Today at Google Cloud Next we announced updates and new tools for Gemini in Workspace.To start, we’re bringing audio capabilities directly into Google Docs so you’ll be …Read More
Check out the latest features from Google Agentspace at Google Cloud Next.
Today at Google Cloud Next, we’re announcing even more features in Google Agentspace to make creating and adopting agents simpler. Starting today, customers can give emp…Read More
New video, image, speech and music generative AI tools are coming to Vertex AI.
Today at Google Cloud Next, we announced four big updates for generative media within Vertex AI, Google Cloud’s fully-managed, unified AI development platform:Lyria, Goo…Read More
Google Cloud Next 25
Here’s a look at what we announced at Google Cloud Next 25.Read More
Here’s what’s new with our Google Cloud AI Hypercomputer.
Our AI Hypercomputer underpins our Cloud customers’ most demanding AI workloads. Its hardware and software layers are optimized to deliver more intelligence per dollar f…Read More
TiC-LM: A Web-Scale Benchmark for Time-Continual LLM Pretraining
This paper was accepted at the Scalable Continual Learning for Lifelong Foundation Models (SCLLFM) Workshop at NeurIPS 2024.
Large Language Models (LLMs) trained on historical web data inevitably become outdated. We investigate evaluation strategies and update methods for LLMs as new data becomes available. We introduce a web-scale dataset for time-continual pretraining of LLMs derived from 114 dumps of Common Crawl (CC) – orders of magnitude larger than previous continual language modeling benchmarks. We also design time-stratified evaluations across both general CC data and specific domains…Apple Machine Learning Research
How iFood built a platform to run hundreds of machine learning models with Amazon SageMaker Inference
Headquartered in São Paulo, Brazil, iFood is a national private company and the leader in food-tech in Latin America, processing millions of orders monthly. iFood has stood out for its strategy of incorporating cutting-edge technology into its operations. With the support of AWS, iFood has developed a robust machine learning (ML) inference infrastructure, using services such as Amazon SageMaker to efficiently create and deploy ML models. This partnership has allowed iFood not only to optimize its internal processes, but also to offer innovative solutions to its delivery partners and restaurants.
iFood’s ML platform comprises a set of tools, processes, and workflows developed with the following objectives:
- Accelerate the development and training of AI/ML models, making them more reliable and reproducible
- Make sure that deploying these models to production is reliable, scalable, and traceable
- Facilitate the testing, monitoring, and evaluation of models in production in a transparent, accessible, and standardized manner
To achieve these objectives, iFood uses SageMaker, which simplifies the training and deployment of models. Additionally, the integration of SageMaker features in iFood’s infrastructure automates critical processes, such as generating training datasets, training models, deploying models to production, and continuously monitoring their performance.
In this post, we show how iFood uses SageMaker to revolutionize its ML operations. By harnessing the power of SageMaker, iFood streamlines the entire ML lifecycle, from model training to deployment. This integration not only simplifies complex processes but also automates critical tasks.
AI inference at iFood
iFood has harnessed the power of a robust AI/ML platform to elevate the customer experience across its diverse touchpoints. Using the cutting edge of AI/ML capabilities, the company has developed a suite of transformative solutions to address a multitude of customer use cases:
- Personalized recommendations – At iFood, AI-powered recommendation models analyze a customer’s past order history, preferences, and contextual factors to suggest the most relevant restaurants and menu items. This personalized approach makes sure customers discover new cuisines and dishes tailored to their tastes, improving satisfaction and driving increased order volumes.
- Intelligent order tracking – iFood’s AI systems track orders in real time, predicting delivery times with a high degree of accuracy. By understanding factors like traffic patterns, restaurant preparation times, and courier locations, the AI can proactively notify customers of their order status and expected arrival, reducing uncertainty and anxiety during the delivery process.
- Automated customer Service – To handle the thousands of daily customer inquiries, iFood has developed an AI-powered chatbot that can quickly resolve common issues and questions. This intelligent virtual agent understands natural language, accesses relevant data, and provides personalized responses, delivering fast and consistent support without overburdening the human customer service team.
- Grocery shopping assistance – Integrating advanced language models, iFood’s app allows customers to simply speak or type their recipe needs or grocery list, and the AI will automatically generate a detailed shopping list. This voice-enabled grocery planning feature saves customers time and effort, enhancing their overall shopping experience.
Through these diverse AI-powered initiatives, iFood is able to anticipate customer needs, streamline key processes, and deliver a consistently exceptional experience—further strengthening its position as the leading food-tech platform in Latin America.
Solution overview
The following diagram illustrates iFood’s legacy architecture, which had separate workflows for data science and engineering teams, creating challenges in efficiently deploying accurate, real-time machine learning models into production systems.
In the past, the data science and engineering teams at iFood operated independently. Data scientists would build models using notebooks, adjust weights, and publish them onto services. Engineering teams would then struggle to integrate these models into production systems. This disconnection between the two teams made it challenging to deploy accurate real-time ML models.
