
Large-scale models are revolutionizing deep learning and AI research, driving major improvements in language understanding, generating creative texts, multi-lingual translation and many more. But despite their remarkable capabilities, the models’ large size creates latency and cost constraints that hinder the deployment of applications on top of them. In particular, increased inference time and memory consumption inhibit deployment of models on latency-sensitive and resource-constrained applications on both server and client devices. To address these deployment challenges, the DeepSpeed team, as part of Microsoft’s AI at Scale initiative, has been exploring innovations in system optimization and model compression. On the former, we released the DeepSpeed inference system, which consists of a diverse set of optimizations, such as highly optimized CUDA kernels and inference-adapted parallelism to accelerate model inference speed, as well as ZeRO-Inference, which breaks the GPU memory wall and fits large models across heterogeneous memories to address hardware accessibility limitations. These optimizations target improving the inference system efficiency while preserving the model sizes, the amount of computation, and model accuracy: the total work remains the same, but the processing capability and speed are higher. On the latter, the emerging compression algorithms show great potential in reducing model size and inference computation. These algorithms use condensed format to represent, store, communicate, and compute DNN models, reducing the total work needed for inference with little or no loss in accuracy. System optimizations and model compression are very much complementary, and they can be synergistically combined to provide a multiplicative reduction on inference latency and cost. Motivated by combining the best of both worlds, we are proud to announce DeepSpeed Compression—a composable library that combines novel compression technologies and highly efficient system optimizations to make DL model size smaller and inference speed faster, all with much lowered compression cost.
Challenges of compressing large deep learning models
Although there have been numerous efforts to compress model sizes and reduce inference computation, applying existing compression techniques to large scale models still has many challenges in practice:
Complex pipeline for achieving high compression ratio. Various strategies have been proposed to overcome optimization difficulty and accuracy degradation when compressing large models. However, no systematic study on best practices for extreme compression exists, such as using aggressive quantization methods and layer reduction. This leaves the underlying question unanswered: do we really need those ad-hoc tricks to recover the accuracy loss or do simpler yet more effective methods exist?
High compression cost. Existing methods for compressing large models incur high training costs. For example, popular compression methods such as quantize-aware training (QAT) and multi-stage distillation methods lead to long training time and large hardware resource requirement as the model size grows into multi-billion parameters or at even larger scale, making compressing these models costly and difficult. For example, the 20B GPT-NeoX model was pre-trained using 96 NVIDIA A100 GPUs in three months. Performing QAT even with 10% of training samples would still require large amounts of computational resources, which many practitioners cannot afford.
Lack of tailored system optimizations for compressed models. To maximize the benefits of compressed models, specialized system optimizations are often required, e.g., quantized and sparsified models need optimized low-bit arithmetic computation and sparse matrix multiplication to boost the inference speed on commodity hardware. Existing methods often focus on reducing theoretical computation overhead but miss the opportunities to offer the best inference latency reduction via tailored system optimizations for the compressed models.
Limited composability. Existing methods have limited composability from two aspects. First, there is limited composability among multiple compression methods. Although well-performing compression solutions have been proposed independently, combining multiple methods together for the best outcome is still a laborious process, requiring building a complex compression pipeline. Second, there is a lack of composability between compression techniques and system optimizations. As we just mentioned, compressed models require specialized system optimizations to maximize latency and cost reduction. However, few existing methods take an end-to-end approach of composing compressions with system optimizations, as it requires significant efforts to bring modeling, algorithm, and system areas of deep learning to work synergistically together.
DeepSpeed Compression overcomes these challenges by offering novel state-of-the-art compression techniques, such as XTC for 32x smaller model size and ZeroQuant for 5000x lower compression cost reduction. It also takes an end-to-end approach to improve the computation efficiency of compressed models via a highly optimized inference engine. Furthermore, our library has multiple built-in state-of-the-art compression methods and supports synergistic composition of these methods together with the system optimizations, offering the best of both worlds while allowing a seamless and easy-to-use pipeline for efficient DL model inference. Each of these features is explained further below.
