Brands today are juggling a million things, and keeping product content up-to-date is at the top of the list. Between decoding the endless requirements of different marketplaces, wrangling inventory across channels, adjusting product listings to catch a customer’s eye, and trying to outpace shifting trends and fierce competition, it’s a lot. And let’s face it—staying ahead of the ecommerce game can feel like running on a treadmill that just keeps speeding up. For many, it results in missed opportunities and revenue that doesn’t quite hit the mark.
“Managing a diverse range of products and retailers is so challenging due to the varying content requirements, imagery, different languages for different regions, formatting and even the target audiences that they serve.”
– Martin Ruiz, Content Specialist, Kanto
Pattern is a leader in ecommerce acceleration, helping brands navigate the complexities of selling on marketplaces and achieve profitable growth through a combination of proprietary technology and on-demand expertise. Pattern was founded in 2013 and has expanded to over 1,700 team members in 22 global locations, addressing the growing need for specialized ecommerce expertise.
Pattern has over 38 trillion proprietary ecommerce data points, 12 tech patents and patents pending, and deep marketplace expertise. Pattern partners with hundreds of brands, like Nestle and Philips, to drive revenue growth. As the top third-party seller on Amazon, Pattern uses this expertise to optimize product listings, manage inventory, and boost brand presence across multiple services simultaneously.
In this post, we share how Pattern uses AWS services to process trillions of data points to deliver actionable insights, optimizing product listings across multiple services.
Content Brief: Data-backed content optimization for product listings
Pattern’s latest innovation, Content Brief, is a powerful AI-driven tool designed to help brands optimize their product listings and accelerate growth across online marketplaces. Using Pattern’s dataset of over 38 trillion ecommerce data points, Content Brief provides actionable insights and recommendations to create standout product content that drives traffic and conversions.
Content Brief analyzes consumer demographics, discovery behavior, and content performance to give brands a comprehensive understanding of their product’s position in the marketplace. What would normally require months of research and work is now done in minutes. Content Brief takes the guesswork out of product strategy with tools that do the heavy lifting. Its attribute importance ranking shows you which product features deserve the spotlight, and the image archetype analysis makes sure your visuals engage customers.
As shown in the following screenshot, the image archetype feature shows attributes that are driving sales in a given category, allowing brands to highlight the most impactful features in the image block and A+ image content.
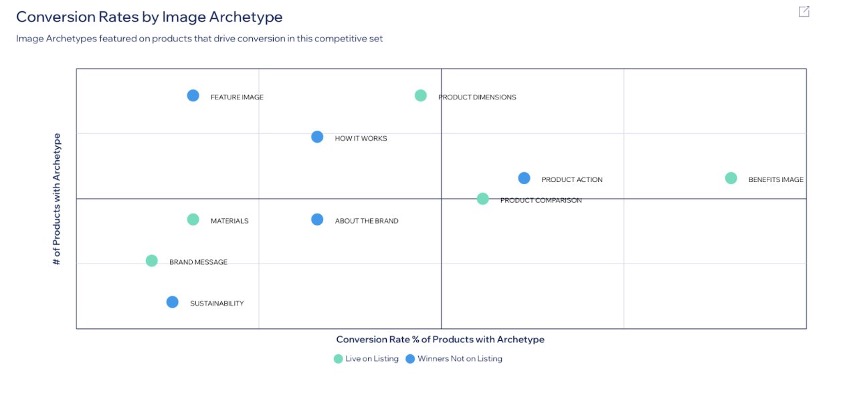
Content Brief incorporates review and feedback analysis capabilities. It uses sentiment analysis to process customer reviews, identifying recurring themes in both positive and negative feedback, and highlights areas for potential improvement.
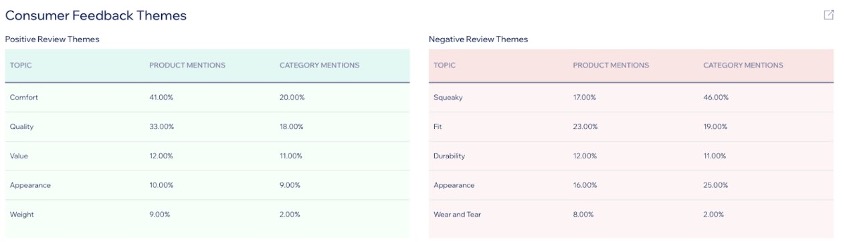
Content Brief’s Search Family analysis groups similar search terms together, helping brands understand distinct customer intent and tailor their content accordingly. This feature combined with detailed persona insights helps marketers create highly targeted content for specific segments. It also offers competitive analysis, providing side-by-side comparisons with competing products, highlighting areas where a brand’s product stands out or needs improvement.
“This is the thing we need the most as a business. We have all of the listening tools, review sentiment, keyword things, but nothing is in a single place like this and able to be optimized to my listing. And the thought of writing all those changes back to my PIM and then syndicating to all of my retailers, this is giving me goosebumps.”