To overcome this challenge, iFood built an internal ML platform that helped bridge this gap. This platform has streamlined the workflow, providing a seamless experience for creating, training, and delivering models for inference. It provides a centralized integration where data scientists could build, train, and deploy models seamlessly from an integrated approach, considering the development workflow of the teams. The interaction with engineering teams could consume these models and integrate them into applications from both an online and offline perspective, enabling a more efficient and streamlined workflow.
By breaking down the barriers between data science and engineering, AWS AI platforms empowered iFood to use the full potential of their data and accelerate the development of AI applications. The automated deployment and scalable inference capabilities provided by SageMaker made sure that models were readily available to power intelligent applications and provide accurate predictions on demand. This centralization of ML services as a product has been a game changer for iFood, allowing them to focus on building high-performing models rather than the intricate details of inference.
One of the core capabilities of iFood’s ML platform is the ability to provide the infrastructure to serve predictions. Several use cases are supported by the inference made available through ML Go!, responsible for deploying SageMaker pipelines and endpoints. The former are used to schedule offline predictions jobs, and the latter are employed to create model services, to be consumed by the application services. The following diagram illustrates iFood’s updated architecture, which incorporates an internal ML platform built to streamline workflows between data science and engineering teams, enabling efficient deployment of machine learning models into production systems.
Integrating model deployment into the service development process was a key initiative to enable data scientists and ML engineers to deploy and maintain those models. The ML platform empowers the building and evolution of ML systems. Several other integrations with other important platforms, like the feature platform and data platform, were delivered to increase the experience for the users as a whole. The process of consuming ML-based decisions was streamlined—but it doesn’t end there. The iFood’s ML platform, ML Go!, is now focusing on new inference capabilities, supported by recent features in which the iFood’s team was responsible for supporting their ideation and development. The following diagram illustrates the final architecture of iFood’s ML platform, showcasing how model deployment is integrated into the service development process, the platform’s connections with feature and data platforms, and its focus on new inference capabilities.
One of the biggest changes is oriented to the creation of one abstraction for connecting with SageMaker Endpoints and Jobs, called ML Go! Gateway, and also, the separation of concerns within the Endpoints, by the use of the Inference Components feature, making the serving faster and more efficient. In this new inference structure, the Endpoints are also managed by the ML Go! CI/CD, leaving for the pipelines, to deal only with model promotions, and not the infrastructure itself. It will reduce the lead time to changes, and change failure ratio over the deployments.
Using SageMaker Inference Model Serving Containers:
One of the key features of modern machine learning platforms is the standardization of machine learning and AI services. By encapsulating models and dependencies as Docker containers, these platforms ensure consistency and portability across different environments and stages of ML. Using SageMaker, data scientists and developers can use pre-built Docker containers, making it straightforward to deploy and manage ML services. As a project progresses, they can spin up new instances and configure them according to their specific requirements. SageMaker provides Docker containers that are designed to work seamlessly with SageMaker. These containers provide a standardized and scalable environment for running ML workloads on SageMaker.
SageMaker provides a set of pre-built containers for popular ML frameworks and algorithms, such as TensorFlow, PyTorch, XGBoost, and many others. These containers are optimized for performance and include all the necessary dependencies and libraries pre-installed, making it straightforward to get started with your ML projects. In addition to the pre-built containers, it provides options to bring your own custom containers to SageMaker, which include your specific ML code, dependencies, and libraries. This can be particularly useful if you’re using a less common framework or have specific requirements that aren’t met by the pre-built containers.
iFood was highly focused on using custom containers for the training and deployment of ML workloads, providing a consistent and reproducible environment for ML experiments, and making it effortless to track and replicate results. The first step in this journey was to standardize the ML custom code, which is actually the piece of code that the data scientists should focus on. Without a notebook, and with BruceML, the way to create the code to train and serve models has changed, to be encapsulated from the start as container images. BruceML was responsible for creating the scaffolding required to seamlessly integrate with the SageMaker platform, allowing the teams to take advantage of its various features, such as hyperparameter tuning, model deployment, and monitoring. By standardizing ML services and using containerization, modern platforms democratize ML, enabling iFood to rapidly build, deploy, and scale intelligent applications.
Automating model deployment and ML system retraining
When running ML models in production, it’s critical to have a robust and automated process for deploying and recalibrating those models across different use cases. This helps make sure the models remain accurate and performant over time. The team at iFood understood this challenge well—not only the model is deployed. Instead, they rely on another concept to keep things running well: ML pipelines.
Using Amazon SageMaker Pipelines, they were able to build a CI/CD system for ML, to deliver automated retraining and model deployment. They also integrated this entire system with the company’s existing CI/CD pipeline, making it efficient and also maintaining good DevOps practices used at iFood. It starts with the ML Go! CI/CD pipeline pushing the latest code artifacts containing the model training and deployment logic. It includes the training process, which uses different containers for implementing the entire pipeline. When training is complete, the inference pipeline can be executed to begin the model deployment. It can be an entirely new model, or the promotion of a new version to increase the performance of an existing one. Every model available for deployment is also secured and registered automatically by ML Go! in Amazon SageMaker Model Registry, providing versioning and tracking capabilities.
