Keeping an up-to-date asset inventory with real devices deployed in the field can be a challenging and time-consuming task. Many electricity providers use manufacturer’s labels as key information to link their physical assets within asset inventory systems. Computer vision can be a viable solution to speed up operator inspections and reduce human errors by automatically extracting relevant data from the label. However, building a standard computer vision application capable of managing hundreds of different types of labels can be a complex and time-consuming endeavor.
In this post, we present a solution using generative AI and large language models (LLMs) to alleviate the time-consuming and labor-intensive tasks required to build a computer vision application, enabling you to immediately start taking pictures of your asset labels and extract the necessary information to update the inventory using AWS services like AWS Lambda, Amazon Bedrock, Amazon Titan, Anthropic’s Claude 3 on Amazon Bedrock, Amazon API Gateway, AWS Amplify, Amazon Simple Storage Service (Amazon S3), and Amazon DynamoDB.
LLMs are large deep learning models that are pre-trained on vast amounts of data. They are capable of understanding and generating human-like text, making them incredibly versatile tools with a wide range of applications. This approach harnesses the image understanding capabilities of Anthropic’s Claude 3 model to extract information directly from photographs taken on-site, by analyzing the labels present in those field images.
Solution overview
The AI-powered asset inventory labeling solution aims to streamline the process of updating inventory databases by automatically extracting relevant information from asset labels through computer vision and generative AI capabilities. The solution uses various AWS services to create an end-to-end system that enables field technicians to capture label images, extract data using AI models, verify the accuracy, and seamlessly update the inventory database.
The following diagram illustrates the solution architecture.
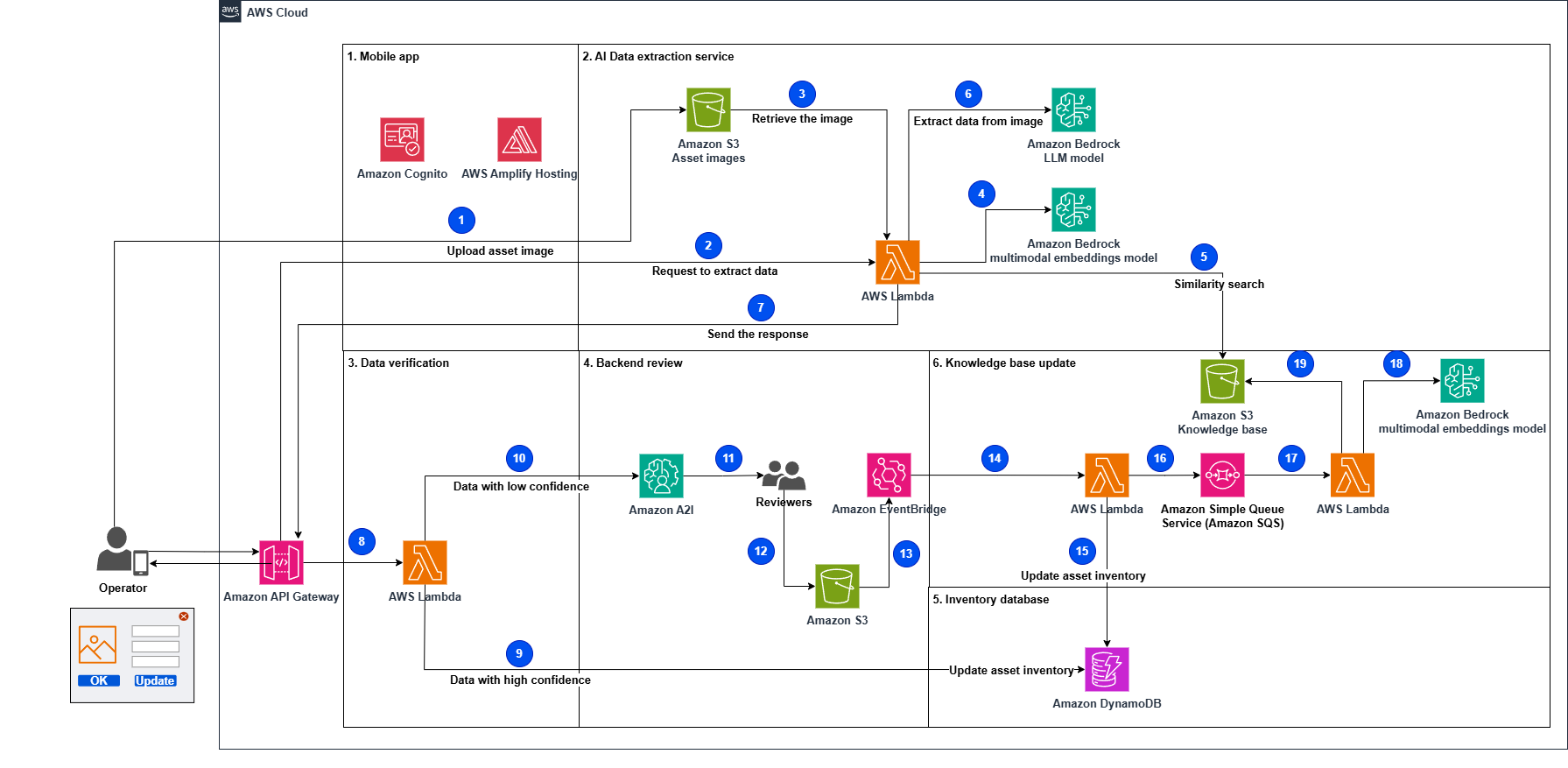
The workflow consists of the following steps:
- The process starts when an operator takes and uploads a picture of the assets using the mobile app.
- The operator submits a request to extract data from the asset image.
- A Lambda function retrieves the uploaded asset image from the uploaded images data store.
- The function generates the asset image embeddings (vector representations of data) invoking the Amazon Titan Multimodal Embeddings G1 model.
- The function performs a similarity search in the knowledge base to retrieve similar asset labels. The most relevant results will augment the prompt as similar examples to improve the response accuracy, and are sent with the instructions to the LLM to extract data from the asset image.
- The function invokes Anthropic’s Claude 3 Sonnet on Amazon Bedrock to extract data (serial number, vendor name, and so on) using the augmented prompt and the related instructions.
- The function sends the response to the mobile app with the extracted data.
- The mobile app verifies the extracted data and assigns a confidence level. It invokes the API to process the data. Data with high confidence will be directly ingested into the system.
- A Lambda function is invoked to update the asset inventory database with the extracted data if the confidence level has been indicated as high by the mobile app.
- The function sends data with low confidence to Amazon Augmented AI (Amazon A2I) for further processing.
- The human reviewers from Amazon A2I validate or correct the low-confidence data.
- Human reviewers, such as subject matter experts, validate the extracted data, flag it, and store it in an S3 bucket.
- A rule in Amazon EventBridge is defined to trigger a Lambda function to get the information from the S3 bucket when the Amazon A2I workflow processing is complete.
- A Lambda function processes the output of the Amazon A2I workflow by loading data from the JSON file that stored the backend operator-validated information.
- The function updates the asset inventory database with the new extracted data.
- The function sends the extracted data marked as new by human reviewers to an Amazon Simple Queue Service (Amazon SQS) queue to be further processed.
- Another Lambda function fetches messages from the queue and serializes the updates to the knowledge base database.
- The function generates the asset image embeddings by invoking the Amazon Titan Multimodal Embeddings G1 model.
- The function updates the knowledge base with the generated embeddings and notifies other functions that the database has been updated.
Let’s look at the key components of the solution in more detail.
Mobile app
The mobile app component plays a crucial role in this AI-powered asset inventory labeling solution. It serves as the primary interface for field technicians on their tablets or mobile devices to capture and upload images of asset labels using the device’s camera. The implementation of the mobile app includes an authentication mechanism that will allow access only to authenticated users. It’s also built using a serverless approach to minimize recurring costs and have a highly scalable and robust solution.
The mobile app has been built using the following services:
- AWS Amplify – This provides a development framework and hosting for the static content of the mobile app. By using Amplify, the mobile app component benefits from features like seamless integration with other AWS services, offline capabilities, secure authentication, and scalable hosting.
- Amazon Cognito – This handles user authentication and authorization for the mobile app.
AI data extraction service
The AI data extraction service is designed to extract critical information, such as manufacturer name, model number, and serial number from images of asset labels.
To enhance the accuracy and efficiency of the data extraction process, the service employs a knowledge base comprising sample label images and their corresponding data fields. This knowledge base serves as a reference guide for the AI model, enabling it to learn and generalize from labeled examples to new label formats effectively. The knowledge base is stored as vector embeddings in a high-performance vector database: Meta’s FAISS (Facebook AI Similarity Search), hosted on Amazon S3.
Embeddings are dense numerical representations that capture the essence of complex data like text or images in a vector space. Each data point is mapped to a vector or ordered list of numbers, where similar data points are positioned closer together. This embedding space allows for efficient similarity calculations by measuring the distance between vectors. Embeddings enable machine learning (ML) models to effectively process and understand relationships within complex data, leading to improved performance on various tasks like natural language processing and computer vision.
The following diagram illustrates an example workflow.
