Editor’s note: This post is part of our weekly In the NVIDIA Studio series, which celebrates featured artists, offers creative tips and tricks, and demonstrates how NVIDIA Studio technology improves creative workflows. We’re also deep diving on new GeForce RTX 40 Series GPU features, technologies and resources, and how they dramatically accelerate content creation.
NVIDIA Studio is debuting at CES powerful new software and hardware upgrades to elevate content creation.
It brings the release of powerful NVIDIA Studio laptops and desktops from Acer, ASUS, Dell, HP, Lenovo, MSI and Samsung, as well as the launch of the new GeForce RTX 40 SUPER Series GPUs — including the GeForce RTX 4080 SUPER, GeForce RTX 4070 Ti SUPER and GeForce RTX 4070 SUPER — to supercharge creating, gaming and AI tasks.
Generative AI by iStock from Getty Images is a new generative AI tool trained by NVIDIA Picasso that uses licensed artwork and the NVIDIA Edify architecture model to ensure that generated assets are commercially safe.
RTX Video HDR coming Jan. 24 transforms standard dynamic range video playing in internet browsers into stunning high dynamic range (HDR). By pairing it with RTX Video Super Resolution, NVIDIA RTX and GeForce RTX GPU owners can achieve dramatic video quality improvements on their HDR10 displays.
Twitch, OBS and NVIDIA are enhancing livestreaming technology with the new Twitch Enhanced Broadcasting beta, powered by GeForce RTX GPUs. Available later this month, the beta will enable users to stream multiple encodes concurrently, providing optimal viewing experiences for a broad range of device types and connections.
And NVIDIA RTX Remix — a free modding platform for quickly remastering classic games with RTX — releases in open beta later this month. It provides full ray tracing, NVIDIA DLSS, NVIDIA Reflex and generative AI texture tools.
This week’s In the NVIDIA Studio installment also features NVIDIA artists Ashlee Martino-Tarr, a 3D content specialist, and Daniela Flamm Jackson, a technical product marketer, who transform 2D illustrations into dynamic 3D scenes using AI and Adobe Firefly — powered by NVIDIA in the cloud and natively with GeForce RTX GPUs.
New Year, New NVIDIA Studio Laptops
The new NVIDIA Studio laptops and desktops level up power and efficiency with exclusive software like Studio Drivers preinstalled — enhancing creative features, reducing time-consuming tasks and speeding workflows.
The Acer Predator Triton Neo 16 features several 16-inch screen options with up to a 3.2K resolution at a 165Hz refresh rate and 16:10 aspect ratio. It provides DCI-P3 100% color gamut and support for NVIDIA Optimus and NVIDIA G-SYNC technology for sharp color hues and tear-free frames. It’s expected to be released in March.
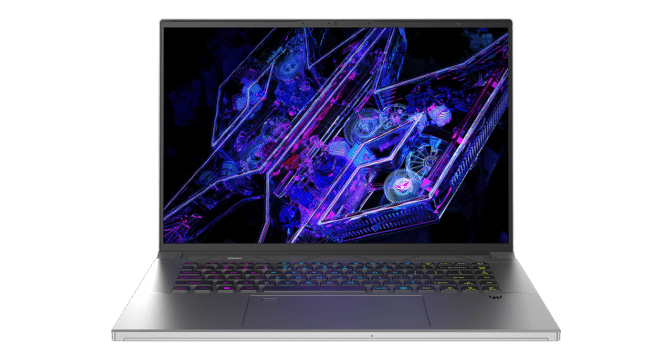
The ASUS ROG Zephryus G14 features a Nebula Display with a OLED panel and a G-SYNC OLED display running at 240Hz. It’s expected to release on Feb. 6.
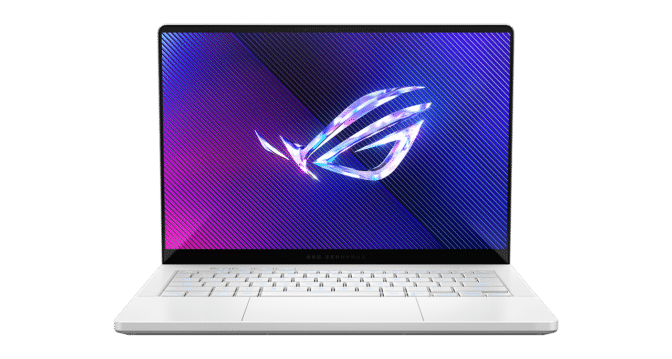
The XPS 16 is Dell’s most powerful laptop featuring a large 16.3” InfinityEdge display, available with a 4K+ OLED touch display, true-to-life color delivering up to 80W of sustained performance, all with tone-on-tone finishes for an elegant, minimalistic design. Stay tuned for an update on release timing.