The final step depends on the intended inference requirements. For batch prediction use cases, the pipeline creates a SageMaker batch transform job to run large-scale predictions. For real-time inference, the pipeline deploys the model to a SageMaker endpoint, carefully selecting the appropriate container variant and instance type to handle the expected production traffic and latency needs. This end-to-end automation has been a game changer for iFood, allowing them to rapidly iterate on their ML models and deploy updates and recalibrations quickly and confidently across their various use cases. SageMaker Pipelines has provided a streamlined way to orchestrate these complex workflows, making sure model operationalization is efficient and reliable.
Running inference in different SLA formats
iFood uses the inference capabilities of SageMaker to power its intelligent applications and deliver accurate predictions to its customers. By integrating the robust inference options available in SageMaker, iFood has been able to seamlessly deploy ML models and make them available for real-time and batch predictions. For iFood’s online, real-time prediction use cases, the company uses SageMaker hosted endpoints to deploy their models. These endpoints are integrated into iFood’s customer-facing applications, allowing for immediate inference on incoming data from users. SageMaker handles the scaling and management of these endpoints, making sure that iFood’s models are readily available to provide accurate predictions and enhance the user experience.
In addition to real-time predictions, iFood also uses SageMaker batch transform to perform large-scale, asynchronous inference on datasets. This is particularly useful for iFood’s data preprocessing and batch prediction requirements, such as generating recommendations or insights for their restaurant partners. SageMaker batch transform jobs enable iFood to efficiently process vast amounts of data, further enhancing their data-driven decision-making.
Building upon the success of standardization to SageMaker Inference, iFood has been instrumental in partnering with the SageMaker Inference team to build and enhance key AI inference capabilities within the SageMaker platform. Since the early days of ML, iFood has provided the SageMaker Inference team with valuable inputs and expertise, enabling the introduction of several new features and optimizations:
- Cost and performance optimizations for generative AI inference – iFood helped the SageMaker Inference team develop innovative techniques to optimize the use of accelerators, enabling SageMaker Inference to reduce foundation model (FM) deployment costs by 50% on average and latency by 20% on average with inference components. This breakthrough delivers significant cost savings and performance improvements for customers running generative AI workloads on SageMaker.
- Scaling improvements for AI inference – iFood’s expertise in distributed systems and auto scaling has also helped the SageMaker team develop advanced capabilities to better handle the scaling requirements of generative AI models. These improvements reduce auto scaling times by up to 40% and auto scaling detection by six times, making sure that customers can rapidly scale their inference workloads on SageMaker to meet spikes in demand without compromising performance.
- Streamlined generative AI model deployment for inference – Recognizing the need for simplified model deployment, iFood collaborated with AWS to introduce the ability to deploy open source large language models (LLMs) and FMs with just a few clicks. This user-friendly functionality removes the complexity traditionally associated with deploying these advanced models, empowering more customers to harness the power of AI.
- Scale-to-zero for inference endpoints – iFood played a crucial role in collaborating with SageMaker Inference to develop and launch the scale-to-zero feature for SageMaker inference endpoints. This innovative capability allows inference endpoints to automatically shut down when not in use and rapidly spin up on demand when new requests arrive. This feature is particularly beneficial for dev/test environments, low-traffic applications, and inference use cases with varying inference demands, because it eliminates idle resource costs while maintaining the ability to quickly serve requests when needed. The scale-to-zero functionality represents a major advancement in cost-efficiency for AI inference, making it more accessible and economically viable for a wider range of use cases.
- Packaging AI model inference more efficiently – To further simplify the AI model lifecycle, iFood worked with AWS to enhance SageMaker’s capabilities for packaging LLMs and models for deployment. These improvements make it straightforward to prepare and deploy these AI models, accelerating their adoption and integration.
- Multi-model endpoints for GPU – iFood collaborated with the SageMaker Inference team to launch multi-model endpoints for GPU-based instances. This enhancement allows you to deploy multiple AI models on a single GPU-enabled endpoint, significantly improving resource utilization and cost-efficiency. By taking advantage of iFood’s expertise in GPU optimization and model serving, SageMaker now offers a solution that can dynamically load and unload models on GPUs, reducing infrastructure costs by up to 75% for customers with multiple models and varying traffic patterns.
- Asynchronous inference – Recognizing the need for handling long-running inference requests, the team at iFood worked closely with the SageMaker Inference team to develop and launch Asynchronous Inference in SageMaker. This feature enables you to process large payloads or time-consuming inference requests without the constraints of real-time API calls. iFood’s experience with large-scale distributed systems helped shape this solution, which now allows for better management of resource-intensive inference tasks, and the ability to handle inference requests that might take several minutes to complete. This capability has opened up new use cases for AI inference, particularly in industries dealing with complex data processing tasks such as genomics, video analysis, and financial modeling.
By closely partnering with the SageMaker Inference team, iFood has played a pivotal role in driving the rapid evolution of AI inference and generative AI inference capabilities in SageMaker. The features and optimizations introduced through this collaboration are empowering AWS customers to unlock the transformative potential of inference with greater ease, cost-effectiveness, and performance.