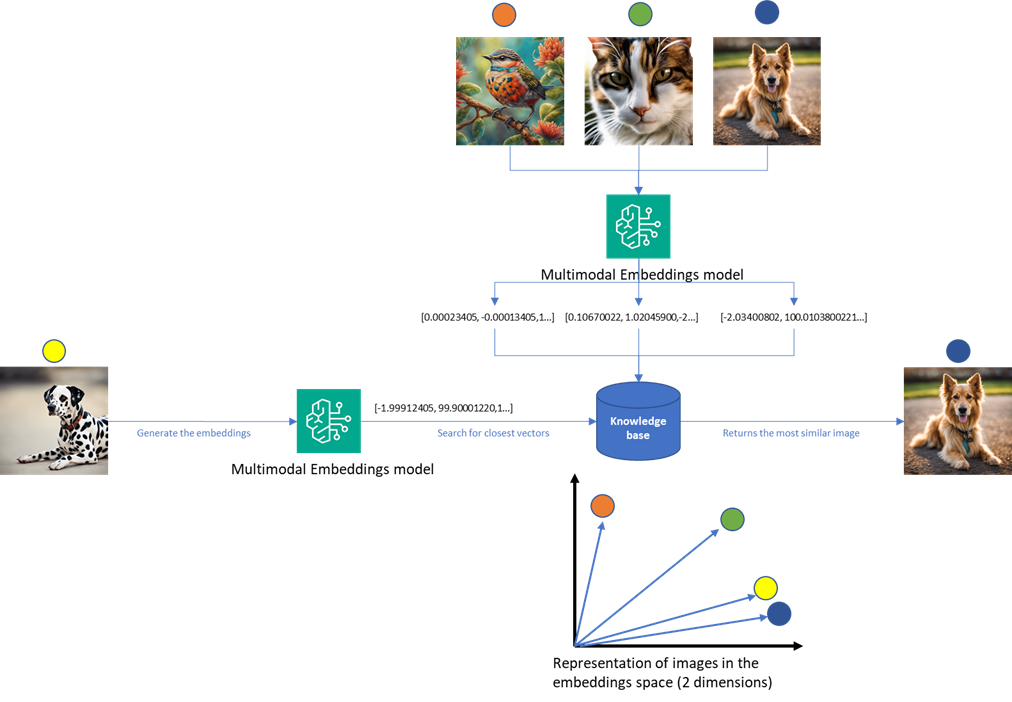
The vector embeddings are generated using Amazon Titan, a powerful embedding generation service, which converts the labeled examples into numerical representations suitable for efficient similarity searches. The workflow consists of the following steps:
- When a new asset label image is submitted for processing, the AI data extraction service, through a Lambda function, retrieves the uploaded image from the bucket where it was uploaded.
- The Lambda function performs a similarity search using Meta’s FAISS vector search engine. This search compares the new image against the vector embeddings in the knowledge base generated by Amazon Titan Multimodal Embeddings invoked through Amazon Bedrock, identifying the most relevant labeled examples.
- Using the augmented prompt with context information from the similarity search, the Lambda function invokes Amazon Bedrock, specifically Anthropic’s Claude 3, a state-of-the-art generative AI model, for image understanding and optical character recognition (OCR) tasks. By using the similar examples, the AI model can more accurately extract and interpret the critical information from the new asset label image.
- The response is then sent to the mobile app to be confirmed by the field technician.
In this phase, the AWS services used are:
- Amazon Bedrock – A fully managed service that offers a choice of high-performing foundation models (FMs) from leading AI companies like AI21 Labs, Anthropic, Cohere, Meta, Mistral AI, Stability AI, and Amazon through a single API, along with a broad set of capabilities.
- AWS Lambda – A serverless computing service that allows you to run your code without the need to provision or manage physical servers or virtual machines. A Lambda function runs the data extraction logic and orchestrates the overall data extraction process.
- Amazon S3 – A storage service offering industry-leading durability, availability, performance, security, and virtually unlimited scalability at low costs. It’s used to store the asset images uploaded by the field technicians.
Data verification
Data verification plays a crucial role in maintaining the accuracy and reliability of the extracted data before updating the asset inventory database and is included in the mobile app.
The workflow consists of the following steps:
- The extracted data is shown to the field operator.
- If the field operator determines that the extracted data is accurate and matches an existing asset label in the knowledge base, they can confirm the correctness of the extraction; if not, they can update the values directly using the app.
- When the field technician confirms the data is correct, that information is automatically forwarded to the backend review component.
Data verification uses the following AWS services:
- Amazon API Gateway – A secure and scalable API gateway that exposes the data verification component’s functionality to the mobile app and other components.
- AWS Lambda – Serverless functions for implementing the verification logic and routing data based on confidence levels.
Backend review
This component assesses the discrepancy of automatically identified data by the AI data extraction service and the final data approved by the field operator and computes the difference. If the difference is below a configured threshold, the data is sent to update the inventory database; otherwise a human review process is engaged:
- Subject matter experts asynchronously review flagged data entries on the Amazon A2I console.
- Significant discrepancies are marked to update the generative AI’s knowledge base.
- Minor OCR errors are corrected without updating the AI model’s knowledge base.
The backend review component uses the following AWS services:
- Amazon A2I – A service that provides a web-based interface for human reviewers to inspect and correct the extracted data and asset label images.
- Amazon EventBridge – A serverless service that uses events to connect application components together. When the Amazon A2I human workflow is complete, EventBridge is used to detect this event and trigger a Lambda function to process the output data.
- Amazon S3 – Object storage to save the marked information in charge of Amazon A2I.
Inventory database
The inventory database component plays a crucial role in storing and managing the verified asset data in a scalable and efficient manner. Amazon DynamoDB, a fully managed NoSQL database service from AWS, is used for this purpose. DynamoDB is a serverless, scalable, and highly available key-value and document database service. It’s designed to handle massive amounts of data and high traffic workloads, making it well-suited for storing and retrieving large-scale inventory data.
The verified data from the AI extraction and human verification processes is ingested into the DynamoDB table. This includes data with high confidence from the initial extraction, as well as data that has been reviewed and corrected by human reviewers.
Knowledge base update
The knowledge base update component enables continuous improvement and adaptation of the generative AI models used for asset label data extraction:
- During the backend review process, human reviewers from Amazon A2I validate and correct the data extracted from asset labels by the AI model.
- The corrected and verified data, along with the corresponding asset label images, is marked as new label examples if not already present in the knowledge base.
- A Lambda function is triggered to update the asset inventory and send the new labels to the FIFO (First-In-First-Out) queue.
- A Lambda function processes the messages in the queue, updating the knowledge base vector store (S3 bucket) with the new label examples.
- The update process generates the vector embeddings by invoking the Amazon Titan Multimodal Embeddings G1 model exposed by Amazon Bedrock and storing the embeddings in a Meta’s FAISS database in Amazon S3.
The knowledge base update process makes sure that the solution remains adaptive and continuously improves its performance over time, reducing the likelihood of unseen label examples and the involvement of subject matter experts to correct the extracted data.
This component uses the following AWS services:
- Amazon Titan Multimodal Embeddings G1 model – This model generates the embeddings (vector representations) for the new asset images and their associated data.
- AWS Lambda – Lambda functions are used to update the asset inventory database, to send and process the extracted data to the FIFO queue, and to update the knowledge base in case of new unseen labels.
- Amazon SQS – Amazon SQS offers fully managed message queuing for microservices, distributed systems, and serverless applications. The extracted data marked as new by human reviewers is sent to an SQS FIFO (First-In-First-Out) queue. This makes sure that the messages are processed in the correct order; FIFO queues preserve the order in which messages are sent and received. If you use a FIFO queue, you don’t have to place sequencing information in your messages.
- Amazon S3 – The knowledge base is stored in an S3 bucket, with the newly generated embeddings. This allows the AI system to improve its accuracy for future asset label recognition tasks.
Navigation flow
This section explains how users interact with the system and how data flows between different components of the solution. We’ll examine each key component’s role in the process, from initial user access through data verification and storage.
Mobile app
The end user accesses the mobile app using the browser included in the handheld device. The application URL to access the mobile app is available after you have deployed the frontend application. Using the browser on a handheld device or your PC, browse to the application URL address, where a login window will appear. Because this is a demo environment, you can register on the application by following the automated registration workflow implemented through Amazon Cognito and choosing Create Account, as shown in the following screenshot.
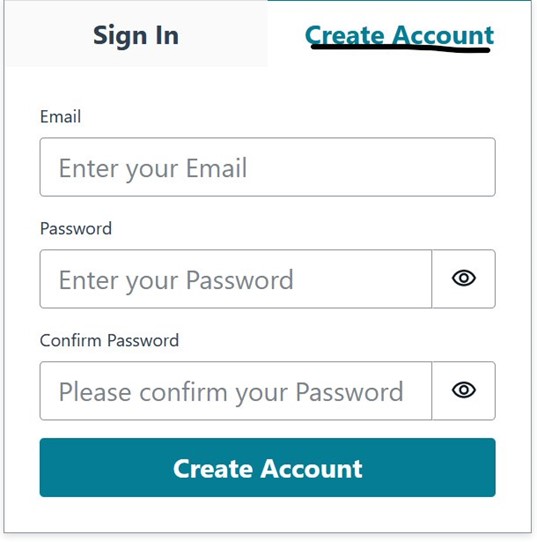
During the registration process, you must provide a valid email address that will be used to verify your identity, and define a password. After you’re registered, you can log in with your credentials.
After authentication is complete, the mobile app appears, as shown in the following screenshot.
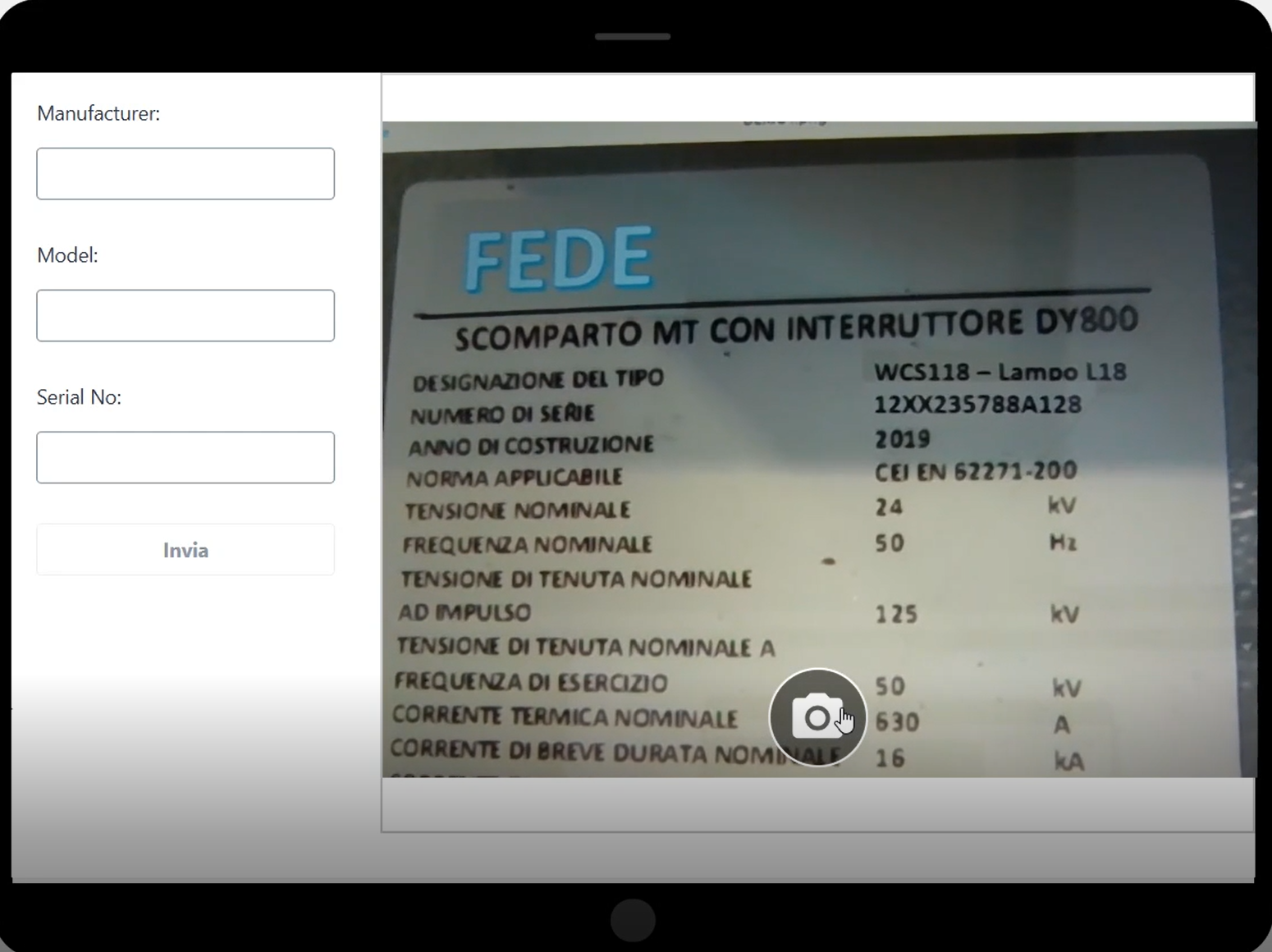
The process to use the app is the following:
- Use the camera button to capture a label image.
