for DP, for which no prior work shows sufficient progress; and (2) it is a public dataset on which other researchers can operate, so it represents an opportunity to collectively improve the utility of real-life DP training. Classification on ImageNet is challenging for DP because it requires large networks with many parameters. This translates into a significant amount of noise added into the computation, because the noise added scales with the size of the model.
Scaling Differential Privacy with JAX
Exploring multiple architectures and training configurations to research what works for DP can be debilitatingly slow. To streamline our efforts, we used JAX, a high-performance computational library based on XLA that can do efficient auto-vectorization and just-in-time compilation of the mathematical computations. Using these JAX features was previously recommended as a good way to speed up DP-SGD in the context of smaller datasets such as CIFAR-10.
We created our own implementation of DP-SGD on JAX and benchmarked it against the large ImageNet dataset (the code is included in our release). The implementation in JAX was relatively simple and resulted in noticeable performance gains simply because of using the XLA compiler. Compared to other implementations of DP-SGD, such as that in Tensorflow Privacy, the JAX implementation is consistently several times faster. It is typically even faster compared to the custom-built and optimized PyTorch Opacus.
Each step of our DP-SGD implementation takes approximately two forward-backward passes through the network. While this is slower than non-private training, which requires only a single forward-backward pass, it is still the most efficient known approach to train with the per-example gradients necessary for DP-SGD. The graph below shows training runtimes for two models on ImageNet with DP-SGD vs. non-private SGD, each on JAX. Overall, we find DP-SGD on JAX sufficiently fast to run large experiments just by slightly reducing the number of training runs used to find optimal hyperparameters compared to non-private training. This is significantly better than alternatives, such as Tensorflow Privacy, which we found to be ~5x–10x slower on our CIFAR10 and MNIST benchmarks.
Time in seconds per training epoch on ImageNet using a Resnet18 or Resnet50 architecture with 8 V100 GPUs. |
Combining Techniques for Improved Accuracy
It is possible that future training algorithms may improve DP’s privacy-utility tradeoff. However, with current algorithms, such as DP-SGD, our experience points to an engineering “bag-of-tricks” approach to make DP more practical on challenging tasks like ImageNet.
Because we can train models faster with JAX, we can iterate quickly and explore multiple configurations to find what works well for DP. We report the following combination of techniques as useful to achieve non-trivial accuracy and privacy on ImageNet:
-
Full-batch training
Theoretically, it is known that larger minibatch sizes improve the utility of DP-SGD, with full-batch training (i.e., where a full dataset is one batch) giving the best utility [1, 2], and empirical results are emerging to support this theory. Indeed, our experiments demonstrate that increasing the batch size along with the number of training epochs leads to a decrease in ε while still maintaining accuracy. However, training with extremely large batches is non-trivial as the batch cannot fit into GPU/TPU memory. So, we employed virtual large-batch training by accumulating gradients for multiple steps before updating the weights instead of applying gradient updates on each training step.
Batch size 1024 4 × 1024 16 × 1024 64 × 1024 Number of epochs 10 40 160 640 Accuracy 56% 57.5% 57.9% 57.2% Privacy loss bound ε 9.8 × 108 6.1 × 107 3.5 × 106 6.7 × 104 -
Transfer learning from public data
Pre-training on public data followed by DP fine-tuning on private data has previously been shown to improve accuracy on other benchmarks [3, 4]. A question that remains is what public data to use for a given task to optimize transfer learning. In this work we simulate a private/public data split by using ImageNet as “private” data and using Places365, another image classification dataset, as a proxy for “public” data. We pre-trained our models on Places365 before fine-tuning them with DP-SGD on ImageNet. Places365 only has images of landscapes and buildings, not of animals as ImageNet, so it is quite different, making it a good candidate to demonstrate the ability of the model to transfer to a different but related domain.
We found that transfer learning from Places365 gave us 47.5% accuracy on ImageNet with a reasonable level of privacy (ε = 10). This is low compared to the 70% accuracy of a similar non-private model, but compared to naïve DP training on ImageNet, which yields either very low accuracy (2 – 5%) or no privacy (ε=109), this is quite good.
Privacy-accuracy tradeoff for Resnet-18 on ImageNet using large-batch training with transfer learning from Places365. |
Next Steps
We hope these early results and source code provide an impetus for other researchers to work on improving DP for ambitious tasks such as ImageNet as a proxy for challenging production-scale tasks. With the much faster DP-SGD on JAX, we urge DP and ML researchers to explore diverse training regimes, model architectures, and algorithms to make DP more practical. To continue advancing the state of the field, we recommend researchers start with a baseline that incorporates full-batch training plus transfer learning.
Acknowledgments
This work was carried out with the support of the Google Visiting Researcher Program while Prof. Geambasu, an Associate Professor with Columbia University, was on sabbatical with Google Research. This work received substantial contributions from Steve Chien, Shuang Song, Andreas Terzis and Abhradeep Guha Thakurta.
Project RADAR: Intelligent Early Fraud Detection System with Humans in the Loop
Introduction
Uber is a worldwide marketplace of services, processing thousands of monetary transactions every second. As a marketplace, Uber takes on all of the risks associated with payment processing. Uber partners who use the marketplace to provide services are paid …
The post Project RADAR: Intelligent Early Fraud Detection System with Humans in the Loop appeared first on Uber Engineering Blog.
Support for New NVIDIA RTX 3080 Ti, 3070 Ti Studio Laptops Now Available in February Studio Driver
Support for the new GeForce RTX 3080 Ti and 3070 Ti Laptop GPUs is available today in the February Studio driver.
Updated monthly, NVIDIA Studio drivers support NVIDIA tools and optimize the most popular creative apps, delivering added performance, reliability and speed to creative workflows.
Creatives will also benefit from the February Studio driver with enhancements to their existing creative apps as well as the latest app releases, including a major update to Maxon’s Redshift renderer.
The NVIDIA Studio platform is being rapidly adopted by aspiring artists, freelancers and creative professionals who seek to take their projects to the next level. The next generation of Studio laptops further powers their ambitions.
Creativity Unleashed — 3080 Ti and 3070 Ti Studio Laptops
Downloading the February Studio driver will help unlock massive time savings, especially for 3080 Ti and 3070 Ti GPU owners, in essential creative apps.
Blender renders are exceptionally fast on GeForce RTX 3080 Ti and 3070 Ti GPUs with RT Cores powering hardware-accelerated ray tracing.
