In the first post of this series, we introduced a comprehensive evaluation framework for Amazon Q Business, a fully managed Retrieval Augmented Generation (RAG) solution that uses your company’s proprietary data without the complexity of managing large language models (LLMs). The first post focused on selecting appropriate use cases, preparing data, and implementing metrics to support a human-in-the-loop evaluation process.
In this post, we dive into the solution architecture necessary to implement this evaluation framework for your Amazon Q Business application. We explore two distinct evaluation solutions:
- Comprehensive evaluation workflow – This ready-to-deploy solution uses AWS CloudFormation stacks to set up an Amazon Q Business application, complete with user access, a custom UI for review and evaluation, and the supporting evaluation infrastructure
- Lightweight AWS Lambda based evaluation – Designed for users with an existing Amazon Q Business application, this streamlined solution employs an AWS Lambda function to efficiently assess the application’s accuracy
By the end of this post, you will have a clear understanding of how to implement an evaluation framework that aligns with your specific needs with a detailed walkthrough, so your Amazon Q Business application delivers accurate and reliable results.
Challenges in evaluating Amazon Q Business
Evaluating the performance of Amazon Q Business, which uses a RAG model, presents several challenges due to its integration of retrieval and generation components. It’s crucial to identify which aspects of the solution need evaluation. For Amazon Q Business, both the retrieval accuracy and the quality of the answer output are important factors to assess. In this section, we discuss key metrics that need to be included for a RAG generative AI solution.
Context recall
Context recall measures the extent to which all relevant content is retrieved. High recall provides comprehensive information gathering but might introduce extraneous data.
For example, a user might ask the question “What can you tell me about the geography of the United States?” They could get the following responses:
- Expected: The United States is the third-largest country in the world by land area, covering approximately 9.8 million square kilometers. It has a diverse range of geographical features.
- High context recall: The United States spans approximately 9.8 million square kilometers, making it the third-largest nation globally by land area. country’s geography is incredibly diverse, featuring the Rocky Mountains stretching from New Mexico to Alaska, the Appalachian Mountains along the eastern states, the expansive Great Plains in the central region, arid deserts like the Mojave in the southwest.
- Low context recall: The United States features significant geographical landmarks. Additionally, the country is home to unique ecosystems like the Everglades in Florida, a vast network of wetlands.
The following diagram illustrates the context recall workflow.
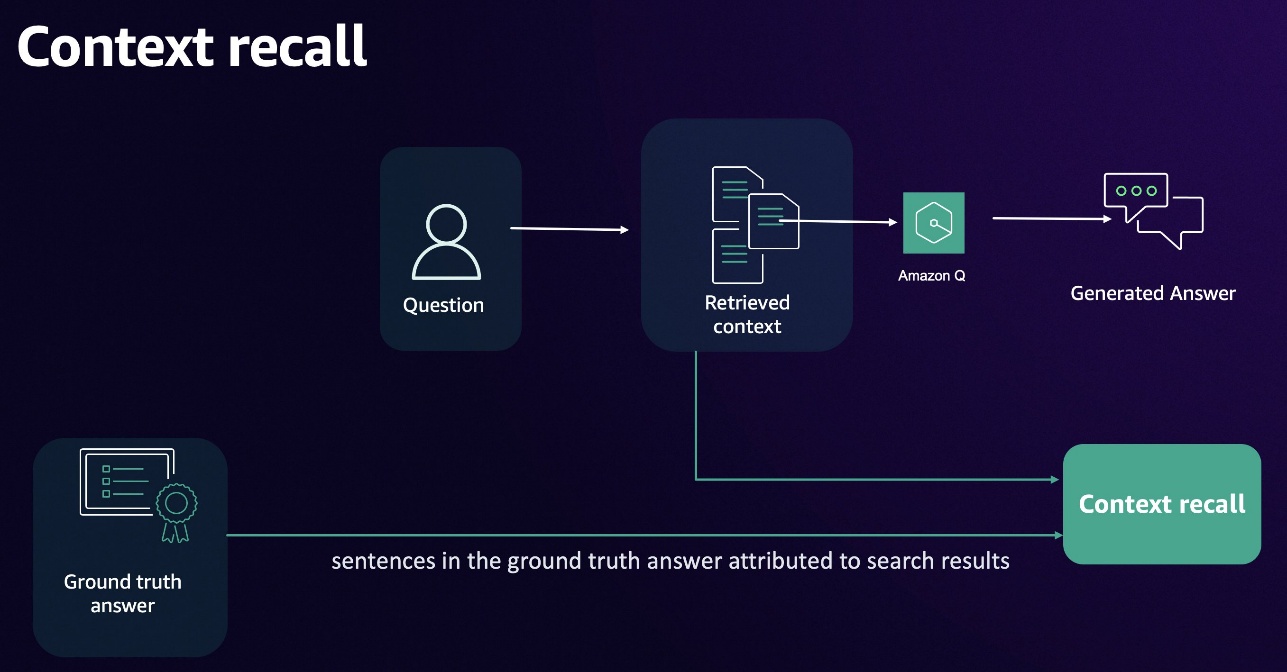
Context precision
Context precision assesses the relevance and conciseness of retrieved information. High precision indicates that the retrieved information closely matches the query intent, reducing irrelevant data.
For example, “Why Silicon Valley is great for tech startups?”might give the following answers:
- Ground truth answer: Silicon Valley is famous for fostering innovation and entrepreneurship in the technology sector.
- High precision context: Many groundbreaking startups originate from Silicon Valley, benefiting from a culture that encourages innovation, risk-taking
- Low precision context: Silicon Valley experiences a Mediterranean climate, with mild, wet, winters and warm, dry summers, contributing to its appeal as a place to live and works
The following diagram illustrates the context precision workflow.