- The app facilitates the upload of the captured image to a private S3 bucket specifically designated for storing asset images. S3 Transfer Acceleration is a separate AWS service that can be integrated with Amazon S3 to improve the transfer speed of data uploads and downloads. It works by using AWS edge locations, which are globally distributed and closer to the client applications, as intermediaries for data transfer. This reduces the latency and improves the overall transfer speed, especially for clients that are geographically distant from the S3 bucket’s AWS Region.
- After the image is uploaded, the app sends a request to the AI data extraction service, triggering the subsequent process of data extraction and analysis. The extracted data returned by the service is displayed and editable within the form, as described later in this post. This allows for data verification.
AI data extraction service
This module uses Anthropic’s Claude 3 FM, a multimodal system capable of processing both images and text. To extract relevant data, we employ a prompt technique that uses samples to guide the model’s output. Our prompt includes two sample images along with their corresponding extracted text. The model identifies which sample image most closely resembles the one we want to analyze and uses that sample’s extracted text as a reference to determine the relevant information in the target image.
We use the following prompt to achieve this result:
{
"role": "user",
"content": [
{
"type": "text",
"text": "first_sample_image:",
},
{
"type": "image",
"source": {
"type": "base64",
"media_type": "image/jpeg",
"data": first_sample_encoded_image,
},
},
{
"type": "text",
"text": "target_image:",
},
{
"type": "image",
"source": {
"type": "base64",
"media_type": "image/jpeg",
"data": encoded_image,
},
},
{"type": "text",
"text": f"""
answer the question using the following example as reference.
match exactly the same set of fields and information as in the provided example.
<example>
analyze first_sample_image and answer with a json file with the following information: Model, SerialN, ZOD.
answer only with json.
Answer:
{first_sample_answer}
</example>
<question>
analyze target_image and answer with a json file with the following information: Model, SerialN, ZOD.
answer only with json.
Answer:
</question>
"""},
],
}
In the preceding code, first_sample_encoded_image
and first_sample_answer
are the reference image and expected output, respectively, and encoded_image
contains the new image that has to be analyzed.
Data verification
After the image is processed by the AI data extraction service, the control goes back to the mobile app:
- The mobile app receives the extracted data from the AI data extraction service, which has processed the uploaded asset label image and extracted relevant information using computer vision and ML models.
- Upon receiving the extracted data, the mobile app presents it to the field operator, allowing them to review and confirm the accuracy of the information (see the following screenshot). If the extracted data is correct and matches the physical asset label, the technician can submit a confirmation through the app, indicating that the data is valid and ready to be inserted into the asset inventory database.
- If the field operator sees any discrepancies or errors in the extracted data compared to the actual asset label, they have the option to correct those values.
- The values returned by the AI data extraction service and the final values validated by the field operators are sent to the backend review service.
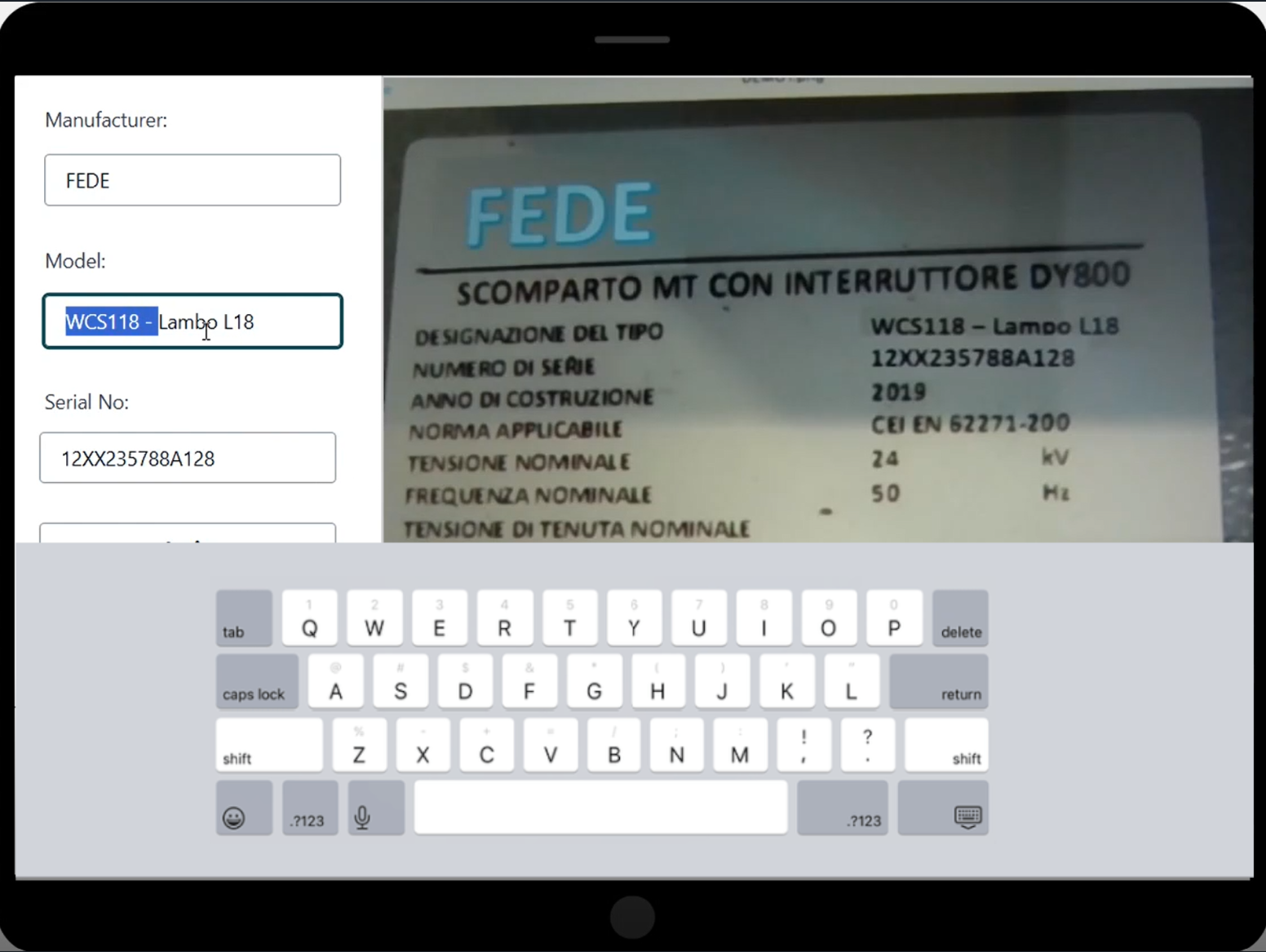
Backend review
This process is implemented using Amazon A2I:
- A distance metric is computed to evaluate the difference between what the data extraction service has identified and the correction performed by the on-site operator.
- If the difference is larger than a predefined threshold, the image and the operator modified data are submitted to an Amazon A2I workflow, creating a human-in-the-loop request.
- When a backend operator becomes available, the new request is assigned.
- The operator uses the Amazon A2I provided web interface, as depicted in the following screenshot, to check what the on-site operator has done and, if it’s found that this type of label is not included in the knowledge base, can decide to add it by entering Yes in the Add to Knowledge Base field.
