This is a guest post by the data science team at Bayer Crop Science.
Farmers have always collected and evaluated a large amount of data with each growing season: seeds planted, crop protection inputs applied, crops harvested, and much more. The rise of data science and digital technologies provides farmers with a wealth of new information. At Bayer Crop Science, we use AI and machine learning (ML) to help farmers achieve more bountiful and sustainable harvests. We also use data science to accelerate our research and development process; create efficiencies in production, operations, and supply chain; and improve customer experience.
To evaluate potential products, like a short-stature line of corn or an advanced herbicide, Bayer scientists often plant a small trial in a greenhouse or field. We then use advanced sensors and analytical models to evaluate the experimental results. For example, we might fly an unmanned aerial vehicle over a field and use computer vision models to count the number of plants or measure their height. In this way, we’ve collected data from millions of test plots around the world and used them to train models that can determine the size and position of every plant in our image library.
Analytical models like these are powerful but require effort and skill to design and train effectively. science@scale, the ML engineering team at Bayer Crop Science, has made these techniques more accessible by integrating Amazon SageMaker with open-source tools like KubeFlow Pipelines to create reproducible templates for analytical model training, hosting, and access. These resources help standardize how our data scientists interact with SageMaker services. They also make it easier to meet Bayer-specific requirements, such as using multiple AWS accounts and resource tags.
Standardizing the ML workflow for Bayer Crop Science
Data science teams at Bayer Crop Science follow a common pattern to develop and deploy ML models:
- A data scientist develops model and training code in a SageMaker notebook or other coding environment running in a project-specific AWS account.
- A data scientist trains the model on data stored in Amazon Simple Storage Service (Amazon S3).
- A data scientist partners with an ML engineer to deploy the trained model as an inference service.
- An ML engineer creates the API proxies required for applications outside of the project-specific account to call the inference service.
- ML and other engineers perform additional steps to meet Bayer-specific infrastructure and security requirements.
To automate this process, our team transformed the steps into a reusable, parameterized workflow using KubeFlow Pipelines (KFP). Each step of a workflow (a KFP component) is associated with a Docker container and connected via the KFP Pipelines framework. Using Kubeflow to host Bayer’s model training and deployment process was enabled through the use of the Amazon SageMaker Components for KubeFlow Pipelines, pre-built modules that simplify the process of running SageMaker operations from within KFP. We combined these with custom components to automate the Bayer-specific engineering steps, particularly those relating to cybersecurity. The resulting pipeline allows data scientists to trigger model training and deployment with only a few lines of code and ensures that the model artifacts are generated and maintained consistently. This provides data scientists more time to focus on improving the models themselves.
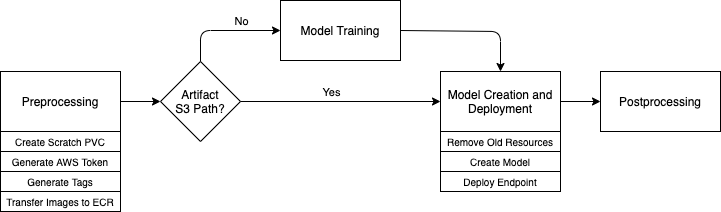
AWS account setup
Bayer Crop Science organizes its cloud resources into a large number of application-, team-, and project-specific accounts. For this reason, many ML projects require resources in at least three AWS accounts:
- ML support account – Contains the shared infrastructure necessary to perform Bayer-specific proxy generation and other activities across multiple projects
- KubeFlow account – Contains an Amazon Elastic Kubernetes Service (Amazon EKS) cluster hosting our KubeFlow deployment
- Scientist account – At least one project-specific account in which data scientists store most of the required data and perform model development and training
The following diagram illustrates this architecture.