– Marketing executive, Fortune 500 brand
Brands using Content Brief can more quickly identify opportunities for growth, adapt to change, and maintain a competitive edge in the digital marketplace. From search optimization and review analysis to competitive benchmarking and persona targeting, Content Brief empowers brands to create compelling, data-driven content that drives both traffic and conversions.
Select Brands looked to improve their Amazon performance and partnered with Pattern. Content Brief’s insights led to updates that caused a transformation for their Triple Buffet Server listing’s image stack. Their old image stack was created for marketplace requirements, whereas the new image stack was optimized with insights based on product attributes to highlight from category and sales data. The updated image stack featured bold product highlights and captured shoppers with lifestyle imagery. The results were a 21% MoM revenue surge, 14.5% more traffic, and a 21 bps conversion lift.
“Content Brief is a perfect example of why we chose to partner with Pattern. After just one month of testing, we see how impactful it can be for driving incremental growth—even on products that are already performing well. We have a product that, together with Pattern, we were able to grow into a top performer in its category in less than 2 years, and it’s exciting to see how adding this additional layer can grow revenue even for that product, which we already considered to be strong.”
– Eric Endres, President, Select Brands
To discover how Content Brief helped Select Brands boost their Amazon performance, refer to the full case study.
The AWS backbone of Content Brief
At the heart of Pattern’s architecture lies a carefully orchestrated suite of AWS services. Amazon Simple Storage Service (Amazon S3) serves as the cornerstone for storing product images, crucial for comprehensive ecommerce analysis. Amazon Textract is employed to extract and analyze text from these images, providing valuable insights into product presentation and enabling comparisons with competitor listings. Meanwhile, Amazon DynamoDB acts as the powerhouse behind Content Brief’s rapid data retrieval and processing capabilities, storing both structured and unstructured data, including content brief object blobs.
Pattern’s approach to data management is both innovative and efficient. As data is processed and analyzed, they create a shell in DynamoDB for each content brief, progressively injecting data as it’s processed and refined. This method allows for rapid access to partial results and enables further data transformations as needed, making sure that brands have access to the most up-to-date insights.
The following diagram illustrates the pipeline workflow and architecture.
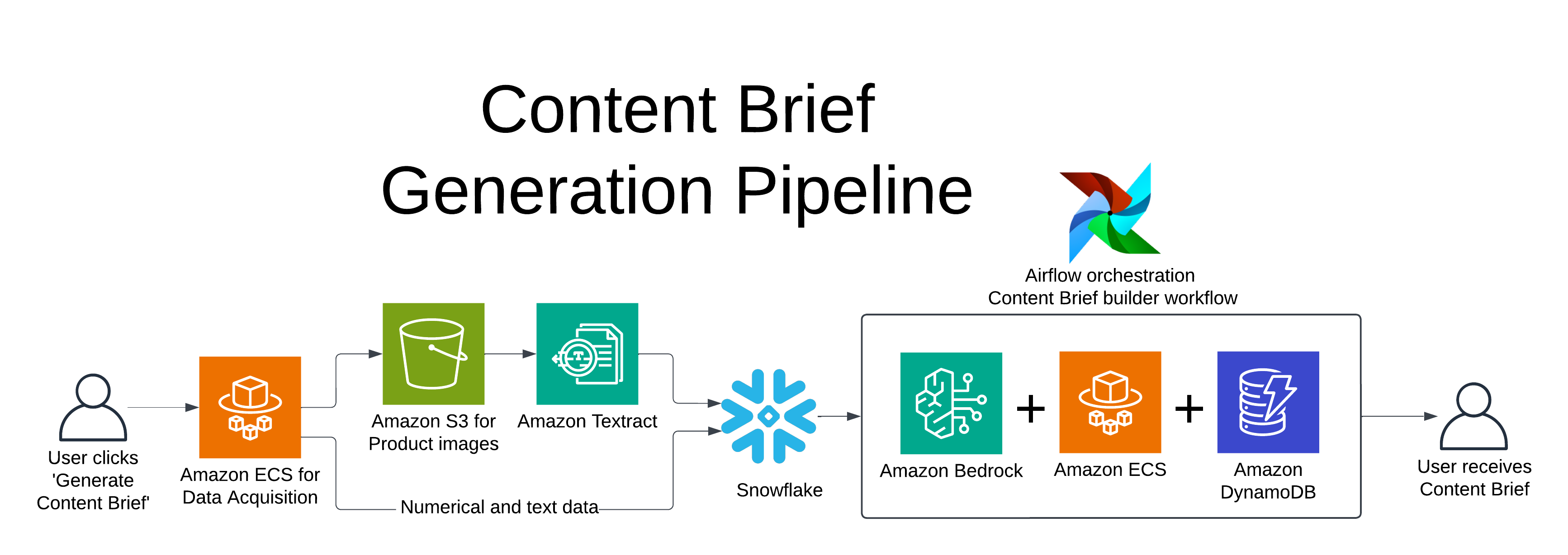
Scaling to handle 38 trillion data points
Processing over 38 trillion data points is no small feat, but Pattern has risen to the challenge with a sophisticated scaling strategy. At the core of this strategy is Amazon Elastic Container Store (Amazon ECS) with GPU support, which handles the computationally intensive tasks of natural language processing and data science. This setup allows Pattern to dynamically scale resources based on demand, providing optimal performance even during peak processing times.