Smaller model size: 32x smaller transformer models via simple yet effective binarized extreme compression
Reducing the size of large models is critical when deploying them on both servers and client devices. In DeepSpeed Compression, we provide extreme compression techniques to reduce model size by 32x with almost no accuracy loss or to achieve 50x model size reduction while retaining 97% of the accuracy. We do this through two main techniques: extreme quantization and layer reduction. Extreme quantization via ternarization/binarization reduces the model size significantly but is considered a particularly challenging task due to the large quantization error resulting in model performance degradation. To improve the accuracy of binarized/ternarized models, existing methods often adopt complicated and computationally expensive compression pipelines, such as multi-stage distillation. However, it remains unclear how different components in extreme quantization affect the resulting performance. To tease apart their effects, we perform a systematic study on the impacts of various techniques currently used for extreme compression.
In this process, we have identified several best practices for extreme compression:
- A longer training iteration with learning rate decay is highly preferred for closing the accuracy gap of extreme quantization;
- Single-stage knowledge distillation with more training budgets is sufficient to match or even exceed accuracy from multi-stage ones;
- Training without data augmentation hurts performance on downstream tasks for various compression tasks, especially on smaller tasks;
- Lightweight layer reduction matches or even exceeds expensive pre-training distillation for task-specific compression.
Based on these findings, we greatly simplify the procedure of extreme compression and propose a new extreme compression technique, XTC, that compresses a model to its limit with lightweight layer reduction and robust binarization. XTC produces models with little loss in accuracy yet up to 50x model size reduction, as shown in Figure 1. XTC reduces the model size by 32x with almost no loss in the average score on the GLUE tasks via simple yet effective binarization technique. By combining extreme quantization and lightweight layer reduction, we can further improve the binarized model, achieving 50x model size reduction while retaining 97% of the accuracy. Given that transformers are becoming the standard architecture choice for AI, we believe the investigation and the proposed solution could be highly impactful to power large-scale models on resource-constrained devices. If you are interested in XTC, you can also find more details in our technical report “Extreme Compression for Pre-trained Transformers Made Simple and Efficient.”
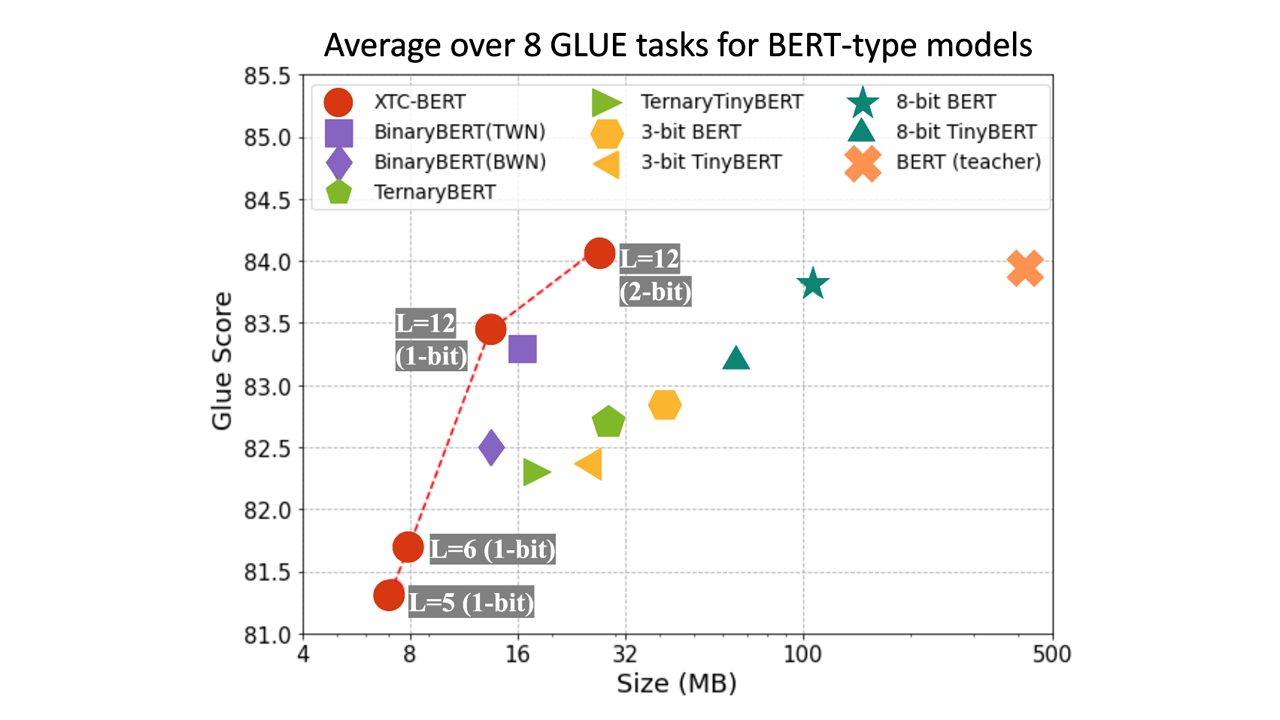
Lower compression cost: Quantizing models with >5000x compression cost reduction and no training data
Large-scale transformer models with hundreds of billions of parameters are usually challenging to quantize due to the lack of training resources and/or data access. To resolve those issues, we propose a method called ZeroQuant, which quantizes large-scale models with little or no fine-tuning cost on limited resources. Under the hood, ZeroQuant contains two major parts: 1) a hardware friendly fine-grained quantization scheme that allows us to quantize weights and activations into low-bit values with minimal errors while still empowering fast inference speed on commodity hardware with low quantization/dequantization cost; and 2) a layer-by-layer knowledge distillation pipeline, which fine-tunes the quantized model to close the accuracy gap from low-precision (e.g., INT4) quantization.