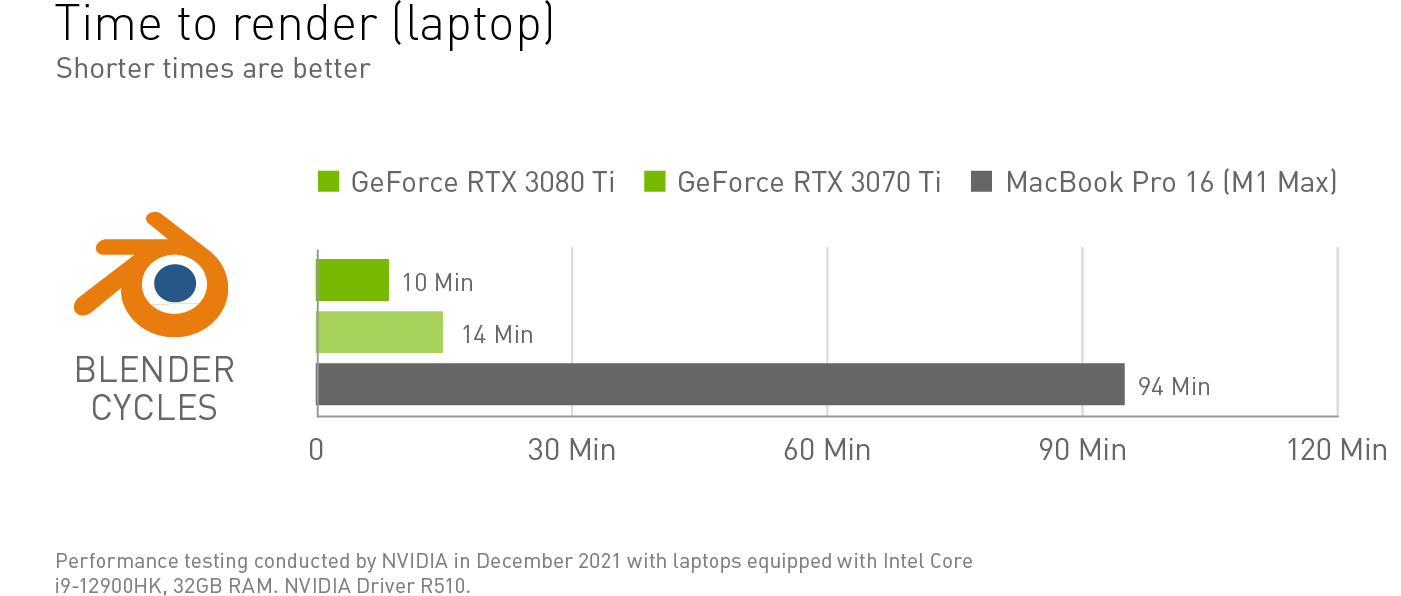
Autodesk aficionados with a GeForce RTX 3080 Ti GPU equipped laptop can render the heaviest of scenes much faster, in this example saving over an hour.
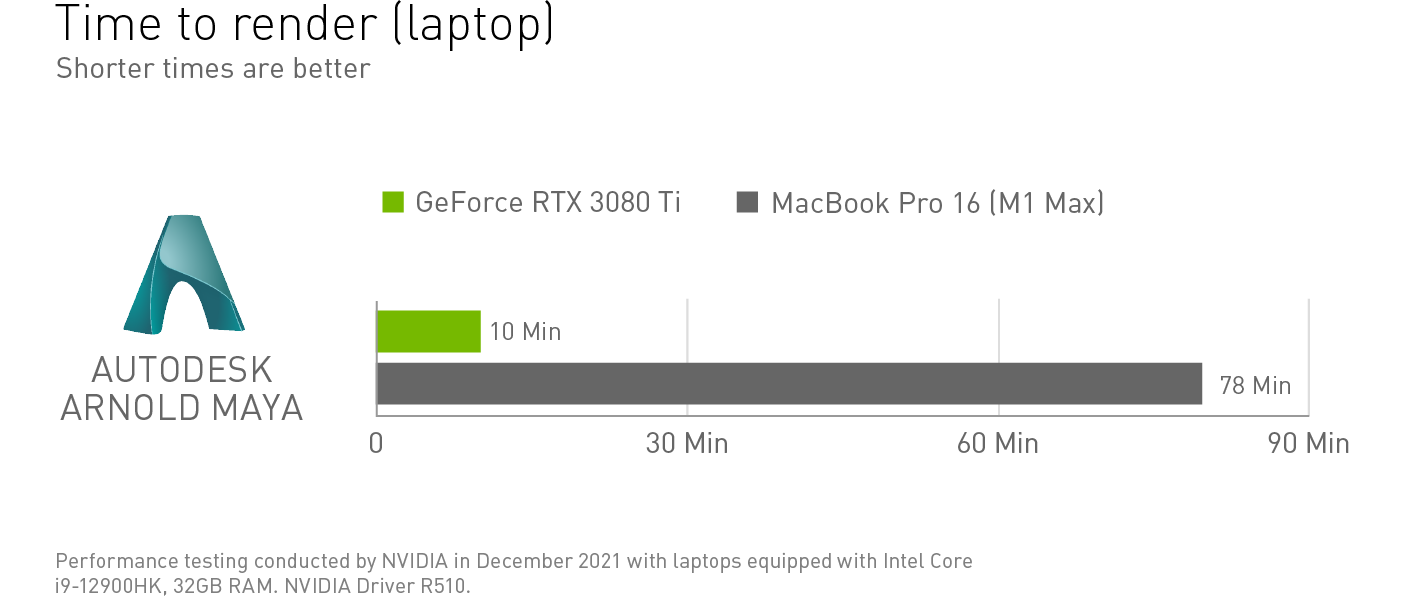
Video production specialists in REDCINE-X PRO have the freedom to edit in real-time with elevated FPS, resulting in more accurate playback, requiring far less time in the editing bay.