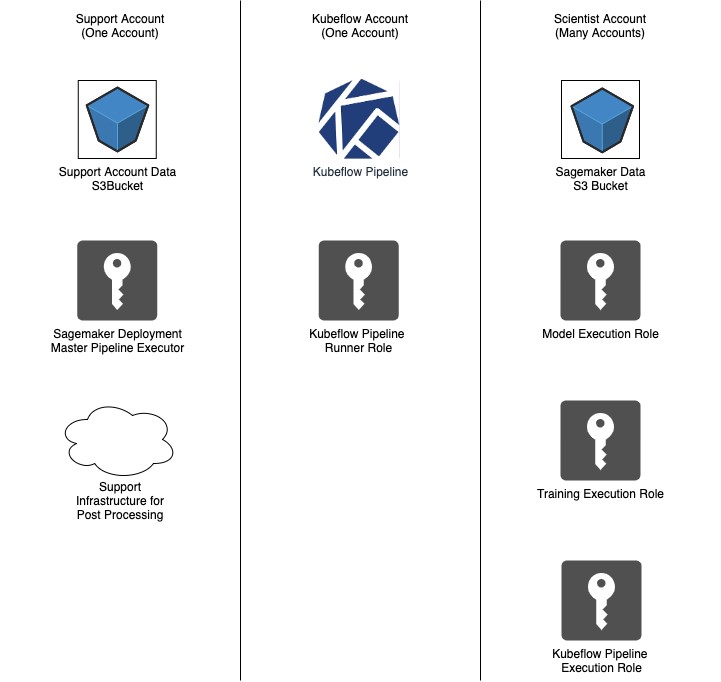
ML support AWS account
One centralized account contains the infrastructure required to perform Bayer-specific post-processing steps across multiple ML projects. Most notably, this includes a KubeFlow Master Pipeline Execution AWS Identity and Access Management (IAM) role. This role has trust relationships with all the pipeline execution roles in the scientist account, which it can assume when running the pipeline. It’s separate from the Pipeline Runner IAM role in the KubeFlow AWS account to allow management of these relationships independent from other entities within the KubeFlow cluster. The following code shows the trust relationship:
Trust Relationship (one element for each scientist account):
{
"Version": "2012-10-17",
"Statement": [
{
"Sid": "",
"Effect": "Allow",
"Principal": {
"AWS": "arn:aws:iam::[kubeflow-account-number]:role/[kubeflow-pipeline-exeution-role-name]"
},
"Action": "sts:AssumeRole"
}
]
}
KubeFlow AWS account
Bayer Crop Science uses a standard installation of KubeFlow hosted on Amazon EKS in a centralized AWS account. At the time of this writing, all KubeFlow pipelines run within the same namespace on a KubeFlow cluster and all components assume a custom IAM role when they run. The components can inherit the role from the worker instance, applied via OIDC integration (preferred) or obtained using open-source methods such as kube2iam.
Scientist AWS account
To enable access by model training and hosting resources, all scientist accounts must contain several IAM roles with standard permission sets. These are typically provisioned on request by an ML engineer using Terraform. These roles include:
- Model Execution – Supports SageMaker inference endpoints
- Training Execution – Supports SageMaker training jobs
- KubeFlow Pipeline Execution – Supports creating, updating, or deleting resources using the Amazon SageMaker Components for KubeFlow Pipelines
These IAM roles are given policies that are appropriate for their associated tasks, which can vary depending on organizational needs. An S3 bucket is also created to store trained model artifacts and any data required by the model during inference or training.
KubeFlow pipeline setup
Our ML pipeline (see the following diagram) uses Amazon SageMaker Components for KubeFlow Pipelines to standardize the integration with SageMaker training and deployment services.
The ML pipeline exposes the parameters summarized in the following table.
Parameter |
Description |
model_name |
Name of the model to train and deploy. Influences the job, endpoint, endpoint config, and model names. |
model_docker_image |
If present, the pipeline attempts to deploy a model using this base Docker image. |
model_artifact_s3_path |
If a model artifact already exists and doesn’t need to be trained, its S3 path can be specified. |
environment |
JSON object containing environment variables injected into the model endpoint. |
training_algorithm_name |
If training without a Docker image, one of preconfigured AWS training algorithms can be specified. |
training_docker_image |
If training with a base Docker image, it can be specified here. |
training_hyperparameters |
JSON object containing hyperparameters for the training job. |
training_instance_count |
Specifies the number of training instances for use in distributed training scenarios. |
training_instance_type |
String indicating which ML instance type is used to host the training process. |
endpoint_instance_type |
String indicating which ML instance type is used to host the endpoint process. |
training_channels |
JSON array of data channels that are injected into the training job. |
training_s3_output_path |
Base S3 path where model artifacts are written in the case of a training job. |
account_id |
Account number of the data scientist account. Used in role assumption logic. |
See the following pipeline code:
@dsl.pipeline(name='Kubeflow Sagemaker Component Deployment Pipeline')
def pipeline(model_name = "",
account_id = "",
model_docker_image = "",
model_artifact_s3_path = "",
environment = '{}',
training_algorithm_name = '',
training_docker_image = "",
training_hyperparameters = '{}',
training_instance_count = 2,
endpoint_instance_type = "ml.m5.large",
training_instance_type = "ml.m5.large",
training_channels = '',
training_s3_output_path = ""
):
….Pipeline Component Code
if __name__ == '__main__':
kfp.compiler.Compiler().compile(pipeline, __file__ + '.tar.gz')
print("Pipeline compiled successfully.")
To create the pipeline, we ran the .py file to compile it into a .tar.gz file and uploaded it into the KubeFlow UI.