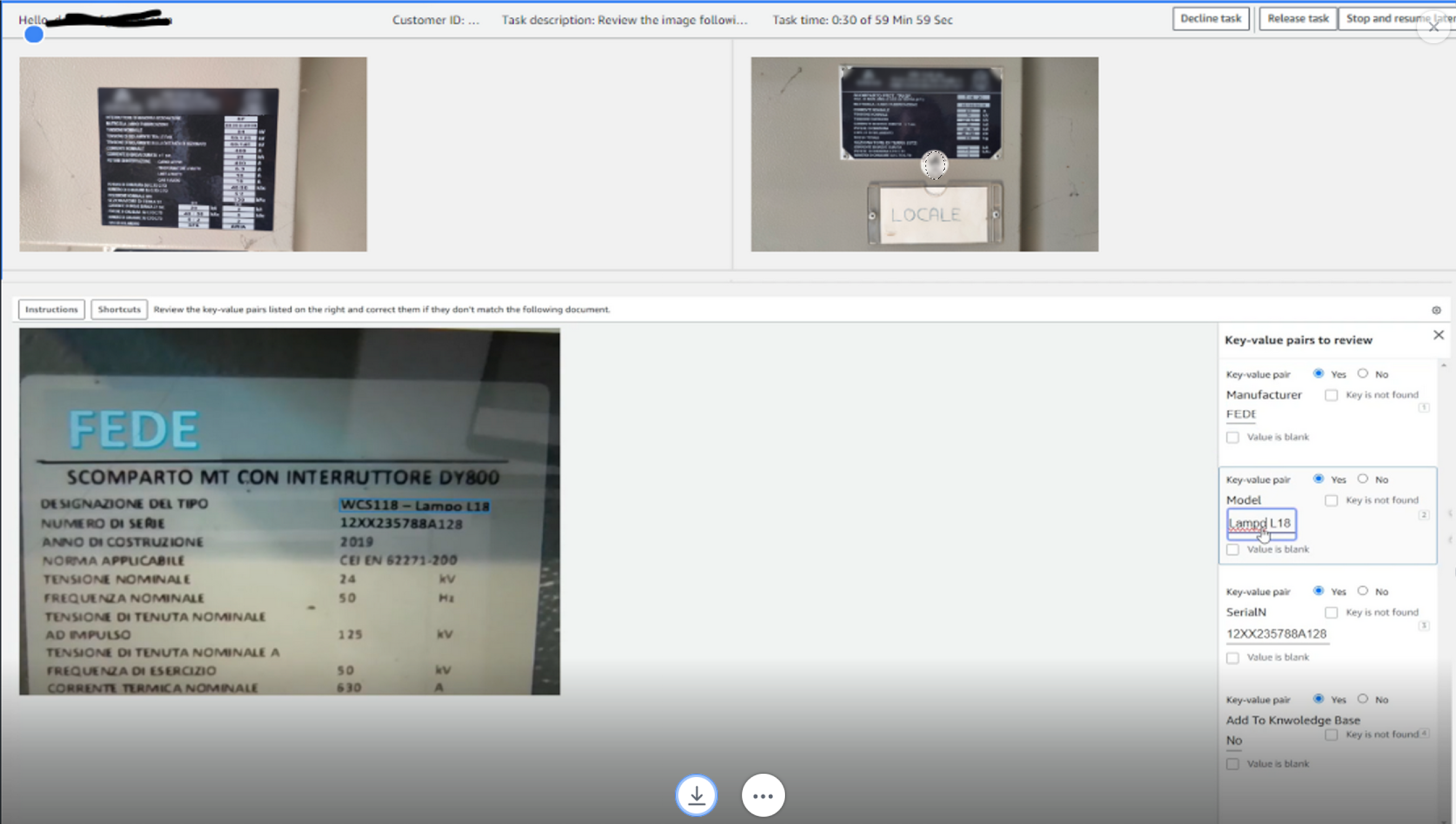
- When the A2I process is complete, a Lambda function is triggered.
- This Lambda function stores the information in the inventory database and verifies whether this image also needs to be used to update the knowledge base.
- If this is the case, the Lambda function files the request with the relevant data in an SQS FIFO queue.
Inventory database
To keep this solution as simple as possible while covering the required capability, we selected DynamoDB as our inventory database. This is a no SQL database, and we will store data in a table with the following information:
- Manufacturers, model ID, and the serial number that is going to be the key of the table
- A link to the picture containing the label used during the on-site inspection
DynamoDB offers an on-demand pricing model that allows costs to directly depend on actual database usage.
Knowledge base database
The knowledge base database is stored as two files in an S3 bucket:
- The first file is a JSON array containing the metadata (manufacturer, serial number, model ID, and link to reference image) for each of the knowledge base entries
- The second file is a FAISS database containing an index with the embedding for each of the images included in the first file
To be able to minimize race conditions when updating the database, a single Lambda function is configured as the consumer of the SQS queue. The Lambda function extracts the information about the link to the reference image and the metadata, certified by the back-office operator, updates both files, and stores the new version in the S3 bucket.
In the following sections, we create a seamless workflow for field data collection, AI-powered extraction, human validation, and inventory updates.
Prerequisites
You need the following prerequisites before you can proceed with solution. For this post, we use the us-east-1
Region. You will also need an AWS Identity and Access Management (IAM) user with administrative privileges to deploy the required components and a development environment with access to AWS resources already configured.
For the development environment, you can use an Amazon Elastic Compute Cloud (Amazon EC2) instance (choose select at least a t3.small instance type in order to be able to build the web application) or use a development environment of your own choice. Install Python 3.9 and install and configure AWS Command Line Interface (AWS CLI).
You will also need to install the Amplify CLI. Refer to Set up Amplify CLI for more information.
The next step is to enable the models used in this workshop in Amazon Bedrock. To do this, complete the following steps:
-
- On the Amazon Bedrock console, choose Model access in the navigation pane.
- Choose Enable specific models.
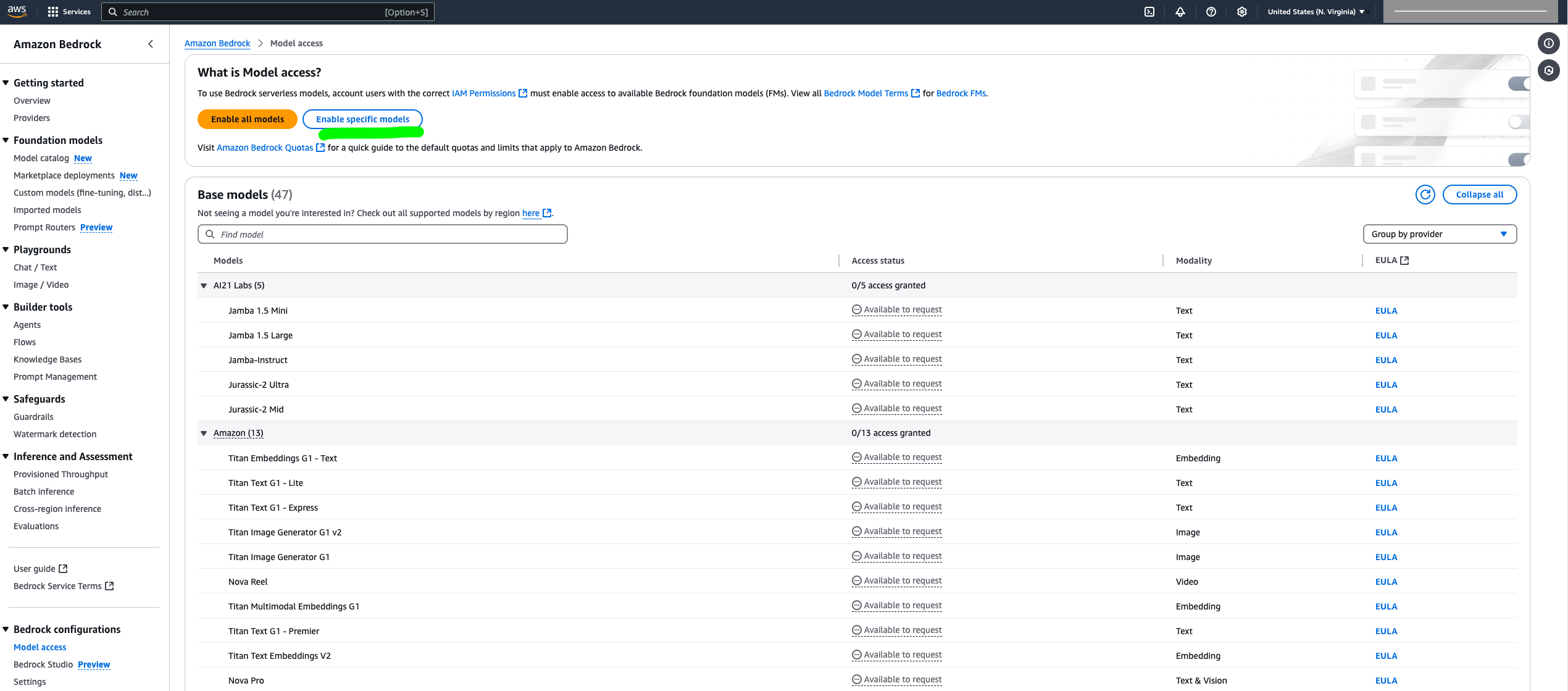
-
- Select all Anthropic and Amazon models and choose Next
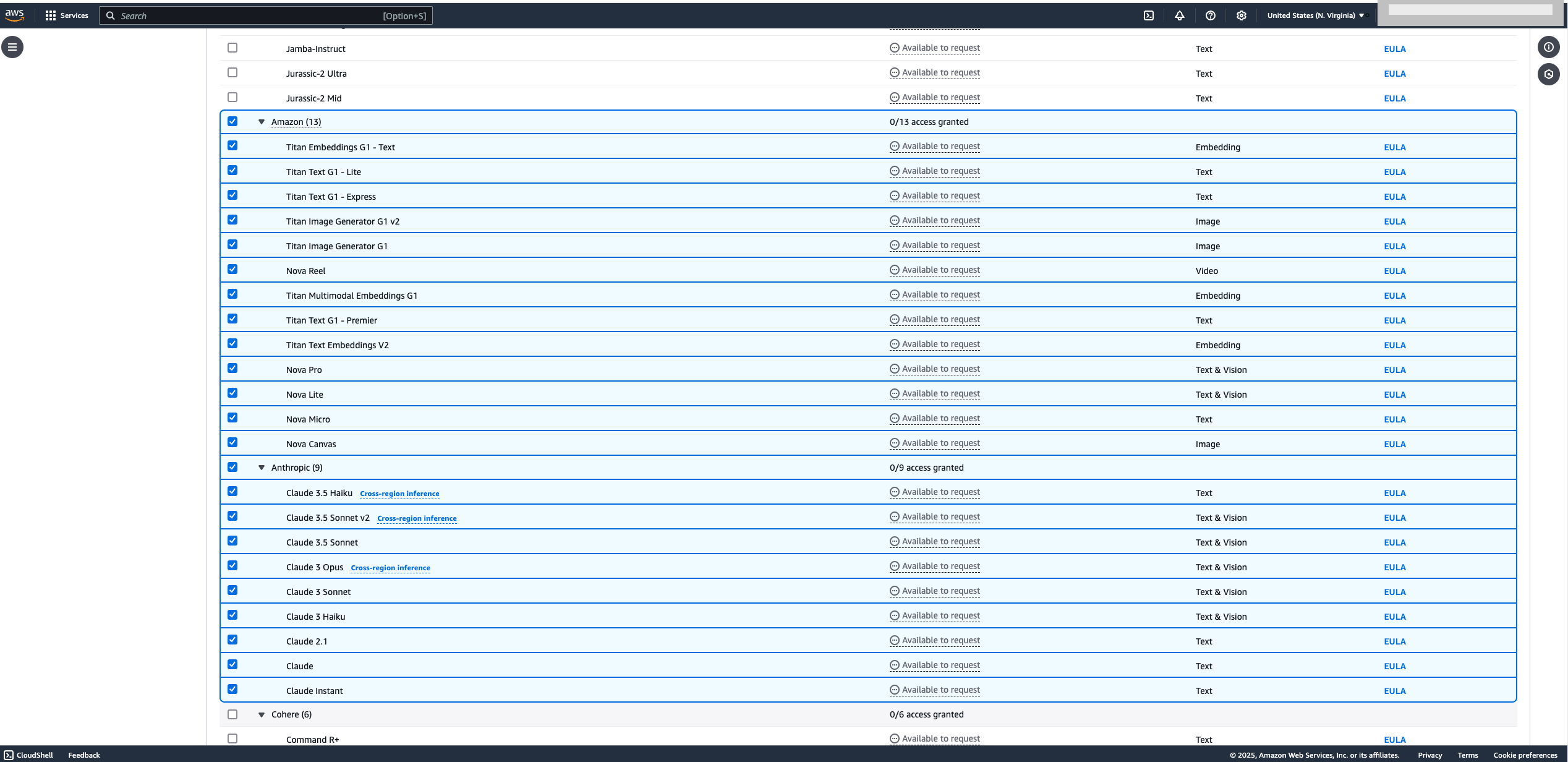
A new window will list the requested models.