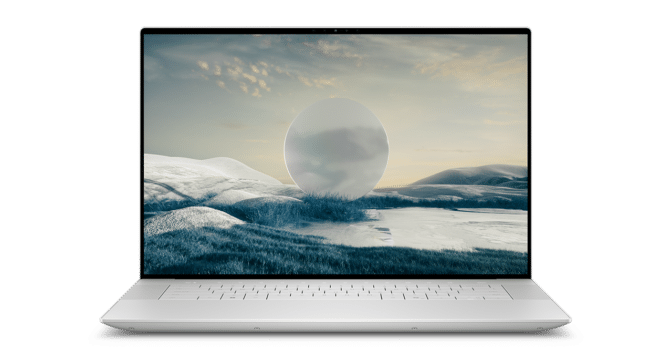
Lenovo’s Yoga Pro 9i sports a 16-inch 3.2K PureSight Pro display, delivering a grid of over 1,600 mini-LED dimming zones, expertly calibrated colors accurate to Delta E< 1 and up to 165Hz. With Microsoft’s Auto Color Management feature, its display toggles automatically between 100% P3, 100% sRGB and 100% Adobe RGB color to ensure the highest-quality color. It’s expected to be released in April.
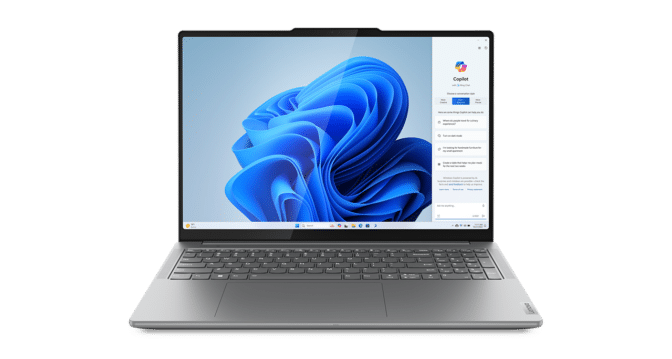
HP’s OMEN 14 Transcend features a 14-inch 4K OLED WQXGA screen, micro-edge, edge-to-edge glass and 100% DCI-P3 with a 240Hz refresh rate. NVIDIA DLSS 3 technology helps unlock more efficient content creation and gaming sessions using only one-third of the expected battery power. It’s targeting a Jan. 19 release.
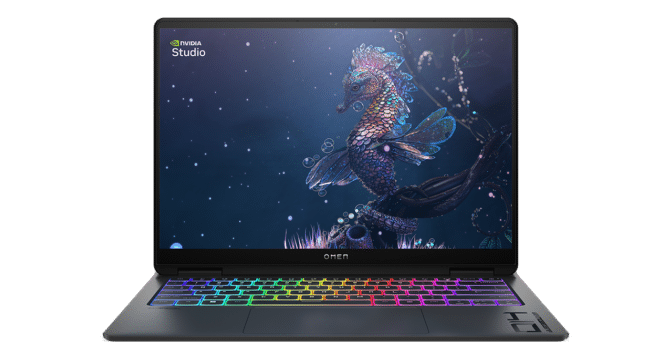
Samsung’s Galaxy Book4 Ultra includes an upgraded Dynamic AMOLED 2X display for high contrast and vivid color, as well as a convenient touchscreen. Its Vision Booster feature uses an Intelligent Outdoor Algorithm to automatically enhance visibility and color reproduction in bright conditions.
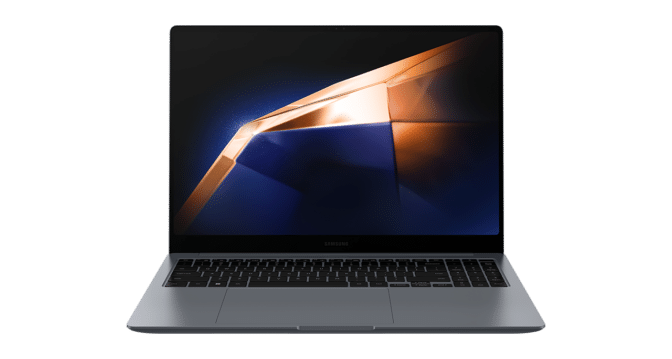
Check back for more information on the new line of Studio systems, including updates to release dates.