The benefits of ZeroQuant are threefold: First, unlike previous quantization-aware training that requires expensive retraining and parameter tuning, ZeroQuant enables quantizing BERT and GPT-style models from FP32/FP16 into INT8 weight and activations to retain accuracy without incurring any retraining cost, as shown in Figure 2. Second, by loading only one layer for low-precision (e.g., INT4) quantization at a time, the maximum memory footprint required to quantize the model depends solely on the size of individual layer instead of the entire model, allowing one to quantize gigantic models with as little as one GPU. Third, our quantization method is data-free, which means that it does not require the original training data of the model to obtain a quantized model. This is especially useful when the data is not available due to privacy related reasons, for example.
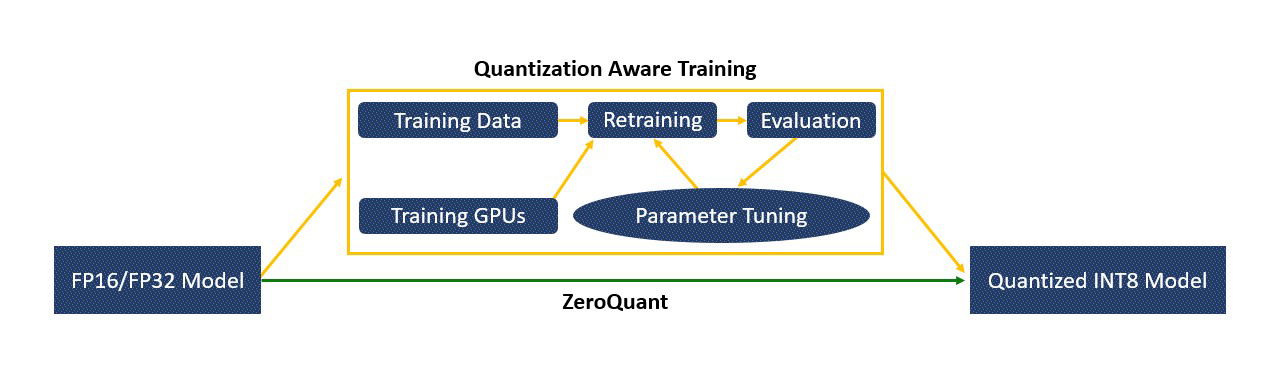
We demonstrated the scalability of ZeroQuant on a GPT-3-style model with 1.3B parameters (GPT-3-1.3B) and one of the largest open-source language models, GPT-NeoX (20B). Particularly, thanks to the fine-grained quantization scheme, ZeroQuant can convert GPT-3-1.3B (trained with 128 NVIDIA A100 with five days) and GPT-NeoX (trained with 96 A100 with three months) to INT8 without any cost or training data while delivering comparable accuracy. Furthermore, with the lightweight layer-by-layer knowledge distillation, ZeroQuant can quantize GPT-3-1.3B with mixed INT4/INT8 precision in three hours on a single GPU, which leads to 5000x compression cost reduction compared to quantization-aware training. To find more details about ZeroQuant, refer to “ZeroQuant: Efficient and Affordable Post-Training Quantization for Large-Scale Transformers”.
