Apple Machine Learning Research
DART: Denoising Autoregressive Transformer for Scalable Text-to-Image Generation
Diffusion models have become the dominant approach for visual generation. They are trained by denoising a Markovian process which gradually adds noise to the input. We argue that the Markovian property limits the model’s ability to fully utilize the generation trajectory, leading to inefficiencies during training and inference. In this paper, we propose DART, a transformer-based model that unifies autoregressive (AR) and diffusion within a non-Markovian framework. DART iteratively denoises image patches spatially and spectrally using an AR model that has the same architecture as standard…Apple Machine Learning Research
Step-by-Step Diffusion: An Elementary Tutorial
We present an accessible first course on the mathematics of diffusion models and flow matching for machine learning. We aim to teach diffusion as simply as possible, with minimal mathematical and machine learning prerequisites, but enough technical detail to reason about its correctness. Unlike most tutorials on this subject, we take neither a Variational Auto Encoder (VAE) nor a Stochastic Differential Equations (SDE) approach. In fact, for the core ideas we will not need any SDEs, Evidence-Based-Lower-Bounds (ELBOs), Langevin dynamics, or even the notion of a score. The reader need only be…Apple Machine Learning Research
Scaling Laws for Native Multimodal Models
Building general-purpose models that can effectively perceive the world through multimodal signals has been a long-standing goal. Current approaches involve integrating separately pre-trained components, such as connecting vision encoders to LLMs and continuing multimodal training. While such approaches exhibit remarkable sample efficiency, it remains an open question whether such late-fusion architectures are inherently superior. In this work, we revisit the architectural design of native multimodal models (NMMs) – those trained from the ground up on all modalities – and conduct an extensive…Apple Machine Learning Research
Thousands of NVIDIA Grace Blackwell GPUs Now Live at CoreWeave, Propelling Development for AI Pioneers
CoreWeave today became one of the first cloud providers to bring NVIDIA GB200 NVL72 systems online for customers at scale, and AI frontier companies Cohere, IBM and Mistral AI are already using them to train and deploy next-generation AI models and applications.
CoreWeave, the first cloud provider to make NVIDIA Grace Blackwell generally available, has already shown incredible results in MLPerf benchmarks with NVIDIA GB200 NVL72 — a powerful rack-scale accelerated computing platform designed for reasoning and AI agents. Now, CoreWeave customers are gaining access to thousands of NVIDIA Blackwell GPUs.
“We work closely with NVIDIA to quickly deliver to customers the latest and most powerful solutions for training AI models and serving inference,” said Mike Intrator, CEO of CoreWeave. “With new Grace Blackwell rack-scale systems in hand, many of our customers will be the first to see the benefits and performance of AI innovators operating at scale.”
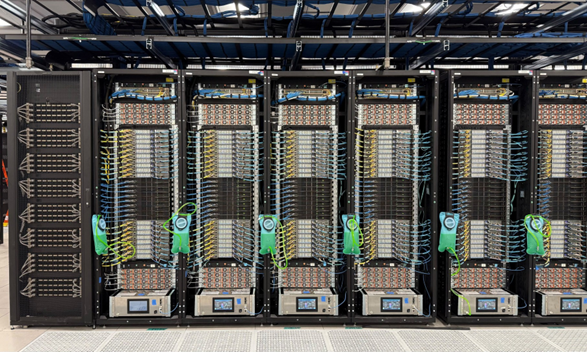
The ramp-up for customers of cloud providers like CoreWeave is underway. Systems built on NVIDIA Grace Blackwell are in full production, transforming cloud data centers into AI factories that manufacture intelligence at scale and convert raw data into real-time insights with speed, accuracy and efficiency.
Leading AI companies around the world are now putting GB200 NVL72’s capabilities to work for AI applications, agentic AI and cutting-edge model development.
Personalized AI Agents
Cohere is using its Grace Blackwell Superchips to help develop secure enterprise AI applications powered by leading-edge research and model development techniques. Its enterprise AI platform, North, enables teams to build personalized AI agents to securely automate enterprise workflows, surface real-time insights and more.
With NVIDIA GB200 NVL72 on CoreWeave, Cohere is already experiencing up to 3x more performance in training for 100 billion-parameter models compared with previous-generation NVIDIA Hopper GPUs — even without Blackwell-specific optimizations.
With further optimizations taking advantage of GB200 NVL72’s large unified memory, FP4 precision and a 72-GPU NVIDIA NVLink domain — where every GPU is connected to operate in concert — Cohere is getting dramatically higher throughput with shorter time to first and subsequent tokens for more performant, cost-effective inference.
“With access to some of the first NVIDIA GB200 NVL72 systems in the cloud, we are pleased with how easily our workloads port to the NVIDIA Grace Blackwell architecture,” said Autumn Moulder, vice president of engineering at Cohere. “This unlocks incredible performance efficiency across our stack — from our vertically integrated North application running on a single Blackwell GPU to scaling training jobs across thousands of them. We’re looking forward to achieving even greater performance with additional optimizations soon.”
AI Models for Enterprise
IBM is using one of the first deployments of NVIDIA GB200 NVL72 systems, scaling to thousands of Blackwell GPUs on CoreWeave, to train its next-generation Granite models, a series of open-source, enterprise-ready AI models. Granite models deliver state-of-the-art performance while maximizing safety, speed and cost efficiency. The Granite model family is supported by a robust partner ecosystem that includes leading software companies embedding large language models into their technologies.
Granite models provide the foundation for solutions like IBM watsonx Orchestrate, which enables enterprises to build and deploy powerful AI agents that automate and accelerate workflows across the enterprise.
CoreWeave’s NVIDIA GB200 NVL72 deployment for IBM also harnesses the IBM Storage Scale System, which delivers exceptional high-performance storage for AI. CoreWeave customers can access the IBM Storage platform within CoreWeave’s dedicated environments and AI cloud platform.
“We are excited to see the acceleration that NVIDIA GB200 NVL72 can bring to training our Granite family of models,” said Sriram Raghavan, vice president of AI at IBM Research. “This collaboration with CoreWeave will augment IBM’s capabilities to help build advanced, high-performance and cost-efficient models for powering enterprise and agentic AI applications with IBM watsonx.”