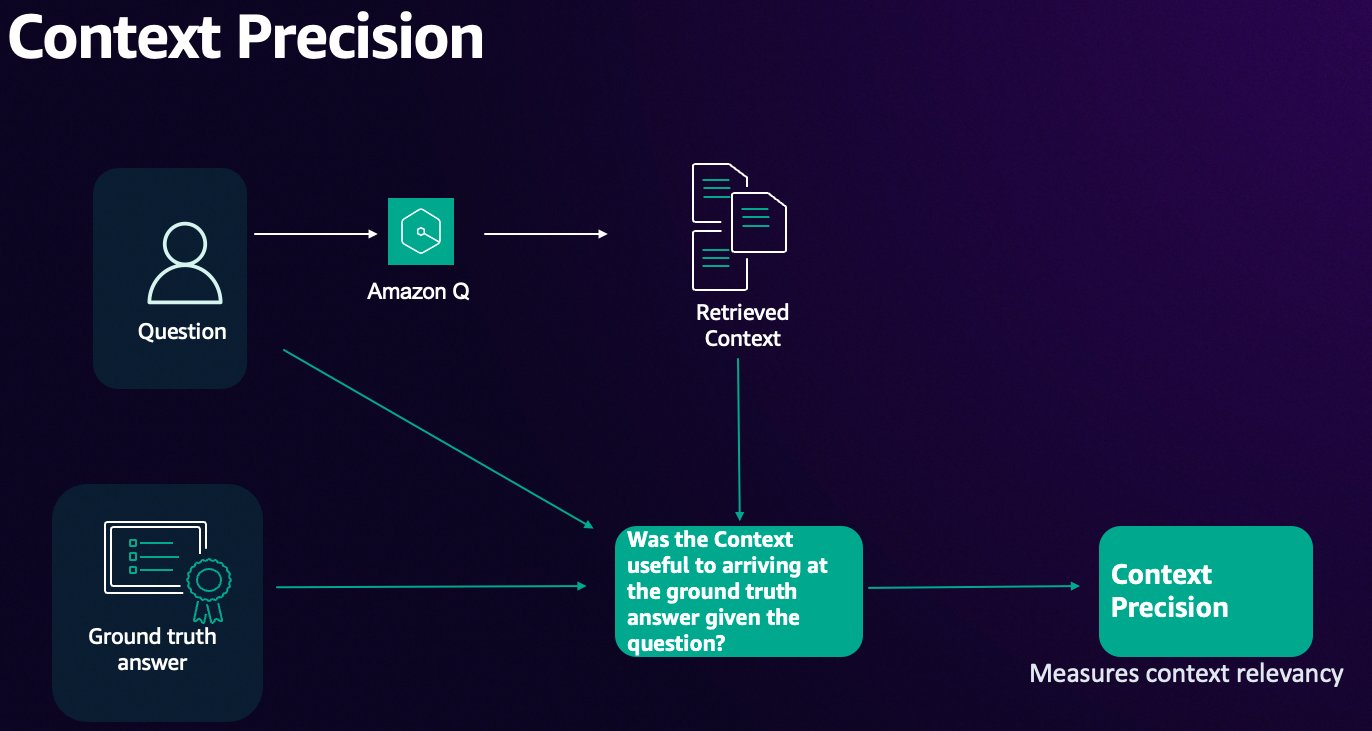
Answer relevancy
Answer relevancy evaluates whether responses fully address the query without unnecessary details. Relevant answers enhance user satisfaction and trust in the system.
For example, a user might ask the question “What are the key features of Amazon Q Business Service, and how can it benefit enterprise customers?” They could get the following answers:
- High relevance answer: Amazon Q Business Service is a RAG Generative AI solution designed for enterprise use. Key features include a fully managed Generative AI solutions, integration with enterprise data sources, robust security protocols, and customizable virtual assistants. It benefits enterprise customers by enabling efficient information retrieval, automating customer support tasks, enhancing employee productivity through quick access to data, and providing insights through analytics on user interactions.
- Low relevance answer: Amazon Q Business Service is part of Amazon’s suite of cloud services. Amazon also offers online shopping and streaming services.
The following diagram illustrates the answer relevancy workflow.
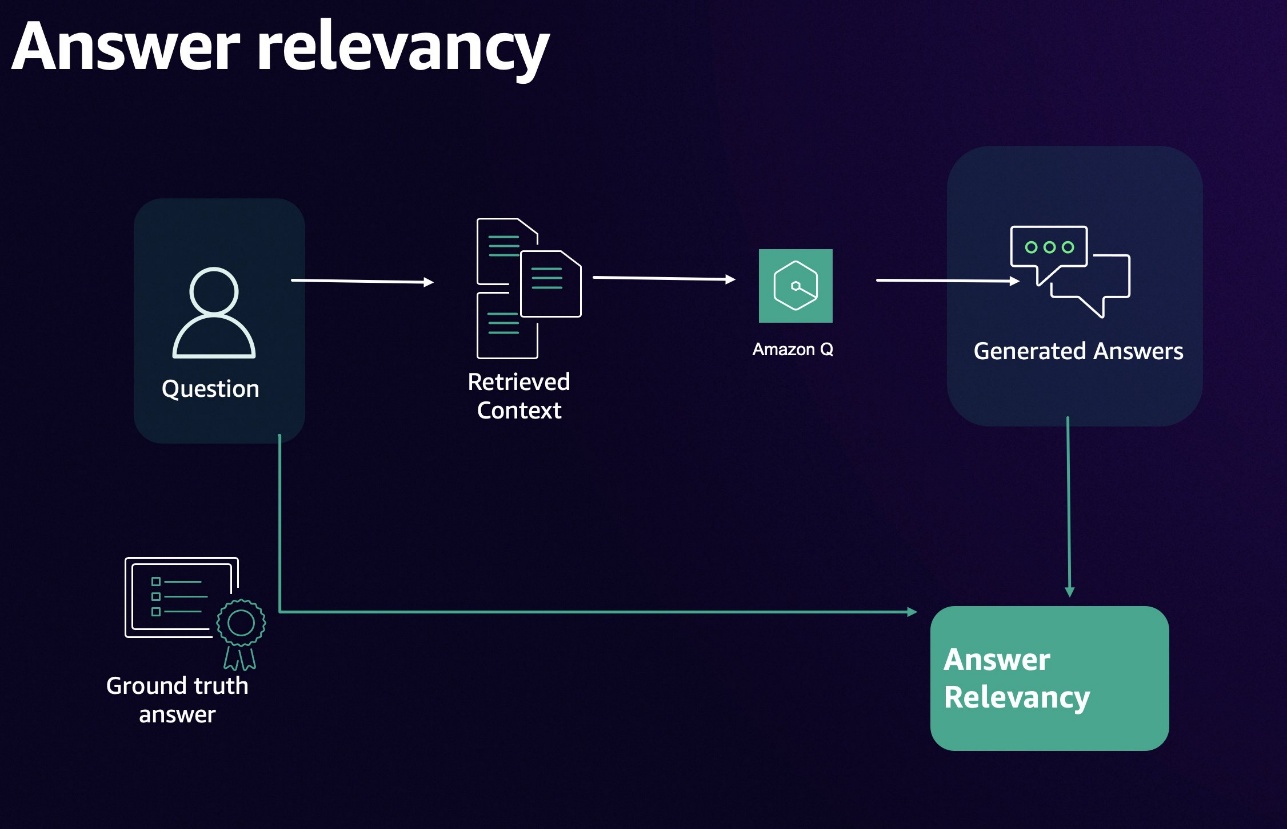
Truthfulness
Truthfulness verifies factual accuracy by comparing responses to verified sources. Truthfulness is crucial to maintain the system’s credibility and reliability.