“At iFood, we were at the forefront of adopting transformative machine learning and AI technologies, and our partnership with the SageMaker Inference product team has been instrumental in shaping the future of AI applications. Together, we’ve developed strategies to efficiently manage inference workloads, allowing us to run models with speed and price-performance. The lessons we’ve learned supported us in the creation of our internal platform, which can serve as a blueprint for other organizations looking to harness the power of AI inference. We believe the features we have built in collaboration will broadly help other enterprises who run inference workloads on SageMaker, unlocking new frontiers of innovation and business transformation, by solving recurring and important problems in the universe of machine learning engineering.”
– says Daniel Vieira, ML Platform manager at iFood.
Conclusion
Using the capabilities of SageMaker, iFood transformed its approach to ML and AI, unleashing new possibilities for enhancing the customer experience. By building a robust and centralized ML platform, iFood has bridged the gap between its data science and engineering teams, streamlining the model lifecycle from development to deployment. The integration of SageMaker features has enabled iFood to deploy ML models for both real-time and batch-oriented use cases. For real-time, customer-facing applications, iFood uses SageMaker hosted endpoints to provide immediate predictions and enhance the user experience. Additionally, the company uses SageMaker batch transform to efficiently process large datasets and generate insights for its restaurant partners. This flexibility in inference options has been key to iFood’s ability to power a diverse range of intelligent applications.
The automation of deployment and retraining through ML Go!, supported by SageMaker Pipelines and SageMaker Inference, has been a game changer for iFood. This has enabled the company to rapidly iterate on its ML models, deploy updates with confidence, and maintain the ongoing performance and reliability of its intelligent applications. Moreover, iFood’s strategic partnership with the SageMaker Inference team has been instrumental in driving the evolution of AI inference capabilities within the platform. Through this collaboration, iFood has helped shape cost and performance optimizations, scale improvements, and simplify model deployment features—all of which are now benefiting a wider range of AWS customers.
By taking advantage of the capabilities SageMaker offers, iFood has been able to unlock the transformative potential of AI and ML, delivering innovative solutions that enhance the customer experience and strengthen its position as the leading food-tech platform in Latin America. This journey serves as a testament to the power of cloud-based AI infrastructure and the value of strategic partnerships in driving technology-driven business transformation.
By following iFood’s example, you can unlock the full potential of SageMaker for your business, driving innovation and staying ahead in your industry.
About the Authors
Daniel Vieira is a seasoned Machine Learning Engineering Manager at iFood, with a strong academic background in computer science, holding both a bachelor’s and a master’s degree from the Federal University of Minas Gerais (UFMG). With over a decade of experience in software engineering and platform development, Daniel leads iFood’s ML platform, building a robust, scalable ecosystem that drives impactful ML solutions across the company. In his spare time, Daniel Vieira enjoys music, philosophy, and learning about new things while drinking a good cup of coffee.
Debora Fanin serves as a Senior Customer Solutions Manager AWS for the Digital Native Business segment in Brazil. In this role, Debora manages customer transformations, creating cloud adoption strategies to support cost-effective, timely deployments. Her responsibilities include designing change management plans, guiding solution-focused decisions, and addressing potential risks to align with customer objectives. Debora’s academic path includes a Master’s degree in Administration at FEI and certifications such as Amazon Solutions Architect Associate and Agile credentials. Her professional history spans IT and project management roles across diverse sectors, where she developed expertise in cloud technologies, data science, and customer relations.
Saurabh Trikande is a Senior Product Manager for Amazon Bedrock and Amazon SageMaker Inference. He is passionate about working with customers and partners, motivated by the goal of democratizing AI. He focuses on core challenges related to deploying complex AI applications, inference with multi-tenant models, cost optimizations, and making the deployment of generative AI models more accessible. In his spare time, Saurabh enjoys hiking, learning about innovative technologies, following TechCrunch, and spending time with his family.
Gopi Mudiyala is a Senior Technical Account Manager at AWS. He helps customers in the financial services industry with their operations in AWS. As a machine learning enthusiast, Gopi works to help customers succeed in their ML journey. In his spare time, he likes to play badminton, spend time with family, and travel.
We’re introducing a new way to analyze geospatial data.
Imagine being able to get complex insights about our planet — like the impacts of hurricanes or best locations for city infrastructure — simply by asking Gemini. Geospat…Read More
Build an enterprise synthetic data strategy using Amazon Bedrock
The AI landscape is rapidly evolving, and more organizations are recognizing the power of synthetic data to drive innovation. However, enterprises looking to use AI face a major roadblock: how to safely use sensitive data. Stringent privacy regulations make it risky to use such data, even with robust anonymization. Advanced analytics can potentially uncover hidden correlations and reveal real data, leading to compliance issues and reputational damage. Additionally, many industries struggle with a scarcity of high-quality, diverse datasets needed for critical processes like software testing, product development, and AI model training. This data shortage can hinder innovation, slowing down development cycles across various business operations.
Organizations need innovative solutions to unlock the potential of data-driven processes without compromising ethics or data privacy. This is where synthetic data comes in—a solution that mimics the statistical properties and patterns of real data while being entirely fictitious. By using synthetic data, enterprises can train AI models, conduct analyses, and develop applications without the risk of exposing sensitive information. Synthetic data effectively bridges the gap between data utility and privacy protection. However, creating high-quality synthetic data comes with significant challenges:
- Data quality – Making sure synthetic data accurately reflects real-world statistical properties and nuances is difficult. The data might not capture rare edge cases or the full spectrum of human interactions.