Compute Resources at Scale
Mistral AI is now getting its first thousand Blackwell GPUs to build the next generation of open-source AI models.
Mistral AI, a Paris-based leader in open-source AI, is using CoreWeave’s infrastructure, now equipped with GB200 NVL72, to speed up the development of its language models. With models like Mistral Large delivering strong reasoning capabilities, Mistral needs fast computing resources at scale.
To train and deploy these models effectively, Mistral AI requires a cloud provider that offers large, high-performance GPU clusters with NVIDIA Quantum InfiniBand networking and reliable infrastructure management. CoreWeave’s experience standing up NVIDIA GPUs at scale with industry-leading reliability and resiliency through tools such as CoreWeave Mission Control met these requirements.
“Right out of the box and without any further optimizations, we saw a 2x improvement in performance for dense model training,” said Thimothee Lacroix, cofounder and chief technology officer at Mistral AI. “What’s exciting about NVIDIA GB200 NVL72 is the new possibilities it opens up for model development and inference.”
A Growing Number of Blackwell Instances
In addition to long-term customer solutions, CoreWeave offers instances with rack-scale NVIDIA NVLink across 72 NVIDIA Blackwell GPUs and 36 NVIDIA Grace CPUs, scaling to up to 110,000 GPUs with NVIDIA Quantum-2 InfiniBand networking.
These instances, accelerated by the NVIDIA GB200 NVL72 rack-scale accelerated computing platform, provide the scale and performance needed to build and deploy the next generation of AI reasoning models and agents.
Everywhere, All at Once: NVIDIA Drives the Next Phase of AI Growth
Every company and country wants to grow and create economic opportunity — but they need virtually limitless intelligence to do so. Working with its ecosystem partners, NVIDIA this week is underscoring its work advancing reasoning, AI models and compute infrastructure to manufacture intelligence in AI factories — driving the next phase of growth in the U.S. and around the world.
Yesterday, NVIDIA announced it will manufacture AI supercomputers in the U.S. for the first time. Within the next four years, the company plans with its partners to produce up to half a trillion dollars of AI infrastructure in the U.S.
Building NVIDIA AI supercomputers in the U.S. for American AI factories is expected to create opportunities for hundreds of thousands of people and drive trillions of dollars in growth over the coming decades. Some of the NVIDIA Blackwell compute engines at the heart of those AI supercomputers are already being produced at TSMC fabs in Arizona.
NVIDIA announced today that NVIDIA Blackwell GB200 NVL72 rack-scale systems are now available from CoreWeave for customers to train next-generation AI models and run applications at scale. CoreWeave has thousands of NVIDIA Grace Blackwell processors available now to train and deploy the next wave of AI.
Beyond hardware innovation, NVIDIA also pioneers AI software to create more efficient and intelligent models.
Marking the latest in those advances, the NVIDIA Llama Nemotron Ultra model was recognized today by Artificial Analysis as the world’s most accurate open-source reasoning model for scientific and complex coding tasks. It’s also now ranked among the top reasoning models in the world.
NVIDIA’s engineering feats serve as the foundation of it all. A team of NVIDIA engineers won first place in the AI Mathematical Olympiad, competing against 2,200 teams to solve complex mathematical reasoning problems, which are key to advancing scientific discovery, disciplines and domains. The same post-training techniques and open datasets from NVIDIA’s winning effort in the math reasoning competition were applied in training the Llama Nemotron Ultra model.
The world’s need for intelligence is virtually limitless, and NVIDIA’s AI platform is helping meet that need — everywhere, all at once.
Math Test? No Problems: NVIDIA Team Scores Kaggle Win With Reasoning Model
The final days of the AI Mathematical Olympiad’s latest competition were a transcontinental relay for team NVIDIA.
Every evening, two team members on opposite ends of the U.S. would submit an AI reasoning model to Kaggle — the online Olympics of data science and machine learning. They’d wait a tense five hours before learning how well the model tackled a sample set of 50 complex math problems.
After seeing the results, the U.S. team would pass the baton to teammates waking up in Armenia, Finland, Germany and Northern Ireland, who would spend their day testing, modifying and optimizing different model versions.
“Every night I’d be so disappointed in our score, but then I’d wake up and see the messages that came in overnight from teammates in Europe,” said Igor Gitman, senior applied scientist. “My hopes would go up and we’d try again.”
While the team was disheartened by their lack of improvement on the public dataset during the competition’s final days, the real test of an AI model is how well it can generalize to unseen data. That’s where their reasoning model leapt to the top of the leaderboard — correctly answering 34 out of 50 Olympiad questions within a five-hour time limit using a cluster of four NVIDIA L4 GPUs.
“We got the magic in the end,” said Northern Ireland-based team member Darragh Hanley, a Kaggle grandmaster and senior large language model (LLM) technologist.
Building a Winning Equation
The NVIDIA team competed under the name NemoSkills — a nod to their use of the NeMo-Skills collection of pipelines for accelerated LLM training, evaluation and inference. The seven members each contributed different areas of expertise, spanning LLM training, model distillation and inference optimization.
For the Kaggle challenge, over 2,200 participating teams submitted AI models tasked with solving 50 math questions — complex problems at the National Olympiad level, spanning algebra, geometry, combinatorics and number theory — within five hours.
The team’s winning model uses a combination of natural language reasoning and Python code execution.
To complete this inference challenge on the small cluster of NVIDIA L4 GPUs available via Kaggle, the NemoSkills team had to get creative.
Their winning model used Qwen2.5-14B-Base, a foundation model with chain-of-thought reasoning capabilities which the team fine-tuned on millions of synthetically generated solutions to math problems.
These synthetic solutions were primarily generated by two larger reasoning models — DeepSeek-R1 and QwQ-32B — and used to teach the team’s foundation model via a form of knowledge distillation. The end result was a smaller, faster, long-thinking model capable of tackling complex problems using a combination of natural language reasoning and Python code execution.