Faster inference speed: Latency reduction via highly optimized DeepSpeed Inference system
System optimizations play a key role in efficiently utilizing the available hardware resources and unleashing their full capability through inference optimization libraries like ONNX runtime and DeepSpeed. We build our work on top of DeepSpeed inference, which provides high-performance model serving with inference optimized kernels, parallelism, and memory optimizations, covering a wide variety of models for both latency sensitive and throughput-oriented applications. Besides leveraging these, we also extend the inference capability to support models in compressed formats. For example, we developed variations of efficient low-bit computation such as INT8 GeMM kernels. These kernels load INT8 parameters and activations from GPU device memory to the registers and use the customized INT8 GeMM implemented on top of CUTLASS tuned for different batch sizes to deliver faster GeMM computation. The kernels also fuse quantization and dequantization operations before and after GeMM, further reducing the kernel invocation overhead and improving the memory bandwidth utilization. Furthermore, our inference engine supports many-GPU transformer layers for serving transformer models across GPUs using inference-adapted parallelism strategies. For compressed models that have a smaller memory footprint, the inference engine can automatically shrink the number of GPUs required to serve a model, leading to reduced cross-GPU communication and hardware cost. For example, DeepSpeed compression leverages INT8 for GPT-NeoX (20B) and reduces the GPU requirement of serving the model from two to one, reducing latency from 65ms to 25ms, and achieving a 5.2x cost reduction. As shown in Figure 3, DeepSpeed INT8 kernels can boost performance by up to 2x compared to our own FP16 kernels, and they achieve 2.8-5.2x latency cost reduction compared to the baseline FP16 in PyTorch, significantly reducing the latency and cost of large-scale model inference.
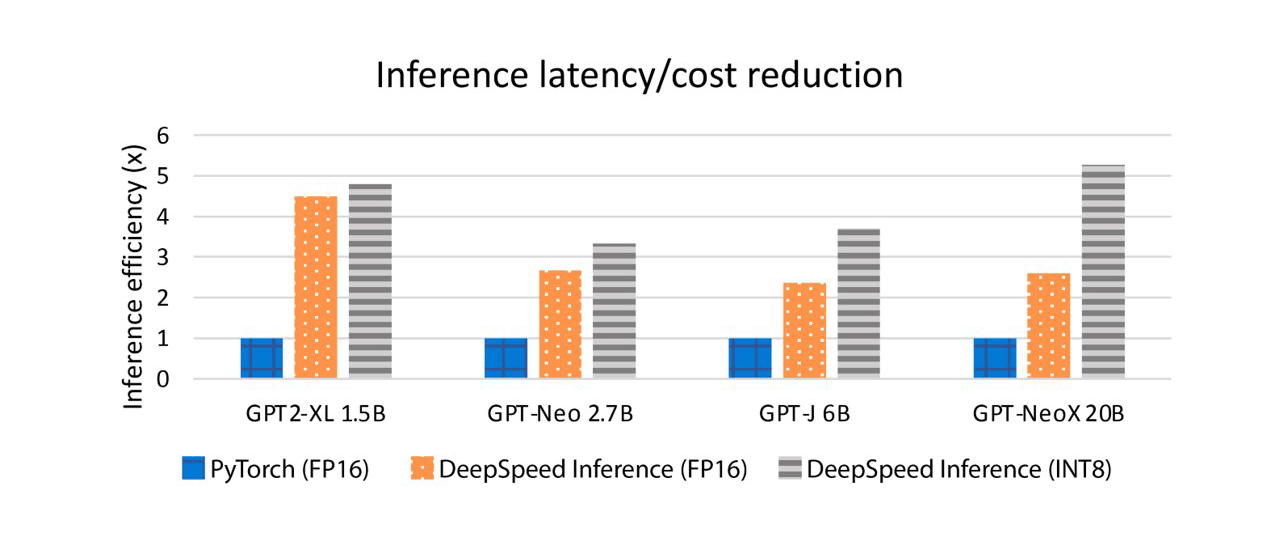
A library that synergistically composes compression algorithms and system optimizations
DeepSpeed Compression proposes a seamless pipeline to address the compression composability challenges, as shown in Figure 4. The core piece of DeepSpeed Compression is a component called compression composer, which includes several significant features:
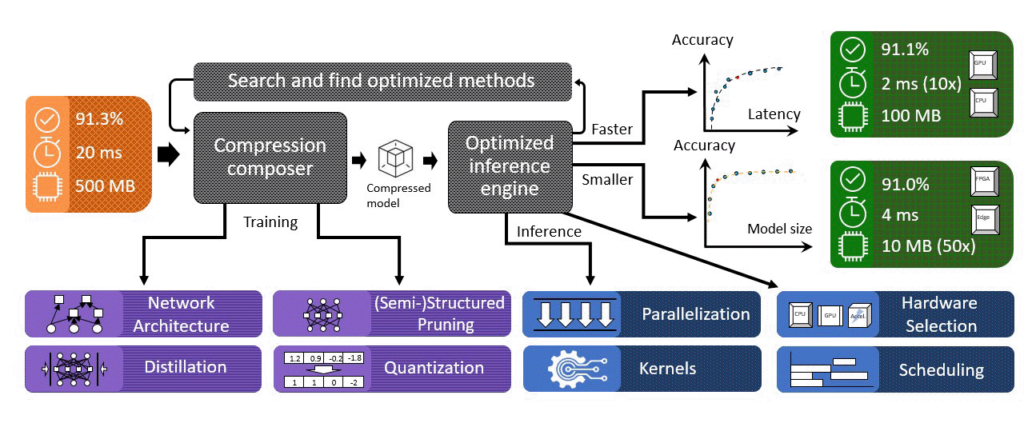
- It offers multiple cutting-edge compression methods, as shown in Table 1, including extreme quantization, head/row/channel pruning, and knowledge distillation, that can effectively reduce model size and inference cost. The list will expand as we continually integrate more state-of-the-art compression methods.
Category | Methods | Targets |
---|---|---|
Quantization | INT8/INT4 | Activations |
INT8/INT4/Ternary/Binary | Weights | |
Sparsification | Head pruning | Attention head (Transformer) |