For example, a user might ask “What is the capital of Canada?” They could get the following responses:
- Context: Canada’s capital city is Ottawa, located in the province of Ontario. Ottawa is known for its historic Parliament Hill, the center of government, and the scenic Rideau Canal, a UNESCO World Heritage site
- High truthfulness answer: The capital of Canada is Ottawa
- Low truthfulness answer: The capital of Canada is Toronto
The following diagram illustrates the truthfulness workflow.
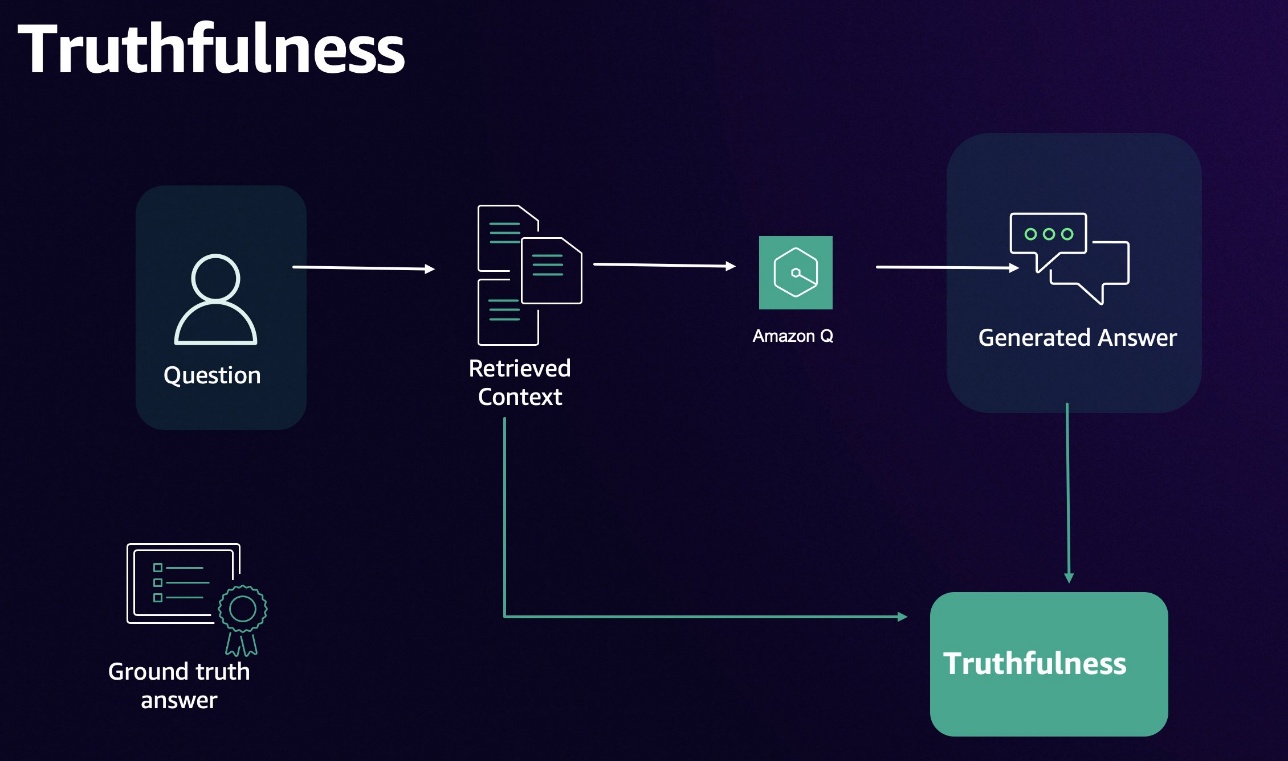
Evaluation methods
Deciding on who should conduct the evaluation can significantly impact results. Options include:
- Human-in-the-Loop (HITL) – Human evaluators manually assess the accuracy and relevance of responses, offering nuanced insights that automated systems might miss. However, it is a slow process and difficult to scale.
- LLM-aided evaluation – Automated methods, such as the Ragas framework, use language models to streamline the evaluation process. However, these might not fully capture the complexities of domain-specific knowledge.
Each of these preparatory and evaluative steps contributes to a structured approach to evaluating the accuracy and effectiveness of Amazon Q Business in supporting enterprise needs.
Solution overview
In this post, we explore two different solutions to provide you the details of an evaluation framework, so you can use it and adapt it for your own use case.
Solution 1: End-to-end evaluation solution
For a quick start evaluation framework, this solution uses a hybrid approach with Ragas (automated scoring) and HITL evaluation for robust accuracy and reliability. The architecture includes the following components:
- User access and UI – Authenticated users interact with a frontend UI to upload datasets, review RAGAS output, and provide human feedback
- Evaluation solution infrastructure – Core components include:
- Ragas scoring – Automated metrics provide an initial layer of evaluation
- HITL review – Human evaluators refine Ragas scores through the UI, providing nuanced accuracy and reliability
By integrating a metric-based approach with human validation, this architecture makes sure Amazon Q Business delivers accurate, relevant, and trustworthy responses for enterprise users. This solution further enhances the evaluation process by incorporating HITL reviews, enabling human feedback to refine automated scores for higher precision.
A quick video demo of this solution is shown below:
Solution architecture
The solution architecture is designed with the following core functionalities to support an evaluation framework for Amazon Q Business:
- User access and UI – Users authenticate through Amazon Cognito, and upon successful login, interact with a Streamlit-based custom UI. This frontend allows users to upload CSV datasets to Amazon Simple Storage Service (Amazon S3), review Ragas evaluation outputs, and provide human feedback for refinement. The application exchanges the Amazon Cognito token for an AWS IAM Identity Center token, granting scoped access to Amazon Q Business.UI
- infrastructure – The UI is hosted behind an Application Load Balancer, supported by Amazon Elastic Compute Cloud (Amazon EC2) instances running in an Auto Scaling group for high availability and scalability.
- Upload dataset and trigger evaluation – Users upload a CSV file containing queries and ground truth answers to Amazon S3, which triggers an evaluation process. A Lambda function reads the CSV, stores its content in a DynamoDB table, and initiates further processing through a DynamoDB stream.
- Consuming DynamoDB stream – A separate Lambda function processes new entries from the DynamoDB stream, and publishes messages to an SQS queue, which serves as a trigger for the evaluation Lambda function.