To further boost performance, the team’s solution reasons through multiple long-thinking responses in parallel before determining a final answer. To optimize this process and meet the competition’s time limit, the team also used an innovative early-stopping technique.
A reasoning model might, for example, be set to answer a math problem 12 different times before picking the most common response. Using the asynchronous processing capabilities of NeMo-Skills and NVIDIA TensorRT-LLM, the team was able to monitor and exit inference early if the model had already converged at the correct answer four or more times.
TensorRT-LLM also enabled the team to harness FP8 quantization, a compression method that resulted in a 1.5x speedup over using the more commonly used FP16 format. ReDrafter, a speculative decoding technique developed by Apple, was used for a further 1.8x speedup.
The final model performed even better on the competition’s unseen final dataset than it did on the public dataset — a sign that the team successfully built a generalizable model and avoided overfitting their LLM to the sample data.
“Even without the Kaggle competition, we’d still be working to improve AI reasoning models for math,” said Gitman. “But Kaggle gives us the opportunity to benchmark and discover how well our models generalize to a third-party dataset.”
Sharing the Wealth
The team will soon release a technical report detailing the techniques used in their winning solution — and plans to share their dataset and a series of models on Hugging Face. The advancements and optimizations they made over the course of the competition have been integrated into NeMo-Skills pipelines available on GitHub.
Key data, technology, and insights from this pipeline were also used to train the just-released NVIDIA Llama Nemotron Ultra model.
“Throughout this collaboration, we used tools across the NVIDIA software stack,” said Christof Henkel, a member of the Kaggle Grandmasters of NVIDIA, known as KGMON. “By working closely with our LLM research and development teams, we’re able to take what we learn from the competition on a day-to-day basis and push those optimizations into NVIDIA’s open-source libraries.”
After the competition win, Henkel regained the title of Kaggle World Champion — ranking No. 1 among the platform’s over 23 million users. Another teammate, Finland-based Ivan Sorokin, earned the Kaggle Grandmaster title, held by just over 350 people around the world.
For their first-place win, the group also won a $262,144 prize that they’re directing to the NVIDIA Foundation to support charitable organizations.
Meet the full team — Igor Gitman, Darragh Hanley, Christof Henkel, Ivan Moshkov, Benedikt Schifferer, Ivan Sorokin and Shubham Toshniwal — in the video below:
Sample math questions in the featured visual above are from the 2025 American Invitational Mathematics Examination. Find the full set of questions and solutions on the Art of Problem Solving wiki.
Clario enhances the quality of the clinical trial documentation process with Amazon Bedrock
This post is co-written with Kim Nguyen and Shyam Banuprakash from Clario.
Clario is a leading provider of endpoint data solutions to the clinical trials industry, generating high-quality clinical evidence for life sciences companies seeking to bring new therapies to patients. Since Clario’s founding more than 50 years ago, the company’s endpoint data solutions have supported clinical trials more than 26,000 times with over 700 regulatory approvals across more than 100 countries. One of the critical challenges Clario faces when supporting its clients is the time-consuming process of generating documentation for clinical trials, which can take weeks.
The business challenge
When medical imaging analysis is part of a clinical trial it is supporting, Clario prepares a medical imaging charter process document that outlines the format and requirements of the central review of clinical trial images (the Charter). Based on the Charter, Clario’s imaging team creates several subsequent documents (as shown in the following figure), including the business requirement specification (BRS), training slides, and ancillary documents. The content of these documents is largely derived from the Charter, with significant reformatting and rephrasing required. This process is time-consuming, can be subject to inadvertent manual error, and carries the risk of inconsistent or redundant information, which can delay or otherwise negatively impact the clinical trial.
Clario’s imaging team recognized the need to modernize the document generation process and streamline the processes used to create end-to-end document workflows. Clario engaged with their AWS account team and AWS Generative AI Innovation Center to explore how generative AI could help streamline the process.
The solution
The AWS team worked closely with Clario to develop a prototype solution that uses AWS AI services to automate the BRS generation process. The solution involves the following key services:
- Amazon Simple Storage Service (Amazon S3): A scalable object storage service used to store the charter-derived and generated BRS documents.
- Amazon OpenSearch Serverless: An on-demand serverless configuration for Amazon OpenSearch Service used as a vector store.
- Amazon Bedrock: Amazon Bedrock is a fully managed service that offers a choice of high-performing foundation models (FMs) from leading AI companies through a single API, along with a broad set of capabilities you need to build generative AI applications with security, privacy, and responsible AI. Using Amazon Bedrock, you can experiment with and evaluate top FMs for your use case, privately customize them with your data using techniques such as fine-tuning and Retrieval Augmented Generation (RAG) and build agents that execute tasks using your enterprise systems and data sources.
The solution is shown in the following figure:
Architecture walkthrough
- Charter-derived documents are processed in an on-premises script in preparation for uploading.
- Files are sent to AWS using AWS Direct Connect.
- The script chunks the documents and calls an embedding model to produce the document embeddings. It then stores the embeddings in an OpenSearch vector database for retrieval by our application. Clario uses an Amazon Titan Text Embeddings model offered by Amazon Bedrock. Each chunk is called to produce an embedding.
- Amazon OpenSearch Serverlessis used as the durable vector store. Document chunk embeddings are stored in an OpenSearch vector index, which enables the application to search for the most semantically relevant documents. Clario also stores attributes for the source document and associated trial to allow for a richer search experience.
- A custom build user interface is the primary access point for users to access the system, initiate generation jobs, and interact with a chat UI. The UI is integrated with the workflow engine that manages the orchestration process.
- The workflow engine calls the Amazon Bedrock API and orchestrates the business requirement specification document generation process. The engine:
- Uses a global specification that stores the prompts to be used as input when calling the large language model.
- Queries OpenSearch for the relevant Imaging charter.
- Loops through every business requirement.
- Calls the Claude 3.7 Sonnet large language model from Amazon Bedrock to generate responses.
- Outputs the business requirement specification document to the user interface, where a business requirement writer can review the answers to produce a final document. Clario uses Claude 3.7 Sonnet from Amazon Bedrock for the question-answering and the conversational AI application.