Sparse/Row pruning | Weights | |
Channel pruning | Conv2D weights | |
Layer Reduction | Arbitrary subset of network layers | Layers |
Distillation | Output logits, feature map, attn. map | Layers |
- It offers an easy-to-use API that automatically takes care of the complexities of assembling different compression techniques to deliver the compound benefits of multiple compression methods. For example, XTC requires composition of lightweight layer reduction, binarization, and knowledge distillation. However, composing them together is non-trivial. With our compression composer, applying extreme compression is as easy as adding two new API calls to enable compression and clean the compressed model.
- It is designed in a modular way so that it will be easy for users to add new compression schemes. For example, additional compression methods can be added through custom compression layers and, by registering them with the compression composer, the new methods can be composed with existing methods that are already managed by the composer.
- It seamlessly works with the existing DeepSpeed library. This has two benefits. First, DeepSpeed Compression can be specified and enabled the same way as DeepSpeed training and inference via a JSON file, where enabling different combination of compression techniques only requires a few lines of modification in the JSON file. Second, once the compression schemes have been configured, the compression composer automatically modifies the model layers and training to enable the compression process and does not require additional changes from the user to the model structure or the training procedure.
After the DNN model has been compressed, DeepSpeed Compression replaces the compressed layers with highly optimized kernels in the DeepSpeed Inference engine to maximize hardware efficiency. Together, the compression composer and inference engine achieve the best of both worlds of compression and system optimization, delivering a compound effect of inference cost reduction.
Use Cases of DeepSpeed Compression
Although we started DeepSpeed Compression quite recently, we have successfully leveraged it to optimize several large-scale open-source models and Microsoft production workloads. It delivers significant latency and cost reduction, widely applicable on both various NLP and CV tasks.
We applied INT8 quantization of DeepSpeed Compression to optimize two large-scale open-source models in GPT-3 style: GPT-J (6B) and GPT-NeoX (20B) on the Azure AI platform. As shown in Figure 5, our quantized models achieve similar accuracy as the original models on 19 zero-shot evaluation tasks and WikiText, while achieving 3.67x and 5.2x inference cost savings, respectively, compared with PyTorch FP16 baseline on ND A100 v4 Azure instances. Very importantly, we quantize these models without requiring any training data, expensive compression time or GPU resources, bringing huge training cost savings compared with QAT!