- Ragas scoring – The evaluation Lambda function consumes SQS messages, sending queries (prompts) to Amazon Q Business for generating answers. It then evaluates the prompt, ground truth, and generated answer using the Ragas evaluation framework. Ragas computes automated evaluation metrics such as context recall, context precision, answer relevancy, and truthfulness. The results are stored in DynamoDB and visualized in the UI.
HITL review – Authenticated users can review and refine RAGAS scores directly through the UI, providing nuanced and accurate evaluations by incorporating human insights into the process.
This architecture uses AWS services to deliver a scalable, secure, and efficient evaluation solution for Amazon Q Business, combining automated and human-driven evaluations.
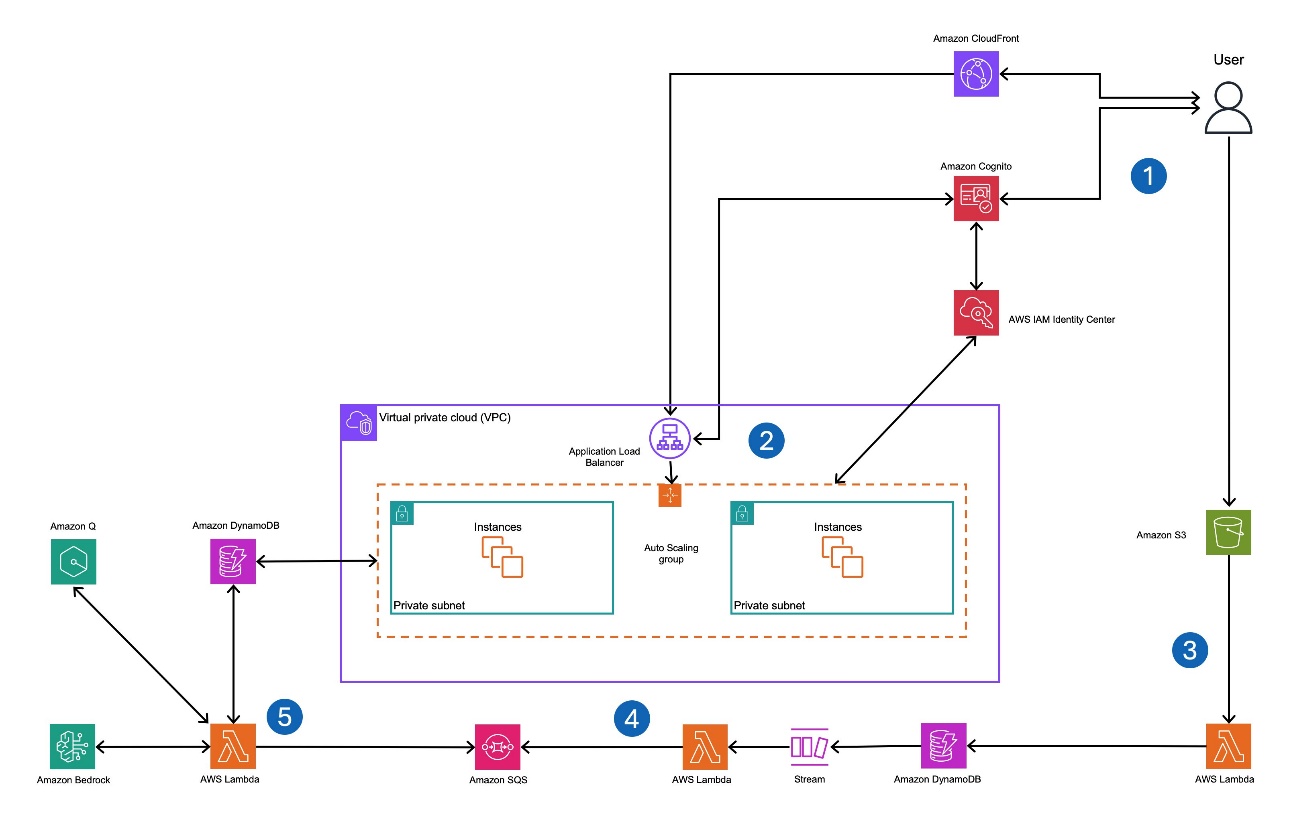
Prerequisites
For this walkthrough, you should have the following prerequisites:
Additionally, make sure that all the resources you deploy are in the same AWS Region.
Deploy the CloudFormation stack
Complete the following steps to deploy the CloudFormation stack:
- Clone the repository or download the files to your local computer.
- Unzip the downloaded file (if you used this option).
- Using your local computer command line, use the ‘cd’ command and change directory into
./sample-code-for-evaluating-amazon-q-business-applications-using-ragas-main/end-to-end-solution
- Make sure the
./deploy.sh
script can run by executing the command chmod 755 ./deploy.sh
.
- Execute the CloudFormation deployment script provided as follows:
./deploy.sh -s [CNF_STACK_NAME] -r [AWS_REGION]
You can follow the deployment progress on the AWS CloudFormation console. It takes approximately 15 minutes to complete the deployment, after which you will see a similar page to the following screenshot.