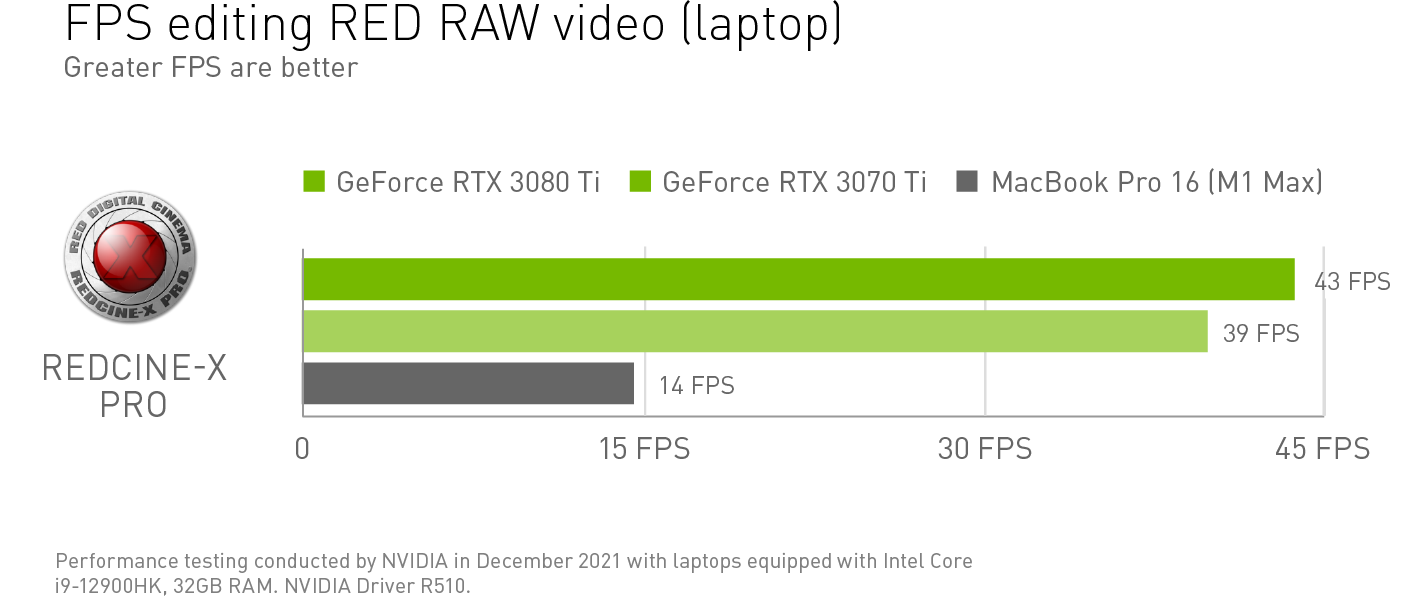
Creators can move at the speed of light with the 2022 lineup of Studio laptops and desktops.
MSI has announced the Creator Z16 and Creator Z17 Studio laptops, set for launch in March, with up to GeForce RTX 3080 Ti Laptop GPUs.

ASUS’s award-winning ZenBook Pro Duo, coming later this year, sports a GeForce RTX 3060 GPU, plus a 15.6-inch 4K UHD OLED touchscreen and secondary 4K screen, unlocking numerous creative possibilities.
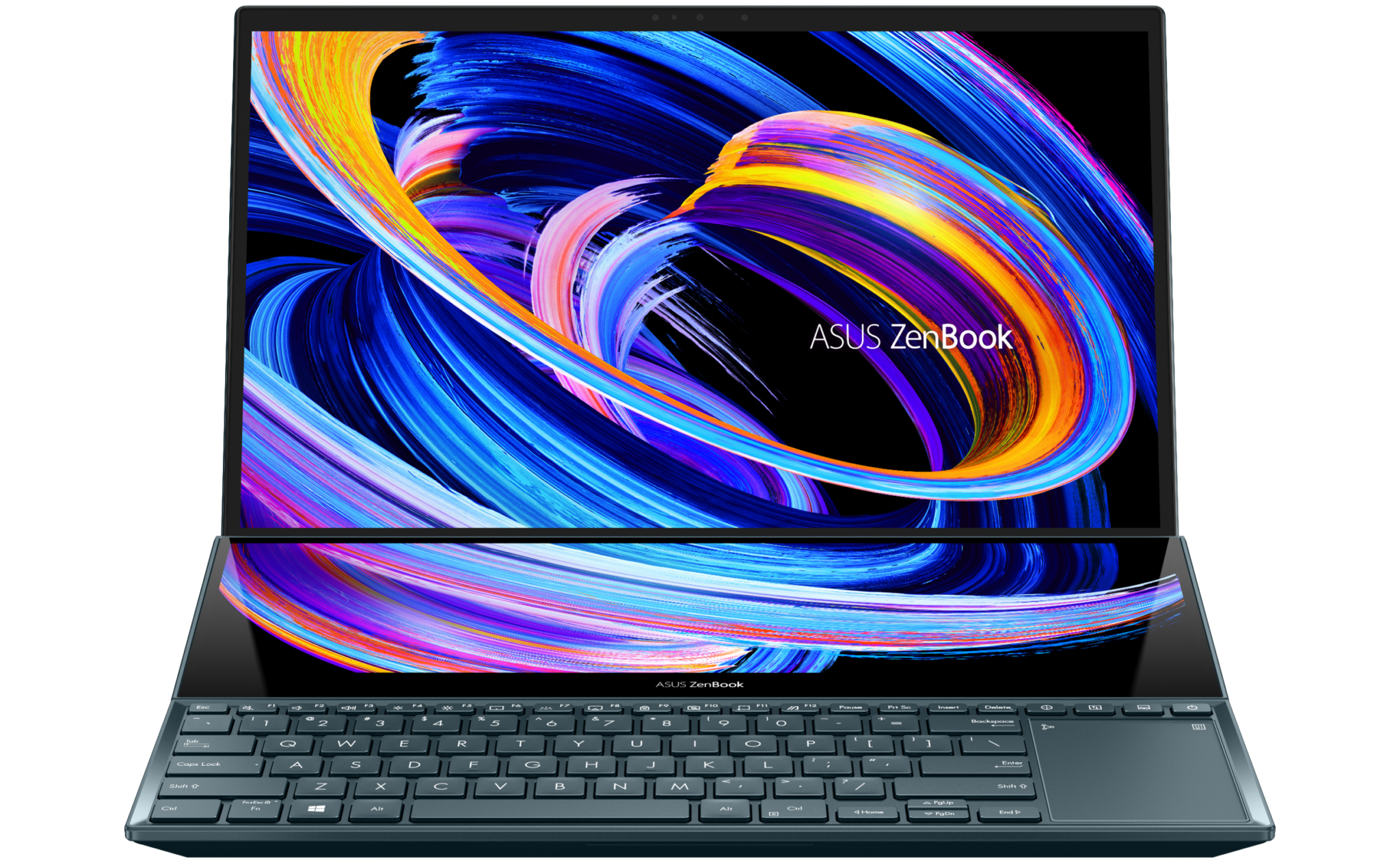
The Razer Blade 17 and 15, available now, come fully loaded with a GeForce RTX 3080 Ti GPU and 32GB of memory — and they’re configurable with a beautiful 4K 144hz, 100-percent DCI-P3 display. Razer Blade 14 will launch on Feb. 17.