- Confirm that the Amazon Titan models and Anthropic Claude models are on this list and choose Submit.
The next step is to create an Amazon SageMaker Ground Truth private labeling workforce that will be used to perform back-office activities. If you don’t already have a private labeling workforce in your account, you can create one following these steps:
- On the SageMaker console, under Ground Truth in the navigation pane, choose Labeling workforce.
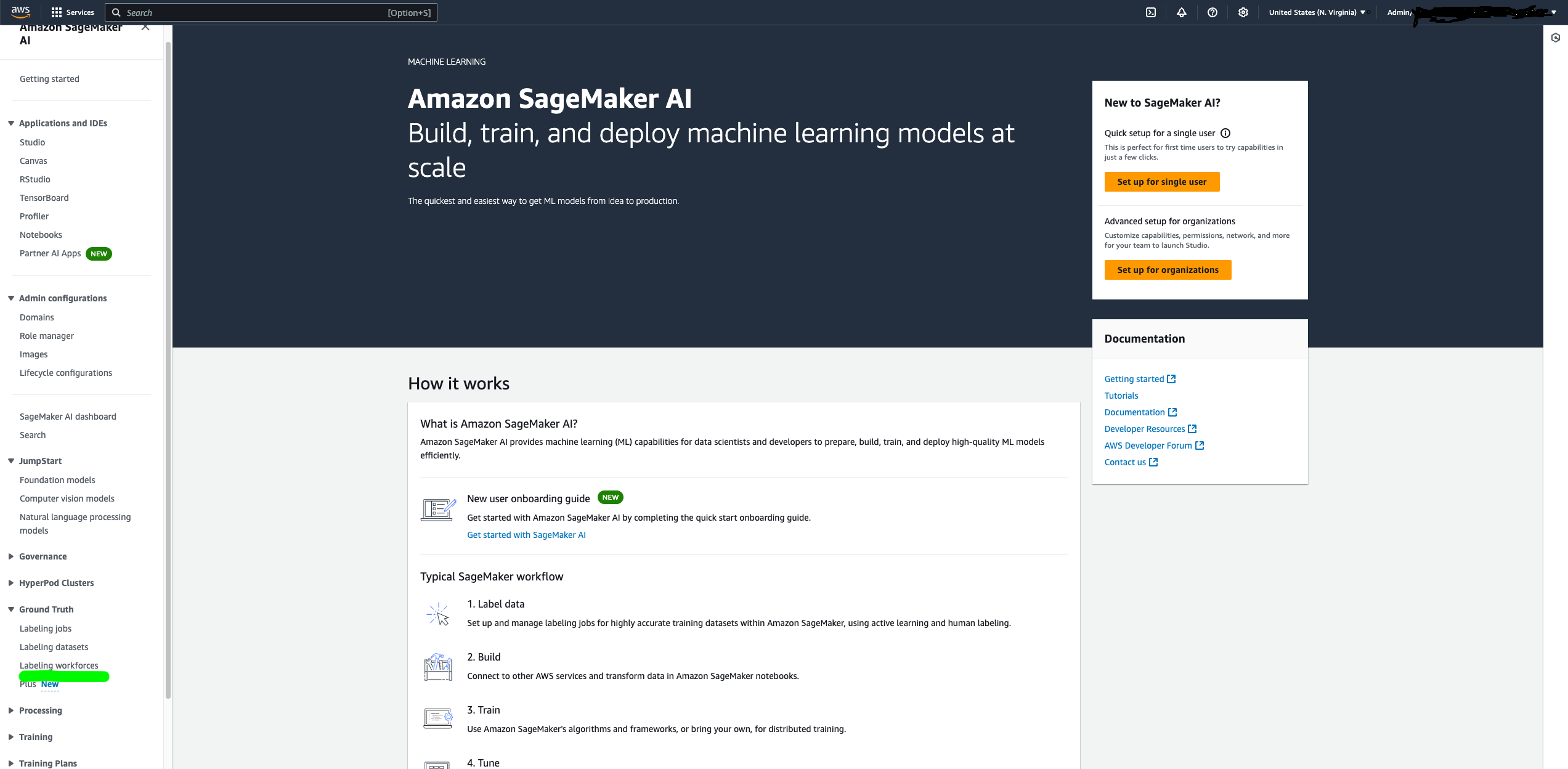
- On the Private tab, choose Create private team.
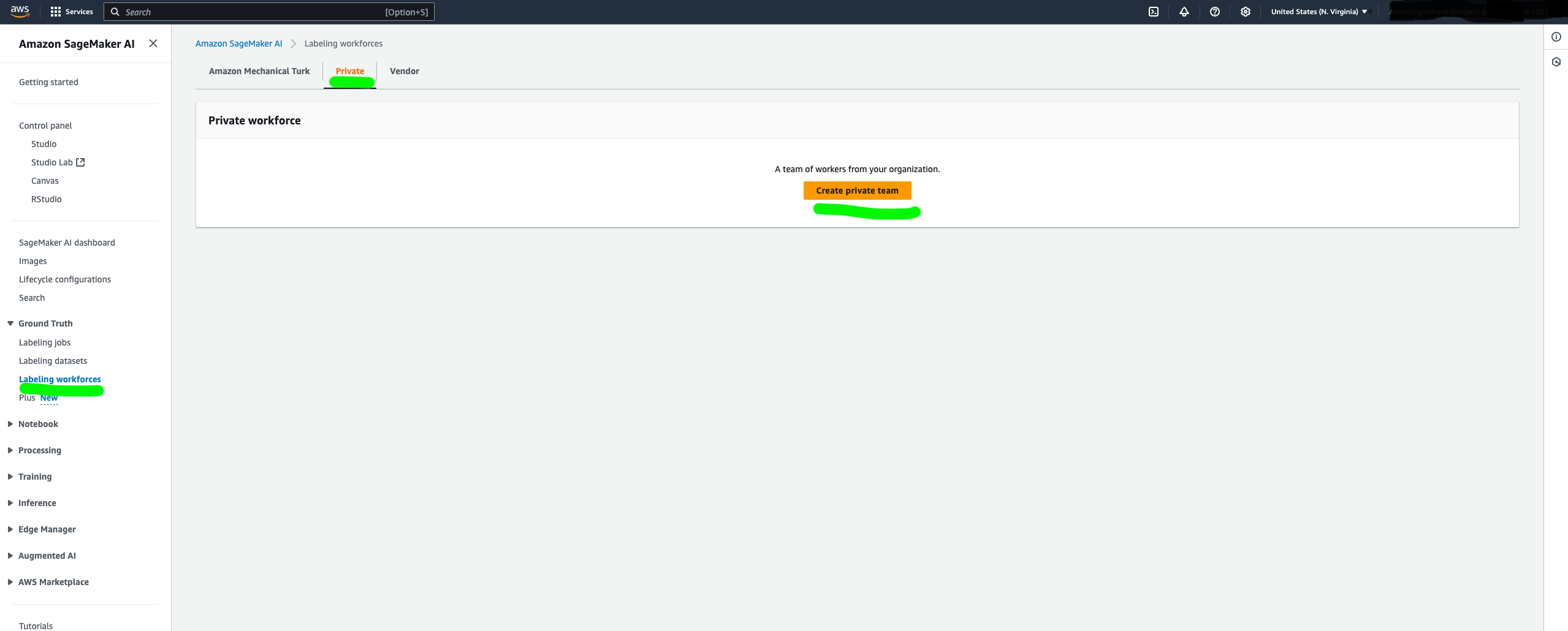
- Provide a name to the team and your organization, and insert your email address (must be a valid one) for both Email addresses and Contact email.
- Leave all the other options as default.