A SUPER Debut for New GeForce RTX 40 Series Graphics Cards
The GeForce RTX 40 Series has been supercharged with the new GeForce RTX 4080 SUPER, GeForce RTX 4070 Ti SUPER and GeForce RTX 4070 SUPER graphics cards. This trio is faster than its predecessors, with RTX platform superpowers that enhance creating, gaming and AI tasks.
The GeForce RTX 4080 SUPER sports more CUDA cores than the GeForce RTX 4080 and includes the world’s fastest GDDR6X video memory at 23 Gbps. In 3D apps like Blender, it can run up to 70% faster than previous generations. In generative AI apps like Stable Diffusion XL or Stable Video Diffusion, it can produce 1,024×1,024 images 1.7x faster and video 1.5x faster. Or play fully ray-traced games, including Alan Wake 2, Cyberpunk 2077: Phantom Liberty and Portal with RTX, in stunning 4K. The RTX 4080 SUPER will be available Jan. 31 as a Founders Edition and as custom boards for partners starting at $999.
The GeForce RTX 4070 Ti SUPER is equipped with more CUDA cores than the RTX 4070, a frame buffer increased to 16GB, and a 256-bit bus. It’s suited for video editing and rendering large 3D scenes and runs up to 1.6x faster than the RTX 3070 Ti and 2.5x faster with DLSS 3 in the most graphics-intensive games. Gamers can max out high-refresh 1440p panels or even game at 4K. The RTX 4070 Ti SUPER will be available Jan. 24 from custom board partners in stock-clocked and factory-overclocked configurations starting at $799.
The GeForce RTX 4070 SUPER has 20% more CUDA cores than the GeForce RTX 4070 and is great for 1440p creating. With DLSS 3, it’s 1.5x faster than a GeForce RTX 3090 while using a fraction of the power.
Read more on the GeForce article.
Creative Vision Meets Reality With Getty Images and NVIDIA
Content creators using the new Generative AI by iStock from Getty Images tool powered by NVIDIA Picasso can now safely, affordably use AI-generated images with full protection.
Generative AI by iStock is trained on Getty Images’ vast creative library of high-quality licensed content, including millions of exclusive photos, illustrations and videos. Users can enter prompts to generate photo-quality images at up to 4K for social media promotion, digital advertisements and more.
Getty Images is also making advanced inpainting and outpainting features available via application programming interfaces. Developers can seamlessly integrate the new APIs with creative applications to add people and objects to images, replace specific elements and expand images to a wide range of aspect ratios.
Customers can use Generative AI by iStock online today. Advanced editing features are coming soon to the iStock website.
RTX Video HDR Brings AI Video Upgrades
RTX Video HDR brings a new AI-enhanced feature that instantly converts any standard dynamic range video playing in internet browsers into vibrant HDR.
HDR delivers stunning video quality but is not widely available because of effort and hardware limitations.
RTX Video HDR allows NVIDIA RTX and GeForce RTX GPU owners to maximize their HDR panel’s ability to display more vivid, dynamic colors, helping preserve intricate details that may be lost in standard dynamic range.
The feature requires an HDR10-compatible display or TV connected to a RTX-powered PC and works with Chromium-based browsers such as Google Chrome or Microsoft Edge.
RTX Video HDR and RTX Video Super Resolution can be used together to produce the clearest livestreamed video.
RTX Video HDR is coming to all NVIDIA RTX and GeForce RTX GPUs as part of a driver update later this month. Once the update goes through, navigate to the NVIDIA control panel and switch it on.
Enhanced Broadcasting Beta Enables Multi-Encode Livestreaming
With Twitch Enhanced Broadcasting beta, GeForce RTX GPU owners will be able to broadcast up to three resolutions simultaneously at up to 1080p. In the coming months, Twitch plans to roll out support for up to five concurrent encodes to further optimize viewer experiences.
As part of the beta, Twitch will test higher input bit rates as well as new codecs, which are expected to further improve visual quality. The new codecs include the latest-generation AV1 for GeForce RTX 40 Series GPUs, which provides 40% more encoding efficiency than H.264, and HEVC for previous-generation GeForce GPUs.