- Bias management – Although synthetic data can help reduce bias, it can also inadvertently amplify existing biases if not carefully managed. The quality of synthetic data heavily depends on the model and data used to generate it.
- Privacy vs. utility – Balancing privacy preservation with data utility is complex. There’s a risk of reverse engineering or data leakage if not properly implemented.
- Validation challenges – Verifying the quality and representation of synthetic data often requires comparison with real data, which can be problematic when working with sensitive information.
- Reality gap – Synthetic data might not fully capture the dynamic nature of the real world, potentially leading to a disconnect between model performance on synthetic data and real-world applications.
In this post, we explore how to use Amazon Bedrock for synthetic data generation, considering these challenges alongside the potential benefits to develop effective strategies for various applications across multiple industries, including AI and machine learning (ML). Amazon Bedrock offers a broad set of capabilities to build generative AI applications with a focus on security, privacy, and responsible AI. Built within the AWS landscape, Amazon Bedrock is designed to help maintain the security and compliance standards required for enterprise use.
Attributes of high-quality synthetic data
To be truly effective, synthetic data must be both realistic and reliable. This means it should accurately reflect the complexities and nuances of real-world data while maintaining complete anonymity. A high-quality synthetic dataset exhibits several key characteristics that facilitate its fidelity to the original data:
- Data structure – The synthetic data should maintain the same structure as the real data, including the same number of columns, data types, and relationships between different data sources
- Statistical properties – The synthetic data should mimic the statistical properties of the real data, such as mean, median, standard deviation, correlation between variables, and distribution patterns.
- Temporal patterns – If the real data exhibits temporal patterns (for example, diurnal or seasonal patterns), the synthetic data should also reflect these patterns.
- Anomalies and outliers – Real-world data often contains anomalies and outliers. The synthetic data should also include a similar proportion and distribution of anomalies and outliers to accurately represent the real-world scenario.
- Referential integrity – If the real data has relationships and dependencies between different data sources, the synthetic data should maintain these relationships to facilitate referential integrity.
- Consistency – The synthetic data should be consistent across different data sources and maintain the relationships and dependencies between them, facilitating a coherent and unified representation of the dataset.
- Scalability – The synthetic data generation process should be scalable to handle large volumes of data and support the generation of synthetic data for different scenarios and use cases.
- Diversity – The synthetic data should capture the diversity present in the real data.
Solution overview
Generating useful synthetic data that protects privacy requires a thoughtful approach. The following figure represents the high-level architecture of the proposed solution. The process involves three key steps:
- Identify validation rules that define the structure and statistical properties of the real data.
- Use those rules to generate code using Amazon Bedrock that creates synthetic data subsets.
- Combine multiple synthetic subsets into full datasets.
Let’s explore these three key steps for creating useful synthetic data in more detail.
Step 1: Define data rules and characteristics
- To create synthetic datasets, start by establishing clear rules that capture the essence of your target data:
- Use domain-specific knowledge to identify key attributes and relationships.
- Study existing public datasets, academic resources, and industry documentation.
- Use tools like AWS Glue DataBrew, Amazon Bedrock, or open source alternatives (such as Great Expectations) to analyze data structures and patterns.
- Develop a comprehensive rule-set covering:
- Data types and value ranges
- Inter-field relationships
- Quality standards
- Domain-specific patterns and anomalies
This foundational step makes sure your synthetic data accurately reflects real-world scenarios in your industry.
Step 2: Generate code with Amazon Bedrock
Transform your data rules into functional code using Amazon Bedrock language models:
- Choose an appropriate Amazon Bedrock model based on code generation capabilities and domain relevance.
- Craft a detailed prompt describing the desired code output, including data structures and generation rules.
- Use the Amazon Bedrock API to generate Python code based on your prompts.
- Iteratively refine the code by:
- Reviewing for accuracy and efficiency
- Adjusting prompts as needed
- Incorporating developer input for complex scenarios
The result is a tailored script that generates synthetic data entries matching your specific requirements and closely mimicking real-world data in your domain.
Step 3: Assemble and scale the synthetic dataset
Transform your generated data into a comprehensive, real-world representative dataset:
- Use the code from Step 2 to create multiple synthetic subsets for various scenarios.
- Merge subsets based on domain knowledge, maintaining realistic proportions and relationships.
- Align temporal or sequential components and introduce controlled randomness for natural variation.
- Scale the dataset to required sizes, reflecting different time periods or populations.
- Incorporate rare events and edge cases at appropriate frequencies.
- Generate accompanying metadata describing dataset characteristics and the generation process.
The end result is a diverse, realistic synthetic dataset for uses like system testing, ML model training, or data analysis. The metadata provides transparency into the generation process and data characteristics. Together, these measures result in a robust synthetic dataset that closely parallels real-world data while avoiding exposure of direct sensitive information. This generalized approach can be adapted to various types of datasets, from financial transactions to medical records, using the power of Amazon Bedrock for code generation and the expertise of domain knowledge for data validation and structuring.