- The final documents are written to Amazon S3 to be consumed and published by additional document workflows that will be built in the future.
- An as-needed AI chat agent to allow document-based discovery and enable users to converse with one or more documents.
Benefits and results
By using AWS AI services, Clario has streamlined the complicated BRS generation process significantly. The prototype solution demonstrated the following benefits:
- Improved accuracy: The use of generative AI models minimized the risk of translation errors and inconsistencies, reducing the need for rework and study delays.
- Scalability and flexibility: The serverless architecture provided by AWS services allows the solution to scale seamlessly as demand increases, while the modular design enables straightforward integration with other Clario systems.
- Security: Clario’s data security strategy revolves around confining all its information within the secure AWS ecosystem using the security features of Amazon Bedrock. By keeping data isolated within the AWS infrastructure, Clario helps ensure protection against external threats and unauthorized access. This approach enables Clario to meet compliance requirements and provide clients with confidence in the confidentiality and integrity of their sensitive data.
Lessons learned
The successful implementation of this prototype solution reinforced the value of using generative AI models for domain-specific applications like those prevalent in the life sciences industry. It also highlighted the importance of involving business stakeholders early in the process and having a clear understanding of the business value to be realized. Following the success of this project, Clario is working to productionize the solution in their Medical Imaging business during 2025 to continue offering state-of-the-art services to its customers for best quality data and successful clinical trials.
Conclusion
The collaboration between Clario and AWS demonstrated the potential of AWS AI and machine learning (AI/ML) services and generative AI models, such as Anthropic’s Claude, to streamline document generation processes in the life sciences industry and, specifically, for complicated clinical trial processes. By using these technologies, Clario was able to enhance and streamline the BRS generation process significantly, improving accuracy and scalability. As Clario continues to adopt AI/ML across its operations, the company is well-positioned to drive innovation and deliver better outcomes for its partners and patients.
About the Authors
Kim Nguyen serves as the Sr Director of Data Science at Clario, where he leads a team of data scientists in developing innovative AI/ML solutions for the healthcare and clinical trials industry. With over a decade of experience in clinical data management and analytics, Kim has established himself as an expert in transforming complex life sciences data into actionable insights that drive business outcomes. His career journey includes leadership roles at Clario and Gilead Sciences, where he consistently pioneered data automation and standardization initiatives across multiple functional teams. Kim holds a Master’s degree in Data Science and Engineering from UC San Diego and a Bachelor’s degree from the University of California, Berkeley, providing him with the technical foundation to excel in developing predictive models and data-driven strategies. Based in San Diego, California, he leverages his expertise to drive forward-thinking approaches to data science in the clinical research space.
Shyam Banuprakash serves as the Senior Vice President of Data Science and Delivery at Clario, where he leads complex analytics programs and develops innovative data solutions for the medical imaging sector. With nearly 12 years of progressive experience at Clario, he has demonstrated exceptional leadership in data-driven decision making and business process improvement. His expertise extends beyond his primary role, as he contributes his knowledge as an Advisory Board Member for both Modal and UC Irvine’s Customer Experience Program. Shyam holds a Master of Advanced Study in Data Science and Engineering from UC San Diego, complemented by specialized training from MIT in data science and big data analytics. His career exemplifies the powerful intersection of healthcare, technology, and data science, positioning him as a thought leader in leveraging analytics to transform clinical research and medical imaging.
John O’Donnell is a Principal Solutions Architect at Amazon Web Services (AWS) where he provides CIO-level engagement and design for complex cloud-based solutions in the healthcare and life sciences (HCLS) industry. With over 20 years of hands-on experience, he has a proven track record of delivering value and innovation to HCLS customers across the globe. As a trusted technical leader, he has partnered with AWS teams to dive deep into customer challenges, propose outcomes, and ensure high-value, predictable, and successful cloud transformations. John is passionate about helping HCLS customers achieve their goals and accelerate their cloud native modernization efforts.
Praveen Haranahalli is a Senior Solutions Architect at Amazon Web Services (AWS) where he provides expert guidance and architects secure, scalable cloud solutions for diverse enterprise customers. With nearly two decades of IT experience, including over ten years specializing in Cloud Computing, he has a proven track record of delivering transformative cloud implementations across multiple industries. As a trusted technical advisor, Praveen has successfully partnered with customers to implement robust DevSecOps pipelines, establish comprehensive security guardrails, and develop innovative AI/ML solutions. Praveen is passionate about solving complex business challenges through cutting-edge cloud architectures and helping organizations achieve successful digital transformations powered by artificial intelligence and machine learning technologies.
Optimizing Mixtral 8x7B on Amazon SageMaker with AWS Inferentia2
Organizations are constantly seeking ways to harness the power of advanced large language models (LLMs) to enable a wide range of applications such as text generation, summarizationquestion answering, and many others. As these models grow more powerful and capable, deploying them in production environments while optimizing performance and cost-efficiency becomes more challenging.
Amazon Web Services (AWS) provides highly optimized and cost-effective solutions for deploying AI models, like the Mixtral 8x7B language model, for inference at scale. The AWS Inferentia and AWS Trainium are AWS AI chips, purpose-built to deliver high throughput and low latency inference and training performance for even the largest deep learning models. The Mixtral 8x7B model adopts the Mixture-of-Experts (MoE) architecture with eight experts. AWS Neuron—the SDK used to run deep learning workloads on AWS Inferentia and AWS Trainium based instances—employs expert parallelism for MoE architecture, sharding the eight experts across multiple NeuronCores.
This post demonstrates how to deploy and serve the Mixtral 8x7B language model on AWS Inferentia2 instances for cost-effective, high-performance inference. We’ll walk through model compilation using Hugging Face Optimum Neuron, which provides a set of tools enabling straightforward model loading, training, and inference, and the Text Generation Inference (TGI) Container, which has the toolkit for deploying and serving LLMs with Hugging Face. This will be followed by deployment to an Amazon SageMaker real-time inference endpoint, which automatically provisions and manages the Inferentia2 instances behind the scenes and provides a containerized environment to run the model securely and at scale.