To simplify the setup process, Enhanced Broadcasting will automatically configure all open broadcaster software encoder settings, including resolution, bit rate and encoding parameters.
Sign up for the Twitch Enhanced Broadcasting beta today.
A Righteous RTX Remix
Built on NVIDIA Omniverse, RTX Remix allows modders to easily capture game assets, automatically enhance materials with generative AI tools, reimagine assets via Omniverse-connected apps and Universal Scene Description (OpenUSD), and quickly create stunning RTX remasters of classic games with full ray tracing and NVIDIA DLSS technology.
The RTX Remix open beta releases later this month.
RTX Remix has already delivered stunning remasters in Portal with RTX and the modder-made Portal: Prelude RTX. Now, Orbifold Studios is using RTX Remix to develop Half-Life 2 RTX: An RTX Remix Project, a community remaster of one of the highest-rated games of all time. Check out the new Half-Life 2 RTX gameplay trailer, showcasing Orbifold Studios’ latest updates to Ravenholm:
AI and RTX Bring Illustrations to Life
NVIDIA artists and this week’s In the NVIDIA Studio features Ashlee Martino-Tarr and Daniela Flamm Jackson are passionate about illustration — whether in work or at play.
They used Adobe Firefly’s generative AI features, powered by NVIDIA GPUs in the cloud and accelerated with Tensor Cores in GeForce RTX GPUs, to animate a 2D illustration with special effects.
To begin, the pair separated the 2D image into multiple layers and expanded the canvas. Firefly’s Generative Expand feature automatically filled the added space with AI-generated content.
Next, the team separated select elements — starting with character — and used the AI Object Select feature to automatically mask the layer. The Generative Fill feature then created new content to fill in the background, saving even more time.
This process continued until all distinct layers were separated and imported into Adobe After Effects. Next, they used the Mercury 3D Engine on local RTX GPUs to accelerate playback, unlocking smoother movement in the viewport. Previews and adjustments like camera shake and depth of field were also GPU-accelerated.
Firefly’s Style Match feature then took the existing illustration and created new imagery in its likeness — in this case, a vibrant butterfly sporting similar colors and tones. The duo also used Adobe Illustrator’s Generative Recolor feature, which enables artists to explore a wide variety of colors and themes without having to manually recolor their work.
Martino-Tarr and Jackson then chose their preferred assets and animated them in Adobe After Effects. Firefly’s powerful AI effects helped speed or entirely eliminate tedious tasks such as patching holes, handpainting set extensions and caching animation playbacks.
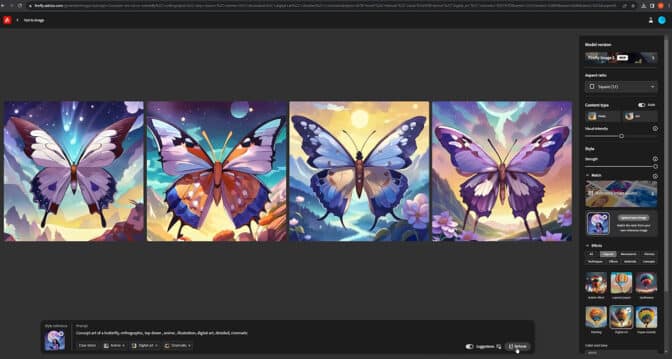
The artists concluded post-production work by putting the finishing touches on their AI animation in After Effects.
Firefly’s powerful AI capabilities were developed with the creative community in mind — guided by AI ethics principles of content and data transparency — to ensure morally responsible output. NVIDIA technology continues to power these features from the cloud for photographers, illustrators, designers, video editors, 3D artists and more.
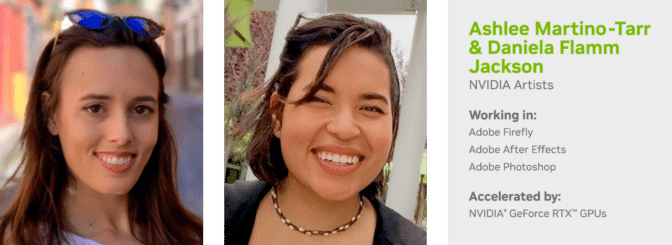
Check out Martino-Tarr’s portfolio on ArtStation and Jackson’s on IMDb.
Follow NVIDIA Studio on Instagram, Twitter and Facebook. Access tutorials on the Studio YouTube channel and get updates directly in your inbox by subscribing to the Studio newsletter.