Importance of differential privacy in synthetic data generation
Although synthetic data offers numerous benefits for analytics and machine learning, it’s essential to recognize that privacy concerns persist even with artificially generated datasets. As we strive to create high-fidelity synthetic data, we must also maintain robust privacy protections for the original data. Although synthetic data mimics patterns in actual data, if created improperly, it risks revealing details about sensitive information in the source dataset. This is where differential privacy enters the picture. Differential privacy is a mathematical framework that provides a way to quantify and control the privacy risks associated with data analysis. It works by injecting calibrated noise into the data generation process, making it virtually impossible to infer anything about a single data point or confidential information in the source dataset.
Differential privacy protects against re-identification exploits by adversaries attempting to extract details about data. The carefully calibrated noise added to synthetic data makes sure that even if an adversary tries, it is computationally infeasible to tie an output back to specific records in the original data, while still maintaining the overall statistical properties of the dataset. This allows the synthetic data to closely reflect real-world characteristics and remain useful for analytics and modeling while protecting privacy. By incorporating differential privacy techniques into the synthetic data generation process, you can create datasets that not only maintain statistical properties of the original data but also offer strong privacy guarantees. It enables organizations to share data more freely, collaborate on sensitive projects, and develop AI models with reduced risk of privacy breaches. For instance, in healthcare, differentially private synthetic patient data can accelerate research without compromising individual patient confidentiality.
As we continue to advance in the field of synthetic data generation, the incorporation of differential privacy is becoming not just a best practice, but a necessary component for responsible data science. This approach paves the way for a future where data utility and privacy protection coexist harmoniously, fostering innovation while safeguarding individual rights. However, although differential privacy offers strong theoretical guarantees, its practical implementation can be challenging. Organizations must carefully balance the trade-off between privacy and utility, because increasing privacy protection often comes at the cost of reduced data utility.
Build synthetic datasets for Trusted Advisor findings with Amazon Bedrock
In this post, we guide you through the process of creating synthetic datasets for AWS Trusted Advisor findings using Amazon Bedrock. Trusted Advisor provides real-time guidance to optimize your AWS environment, improving performance, security, and cost-efficiency through over 500 checks against AWS best practices. We demonstrate the synthetic data generation approach using the “Underutilized Amazon EBS Volumes” check (checkid: DAvU99Dc4C) as an example.
By following this post, you will gain practical knowledge on:
- Defining data rules for Trusted Advisor findings
- Using Amazon Bedrock to generate data creation code
- Assembling and scaling synthetic datasets
This approach can be applied across over 500 Trusted Advisor checks, enabling you to build comprehensive, privacy-aware datasets for testing, training, and analysis. Whether you’re looking to enhance your understanding of Trusted Advisor recommendations or develop new optimization strategies, synthetic data offers powerful possibilities.
Prerequisites
To implement this approach, you must have an AWS account with the appropriate permissions.
- AWS Account Setup:
- IAM permissions for:
- Amazon Bedrock
- AWS Trusted Advisor
- Amazon EBS
- IAM permissions for:
- AWS Service Access:
- Access enabled for Amazon Bedrock in your Region
- Access to Anthropic Claude model in Amazon Bedrock
- Enterprise or Business support plan for full Trusted Advisor access
- Development Environment:
- Python 3.8 or later installed
- Required Python packages:
- pandas
- numpy
- random
- boto3
- Knowledge Requirements:
- Basic understanding of:
- Python programming
- AWS services (especially EBS and Trusted Advisor)
- Data analysis concepts
- JSON/YAML file format
- Basic understanding of:
Define Trusted Advisor findings rules
Begin by examining real Trusted Advisor findings for the “Underutilized Amazon EBS Volumes” check. Analyze the structure and content of these findings to identify key data elements and their relationships. Pay attention to the following:
- Standard fields – Check ID, volume ID, volume type, snapshot ID, and snapshot age
- Volume attributes – Size, type, age, and cost
- Usage metrics – Read and write operations, throughput, and IOPS
- Temporal patterns – Volume type and size variations
- Metadata – Tags, creation date, and last attached date
As you study these elements, note the typical ranges, patterns, and distributions for each attribute. For example, observe how volume sizes correlate with volume types, or how usage patterns differ between development and production environments. This analysis will help you create a set of rules that accurately reflect real-world Trusted Advisor findings.
After analyzing real Trusted Advisor outputs for the “Underutilized Amazon EBS Volumes” check, we identified the following crucial patterns and rules:
- Volume type – Consider gp2, gp3, io1, io2, and st1 volume types. Verify the volume sizes are valid for volume types.
- Criteria – Represent multiple AWS Regions, with appropriate volume types. Correlate snapshot ages with volume ages.
- Data structure – Each finding should include the same columns.
The following is an example ruleset:
Generate code with Amazon Bedrock
With your rules defined, you can now use Amazon Bedrock to generate Python code for creating synthetic Trusted Advisor findings.
The following is an example prompt for Amazon Bedrock:
You can submit this prompt to the Amazon Bedrock chat playground using Anthropic’s Claude 3.5 Sonnet on Amazon Bedrock, and receive generated Python code. Review this code carefully, verifying it meets all specifications and generates realistic data. If necessary, iterate on your prompt or make manual adjustments to the code to address any missing logic or edge cases.