While pre-compiled model versions exist, we’ll cover the compilation process to illustrate important configuration options and instance sizing considerations. This end-to-end guide combines Amazon Elastic Compute Cloud (Amazon EC2)-based compilation with SageMaker deployment to help you use Mixtral 8x7B’s capabilities with optimal performance and cost efficiency.
Step 1: Set up Hugging Face access
Before you can deploy the Mixtral 8x7B model, there some prerequisites that you need to have in place.
- The model is hosted on Hugging Face and uses their transformers library. To download and use the model, you need to authenticate with Hugging Face using a user access token. These tokens allow secure access for applications and notebooks to Hugging Face’s services. You first need to create a Hugging Face account if you don’t already have one, which you can then use to generate and manage your access tokens through the user settings.
- The mistralai/Mixtral-8x7B-Instruct-v0.1 model that you will be working with in this post is a gated model. This means that you need to specifically request access from Hugging Face before you can download and work with the model.
Step 2: Launch an Inferentia2-powered EC2 Inf2 instance
To get started with an Amazon EC2 Inf2 instance for deploying the Mixtral 8x7B, either deploy the AWS CloudFormation template or use the AWS Management Console.
To launch an Inferentia2 instance using the console:
- Navigate to the Amazon EC2 console and choose Launch Instance.
- Enter a descriptive name for your instance.
- Under the Application and OS Images search for and select the Hugging Face Neuron Deep Learning AMI, which comes pre-configured with the Neuron software stack for AWS Inferentia.
- For Instance type, select 24xlarge, which contains six Inferentia chips (12 NeuronCores).
- Create or select an existing key pair to enable SSH access.
- Create or select a security group that allows inbound SSH connections from the internet.
- Under Configure Storage, set the root EBS volume to 512 GiB to accommodate the large model size.
- After the settings are reviewed, choose Launch Instance.
With your Inf2 instance launched, connect to it over SSH by first locating the public IP or DNS name in the Amazon EC2 console. Later in this post, you will connect to a Jupyter notebook using a browser on port 8888. To do that, SSH tunnel to the instance using the key pair you configured during instance creation.
After signing in, list the NeuronCores attached to the instance and their associated topology:
For inf2.24xlarge, you should see the following output listing six Neuron devices:
For more information on the neuron-ls
command, see the Neuron LS User Guide.
Make sure the Inf2 instance is sized correctly to host the model. Each Inferentia NeuronCore processor contains 16 GB of high-bandwidth memory (HBM). To accommodate an LLM like the Mixtral 8x7B on AWS Inferentia2 (inf2) instances, a technique called tensor parallelism is used. This allows the model’s weights, activations, and computations to be split and distributed across multiple NeuronCores in parallel. To determine the degree of tensor parallelism required, you need to calculate the total memory footprint of the model. This can be computed as:
total memory = bytes per parameter * number of parameters
The Mixtral-8x7B model consists of 46.7 billion parameters. With float16
casted weights, you need 93.4 GB to store the model weights. The total space required is often greater than just the model parameters because of caching attention layer projections (KV caching). This caching mechanism grows memory allocations linearly with sequence length and batch size. With a batch size of 1 and a sequence length of 1024 tokens, the total memory footprint for the caching is 0.5 GB. The exact formula can be found in the AWS Neuron documentation and the hyper-parameter configuration required for these calculations is stored in the model config.json file.
Given that each NeuronCore has 16 GB of HBM, and the model requires approximately 94 GB of memory, a minimum tensor parallelism degree of 6 would theoretically suffice. However, with 32 attention heads, the tensor parallelism degree must be a divisor of this number.
Furthermore, considering the model’s size and the MoE implementation in transformers-neuronx
, the supported tensor parallelism degrees are limited to 8, 16, and 32. For the example in this post, you will distribute the model across eight NeuronCores.
Compile Mixtral-8x7B model to AWS Inferentia2
The Neuron SDK includes a specialized compiler that automatically optimizes the model format for efficient execution on AWS Inferentia2.
- To start this process, launch the container and pass the Inferentia devices to the container. For more information about launching the neuronx-tgi container see Deploy the Text Generation Inference (TGI) Container on a dedicated host.
- Inside the container, sign in to the Hugging Face Hub to access gated models, such as the Mixtral-8x7B-Instruct-v0.1. See the previous section for Setup Hugging Face Access. Make sure to use a token with read and write permissions so you can later save the compiled model to the Hugging Face Hub.
- After signing in, compile the model with optimum-cli. This process will download the model artifacts, compile the model, and save the results in the specified directory.
- The Neuron chips are designed to execute models with fixed input shapes for optimal performance. This requires that the compiled artifact shapes must be known at compilation time. In the following command, you will set the batch size, input/output sequence length, data type, and tensor-parallelism degree (number of neuron cores). For more information about these parameters, see Export a model to Inferentia.
Let’s discuss these parameters in more detail:
- The parameter
batch_size
is the number of input sequences that the model will accept. sequence_length
specifies the maximum number of tokens in an input sequence. This affects memory usage and model performance during inference or training on Neuron hardware. A larger number will increase the model’s memory requirements because the attention mechanism needs to operate over the entire sequence, which leads to more computations and memory usage; while a smaller number will do the opposite. The value 1024 will be adequate for this example.auto_cast_type
parameter controls quantization. It allows type casting for model weights and computations during inference. The options are:bf16
,fp16
, ortf32
. For more information about defining which lower-precision data type the compiler should use see Mixed Precision and Performance-accuracy Tuning. For models trained in float32, the 16-bit mixed precision options (bf16
,f16
) generally provide sufficient accuracy while significantly improving performance. We use data typefloat16
with the argumentauto_cast_type fp16
.- The
num_cores
parameter controls the number of cores on which the model should be deployed. This will dictate the number of parallel shards or partitions the model is split into. Each shard is then executed on a separate NeuronCore, taking advantage of the 16 GB high-bandwidth memory available per core. As discussed in the previous section, given the Mixtral-8x7B model’s requirements, Neuron supports 8, 16, or 32 tensor parallelism The inf2.24xlarge instance contains 12 Inferentia NeuronCores. Therefore, to optimally distribute the model, we setnum_cores
to 8.