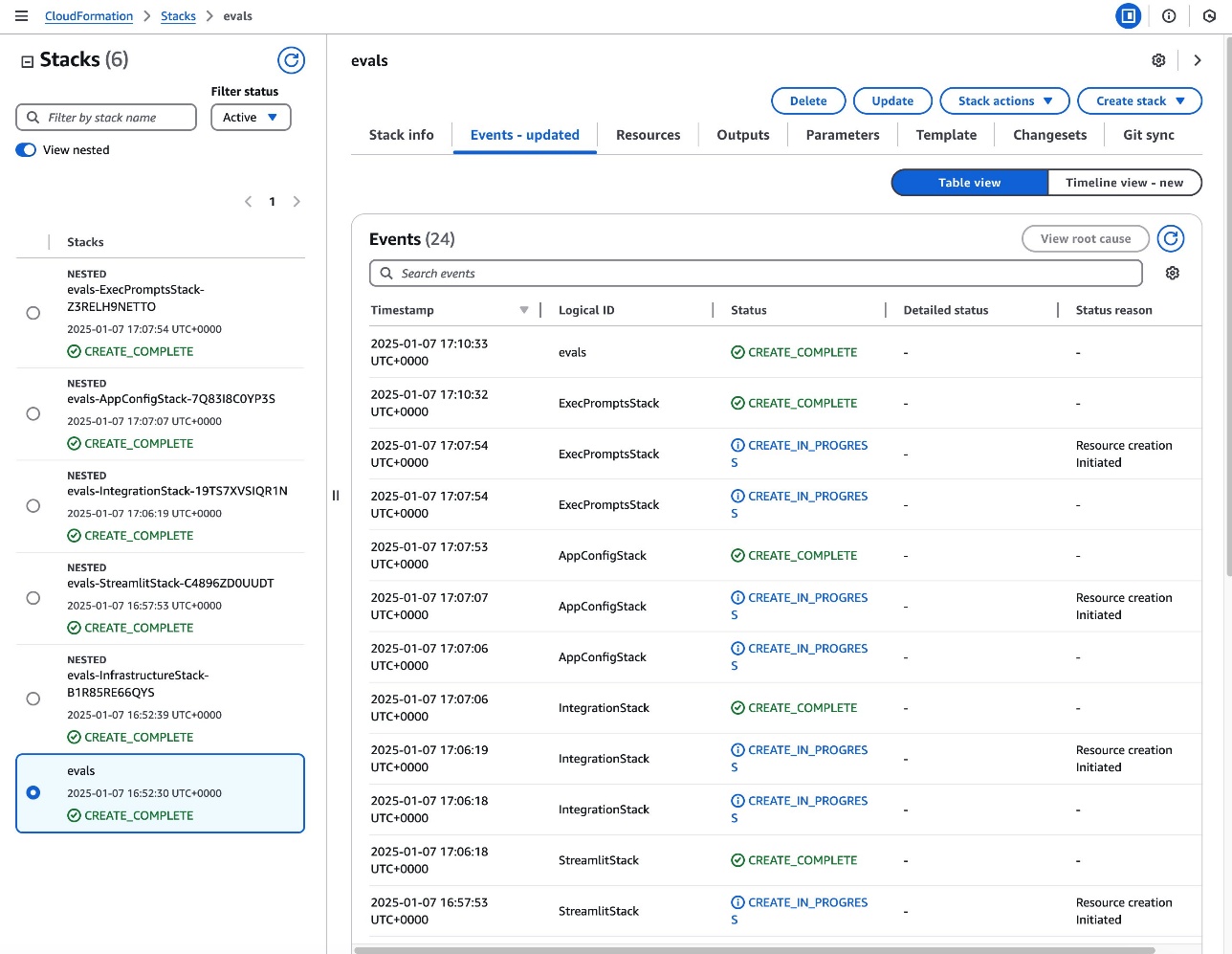
Add users to Amazon Q Business
You need to provision users for the pre-created Amazon Q Business application. Refer to Setting up for Amazon Q Business for instructions to add users.
Upload the evaluation dataset through the UI
In this section, you review and upload the following CSV file containing an evaluation dataset through the deployed custom UI.
This CSV file contains two columns: prompt
and ground_truth
. There are four prompts and their associated ground truth in this dataset:
- What are the index types of Amazon Q Business and the features of each?
- I want to use Q Apps, which subscription tier is required to use Q Apps?
- What is the file size limit for Amazon Q Business via file upload?
- What data encryption does Amazon Q Business support?
To upload the evaluation dataset, complete the following steps:
- On the AWS CloudFormation console, choose Stacks in the navigation pane.
- Choose the
evals
stack that you already launched.
- On the Outputs tab, take note of the user name and password to log in to the UI application, and choose the UI URL.
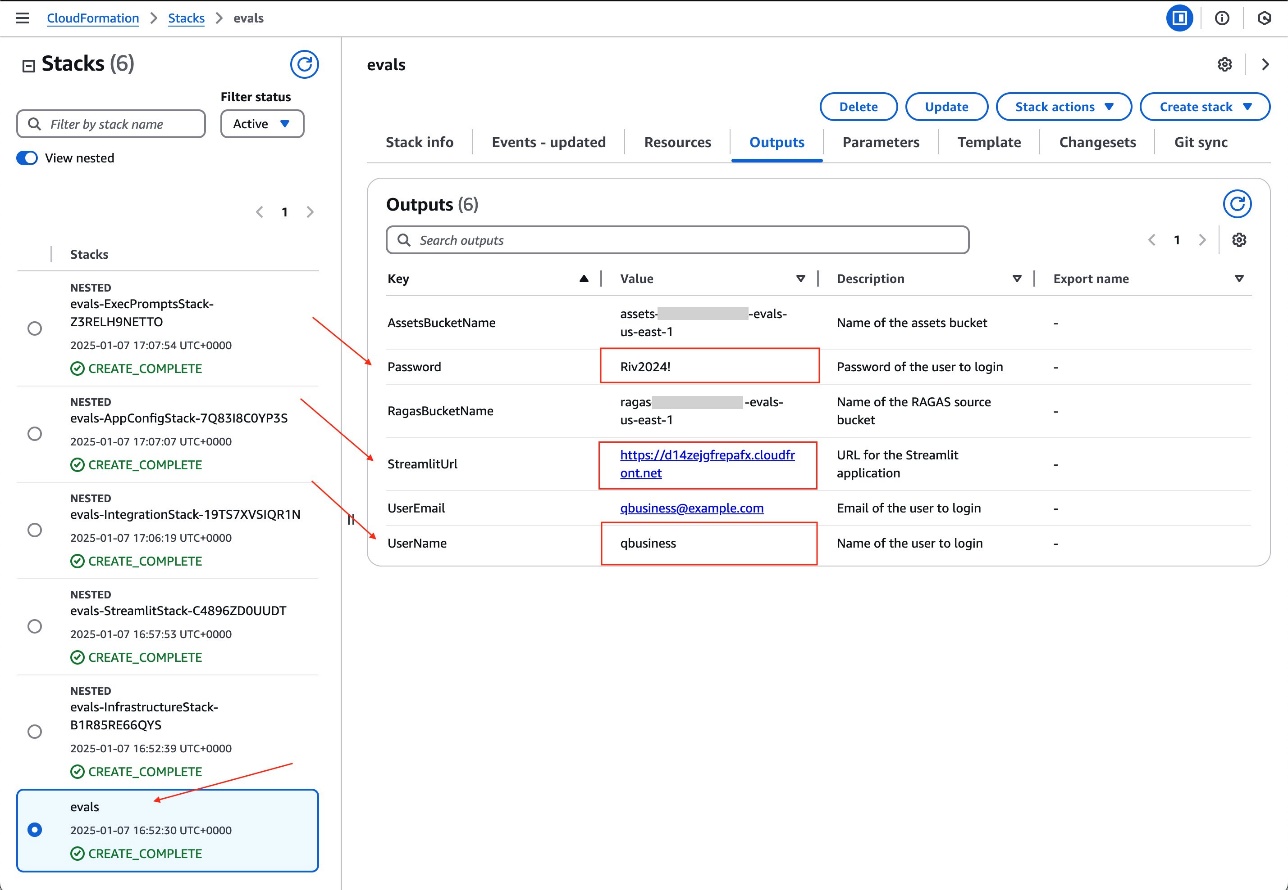
The custom UI will redirect you to the Amazon Cognito login page for authentication.