To manage the complex flow of data between various AWS services, Pattern employs Apache Airflow. This orchestration tool manages the intricate dance of data with a primary DAG, creating and managing numerous sub-DAGs as needed. This innovative use of Airflow allows Pattern to efficiently manage complex, interdependent data processing tasks at scale.
But scaling isn’t just about processing power—it’s also about efficiency. Pattern has implemented batching techniques in their AI model calls, resulting in up to 50% cost reduction for two-batch processing while maintaining high throughput. They’ve also implemented cross-region inference to improve scalability and reliability across different geographical areas.
To keep a watchful eye on their system’s performance, Pattern employs LLM observability techniques. They monitor AI model performance and behavior, enabling continuous system optimization and making sure that Content Brief is operating at peak efficiency.
Using Amazon Bedrock for AI-powered insights
A key component of Pattern’s Content Brief solution is Amazon Bedrock, which plays a pivotal role in their AI and machine learning (ML) capabilities. Pattern uses Amazon Bedrock to implement a flexible and secure large language model (LLM) strategy.
Model flexibility and optimization
Amazon Bedrock offers support for multiple foundation models (FMs), which allows Pattern to dynamically select the most appropriate model for each specific task. This flexibility is crucial for optimizing performance across various aspects of Content Brief:
- Natural language processing – For analyzing product descriptions, Pattern uses models optimized for language understanding and generation.
- Sentiment analysis – When processing customer reviews, Amazon Bedrock enables the use of models fine-tuned for sentiment classification.
- Image analysis – Pattern currently uses Amazon Textract for extracting text from product images. However, Amazon Bedrock also offers advanced vision-language models that could potentially enhance image analysis capabilities in the future, such as detailed object recognition or visual sentiment analysis.
The ability to rapidly prototype on different LLMs is a key component of Pattern’s AI strategy. Amazon Bedrock offers quick access to a variety of cutting-edge models o facilitate this process, allowing Pattern to continuously evolve Content Brief and use the latest advancements in AI technology. Today, this allows the team to build seamless integration and use various state-of-the-art language models tailored to different tasks, including the new, cost-effective Amazon Nova models.
Prompt engineering and efficiency
Pattern’s team has developed a sophisticated prompt engineering process, continually refining their prompts to optimize both quality and efficiency. Amazon Bedrock offers support for custom prompts, which allows Pattern to tailor the model’s behavior precisely to their needs, improving the accuracy and relevance of AI-generated insights.
Moreover, Amazon Bedrock offers efficient inference capabilities that help Pattern optimize token usage, reducing costs while maintaining high-quality outputs. This efficiency is crucial when processing the vast amounts of data required for comprehensive ecommerce analysis.
Security and data privacy
Pattern uses the built-in security features of Amazon Bedrock to uphold data protection and compliance. By employing AWS PrivateLink, data transfers between Pattern’s virtual private cloud (VPC) and Amazon Bedrock occur over private IP addresses, never traversing the public internet. This approach significantly enhances security by reducing exposure to potential threats.
Furthermore, the Amazon Bedrock architecture makes sure that Pattern’s data remains within their AWS account throughout the inference process. This data isolation provides an additional layer of security and helps maintain compliance with data protection regulations.
“Amazon Bedrock’s flexibility is crucial in the ever-evolving landscape of AI, enabling Pattern to utilize the most effective and efficient models for their diverse ecommerce analysis needs. The service’s robust security features and data isolation capabilities give us peace of mind, knowing that our data and our clients’ information are protected throughout the AI inference process.”
– Jason Wells, CTO, Pattern
Building on Amazon Bedrock, Pattern has created a secure, flexible, and efficient AI-powered solution that continuously evolves to meet the dynamic needs of ecommerce optimization.
Conclusion
Pattern’s Content Brief demonstrates the power of AWS in revolutionizing data-driven solutions. By using services like Amazon Bedrock, DynamoDB, and Amazon ECS, Pattern processes over 38 trillion data points to deliver actionable insights, optimizing product listings across multiple services.
Inspired to build your own innovative, high-performance solution? Explore AWS’s suite of services at aws.amazon.com and discover how you can harness the cloud to bring your ideas to life. To learn more about how Content Brief could help your brand optimize its ecommerce presence, visit pattern.com.
About the Author
Parker Bradshaw is an Enterprise SA at AWS who focuses on storage and data technologies. He helps retail companies manage large data sets to boost customer experience and product quality. Parker is passionate about innovation and building technical communities. In his free time, he enjoys family activities and playing pickleball.
Read More