- Download and compilation should take 10–20 minutes. After the compilation completes successfully, you can check the artifacts created in the output directory:
- Push the compiled model to the Hugging Face Hub with the following command. Make sure to change
<user_id>
to your Hugging Face username. If the model repository doesn’t exist, it will be created automatically. Alternatively, store the model on Amazon Simple Storage Service (Amazon S3).
huggingface-cli upload <user_id>/Mixtral-8x7B-Instruct-v0.1 ./neuron_model_path ./
Deploy Mixtral-8x7B SageMaker real-time inference endpoint
Now that the model has been compiled and stored, you can deploy it for inference using SageMaker. To orchestrate the deployment, you will run Python code from a notebook hosted on an EC2 instance. You can use the instance created in the first section or create a new instance. Note that this EC2 instance can be of any type (for example t2.micro
with an Amazon Linux 2023 image). Alternatively, you can use a notebook hosted in Amazon SageMaker Studio.
Set up AWS authorization for SageMaker deployment
You need AWS Identity and Access Management (IAM) permissions to manage SageMaker resources. If you created the instance with the provided CloudFormation template, these permissions are already created for you. If not, the following section takes you through the process of setting up the permissions for an EC2 instance to run a notebook that deploys a real-time SageMaker inference endpoint.
Create an AWS IAM role and attach SageMaker permission policy
- Go to the IAM console.
- Choose the Roles tab in the navigation pane.
- Choose Create role.
- Under Select trusted entity, select AWS service.
- Choose Use case and select EC2.
- Select EC2 (Allows EC2 instances to call AWS services on your behalf.)
- Choose Next: Permissions.
- In the Add permissions policies screen, select AmazonSageMakerFullAccess and IAMReadOnlyAccess. Note that the AmazonSageMakerFullAccess permission is overly permissive. We use it in this example to simplify the process but recommend applying the principle of least privilege when setting up IAM permissions.
- Choose Next: Review.
- In the Role name field, enter a role name.
- Choose Create role to complete the creation.
- With the role created, choose the Roles tab in the navigation pane and select the role you just created.
- Choose the Trust relationships tab and then choose Edit trust policy.
- Choose Add next to Add a principal.
- For Principal type, select AWS services.
- Enter
sagemaker.amazonaws.com
and choose Add a principal. - Choose Update policy. Your trust relationship should look like the following:
Attach the IAM role to your EC2 instance
- Go to the Amazon EC2 console.
- Choose Instances in the navigation pane.
- Select your EC2 instance.
- Choose Actions, Security, and then Modify IAM role.
- Select the role you created in the previous step.
- Choose Update IAM role.
Launch a Jupyter notebook
Your next goal is to run a Jupyter notebook hosted in a container running on the EC2 instance. The notebook will be run using a browser on port 8888 by default. For this example, you will use SSH port forwarding from your local machine to the instance to access the notebook.
- Continuing from the previous section, you are still within the container. The following steps install Jupyter Notebook:
- Launch the notebook server using:
- Then connect to the notebook using your browser over SSH tunneling
http://localhost:8888/tree?token=…
If you get a blank screen, try opening this address using your browser’s incognito mode.
Deploy the model for inference with SageMaker
After connecting to Jupyter Notebook, follow this notebook. Alternatively, choose File, New, Notebook, and then select Python 3 as the kernel. Use the following instructions and run the notebook cells.
- In the notebook, install the
sagemaker
andhuggingface_hub
libraries.
- Next, get a SageMaker session and execution role that will allow you to create and manage SageMaker resources. You’ll use a Deep Learning Container.
- Deploy the compiled model to a SageMaker real-time endpoint on AWS Inferentia2.
Change user_id
in the following code to your Hugging Face username. Make sure to update HF_MODEL_ID
and HUGGING_FACE_HUB_TOKEN
with your Hugging Face username and your access token.
- You’re now ready to deploy the model to a SageMaker real-time inference endpoint. SageMaker will provision the necessary compute resources instance and retrieve and launch the inference container. This will download the model artifacts from your Hugging Face repository, load the model to the Inferentia devices and start inference serving. This process can take several minutes.
- Next, run a test to check the endpoint. Update
user_id
to match your Hugging Face username, then create the prompt and parameters.
- Send the prompt to the SageMaker real-time endpoint for inference
- In the future, if you want to connect to this inference endpoint from other applications, first find the name of the inference endpoint. Alternatively, you can use the SageMaker console and choose Inference, and then Endpoints to see a list of the SageMaker endpoints deployed in your account.
- Use the endpoint name to update the following code, which can also be run in other locations.
Cleanup
Delete the endpoint to prevent future charges for the provisioned resources.
Conclusion
In this post, we covered how to compile and deploy the Mixtral 8x7B language model on AWS Inferentia2 using the Hugging Face Optimum Neuron container and Amazon SageMaker. AWS Inferentia2 offers a cost-effective solution for hosting models like Mixtral, providing high-performance inference at a lower cost.
For more information, see Deploy Mixtral 8x7B on AWS Inferentia2 with Hugging Face Optimum.
For other methods to compile and run Mixtral inference on Inferentia2 and Trainium see the Run Hugging Face mistralai/Mixtral-8x7B-v0.1 autoregressive sampling on Inf2 & Trn1 tutorial located in the AWS Neuron Documentation and Notebook.
About the authors
Lior Sadan is a Senior Solutions Architect at AWS, with an affinity for storage solutions and AI/ML implementations. He helps customers architect scalable cloud systems and optimize their infrastructure. Outside of work, Lior enjoys hands-on home renovation and construction projects.
Stenio de Lima Ferreira is a Senior Solutions Architect passionate about AI and automation. With over 15 years of work experience in the field, he has a background in cloud infrastructure, devops and data science. He specializes in codifying complex requirements into reusable patterns and breaking down difficult topics into accessible content.