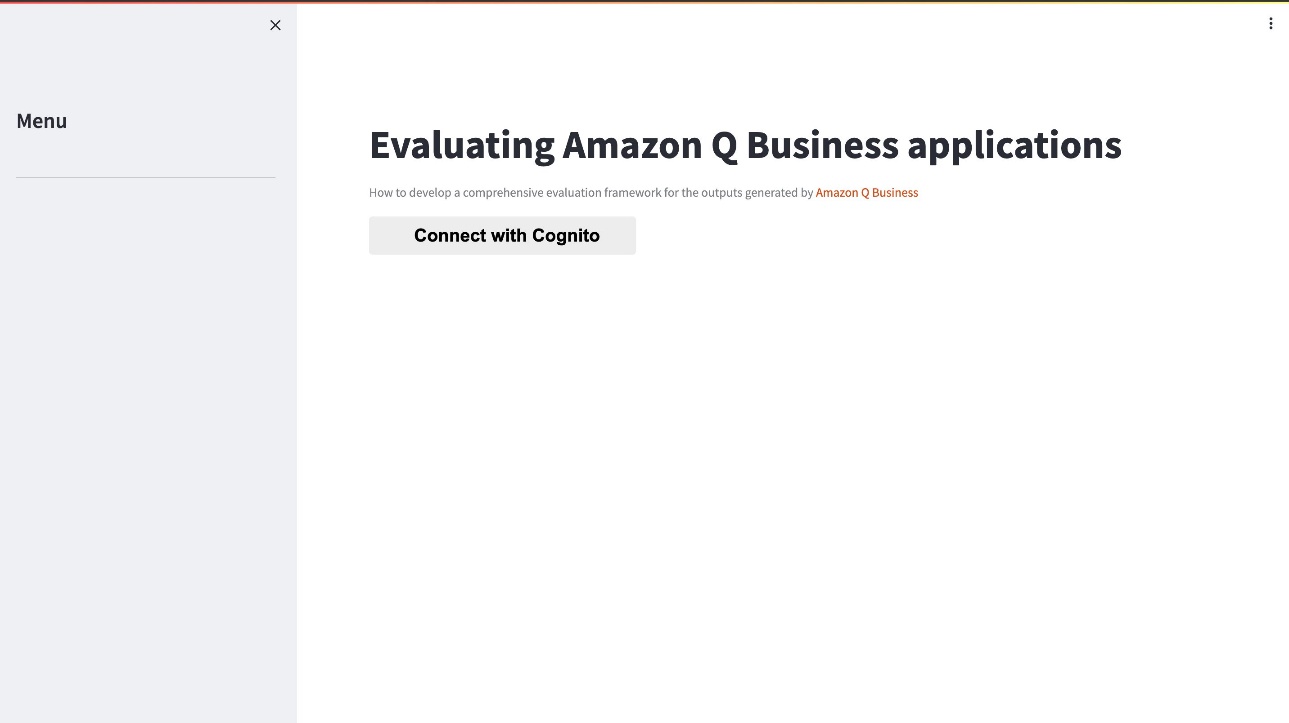
The UI application authenticates the user with Amazon Cognito, and initiates the token exchange workflow to implement a secure Chatsync API call with Amazon Q Business.
- Use the credentials you noted earlier to log in.
For more information about the token exchange flow between IAM Identity Center and the identity provider (IdP), refer to Building a Custom UI for Amazon Q Business.
- After you log in to the custom UI used for Amazon Q evaluation, choose Upload Dataset, then upload the dataset CSV file.
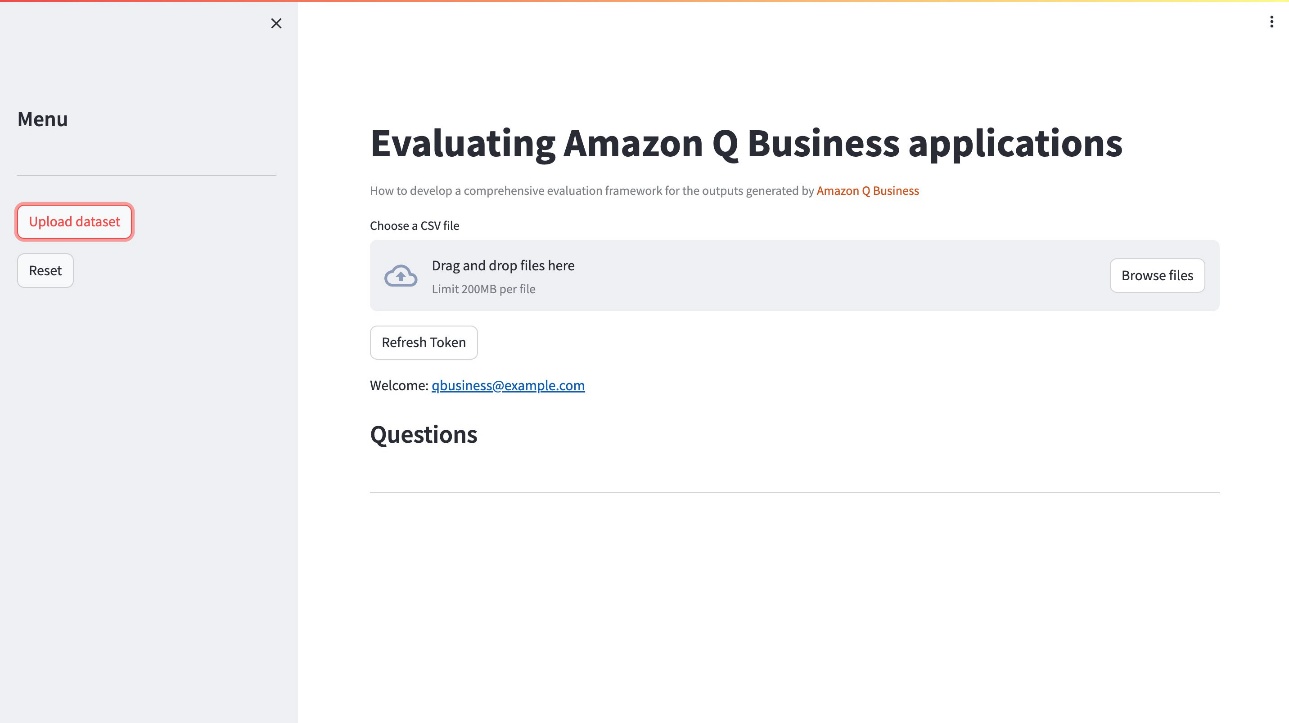
After the file is uploaded, the evaluation framework will send the prompt to Amazon Q Business to generate the answer, and then send the prompt, ground truth, and answer to Ragas to evaluate. During this process, you can also review the uploaded dataset (including the four questions and associated ground truth) on the Amazon Q Business console, as shown in the following screenshot.