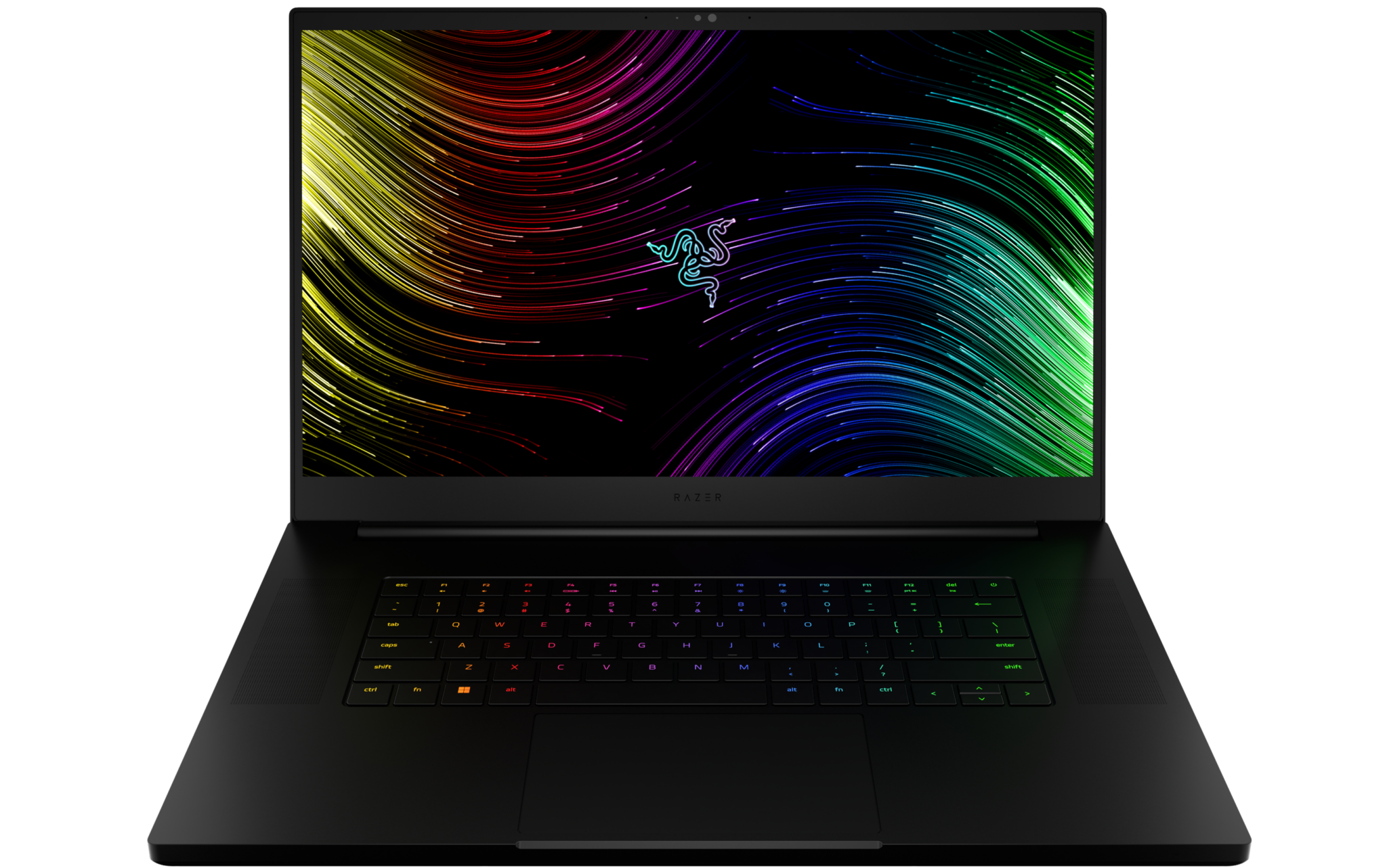
GIGABYTE’s newly refreshed AERO 16 and 17 Studio laptops, equipped with GeForce RTX 3070 Ti and 3080 Ti GPUS, are also now available.
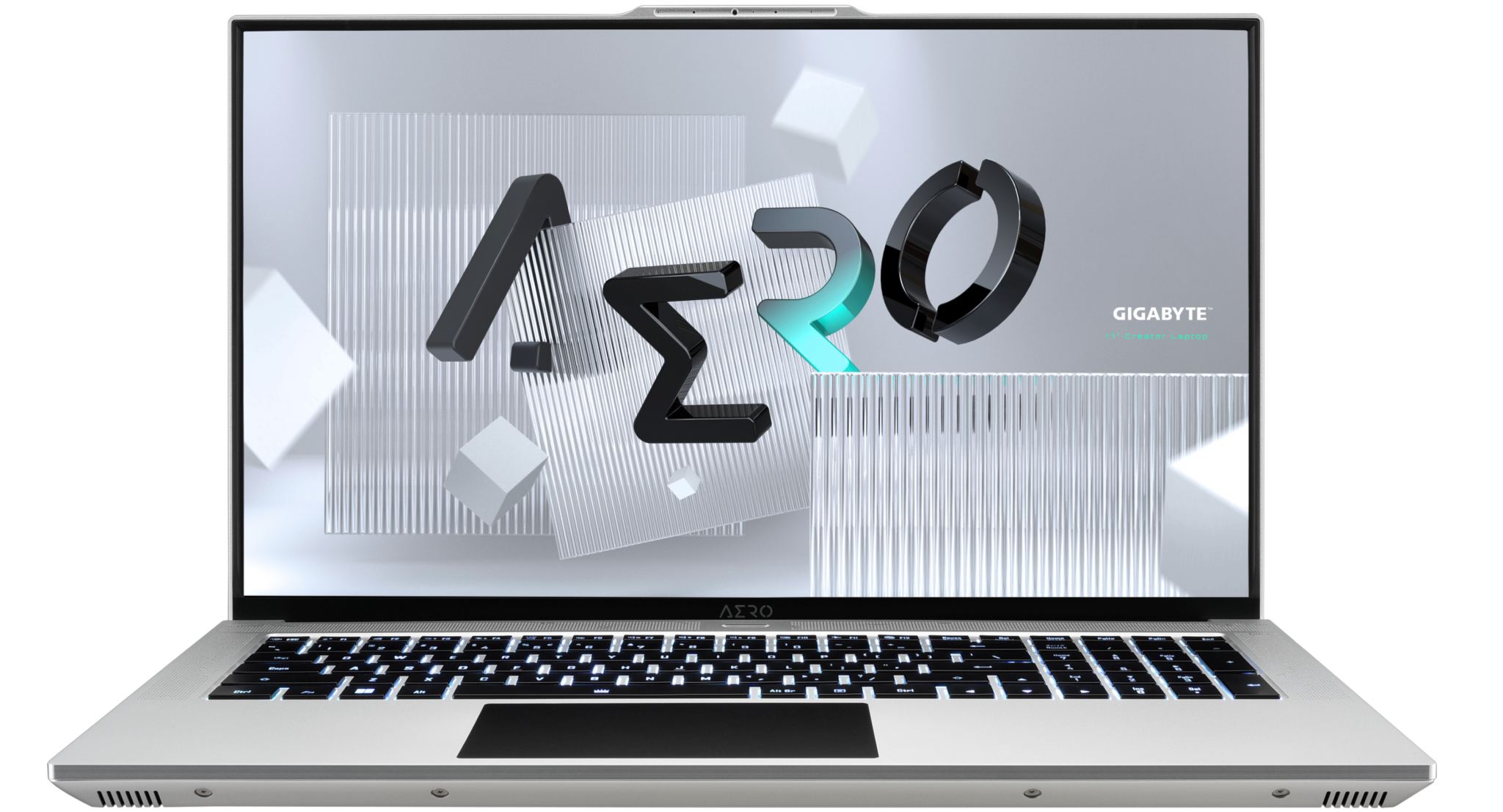
These creative machines power RTX-accelerated tools, including NVIDIA Omniverse, Canvas and Broadcast, making next-generation AI technology even more accessible while reducing and removing tedious work.
Fourth-generation Max-Q technologies — including CPU Optimizer and Rapid Core Scaling — maximize creative performance in remarkably thin laptop designs.
Stay tuned for more Studio product announcements in the coming months.
Shift to Redshift RT
Well-known to 3D artists, Maxon’s Redshift renderer is powerful, biased and GPU accelerated — built to exceed the demands of contemporary high-end production rendering.
Redshift recently launched Redshift RT — a real-time rendering feature — in beta, allowing 3D artists to omit unnecessary wait times for renders to finalize.
Redshift RT runs exclusively on NVIDIA RTX GPUs, bolstered by RT Cores, powering hardware-accelerated, interactive ray tracing.
Redshift RT, which is part of the current release, enables a more natural, intuitive way of working. It offers increased freedom to try different options for creating spectacular content, and is best used for scene editing and rendering previews. Redshift Production remains the highest possible quality and control renderer.
Redshift RT technology is integrated in the Maxon suite of creative apps including Cinema 4D, and is available for Autodesk 3ds Max and Maya, Blender, Foundry Katana and SideFX Houdini, as well as architectural products Vectorworks, Archicad and Allplan, dramatically speeding up all types of visual workflows.
With so many options, now’s the time to take a leap into 3D. Check out our Studio YouTube channel for standouts, tutorials, tips and tricks from industry-leading artists on how to get started.
Get inspired by the latest NVIDIA Studio Standouts video featuring some of our favorite digital art from across the globe.
Follow NVIDIA Studio on Facebook, Twitter and Instagram for the latest information on creative app updates, new Studio apps, creator contests and more. Get updates directly to your inbox by subscribing to the Studio newsletter.
The post Support for New NVIDIA RTX 3080 Ti, 3070 Ti Studio Laptops Now Available in February Studio Driver appeared first on The Official NVIDIA Blog.
How to Improve User Experience (and Behavior): Three Papers from Stanford’s Alexa Prize Team
Introduction
In 2019, Stanford entered the Alexa Prize Socialbot Grand Challenge 3 for the first time, with its bot Chirpy Cardinal, which went on to win 2nd place in the competition. In our previous post, we discussed the technical structure of our socialbot and how developers can use our open-source code to develop their own. In this post we share further research conducted while developing Chirpy Cardinal to discover common pain points that users encounter when interacting with socialbots, and strategies for addressing them.