Elevate business productivity with Amazon Q and Amazon Connect
Modern banking faces dual challenges: delivering rapid loan processing while maintaining robust security against sophisticated fraud. Amazon Q Business provides AI-driven analysis of regulatory requirements and lending patterns. Additionally, you can now report fraud from the same interface with a custom plugin capability that can integrate with Amazon Connect. This fusion of technology transforms traditional lending by enabling faster processing times, faster fraud prevention, and a seamless user experience.
Amazon Q Business is a generative AI-powered assistant that can answer questions, provide summaries, generate content, and securely complete tasks based on data and information in your enterprise systems. Amazon Q Business provides plugins to interact with popular third-party applications, such as Jira, ServiceNow, Salesforce, PagerDuty, and more. Administrators can enable these plugins with a ready-to-use library of over 50 actions to their Amazon Q Business application. Where pre-built plugins are not available, Amazon Q Business provides capabilities to build custom plugins to integrate with your application. Plugins help streamline tasks and boost productivity by integrating external services into the Amazon Q Business chat interface.
Amazon Connect is an AI-powered application that provides one seamless experience for your contact center customers and users. It’s comprised of a full suite of features across communication channels. Amazon Connect Cases, a feature of Amazon Connect, allows your agents to track and manage customer issues that require multiple interactions, follow-up tasks, and teams in your contact center. Agents can document customer issues with the relevant case details, such as date/time opened, issue summary, customer information, and status, in a single unified view.
The solution integrates with Okta Identity Management Platform to provide robust authentication, authorization, and single sign-on (SSO) capabilities across applications. Okta can support enterprise federation clients like Active Directory, LDAP, or Ping.
For loan approval officers reviewing mortgage applications, the seamless integration of Amazon Q Business directly into their primary workflow transforms the user experience. Rather than context-switching between applications, officers can harness the capabilities of Amazon Q to conduct research, analyze data, and report potential fraud cases within their mortgage approval interface.
In this post, we demonstrate how to elevate business productivity by leveraging Amazon Q to provide insights that enable research, data analysis, and report potential fraud cases within Amazon Connect.
Solution overview
The following diagram illustrates the solution architecture.
The solution includes the following steps:
- Users in Okta are configured to be federated to AWS IAM Identity Center, and a unique ID (audience) is configured for an Amazon API Gateway
- When the user chooses to chat in the web application, the following flow is initiated:
- The Amazon Q Business application uses the client ID and client secret key to exchange the Okta-generated JSON Web Token (JWT) with IAM Identity Center. The token includes the AWS Security Token Service (AWS STS) context identity.
- A temporary token is issued to the application server to assume the role and access the Amazon Q Business API.
- The Amazon Q Business application fetches information from the Amazon Simple Storage Service (Amazon S3) data source to answer questions or generate summaries.
- The Amazon Q custom plugin uses an Open API schema to discover and understand the capabilities of the API Gateway API.
- A client secret is stored in AWS Secrets Manager and the information is provided to the plugin.
- The plugin assumes the AWS Identity and Access Management (IAM) role with the kms:decrypt action to access the secrets in Secret Manager.
- When a user wants to send a case, the custom plugin invokes the API hosted on API Gateway.
- API Gateway uses the same Okta user’s session and authorizes the access.
- API Gateway invokes AWS Lambda to create a case in Amazon Connect.
- Lambda hosted in Amazon Virtual Private Cloud (Amazon VPC) internally calls the Amazon Connect API using an Amazon Connect VPC interface endpoint powered by AWS PrivateLink.
- The contact center agents can also use Amazon Q in Connect to further assist the user.
Prerequisites
The following prerequisites need to be met before you can build the solution:
- Have a valid AWS account.
- Have an Amazon Q Business Pro subscription to create Amazon Q applications.
- Have the service-linked IAM role
AWSServiceRoleForQBusiness
. If you don’t have one, create it with the amazonaws.com service name. - Have an IAM role in the account that will allow the AWS CloudFormation template to create new roles and add policies. If you have administrator access to the account, no action is required.
- Enable logging in AWS CloudTrail for operational and risk auditing.
Okta prerequisites:
- Have an Okta developer account and setup an application and API. If you do not have an Okta, please see the following instructions.
Set up an application and API in Okta
Complete the following steps to set up an application and API in Okta:
- Log in to the Okta console.
- Provide credentials and choose Login.
- Choose Continue with Google.
- You might need to set up multi-factor authentication following the instructions on the page.
- Log in using the authentication code.
- In the navigation pane, choose Applications and choose Create App Integration.
- Select OIDC – OpenID for Sign-in method and Web Application for Application type, then choose Next.
- For App integration name, enter a name (for example,
myConnectApp
). - Select Authorization Code and Refresh Token for Grant type.
- Select Skip group assignment for now for Control Access.
- Choose Save to create an application.
- Take note of the client ID and secret.
Add Authentication server and metadata
- In the navigation pane, choose Security, then choose API.
- Choose Add Authorization Server, provide the necessary details, and choose Save.
- Take note of the Audience value and choose Metadata URI.
Audience is provided as an input to the CloudFormation template later in the section.
The response will provide the metadata.
- From the response, take note of the following:
issuer
authorization_endpoint
token_endpoint
- Under Scopes, choose Add Scope, provide the name write/tasks, and choose Create.
- On the Access Policies tab, choose Add Policy.
- Provide a name and description.
- Select The following clients and choose the application by entering my in the text box and choosing the application created earlier.
- Choose Create Policy to add a policy.
- Choose Add Rule to add a rule and select only Authorization Code for Grant type is.
- For Scopes requested, select The following scopes, then enter write in the text box and select the write/tasks
- Adjust Access token lifetime is and Refresh token lifetime is to minutes.
- Add but will expire if not used every as 5 minutes.
- Choose Create rule to create the rule.
Add users
- In the navigation pane, choose Directory and choose People.
- Choose Add person.
- Complete the fields:
- First name
- Last name
- Username (use the same as the primary email)
- Primary email
- Select Send user activation email now.
- Choose Save to save the user.