After about 7 minutes, the workflow will finish, and you should see the evaluation result for first question.

Perform HITL evaluation
After the Lambda function has completed its execution, Ragas scoring will be shown in the custom UI. Now you can review metric scores generated using Ragas (an-LLM aided evaluation method), and you can provide human feedback as an evaluator to provide further calibration. This human-in-the-loop calibration can further improve the evaluation accuracy, because the HITL process is particularly valuable in fields where human judgment, expertise, or ethical considerations are crucial.
Let’s review the first question: “What are the index types of Amazon Q Business and the features of each?” You can read the question, Amazon Q Business generated answers, ground truth, and context.
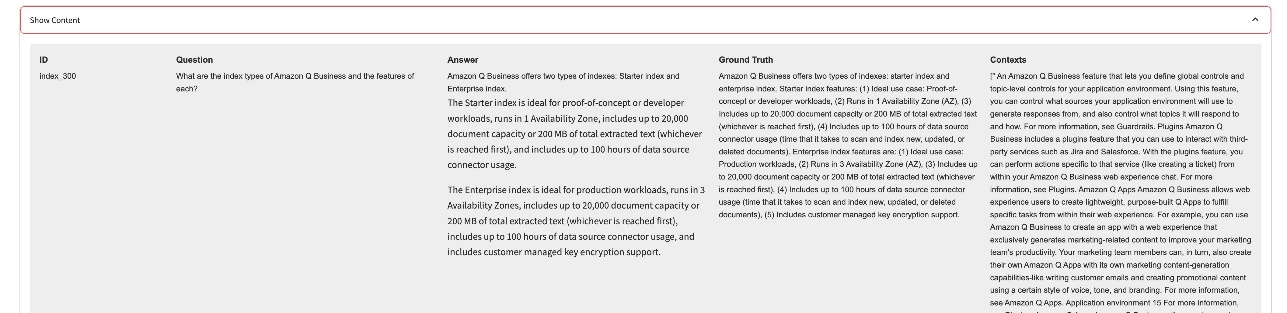
Next, review the evaluation metrics scored by using Ragas. As discussed earlier, there are four metrics:
- Answer relevancy – Measures relevancy of answers. Higher scores indicate better alignment with the user input, and lower scores are given if the response is incomplete or includes redundant information.
- Truthfulness – Verifies factual accuracy by comparing responses to verified sources. Higher scores indicate a better consistency with verified sources.
- Context precision – Assesses the relevance and conciseness of retrieved information. Higher scores indicate that the retrieved information closely matches the query intent, reducing irrelevant data.
- Context recall – Measures how many of the relevant documents (or pieces of information) were successfully retrieved. It focuses on not missing important results. Higher recall means fewer relevant documents were left out.
For this question, all metrics showed Amazon Q Business achieved a high-quality response. It’s worthwhile to compare your own evaluation with these scores generated by Ragas.

Next, let’s review a question that returned with a low answer relevancy score. For example: “I want to use Q Apps, which subscription tier is required to use Q Apps?”
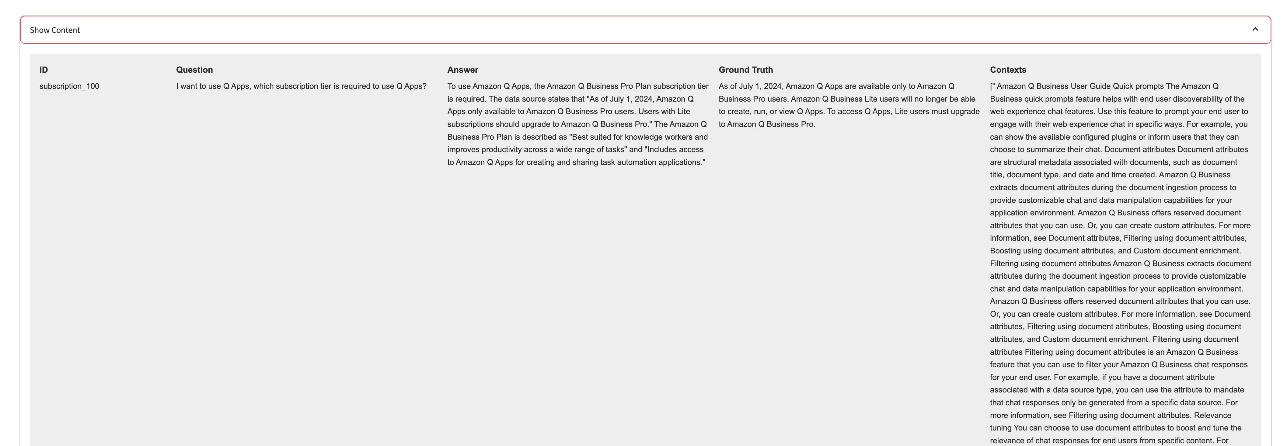
Analyzing both question and answer, we can consider the answer relevant and aligned with the user question, but the answer relevancy score from Ragas doesn’t reflect this human analysis, showing a lower score than expected. It’s important to calibrate Ragas evaluation judgement as Human in the Lopp. You should read the question and answer carefully, and make necessary changes of the metric score to reflect the HITL analysis. Finally, the results will be updated in DynamoDB.

Lastly, save the metric score in the CSV file, and you can download and review the final metric scores.