The Alexa Prize is a unique research setting, as it allows researchers to study how users interact with a bot when doing so solely for their own motivations. During the competition, US-based Alexa users can say the phrase “let’s chat” to speak in English to an anonymous and randomly-selected competing bot. They are free to end the conversation at any time. Since Alexa Prize socialbots are intended to create as natural an experience as possible, they should be capable of long, open-domain social conversations with high coverage of topics. We observed that Chirpy users were interested in many different subjects, from current events (e.g., the coronavirus) to pop culture (e.g., the movie Frozen 2) to personal interests (e.g,. their pets). Chirpy achieves its coverage of these diverse topics by using a modular design that combines both neural generation and scripted dialogue, as described in our previous post.
We used this setting to study three questions about socialbot conversations:
- What do users complain about, and how can we learn from the complaints to improve neurally generated dialogue?
- What strategies are effective and ineffective in handling and deterring offensive user behavior?
- How can we shift the balance of power, such that both users and the bot are meaningfully controlling the conversation?
We’ve published papers on each of these topics at SIGDIAL 2021 and in this post, we’ll share key findings which provide practical insights for both chatbot researchers and developers.
1. Understanding and Predicting User Dissatisfaction
paper | video |
Neural generative dialogue models like DialoGPT1, Meena2, and BlenderBot3 use large pretrained neural language models to generate responses given a dialogue history. These models perform well when evaluated by crowdworkers in carefully-controlled settings–typically written conversations with certain topical or length constraints.
However, real-life settings like the Alexa Prize are not so tidy. Users have widely varying expectations and personalities, and require fast response times as they speak with the bot in home environments that might feature cross-talk and background noise. Through Chirpy Cardinal, we have a unique opportunity to investigate how modern neural generative dialogue models hold up in this kind of environment.
Chirpy Cardinal uses a GPT2-medium model fine-tuned on the EmpatheticDialogues4 dataset to hold short discussions with users about their everyday experiences and emotions. Particularly during the pandemic, we found it was important for Chirpy to ask users about these issues. Though larger and more powerful pretrained generative models are available, we used GPT2-medium due to budget and latency constraints.