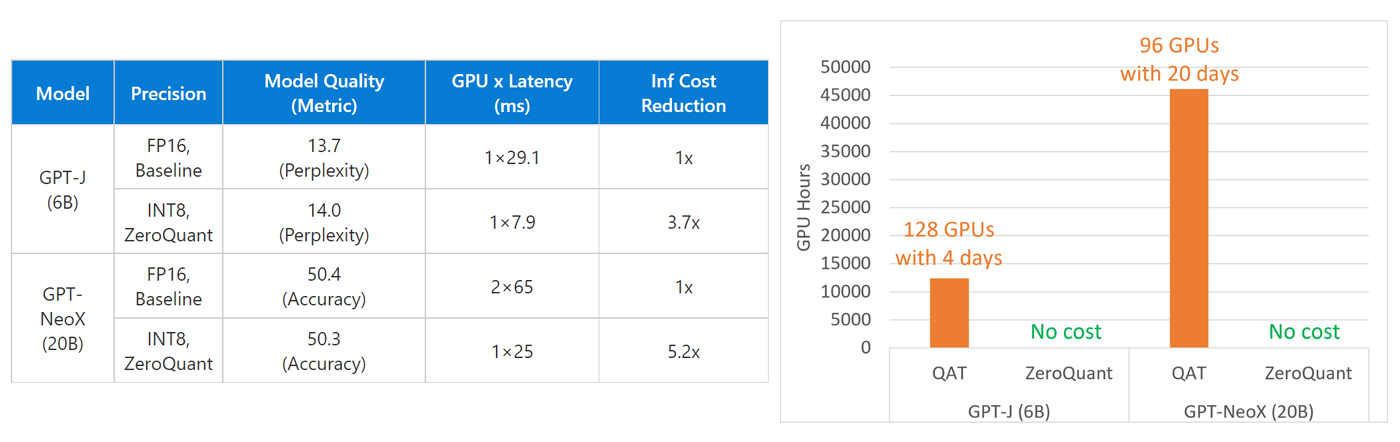
Beyond open-source models, DeepSpeed Compression has also demonstrated its effectiveness to optimize production workloads in Microsoft:
- It reduces the Microsoft Turing Image Super Resolution model (T-ISR) model size by 3.1x together with 1.85x latency reduction by composing different compression schemes like pruning and distillation with efficient system optimizations. The model has also been deployed in Bing Maps and Microsoft Edge, where it automatically derives high-resolutions images from lower-resolution images, which can be seen in this blog post.
- It also successfully compresses the Microsoft Relevance Fusion models—a Transformer-based ranking model used in Bing’s core search stack. Without DeepSpeed Compression, it took three days to quantize the model using QAT. With DeepSpeed Compression, we can quantize the model in a few minutes with improved accuracy and reduced latency compared to QAT.
DeepSpeed Compression release plan
DeepSpeed Compression is still at its early stage and under active development, but we’d like to share the results and tools to DeepSpeed users as soon as possible. At this first release, we open-source the core DeepSpeed Compression components, including the compression composer, which supports various compression methods consisting of INT8/INT4/Ternary/Binary quantization, lightweight layer reduction, pretraining and task specific knowledge distillation, head pruning, row pruning, and channel pruning, for compressing both NLP and computer vision models. Together with the compression composer, we are releasing the two novel technologies XTC and ZeroQuant introduced in this blog as part of the library.
We hope you will try DeepSpeed Compression. Please find the code, tutorial, and documents at the DeepSpeed GitHub, and website. We highly value your feedback and comments, so let us know what you think and how we can improve. As for the next steps, we plan to extend our offerings with more compression methods, an extended coverage of specialized kernels for compressed models, and an optimization module that automatically finds the best compression schemes. We believe that our composable library and new innovations will help close the gap between what is possible in AI and what is deployable as well as making DL inference faster, cheaper, and simpler.
Acknowledgement
We are a group of system and modeling researchers—Zhewei Yao, Xiaoxia Wu, Minjia Zhang, Conglong Li, Reza Yazdani Aminabadi, Elton Zheng, Samyam Rajbhandari, Ammar Ahmad Awan, Jeff Rasley, Cheng Li, Olatunji Ruwase, Shaden Smith, Du Li, Michael Wyatt, Arash Bakhtiari, Guanhua Wang, Connor Holmes, Sam Ade Jacobs, Martin Cai, Yuxiong He (team lead)—who are enthusiastic about performance optimization of large-scale systems. We have recently focused on deep learning systems, optimizing deep learning’s speed to train, speed to convergence, and speed to develop.
The post DeepSpeed Compression: A composable library for extreme compression and zero-cost quantization appeared first on Microsoft Research.