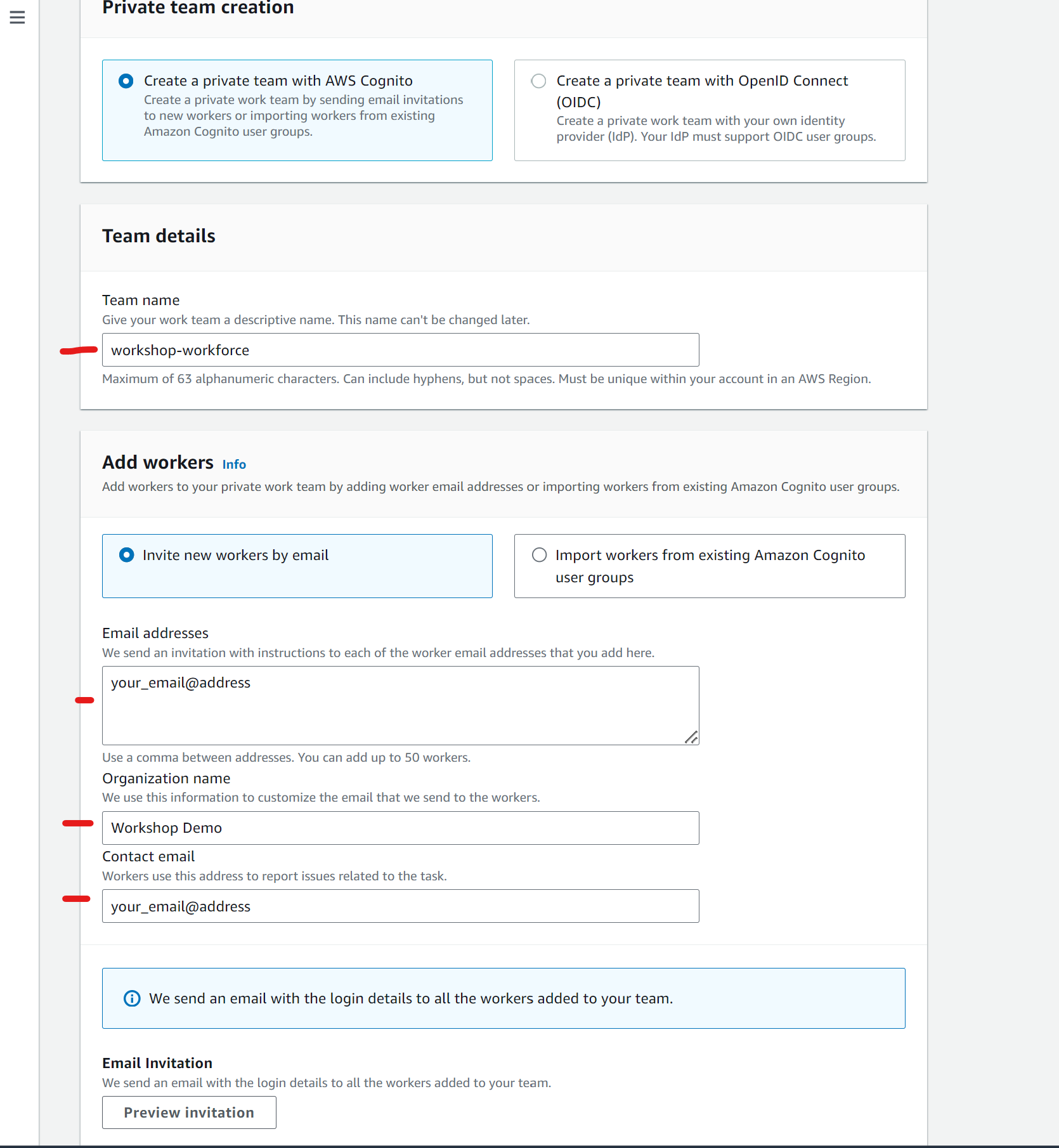
- Choose Create private team.
- After your workforce is created, copy your workforce Amazon Resource Name (ARN) on the Private tab and save for later use.
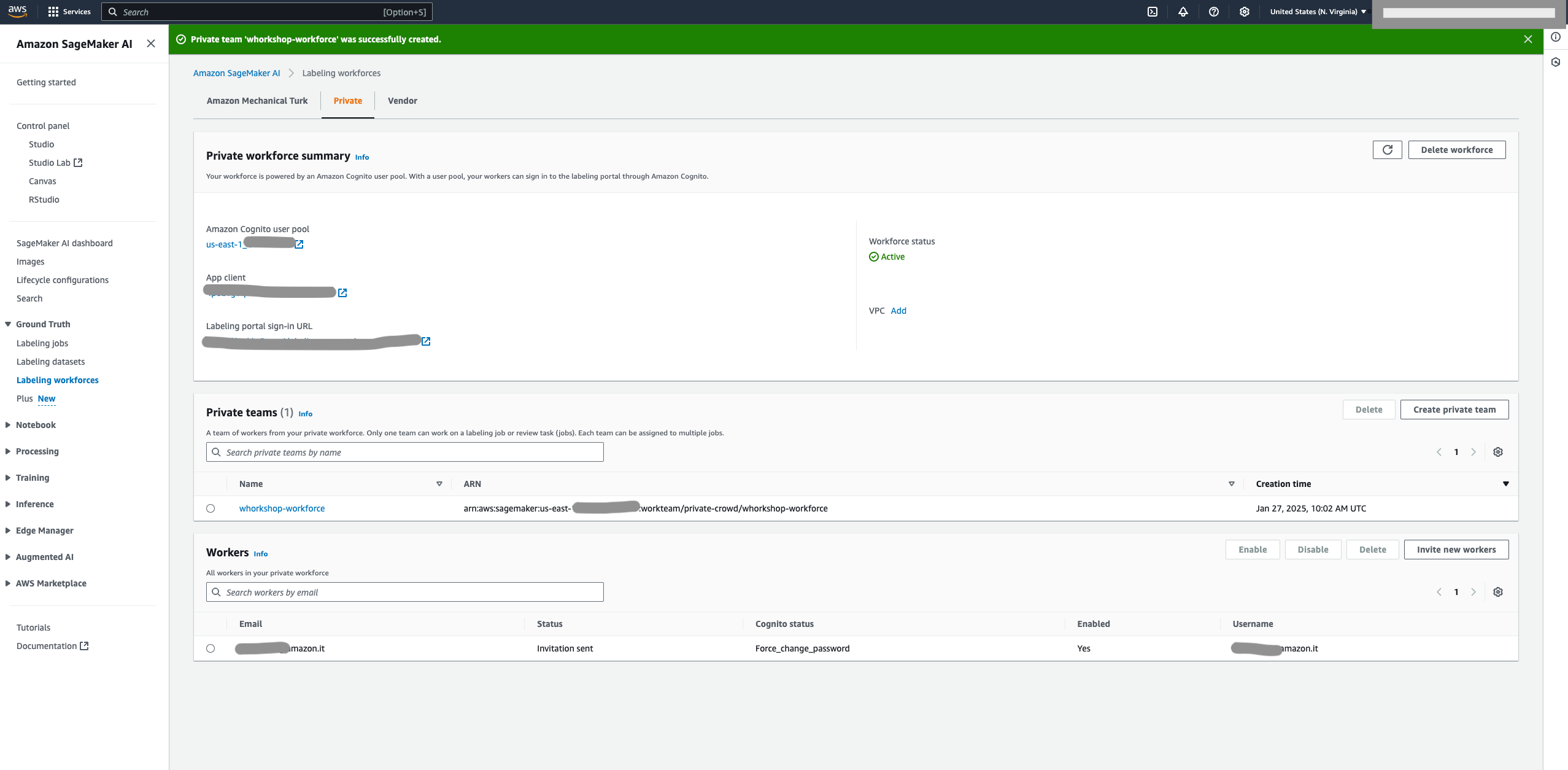
Lastly, build a Lambda layer that includes two Python libraries. To build this layer, connect to your development environment and issue the following commands:
git clone https://github.com/aws-samples/Build_a_computer_vision_based_asset_inventory_app_with_low_no_training
cd Build_a_computer_vision_based_asset_inventory_app_with_low_no_training
bash build_lambda_layer.sh
You should get an output similar to the following screenshot.
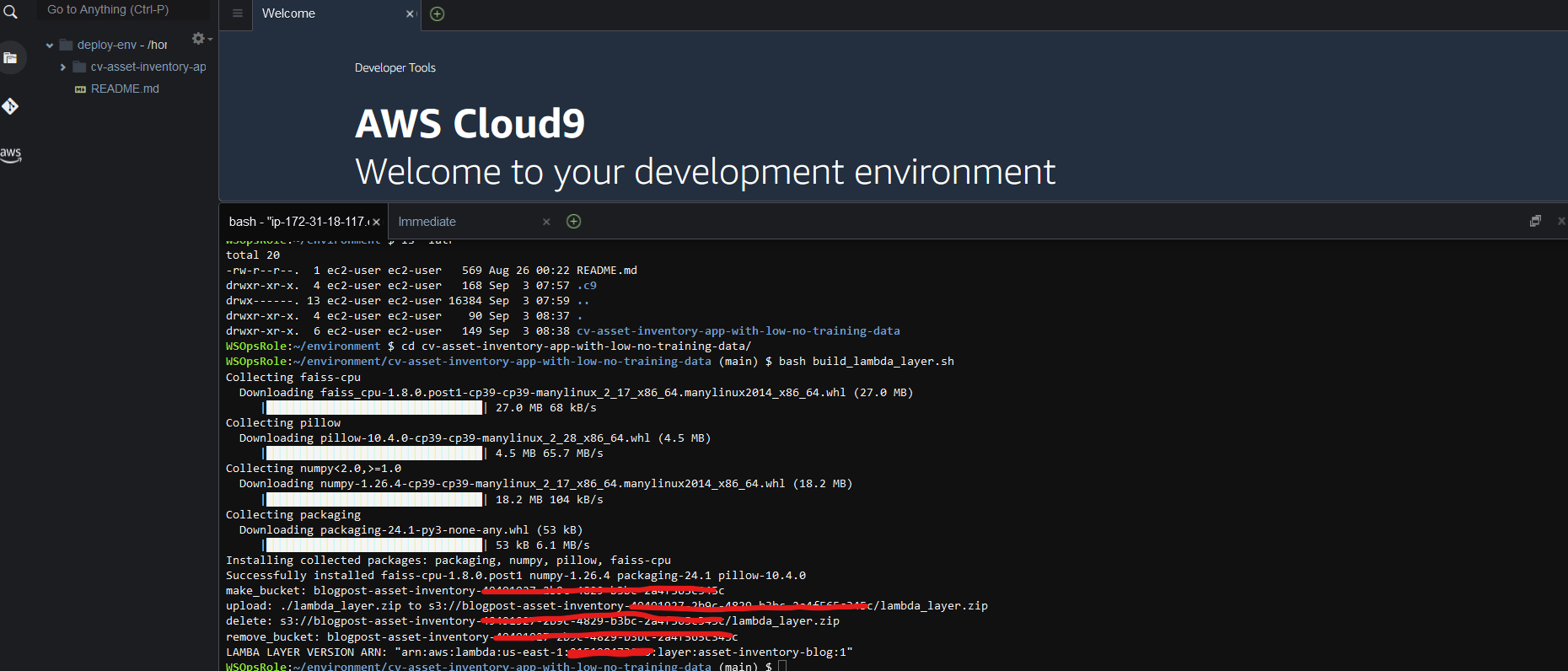
Save theLAMBDA_LAYER_VERSION_ARN
for later use.