- You will receive an email. Choose the link in the email to activate the user.
- Choose Groups, then choose Add group to add the group.
- Provide a name and optional description.
- Refresh the page and choose the newly created group.
- Choose Assign people to assign users.
- Add the newly created user by choosing the plus sign next to the user.
- Under Applications, select the application name created earlier.
- On the Assignments tab, choose Assign to People.
- Select the user and choose Assign.
- Choose Done to complete the assignment.
Set up Okta as an identity source in IAM Identity Center
Complete the following steps to set up Okta as an identity source:
- Enable an IAM Identity Center instance.
- Configure SAML and SCIM with Okta and IAM Identity Center.
- On the IAM Identity Center console, navigate to the instance.
- Under Settings, copy the value Instance ARN. You will need it when you run the CloudFormation template.
Deploy resources using AWS CloudFormation
In this step, we use a CloudFormation template to deploy a Lambda function, configure the REST API, and create identities. Complete the following steps:
- Open the AWS CloudFormation console in the
us-east-1
AWS Region. - Choose Create stack.
- Download the CloudFormation template and upload it in the Specify template
- Choose Next.
- For Stack name, enter a name (for example,
QIntegrationWithConnect
). - In the Parameters section, provide values for the following:
- Audience
- AuthorizationUrl
- ClientId
- ClientSecret
- IdcInstanceArn
- Issuer
- TokenUrl
- Choose Next.
- Keep the other values as default and select I acknowledge that AWS CloudFormation might create IAM resources in the Capabilities.
- Select I acknowledge that AWS CloudFormation might require the following capability: CAPABILITY_AUTO_EXPAND in the Capabilities.
- Choose Submit to create the CloudFormation stack.
- After the successful deployment of the stack, on the Outputs tab, note the value for
ALBDNSName
.
The CloudFormation template does not deploy certificates for Application Load Balancer. We strongly recommend creating a secure listener for the Application Load Balancer and deploying at least one certificate.
Assign user to Amazon Q Application
- On the Amazon Q Business console, navigate to the application named qbusiness-connect-case.
- Under User Access, choose Manage user access.
- On the user tab, choose Add groups and users and search for the user you created in Okta and propagated in IAM Identity Center.
- Choose Assign and Done.
- Choose Confirm to confirm the subscription.
- Copy the link for Deployed URL.
- Create a callback URL:
<Deployed URL>/oauth/callback
.
We recommend that you enable a budget policy notification to prevent unwanted billing.
Configure login credentials for the web application
Complete the following steps to configure login credentials for the web application:
- Navigate to the Okta developer login.
- Under Applications, choose the web application
myConnectApp
created earlier. - Choose Edit in the General Settings
- Enter the callback URL for Sign-in redirect URIs.
- Choose Save.
Sync the knowledge base
Complete the following steps to sync your knowledge base:
- On the Amazon S3 console, choose Buckets in the navigation pane.
- Search for
AmazonQDataSourceBucket
and choose the bucket. - Download the sample AnyBank regulations document.
- Upload the PDF file to the S3 bucket.
- On the Amazon Q Business console, navigate to the Amazon Q Business application.
- In the Data sources section, select the data source.
- Choose Sync now to sync the data source.
Embed the web application
Complete the following steps to embed the web application:
- On the Amazon Q Business console, under Enhancements, choose Amazon Q embedded.
- Choose Add allowed website.
- For Enter website URL, enter
http://<ALBDNSName>
.
Test the solution
Complete the following steps to test the solution:
- Copy the
ALBDNSName
value from the outputs section of the CloudFormation stack and open it in a browser.
You will see an AnyBank website.
- Choose Chat with us and the Okta sign-in page will pop up.
- Provide the sign-in details.
- Upon verification, close the browser tab.
- Navigate to the Amazon Q Business application in the chat window.
- In the chat window, enter “What are the Fraud Detection and Prevention Measures?”
Amazon Q Business will provide the answers from the knowledge base.
Next, let’s assume that you detected a fraud and want to create a case.
- Choose the plugin CreateCase and ask the question, “Can you create a case reporting fraud?”
Amazon Q Business generates the title of the case based on the question.
- Choose Submit.
- If Amazon Q Business asks you to authorize your access, choose Authorize.
The CreateCase plugin will create a case in Amazon Connect
- Navigate to Amazon Connect and open the access URL in a browser.
- Provide the user name admin and get the password from visiting the parameter store in AWS Systems Manager.
- Choose Agent Workspace.
You can see the case that was created by Amazon Q Business using the custom plugin.
Clean up
To avoid incurring future charges, delete the resources that you created and clean up your account:
- Empty the contents of the S3 buckets you created as part of the CloudFormation stack.
- Delete the CloudFormation stack you created as part of this post.
- Disable the application from IAM Identity Center.
Conclusion
As businesses navigate the ever-changing corporate environment, the combination of Amazon Q Business and Amazon Connect emerges as a transformative approach to optimizing employee assistance and operational effectiveness. Harnessing the capabilities of AI-powered assistants and advanced contact center tools, organizations can empower their teams to access data, initiate support requests, and collaborate cohesively through a unified solution. This post showcased a banking portal, but this can be used for other industrial sectors or organizational verticals.
Stay up to date with the latest advancements in generative AI and start building on AWS. If you’re seeking assistance on how to begin, check out the Generative AI Innovation Center.
About the Authors
Sujatha Dantuluri is a seasoned Senior Solutions Architect in the US federal civilian team at AWS, with over two decades of experience supporting commercial and federal government clients. Her expertise lies in architecting mission-critical solutions and working closely with customers to ensure their success. Sujatha is an accomplished public speaker, frequently sharing her insights and knowledge at industry events and conferences. She has contributed to IEEE standards and is passionate about empowering others through her engaging presentations and thought-provoking ideas.
Dr Anil Giri is a Solutions Architect at Amazon Web Services. He works with enterprise software and SaaS customers to help them build generative AI applications and implement serverless architectures on AWS. His focus is on guiding clients to create innovative, scalable solutions using cutting-edge cloud technologies.