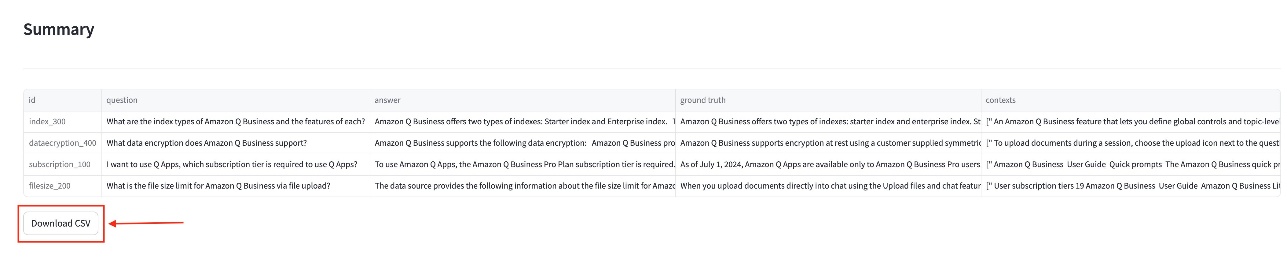
Solution 2: Lambda based evaluation
If you’re already using Amazon Q Business, AmazonQEvaluationLambda
allows for quick integration of evaluation methods into your application without setting up a custom UI application. It offers the following key features:
- Evaluates responses from Amazon Q Business using Ragas against a predefined test set of questions and ground truth data
- Outputs evaluation metrics that can be visualized directly in Amazon CloudWatch
- Both solutions provide you results based on the input dataset and the responses from the Amazon Q Business application, using Ragas to evaluate four key evaluation metrics (context recall, context precision, answer relevancy, and truthfulness).
This solution provides you sample code to evaluate the Amazon Q Business application response. To use this solution, you need to have or create a working Amazon Q Business application integrated with IAM Identity Center or Amazon Cognito as an IdP. This Lambda function works in the same way as the Lambda function in the end-to-end evaluation solution, using RAGAS against a test set of questions and ground truth. This lightweight solution doesn’t have a custom UI, but it can provide result metrics (context recall, context precision, answer relevancy, truthfulness), for visualization in CloudWatch. For deployment instructions, refer to the following GitHub repo.
Using evaluation results to improve Amazon Q Business application accuracy
This section outlines strategies to enhance key evaluation metrics—context recall, context precision, answer relevance, and truthfulness—for a RAG solution in the context of Amazon Q Business.
Context recall
Let’s examine the following problems and troubleshooting tips:
- Aggressive query filtering – Overly strict search filters or metadata constraints might exclude relevant records. You should review the metadata filters or boosting settings applied in Amazon Q Business to make sure they don’t unnecessarily restrict results.
- Data source ingestion errors – Documents from certain data sources aren’t successfully ingested into Amazon Q Business. To address this, check the document sync history report in Amazon Q Business to confirm successful ingestion and resolve ingestion errors.
Context precision
Consider the following potential issues:
- Over-retrieval of documents – Large top-K values might retrieve semi-related or off-topic passages, which the LLM might incorporate unnecessarily. To address this, refine metadata filters or apply boosting to improve passage relevance and reduce noise in the retrieved context.
- Poor query specificity – Broad or poorly formed user queries can yield loosely related results. You should make sure user queries are clear and specific. Train users or implement query refinement mechanisms to optimize query quality.
Answer relevance
Consider the following troubleshooting methods:
- Partial coverage – Retrieved context addresses parts of the question but fails to cover all aspects, especially in multi-part queries. To address this, decompose complex queries into sub-questions. Instruct the LLM or a dedicated module to retrieve and answer each sub-question before composing the final response. For example:
- Break down the query into sub-questions.
- Retrieve relevant passages for each sub-question.
- Compose a final answer addressing each part.
- Context/answer mismatch – The LLM might misinterpret retrieved passages, omit relevant information, or merge content incorrectly due to hallucination. You can use prompt engineering to guide the LLM more effectively. For example, for the original query “What are the top 3 reasons for X?” you can use the rewritten prompt “List the top 3 reasons for X clearly labeled as #1, #2, and #3, based strictly on the retrieved context.”
Truthfulness
Consider the following:
- Stale or inaccurate data sources – Outdated or conflicting information in the knowledge corpus might lead to incorrect answers. To address this, compare the retrieved context with verified sources to provide accuracy. Collaborate with SMEs to validate the data.
- LLM hallucination – The model might fabricate or embellish details, even with accurate retrieved context. Although Amazon Q Business is a RAG generative AI solution, and should significantly reduce the hallucination, it’s not possible to eliminate hallucination totally. You can measure the frequency of low context precision answers to identify patterns and quantify the impact of hallucinations to gain an aggregated view with the evaluation solution.
By systematically examining and addressing the root causes of low evaluation metrics, you can optimize your Amazon Q Business application. From document retrieval and ranking to prompt engineering and validation, these strategies will help enhance the effectiveness of your RAG solution.
Clean up
Don’t forget to go back to the CloudFormation console and delete the CloudFormation stack to delete the underlying infrastructure that you set up, to avoid additional costs on your AWS account.
Conclusion
In this post, we outlined two evaluation solutions for Amazon Q Business: a comprehensive evaluation workflow and a lightweight Lambda based evaluation. These approaches combine automated evaluation approaches such as Ragas with human-in-the-loop validation, providing reliable and accurate assessments.
By using our guidance on how to improve evaluation metrics, you can continuously optimize your Amazon Q Business application to meet enterprise needs with Amazon Q Business. Whether you’re using the end-to-end solution or the lightweight approach, these frameworks provide a scalable and efficient path to improve accuracy and relevance.
To learn more about Amazon Q Business and how to evaluate Amazon Q Business results, explore these hands-on workshops:
About the authors
Rui Cardoso is a partner solutions architect at Amazon Web Services (AWS). He is focusing on AI/ML and IoT. He works with AWS Partners and support them in developing solutions in AWS. When not working, he enjoys cycling, hiking and learning new things.
Julia Hu is a Sr. AI/ML Solutions Architect at Amazon Web Services. She is specialized in Generative AI, Applied Data Science and IoT architecture. Currently she is part of the Amazon Bedrock team, and a Gold member/mentor in Machine Learning Technical Field Community. She works with customers, ranging from start-ups to enterprises, to develop AWSome generative AI solutions. She is particularly passionate about leveraging Large Language Models for advanced data analytics and exploring practical applications that address real-world challenges.
Amit Gupta is a Senior Q Business Solutions Architect Solutions Architect at AWS. He is passionate about enabling customers with well-architected generative AI solutions at scale.
Neil Desai is a technology executive with over 20 years of experience in artificial intelligence (AI), data science, software engineering, and enterprise architecture. At AWS, he leads a team of Worldwide AI services specialist solutions architects who help customers build innovative Generative AI-powered solutions, share best practices with customers, and drive product roadmap. He is passionate about using technology to solve real-world problems and is a strategic thinker with a proven track record of success.
Ricardo Aldao is a Senior Partner Solutions Architect at AWS. He is a passionate AI/ML enthusiast who focuses on supporting partners in building generative AI solutions on AWS.
Read More