While the GPT2-medium model is capable of chatting about these simple topics for a few utterances, discussions that extend longer tend to derail. Sooner or later, the bot gives a response that doesn’t quite make sense, and it’s hard for the user or the model to recover the conversation.
To understand how these conversations are derailing, we defined 7 types of errors made by the neural generative model – repetition, redundant questions, unclear utterances, hallucination, ignoring, logical errors, and insulting utterances. After annotating a sample of user conversations, we found that bot errors were common, with over half (53%) of neural-generated utterances containing some kind of error.
We also found that due to the challenging noisy environment (which may involve background noise, cross-talk, and ASR errors), almost a quarter (22%) of user utterances were incomprehensible, even to a human annotator. This accounts for some of the more basic bot errors, such as ignoring, hallucination, unclear and repetitive utterances.
Of the remaining bot errors, redundant questions and logical errors are particularly common, indicating that better reasoning and use of the conversational history are a priority for neural generative model development.
We also tracked 9 ways that users express dissatisfaction, such as asking for clarification, criticising the bot, and ending the conversation. Though there is a relationship between bot errors and user dissatisfaction, the correlation is noisy. Even after a bot error, many users do not express dissatisfaction, instead attempting to continue the conversation. This is particularly true after logical errors, in which the bot shows a lack of real-world knowledge or commonsense – some kind-hearted users even take this as an opportunity to educate the bot. Conversely, some users express dissatisfaction unrelated to any obvious bot error – for example, users have widely differing expectations regarding what kinds of personal questions are appropriate from the bot.
Having better understood how and why users express dissatisfaction, we asked: can we learn to predict dissatisfaction, and thus prevent it before it happens?
With the user conversations collected during the competition, we trained a model to predict the probability that a certain bot utterance would lead the user to express dissatisfaction. Given the noisy correlation between bot errors and user dissatisfaction, this is inherently challenging. Despite this noise, our predictor model was able to find signal in the users’ dissatisfaction.
Once trained, our dissatisfaction predictor can be used mid-conversation to choose between multiple alternative neural-generated bot utterances. Through human evaluation, we found that the bot responses chosen by the predictor – i.e., those judged least likely to cause user dissatisfaction – are overall better quality than randomly chosen responses.
Though we have not yet incorporated this feedback loop into Chirpy Cardinal, our method demonstrates one viable way to implement a semi-supervised online learning method to continuously improve a neural generative dialogue system.
2. Handling Offensive Users
paper | video |
Voice assistants are becoming increasingly popular, and with their popularity, they are subject to growing abuse from their user populations. We estimate that more than 10% of user conversations with our bot, Chirpy Cardinal, contain profanity and overtly offensive language. While there is a large body of prior work attempting to address this issue, most prior approaches use qualitative metrics based on surveys conducted in lab settings. In this work, we conduct a large-scale quantitative evaluation of response strategies against offensive users in-the-wild. In our experiments, we found that politely rejecting the user’s offense while redirecting the user to an alternative topic is the best strategy in curbing offenses.
Informed by prior work, we test the following 4 hypotheses:
- Redirect – Inspired by Brahnam5, we hypothesize that using explicit redirection when responding to an offensive user utterance is an effective strategy. For example, “I’d rather not talk about that. So, who’s your favorite musician?”
- Name – Inspired by Suler6 and Chen and Williams7, we hypothesize that including the user’s name in the bot’s response is an effective strategy. For example, “I’d rather not talk about that, Peter.”
- Why – Inspired by Shapiro et al.8, we hypothesize that politely asking the user the reason why they made an offensive remark invites them to reflect on their behavior, reducing future offenses. For example, “Why would you say that?”
- Empathetic & Counter – Inspired by Chin et al.9, we hypothesize that empathetic responses are more effective than generic avoidance responses, while counter-attack responses make no difference. For example, an empathetic response would be “If I could talk about it I would, but I really can’t. Sorry to disappoint”, and a counter-attack response would be “That’s a very suggestive thing to say. I don’t think we should be talking about that.”
We constructed the responses crossing multiple factors listed above. For example, avoidance + name + redirect would yield the utterance “I’d rather not talk about that (avoidance), Peter (name). So, who’s your favorite musician? (redirect)”
To measure the effectiveness of a response strategy, we propose 3 metrics:
- Re-offense – measured as the number of conversations that contained another offensive utterance after the initial bot response.
- End – measured as the length of the conversation after bot response assuming no future offenses.
- Next – measured as the number of turns passed until the user offends again.
We believe that these metrics measure the effectiveness of a response strategy more directly than user ratings as done in Cohn et al.10 which measure the overall quality of the conversation.
The figure above shows the differences of strategies on the Re-offense ratio. As we can see, strategies with (redirects) performed significantly better than strategies without redirects, reducing re-offense rate by as much as 53%. Our pairwise hypothesis tests further shows that using user’s name with a redirect further reduces re-offense rate by about 6%, and that asking the user why they made an offensive remark had a 3% increase in re-offense rate which shows that asking the user why only invites user re-offenses instead of self-reflection. Empathetic responses also reduced re-offense rate by 3%, while counter responses did not have any significant effect.
The figure on the left shows the differences in average number of turns until the next re-offense (Next), and the figure on the right shows the differences in average number of turns until the end of the conversation (End). We again see that strategies with (redirects are able to significantly prolong a non-offensive conversation. This further shows that redirection is incredibly effective method to curb user offenses.
The main takeaway from this is that the bot should always empathetically respond to user offenses with a redirection, and use the user’s name whenever possible.
Despite the empirical effectiveness of the passive avoidance and redirection strategy, we would like to remind researchers of the societal dangers of adopting similar strategies. Since most voice-based agents have a default female voice, these strategies could further gender stereotypes and set unreasonable expectations of how women would react to verbal abuse in the real world 11 12 13. Thus, caution must be taken when deploying these strategies.
3. Increasing User Initiative
paper | video |
Conversations are either controlled by the user (for example, bots such as Apple’s Siri, which passively waits for user commands) or the bot (for example, CVS’s customer service bot, which repeatedly prompts the user for specific pieces of information).
This property – which agent has control at a given moment – is called initiative.
It wouldn’t be fun to go to a cocktail party and have a single person choose every topic, never giving you the opportunity to share your own interests. It’s also tedious to talk to someone who forces you to carry the conversation by refusing to bring up their own subjects. Ideally, everyone would take turns responding to prompts, sharing information about themselves, and introducing new topics. We call this pattern of dialogue mixed initiative and hypothesize that just as it’s an enjoyable type of human-human social conversation, it’s also a more engaging and desirable form of human-bot dialogue.