The resulting code will serve as the foundation for creating varied and realistic synthetic Trusted Advisor findings that adhere to the defined parameters. By using Amazon Bedrock in this way, you can quickly develop sophisticated data generation code that would otherwise require significant manual effort and domain expertise to create.
Create data subsets
With the code generated by Amazon Bedrock and refined with your custom functions, you can now create diverse subsets of synthetic Trusted Advisor findings for the “Underutilized Amazon EBS Volumes” check. This approach allows you to simulate a wide range of real-world scenarios. In the following sample code, we have customized the volume_id and snapshot_id format to begin with vol-9999 and snap-9999, respectively:
This code creates subsets that include:
- Various volume types and instance types
- Different levels of utilization
- Occasional misconfigurations (for example, underutilized volumes)
- Diverse regional distribution
Combine and scale the dataset
The process of combining and scaling synthetic data involves merging multiple generated datasets while introducing realistic anomalies to create a comprehensive and representative dataset. This step is crucial for making sure that your synthetic data reflects the complexity and variability found in real-world scenarios. Organizations typically introduce controlled anomalies at a specific rate (usually 5–10% of the dataset) to simulate various edge cases and unusual patterns that might occur in production environments. These anomalies help in testing system responses, developing monitoring solutions, and training ML models to identify potential issues.
When generating synthetic data for underutilized EBS volumes, you might introduce anomalies such as oversized volumes (5–10 times larger than needed), volumes with old snapshots (older than 365 days), or high-cost volumes with low utilization. For instance, a synthetic dataset might include a 1 TB gp2 volume that’s only using 100 GB of space, simulating a real-world scenario of overprovisioned resources. See the following code:
The following screenshot shows an example of sample rows generated.
Validate the synthetic Trusted Advisor findings
Data validation is a critical step that verifies the quality, reliability, and representativeness of your synthetic data. This process involves performing rigorous statistical analysis to verify that the generated data maintains proper distributions, relationships, and patterns that align with real-world scenarios. Validation should include both quantitative metrics (statistical measures) and qualitative assessments (pattern analysis). Organizations should implement comprehensive validation frameworks that include distribution analysis, correlation checks, pattern verification, and anomaly detection. Regular visualization of the data helps in identifying inconsistencies or unexpected patterns.
For EBS volume data, validation might include analyzing the distribution of volume sizes across different types (gp2, gp3, io1), verifying that cost correlations match expected patterns, and making sure that introduced anomalies (like underutilized volumes) maintain realistic proportions. For instance, validating that the percentage of underutilized volumes aligns with typical enterprise environments (perhaps 15–20% of total volumes) and that the cost-to-size relationships remain realistic across volume types.
The following figures show examples of our validation checks.
- The following screenshot shows statistics of the generated synthetic datasets.
- The following figure shows the proportion of underutilized volumes in the generated synthetic datasets.
- The following figure shows the distribution of volume sizes in the generated synthetic datasets.
- The following figure shows the distribution of volume types in the generated synthetic datasets.
- The following figure shows the distribution of snapshot ages in the generated synthetic datasets.
Enhancing synthetic data with differential privacy
After exploring the steps to create synthetic datasets for the Trusted Advisor “Underutilized Amazon EBS Volumes” check, it’s worth revisiting how differential privacy strengthens this approach. When a cloud consulting firm analyzes aggregated Trusted Advisor data across multiple clients, differential privacy through OpenDP provides the critical privacy-utility balance needed. By applying carefully calibrated noise to computations of underutilized volume statistics, consultants can generate synthetic datasets that preserve essential patterns across Regions and volume types while mathematically guaranteeing individual client confidentiality. This approach verifies that the synthetic data maintains sufficient accuracy for meaningful trend analysis and recommendations, while eliminating the risk of revealing sensitive client-specific infrastructure details or usage patterns—making it an ideal complement to our synthetic data generation pipeline.
Conclusion
In this post, we showed how to use Amazon Bedrock to create synthetic data for enterprise needs. By combining language models available in Amazon Bedrock with industry knowledge, you can build a flexible and secure way to generate test data. This approach helps create realistic datasets without using sensitive information, saving time and money. It also facilitates consistent testing across projects and avoids ethical issues of using real user data. Overall, this strategy offers a solid solution for data challenges, supporting better testing and development practices.
In part 2 of this series, we will demonstrate how to use pattern recognition for different datasets to automate rule-set generation needed for the Amazon Bedrock prompts to generate corresponding synthetic data.
About the authors
Devi Nair is a Technical Account Manager at Amazon Web Services, providing strategic guidance to enterprise customers as they build, operate, and optimize their workloads on AWS. She focuses on aligning cloud solutions with business objectives to drive long-term success and innovation.
Vishal Karlupia is a Senior Technical Account Manager/Lead at Amazon Web Services, Toronto. He specializes in generative AI applications and helps customers build and scale their AI/ML workloads on AWS. Outside of work, he enjoys being outdoors and keeping bonfires alive.