You are now ready to deploy the backend infrastructure and frontend application.
Deploy the backend infrastructure
The backend is deployed using AWS CloudFormation to build the following components:
-
-
- An API Gateway to act as an integration layer between the frontend application and the backend
- An S3 bucket to store the uploaded images and the knowledge base
- Amazon Cognito to allow end-user authentication
- A set of Lambda functions to implement backend services
- An Amazon A2I workflow to support the back-office activities
- An SQS queue to store knowledge base update requests
- An EventBridge rule to trigger a Lambda function as soon as an Amazon A2I workflow is complete
- A DynamoDB table to store inventory data
- IAM roles and policies to allow access to the different components to interact with each other and also access Amazon Bedrock for generative AI-related tasks
Download the CloudFormation template, then complete the following steps:
-
-
- On the AWS CloudFormation console, chose Create stack.
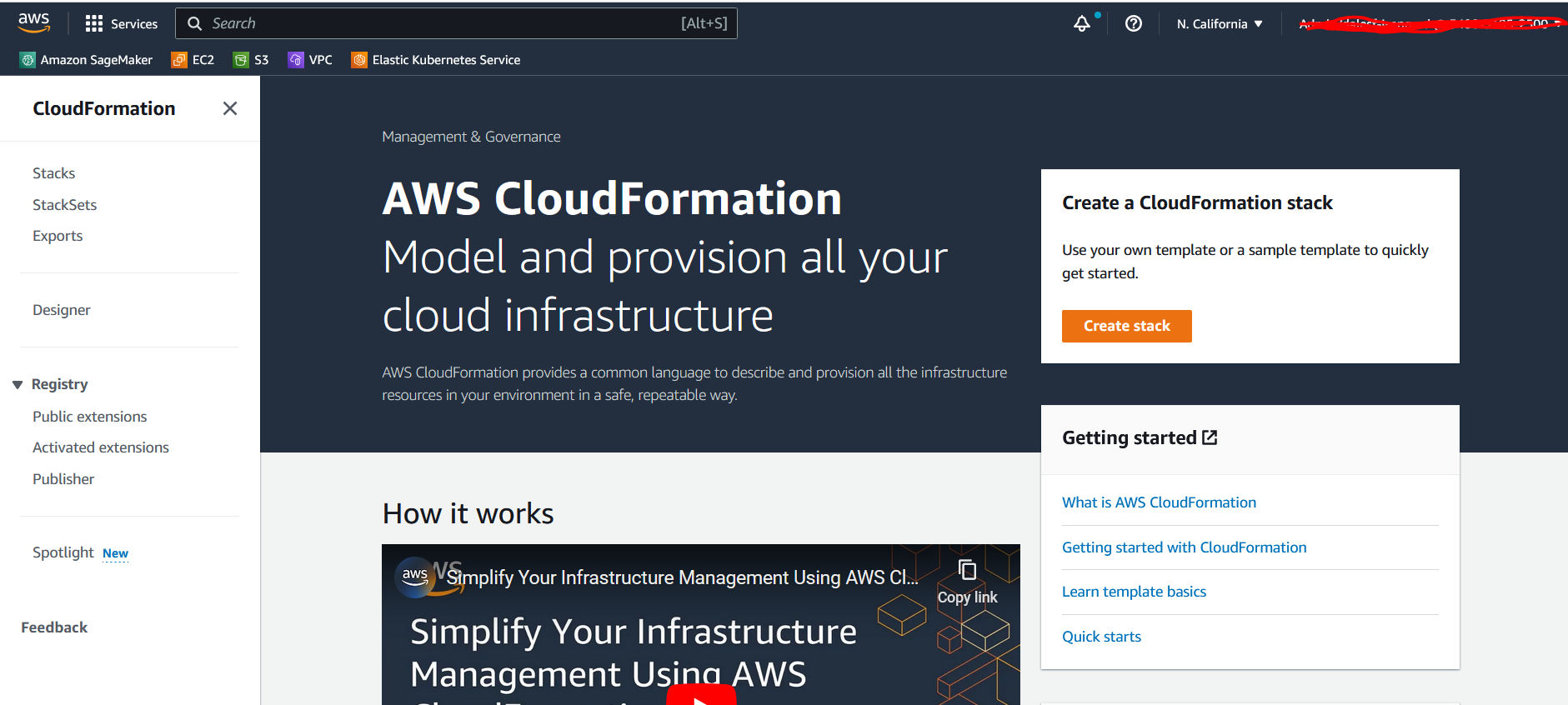
- Choose Upload a template file and choose Choose file to upload the downloaded template.
- Choose Next.
- For Stack name, enter a name (for example,
asset-inventory
).
- For A2IWorkforceARN, enter the ARN of the labeling workforce you identified.
- For LambdaLayerARN, enter the ARN of the Lambda layer version you uploaded.
- Choose Next and Next again.
- Acknowledge that AWS CloudFormation is going to create IAM resources and choose Submit.
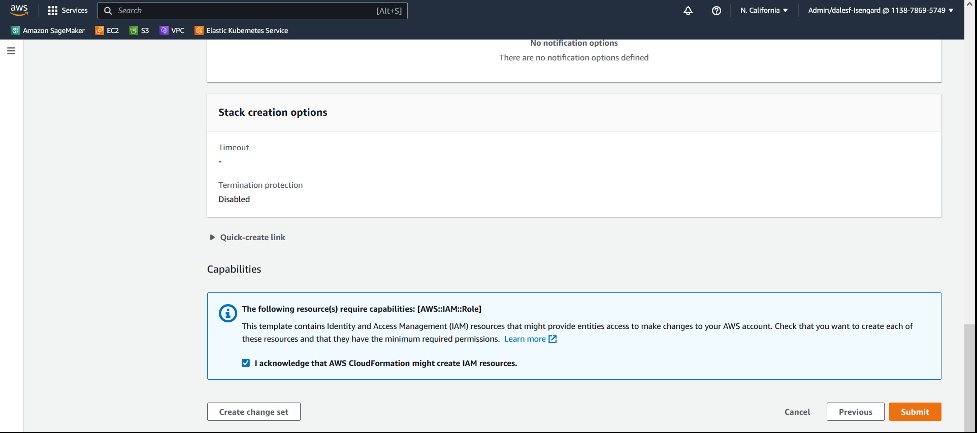
Wait until the CloudFormation stack creation process is complete; it will take about 15–20 minutes. You can then view the stack details.
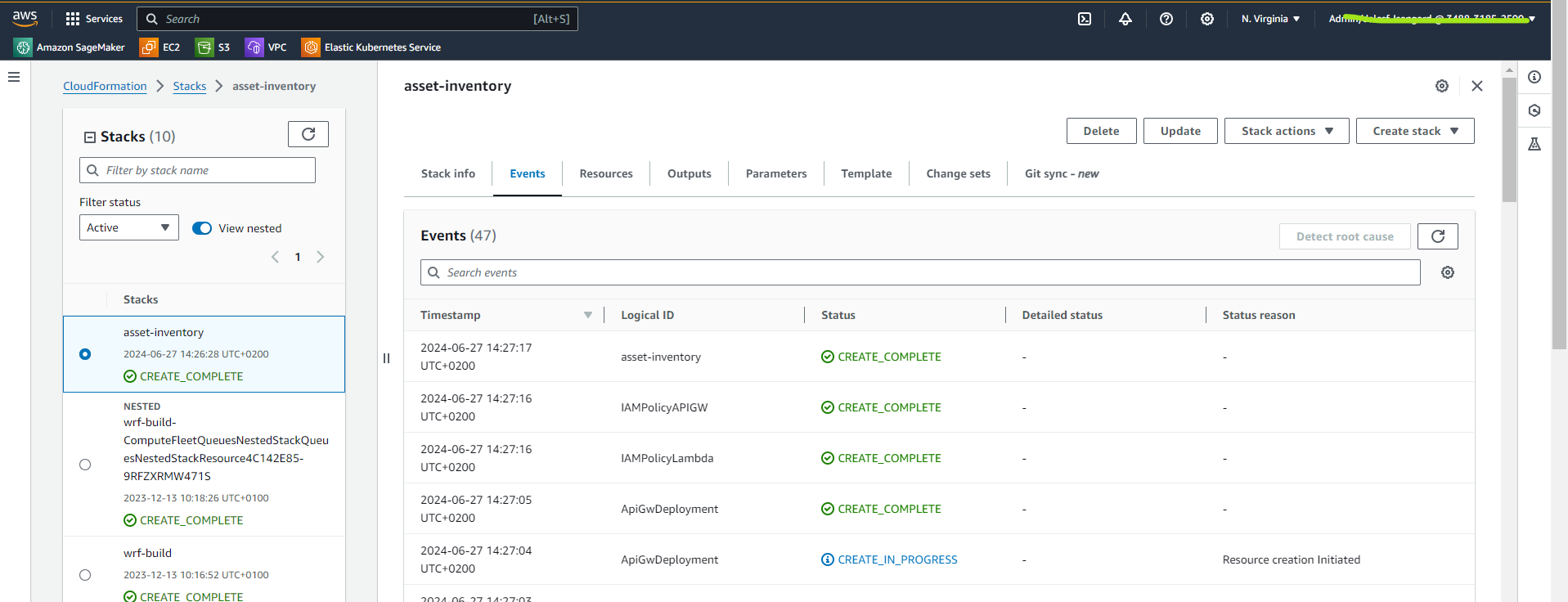
Note the values on the Outputs tab. You will use the output data later to complete the configuration of the frontend application.