We designed our bot, Chirpy Cardinal, to keep conversations moving forward by asking questions on every turn. Although this helped prevent conversations from stagnating, it also made it difficult for users to take initiative. In our data, we observe users complaining about this, with comments such as you ask too many questions, or that’s not what I wanted to talk about.
Since our goal in studying initiative was to make human-bot conversations more like human-human ones, we looked to research on human dialogue for inspiration.
Based on this research, we formed three hypotheses for how to increase user initiative.
The images below show the types of utterances we experimented with as well as representative user utterances. Per Alexa Prize competition rules, these are not actual user utterances received by our bot.
1. Giving statements instead of questions
In human dialogue research 14, the person asking a question has initiative, since they are giving a direction that the person answering follows. By contrast, an open-ended statement gives the listener an opportunity to take initiative. This was the basis of our first strategy: using statements instead of questions.
2. Sharing personal information
Work on both human-human 15 and human-bot 16 dialogue has found that personal self disclosure has a reciprocal effect. If one participant shares about themself, then the other person is more likely to do the same. We hypothesized that if Chirpy gave personal statements rather than general ones, then users would take initiative and reciprocate.
The figure on the left is an example of a conversation with back-channeling, the right, without. In this case, back-channeling allows the user to direct the conversation towards what they want (getting suggestions) rather than forcing them to talk about something they’re not interested in (hobbies).
3. Introducing back-channeling
Back-channels, such as “hmm”, “I see”, and “mm-hmm”, are brief utterances which are used as a signal from the listener to the speaker that the speaker should continue taking initiative. Our final hypothesis was that they could be used in human-bot conversation to the same effect, i.e. that if our bot back-channeled, then the user would direct the conversation.
Experiments and results
To test these strategies, we altered different components of our bot. We conducted small experiments, only altering a single turn of conversation, to test questions vs statements and personal vs general statements. To test the effect of replacing statements with questions on a larger number of turns, we altered components of our bot that used neurally generated dialogue, since these were more flexible to changing user inputs. Finally, we experimented with back-channeling in a fully neural module of our bot.
Using a set of automated metrics, which we validated using manual annotations, we found the following results, which provide direction for future conversational design:
- Using statements alone outperformed questions or combined statements and questions
- Giving personal opinion statements (e.g. “I like Bojack Horseman”) was more effective than both personal experience statements (e.g. “I watched Bojack Horseman yesterday”) and general statements (e.g. “Bojack Horseman was created by Raphael Bob-Waksberg and Lisa Hanawalt”)
- As the number of questions decreased, user initiative increased
- User initiative was greatest when we back-channeled 33% of the time (as opposed to 0%, 66%, or 100%)
Since these experiments were conducted in a limited environment, we do not expect that they would transfer perfectly to all social bots; however, we believe that these simple yet effective strategies are a promising direction for building more natural conversational AI.
4. Listen with empathy
Each of our projects began with dissatisfied users who told us, in their own words, what our bot could do better. By conducting a systematic analysis of these complaints, we gained a more precise understanding of what specifically was bothering users about our neurally generated responses. Using this feedback, we trained a model which was able to successfully predict when a generated response might lead the conversation astray. At times, it was the users who would make an offensive statement. We studied these cases and determined that an empathetic redirection, which incorporated the users name, was most effective at keeping the conversation on track. Finally, we experimented with simply saying less and creating greater opportunities for the user to lead the conversation. When presented with that chance, many took it, leading to longer and more informative dialogues.
Across all of our work, the intuitive principles of human conversation apply to socialbots: be a good listener, respond with empathy, and when you’re given feedback and the opportunity to learn, take it.
-
Zhang, Yizhe, Siqi Sun, Michel Galley, Yen-Chun Chen, Chris Brockett, Xiang Gao, Jianfeng Gao, Jingjing Liu, and Bill Dolan. Dialogpt: Large-scale generative pre-training for conversational response generation](https://www.google.com/url?q=https://arxiv.org/abs/1911.00536&sa=D&source=editors&ust=1643077986262380&usg=AOvVaw1khQv7HglJrP1gK8dkiE3n).” arXiv preprint arXiv:1911.00536 (2019). ↩
-
Adiwardana, Daniel, Minh-Thang Luong, David R. So, Jamie Hall, Noah Fiedel, Romal Thoppilan, Zi Yang et al. Towards a human-like open-domain chatbot arXiv preprint arXiv:2001.09977 (2020). ↩
-
Roller, Stephen, Emily Dinan, Naman Goyal, Da Ju, Mary Williamson, Yinhan Liu, Jing Xu et al. Recipes for building an open-domain chatbot arXiv preprint arXiv:2004.13637 (2020). ↩
-
Hannah Raskin, Eric Michael Smith, Margaret Li, and Y-Lan Boureau. 2019. Towards empathetic open-domain conversation models: A new benchmark and dataset. In Proceedings of the 57th Annual Meeting of the Association for Computational Linguistics, pages 5370-5381, Florence, Italy. Association for Computational Linguistics. ↩
-
Sheryl Brahnam. 2005. Strategies for handling cus- tomer abuse of ECAs. In Proc. Interact 2005 work- shop Abuse: The darker side of Human-Computer Interaction, pages 62–67. ↩
-
John Suler. 2004. The online disinhibition effect. Cyberpsychology & behavior, 7(3):321–326. ↩
-
Xiangyu Chen and Andrew Williams. 2020. Improving Engagement by Letting Social Robots Learn and Call Your Name. In Companion of the 2020 ACM/IEEE International Conference on Human-Robot Interaction, HRI ’20, page 160–162, New York, NY, USA. Association for Computing Machinery. ↩
-
Shauna Shapiro, Kristen Lyons, Richard Miller, Britta Butler, Cassandra Vieten, and Philip Zelazo. 2014. Contemplation in the Classroom: a New Direction for Improving Childhood Education. Educational Psychology Review, 27. ↩
-
Hyojin Chin, Lebogang Wame Molefi, and Mun Yong Yi. 2020. Empathy Is All You Need: How a Conversational Agent Should Sespond to Verbal Abuse. In Proceedings of the 2020 CHI Conference on Human Factors in Computing Systems, pages 1–13. ↩
-
Michelle Cohn, Chun-Yen Chen, and Zhou Yu. 2019. A large-scale user study of an Alexa Prize chatbot: Effect of TTS dynamism on perceived quality of social dialog. In Proceedings of the 20th Annual SIGdial Meeting on Discourse and Dialogue, pages 293– 306, Stockholm, Sweden. Association for Computational Linguistics. ↩
-