Srinivas Ganapathi is a Principal Technical Account Manager at Amazon Web Services. He is based in Toronto, Canada, and works with games customers to run efficient workloads on AWS.
Nicolas Simard is a Technical Account Manager based in Montreal. He helps organizations accelerate their AI adoption journey through technical expertise, architectural best practices, and enables them to maximize business value from AWS’s Generative AI capabilities.
National Robotics Week — Latest Physical AI Research, Breakthroughs and Resources
Check back here throughout the week to learn the latest on physical AI, which enables machines to perceive, plan and act with greater autonomy and intelligence in real-world environments.
This National Robotics Week, running through April 12, NVIDIA is highlighting the pioneering technologies that are shaping the future of intelligent machines and driving progress across manufacturing, healthcare, logistics and more.
Advancements in robotics simulation and robot learning are driving this fundamental shift in the industry. Plus, the emergence of world foundation models is accelerating the evolution of AI-enabled robots capable of adapting to dynamic and complex scenarios.
For example, by providing robot foundation models like NVIDIA GR00T N1, frameworks such as NVIDIA Isaac Sim and Isaac Lab for robot simulation and training, and synthetic data generation pipelines to help train robots for diverse tasks, the NVIDIA Isaac and GR00T platforms are empowering researchers and developers to push the boundaries of robotics.
Hackathon Features Robots Powered by NVIDIA Isaac GR00T N1 
The Seeed Studio Embodied AI Hackathon, which took place last month, brought together the robotics community to showcase innovative projects using the LeRobot SO-100ARM motor kit.
The event highlighted how robot learning is advancing AI-driven robotics, with teams successfully integrating the NVIDIA Isaac GR00T N1 model to speed humanoid robot development. A notable project involved developing leader-follower robot pairs capable of learning pick-and-place tasks by post-training robot foundation models on real-world demonstration data.
How the project worked:
- Real-World Imitation Learning: Robots observe and mimic human-led demonstrations, recorded through Arducam vision systems and an external camera.
- Post-Training Pipeline: Captured data is structured into a modality.json dataset for efficient GPU-based training with GR00T N1.
- Bimanual Manipulation: The model is optimized for controlling two robotic arms simultaneously, enhancing cooperative skills.
The dataset is now publicly available on Hugging Face, with implementation details on GitHub.
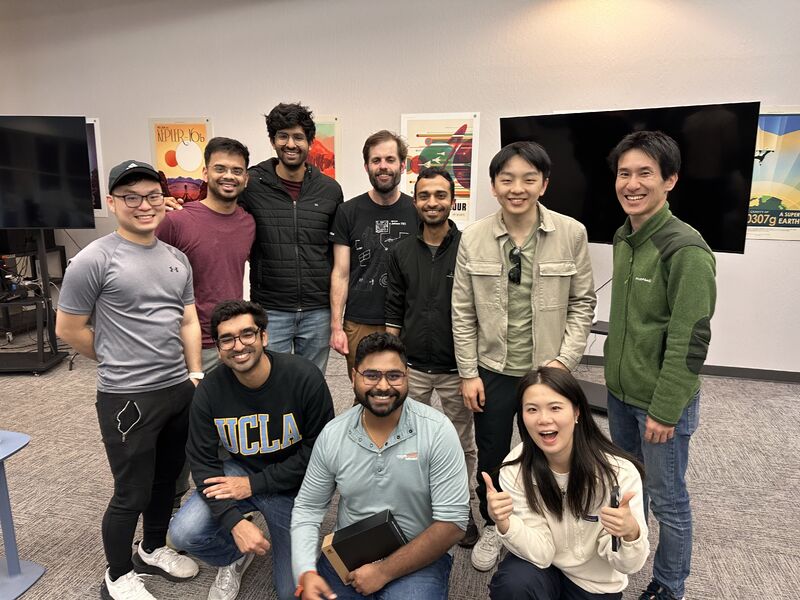
Learn more about the project.
Advancing Robotics: IEEE Robotics and Automation Society Honors Emerging Innovators 
The IEEE Robotics and Automation Society in March announced the recipients of its 2025 Early Academic Career Award, recognizing outstanding contributions to the fields of robotics and automation.
This year’s honorees — including NVIDIA’s Shuran Song, Abhishek Gupta and Yuke Zhu — are pioneering advancements in scalable robot learning, real-world reinforcement learning and embodied AI. Their work is shaping the next generation of intelligent systems, driving innovation that impacts both research and real-world applications.
Learn more about the award winners:
- Shuran Song, principal research scientist at NVIDIA, was recognized for her contributions to scalable robot learning. Notable recent papers include:
- Abhishek Gupta, visiting professor at NVIDIA, was honored for his pioneering work in real-world robotic reinforcement learning. Notable recent papers include:
- Yuke Zhu, principal research scientist at NVIDIA, was awarded for his contributions to embodied AI and widely used open-source software platforms. Notable recent papers include:
These researchers will be recognized at the International Conference on Robotics and Automation in May.
Stay up to date on NVIDIA’s leading robotics research through the Robotics Research and Development Digest (R2D2) tech blog series, subscribing to this newsletter and following NVIDIA Robotics on YouTube, Discord and developer forums.