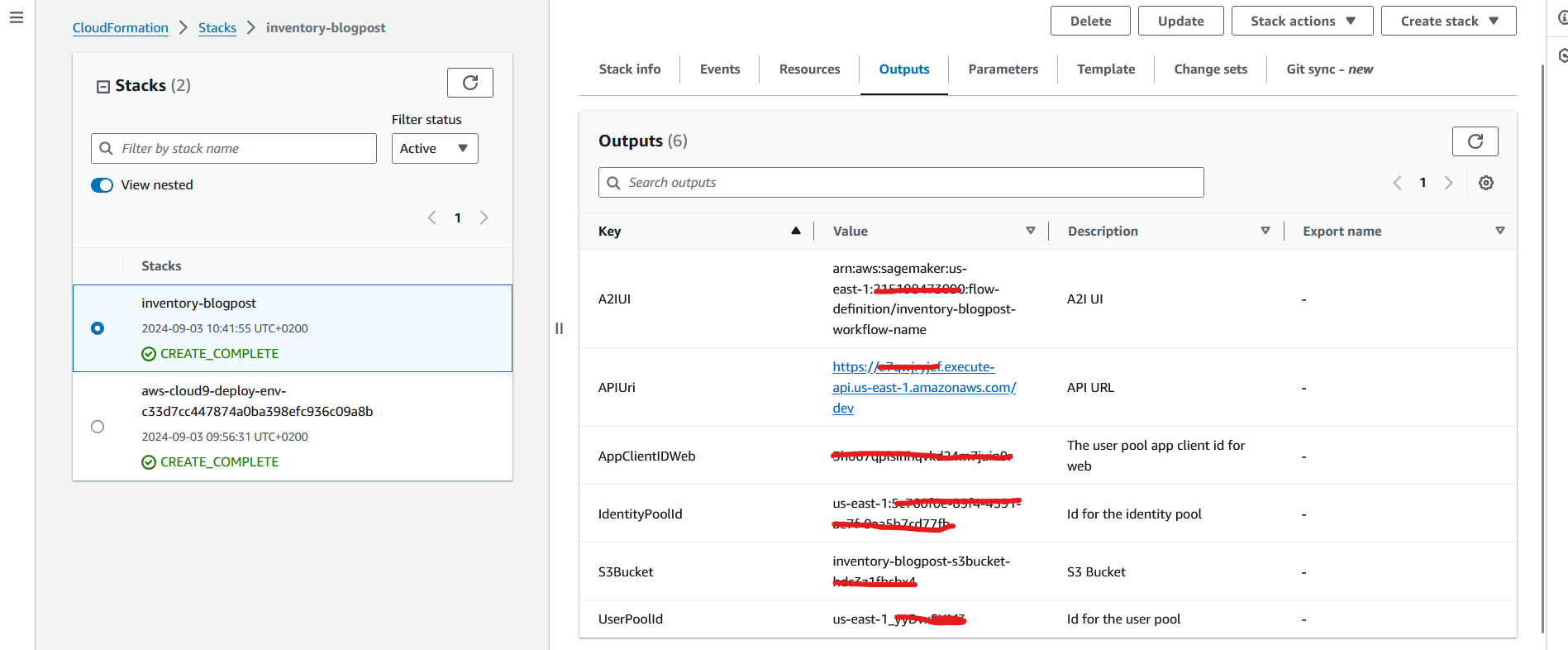
Deploy the frontend application
In this section, you will build the web application that is used by the on-site operator to collect a picture of the labels, submit it to the backend services to extract relevant information, validate or correct returned information, and submit the validated or corrected information to be stored in the asset inventory.
The web application uses React and will use the Amplify JavaScript Library.
Amplify provides several products to build full stack applications:
-
-
- Amplify CLI – A simple command line interface to set up the needed services
- Amplify Libraries – Use case-centric client libraries to integrate the frontend code with the backend
- Amplify UI Components – UI libraries for React, React Native, Angular, Vue, and Flutter
In this example, you have already created the needed services with the CloudFormation template, so the Amplify CLI will deploy the application on the Amplify provided hosting service.
-
-
- Log in to your development environment and download the client code from the GitHub repository using the following command:
git clone https://github.com/aws-samples/Build_a_computer_vision_based_asset_inventory_app_with_low_no_training
cd Build_a_computer_vision_based_asset_inventory_app_with_low_no_training
cd webapp
-
-
- If you’re running on AWS Cloud9 as a development environment, issue the following command to let the Amplify CLI use AWS Cloud9 managed credentials:
ln -s $HOME/.aws/credentials $HOME/.aws/config
-
-
- Now you can initialize the Amplify application using the CLI:
After issuing this command, the Amplify CLI will ask you for some parameters.
-
-
- Accept the default values by pressing Enter for each question.
- The next step is to modify
amplifyconfiguration.js.template
(you can find it in folder webapp/src
) with the information collected from the output of the CloudFormation stack and save as amplifyconfiguration.js
. This file tells Amplify which is the correct endpoint to use to interact with the backend resources created for this application. The information required is as follows:
- aws_project_region and aws_cognito_region – To be filled in with the Region in which you ran the CloudFormation template (for example, us-east-1).
- aws_cognito_identity_pool_id, aws_user_pools_id, aws_user_pools_web_client_id – The values from the Outputs tab of the CloudFormation stack.
- Endpoint – In the API section, update the endpoint with the API Gateway URL listed on the Outputs tab of the CloudFormation stack.
- You now need to add a hosting option for the single-page application. You can use Amplify to configure and host the web application by issuing the following command:
The Amplify CLI will ask you which type of hosting service you prefer and what type of deployment.
-
-
- Answer both questions by accepting the default option by pressing Enter key.
- You now need to install the JavaScript libraries used by this application using npm:
-
-
- Deploy the application using the following command:
-
-
- Confirm you want to proceed by entering
Y
.
At the end of the deployment phase, Amplify will return the public URL of the web application, similar to the following:
...
Find out more about deployment here:
https://cra.link/deployment
Zipping artifacts completed.
Deployment complete!
https://dev.xxx.amplifyapp.com
Now you can use your browser to connect to the application using the provided URL.
Clean up
To delete the resources used to build this solution, complete the following steps:
-
-
- Delete the Amplify application:
- Issue the following command:
-
-
-
- Confirm that you are willing to delete the application.
- Remove the backend resources:
- On the AWS CloudFormation console, choose Stacks in the navigation pane.
- Select the stack and choose Delete.
- Choose Delete to confirm.
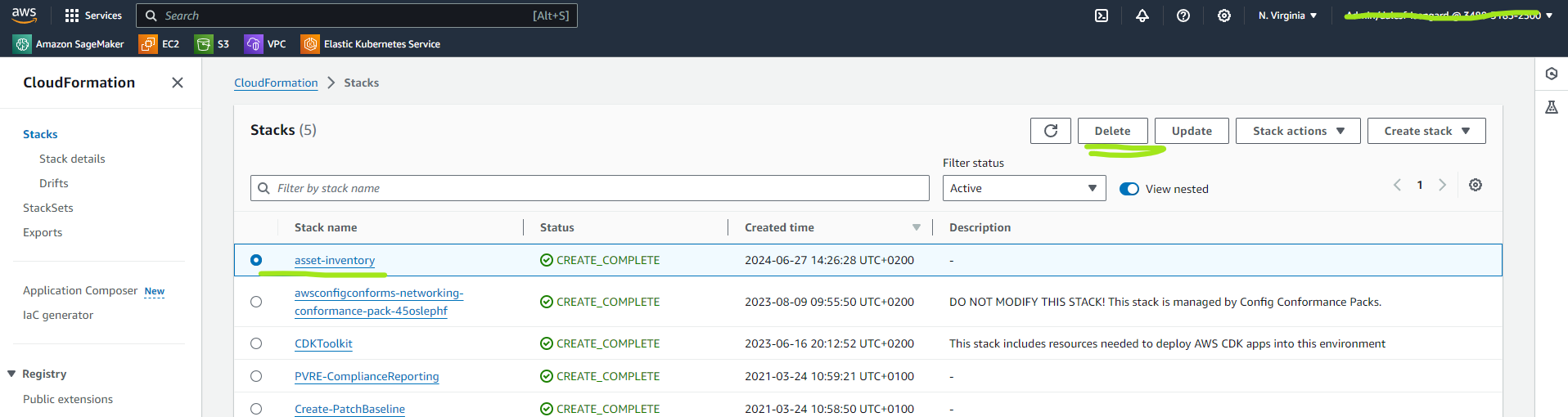
At the end of the deletion process, you should not see the entry related to asset-inventory
on the list of stacks.
-
-
- Remove the Lambda layer by issuing the following command in the development environment:
aws lambda delete-layer-version —layer-name asset-inventory-blog —version-number 1
-
-
- If you created a new labeling workforce, remove it by using the following command:
aws delete-workteam —workteam-name <the name you defined when you created the workteam>
Conclusion
In this post, we presented a solution that incorporates various AWS services to handle image storage (Amazon S3), mobile app development (Amplify), AI model hosting (Amazon Bedrock using Anthropic’s Claude), data verification (Amazon A2I), database (DynamoDB), and vector embeddings (Amazon Bedrock using Amazon Titan Multimodal Embeddings). It creates a seamless workflow for field data collection, AI-powered extraction, human validation, and inventory updates.
By taking advantage of the breadth of AWS services and integrating generative AI capabilities, this solution dramatically improves the efficiency and accuracy of asset inventory management processes. It reduces manual labor, accelerates data entry, and maintains high-quality inventory records, enabling organizations to optimize asset tracking and maintenance operations.
You can deploy this solution and immediately start collecting images of your assets to build or update your asset inventory.
About the authors
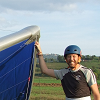
Federico D’Alessio is an AWS Solutions Architect and joined AWS in 2018. He is currently working in the Power and Utility and Transportation market. Federico is cloud addict and when not at work, he tries to reach clouds with his hang glider.
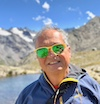
Leonardo Fenu is a Solutions Architect, who has been helping AWS customers align their technology with their business goals since 2018. When he is not hiking in the mountains or spending time with his family, he enjoys tinkering with hardware and software, exploring the latest cloud technologies, and finding creative ways to solve complex problems.
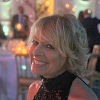
Elisabetta Castellano is an AWS Solutions Architect focused on empowering customers to maximize their cloud computing potential, with expertise in machine learning and generative AI. She enjoys immersing herself in cinema, live music performances, and books.
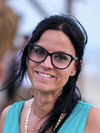
Carmela Gambardella is an AWS Solutions Architect since April 2018. Before AWS, Carmela has held various roles in large IT companies, such as software engineer, security consultant and solutions architect. She has been using her experience in security, compliance and cloud operations to help public sector organizations in their transformation journey to the cloud. In her spare time, she is a passionate reader, she enjoys hiking, traveling and playing yoga.
Read More