Amanda Cercas Curry and Verena Rieser. 2019. A crowd-based evaluation of abuse response strategies in conversational agents. In Proceedings of the 20th Annual SIGdial Meeting on Discourse and Dialogue, pages 361–366, Stockholm, Sweden. Association for Computational Linguistics. ↩
-
Mark West, Rebecca Kraut, and Han Ei Chew. 2019. I’d blush if i could: closing gender divides in digital skills through education. ↩
-
Amanda Cercas Curry, Judy Robertson, and Verena Rieser. 2020. Conversational assistants and gender stereotypes: Public perceptions and desiderata for voice personas. In Proceedings of the Second Work- shop on Gender Bias in Natural Language Processing, pages 72–78, Barcelona, Spain (Online). Association for Computational Linguistics. ↩
-
Marilyn Walker and Steve Whittaker. 1990. Mixed initiative in dialogue: An investigation into discourse segmentation. In Proceedings of the 28th Annual Meeting on Association for Computational Linguistics, ACL ’90, page 70–78, USA. Association for Computational Linguistics. ↩
-
Nancy Collins and Lynn Miller. 1994. Self-disclosure and liking: A meta-analytic review. Psychological bulletin, 116:457–75. ↩
-
Yi-Chieh Lee, Naomi Yamashita, Yun Huang, and Wai Fu. 2020. “I hear you, I feel you”: Encouraging deep self-disclosure through a chatbot. In Proceedings of the 2020 CHI Conference on Human Factors in Computing Systems, CHI ’20, page 1–12, New York, NY, USA. Association for Computing Machinery. ↩
Artificial intelligence system rapidly predicts how two proteins will attach
Antibodies, small proteins produced by the immune system, can attach to specific parts of a virus to neutralize it. As scientists continue to battle SARS-CoV-2, the virus that causes Covid-19, one possible weapon is a synthetic antibody that binds with the virus’ spike proteins to prevent the virus from entering a human cell.
To develop a successful synthetic antibody, researchers must understand exactly how that attachment will happen. Proteins, with lumpy 3D structures containing many folds, can stick together in millions of combinations, so finding the right protein complex among almost countless candidates is extremely time-consuming.
To streamline the process, MIT researchers created a machine-learning model that can directly predict the complex that will form when two proteins bind together. Their technique is between 80 and 500 times faster than state-of-the-art software methods, and often predicts protein structures that are closer to actual structures that have been observed experimentally.
This technique could help scientists better understand some biological processes that involve protein interactions, like DNA replication and repair; it could also speed up the process of developing new medicines.
“Deep learning is very good at capturing interactions between different proteins that are otherwise difficult for chemists or biologists to write experimentally. Some of these interactions are very complicated, and people haven’t found good ways to express them. This deep-learning model can learn these types of interactions from data,” says Octavian-Eugen Ganea, a postdoc in the MIT Computer Science and Artificial Intelligence Laboratory (CSAIL) and co-lead author of the paper.
Ganea’s co-lead author is Xinyuan Huang, a graduate student at ETH Zurich. MIT co-authors include Regina Barzilay, the School of Engineering Distinguished Professor for AI and Health in CSAIL, and Tommi Jaakkola, the Thomas Siebel Professor of Electrical Engineering in CSAIL and a member of the Institute for Data, Systems, and Society. The research will be presented at the International Conference on Learning Representations.
Protein attachment
The model the researchers developed, called Equidock, focuses on rigid body docking — which occurs when two proteins attach by rotating or translating in 3D space, but their shapes don’t squeeze or bend.
The model takes the 3D structures of two proteins and converts those structures into 3D graphs that can be processed by the neural network. Proteins are formed from chains of amino acids, and each of those amino acids is represented by a node in the graph.
The researchers incorporated geometric knowledge into the model, so it understands how objects can change if they are rotated or translated in 3D space. The model also has mathematical knowledge built in that ensures the proteins always attach in the same way, no matter where they exist in 3D space. This is how proteins dock in the human body.
Using this information, the machine-learning system identifies atoms of the two proteins that are most likely to interact and form chemical reactions, known as binding-pocket points. Then it uses these points to place the two proteins together into a complex.
“If we can understand from the proteins which individual parts are likely to be these binding pocket points, then that will capture all the information we need to place the two proteins together. Assuming we can find these two sets of points, then we can just find out how to rotate and translate the proteins so one set matches the other set,” Ganea explains.
One of the biggest challenges of building this model was overcoming the lack of training data. Because so little experimental 3D data for proteins exist, it was especially important to incorporate geometric knowledge into Equidock, Ganea says. Without those geometric constraints, the model might pick up false correlations in the dataset.
Seconds vs. hours
Once the model was trained, the researchers compared it to four software methods. Equidock is able to predict the final protein complex after only one to five seconds. All the baselines took much longer, from between 10 minutes to an hour or more.
In quality measures, which calculate how closely the predicted protein complex matches the actual protein complex, Equidock was often comparable with the baselines, but it sometimes underperformed them.
“We are still lagging behind one of the baselines. Our method can still be improved, and it can still be useful. It could be used in a very large virtual screening where we want to understand how thousands of proteins can interact and form complexes. Our method could be used to generate an initial set of candidates very fast, and then these could be fine-tuned with some of the more accurate, but slower, traditional methods,” he says.
In addition to using this method with traditional models, the team wants to incorporate specific atomic interactions into Equidock so it can make more accurate predictions. For instance, sometimes atoms in proteins will attach through hydrophobic interactions, which involve water molecules.
Their technique could also be applied to the development of small, drug-like molecules, Ganea says. These molecules bind with protein surfaces in specific ways, so rapidly determining how that attachment occurs could shorten the drug development timeline.
In the future, they plan to enhance Equidock so it can make predictions for flexible protein docking. The biggest hurdle there is a lack of data for training, so Ganea and his colleagues are working to generate synthetic data they could use to improve the model.
This work was funded, in part, by the Machine Learning for Pharmaceutical Discovery and Synthesis consortium, the Swiss National Science Foundation, the Abdul Latif Jameel Clinic for Machine Learning in Health, the DTRA Discovery of Medical Countermeasures Against New and Emerging (DOMANE) threats program, and the DARPA Accelerated Molecular Discovery program.