Running the pipeline
After pipeline creation is complete, data scientists can invoke the pipeline from multiple Jupyter notebooks using the KubeFlow SDK. They can then track the pipeline run for their model in the KubeFlow UI. See the following code:
kfp_token = get_oauth_token(client_id, client_secret)
kfp_client = kfp.Client(host=kubeflow_api, client_id=client_id, existing_token=kfp_token)
print("Connect to: " + str(kfp_client._run_api.api_client.configuration.host))
experiment = kfp_client.get_experiment(experiment_name="Default")
print(experiment)
def get_sgm_deploy_pipeline_id():
pipelines = kfp_client.list_pipelines(page_size=1000)
pipeline_id = None
for pipeline in pipelines.pipelines:
if pipeline.name == "sagemaker-components-poc":
pipeline_id = pipeline.id
break
return pipeline_id
sagemaker_deployment_parameters = {
"model_name": "your-model-name",
"account_id": boto3.client("sts").get_caller_identity()["Account"],
"model_docker_image": "520713654638.dkr.ecr.us-east-1.amazonaws.com/sagemaker-tensorflow-serving:1.12-cpu",
"environment": json.dumps({ "SAGEMAKER_TFS_NGINX_LOGLEVEL": "info"}),
"training_docker_image": "520713654638.dkr.ecr.us-east-1.amazonaws.com/sagemaker-tensorflow-scriptmode:1.12-cpu-py3",
"training_hyperparameters": json.dumps({
"model_dir": "/opt/ml/model",
"sagemaker_container_log_level": "20",
"sagemaker_enable_cloudwatch_metrics": "false",
"sagemaker_mpi_custom_mpi_options": "-verbose --NCCL_DEBUG=INFO -x OMPI_MCA_btl_vader_single_copy_mechanism=none",
"sagemaker_mpi_enabled": "true",
"sagemaker_mpi_num_of_processes_per_host": "2",
"sagemaker_program": "train_mnist.py",
"sagemaker_region": "us-east-1",
"sagemaker_submit_directory": "s3://path/to/sourcedir.zip"
}),
"training_instance_count": "2",
"training_channels": '[{"ChannelName":"train","DataSource":{"S3DataSource":{"S3Uri":"s3://path/to/training-data","S3DataType":"S3Prefix","S3DataDistributionType":"FullyReplicated"}},"ContentType":"","CompressionType":"None","RecordWrapperType":"None","InputMode":"File"},{"ChannelName":"test","DataSource":{"S3DataSource":{"S3Uri":"s3://path/to/test/data","S3DataType":"S3Prefix","S3DataDistributionType":"FullyReplicated"}},"ContentType":"","CompressionType":"None","RecordWrapperType":"None","InputMode":"File"}]',
"training_s3_output_path": "s3://path/to/model/artifact/output/"
}
run = {
"name": "my-run-name",
"pipeline_spec": {
"parameters": [
{ "name": param, "value": sagemaker_deployment_parameters[param] } for param in sagemaker_deployment_parameters.keys()
],
"pipeline_id": get_sgm_deploy_pipeline_id()
},
"resource_references": [
{
"key": {
"id": experiment.id,
"type": "EXPERIMENT"
},
"relationship": "OWNER"
}
]
}
requests.post("{}/apis/v1beta1/runs".format(kubeflow_api), data=json.dumps(run), headers={ "Authorization": "Bearer " + kfp_token })
Each run consists of a series of steps:
- Create a persistent volume claim.
- Generate AWS credentials.
- Generate resource tags.
- (Optional) Transfer the Docker image to Amazon Elastic Container Registry (Amazon ECR).
- Train the model.
- Generate a model artifact.
- Deploy the model on SageMaker hosting services.
- Perform Bayer-specific postprocessing.
Step 1: Creating a persistent volume claim
The first step of the process verifies that a persistent volume claim (PVC) exists within the Kubernetes cluster hosting the KubeFlow instances. This volume is returned to the pipeline and used to pass data to various components within the pipeline. See the following code:
def get_namespace():
return open("/var/run/secrets/kubernetes.io/serviceaccount/namespace").read()
def check_pvc_exists(pvc):
config.load_incluster_config()
v1 = client.CoreV1Api()
namespace = get_namespace()
try:
response = v1.read_namespaced_persistent_volume_claim(pvc, namespace)
except ApiException as error:
if error.status == 404:
print("PVC {} does not exist, so it will be created.".format(pvc))
return False
raise
print(response)
return True
def create_pvc(pvc_name):
config.load_incluster_config()
v1 = client.CoreV1Api()
namespace = get_namespace()
pvc_metadata = client.V1ObjectMeta(name=pvc_name)
requested_resources = client.V1ResourceRequirements(requests={"storage": "50Mi"})
pvc_spec = client.V1PersistentVolumeClaimSpec(
access_modes=["ReadWriteMany"],
resources=requested_resources,
storage_class_name="efs",
data_source=None,
volume_name=None
)
k8s_resource = client.V1PersistentVolumeClaim(
api_version="v1",
kind="PersistentVolumeClaim",
metadata=pvc_metadata,
spec=pvc_spec
)
response = v1.create_namespaced_persistent_volume_claim(namespace, k8s_resource)
print(response)
Step 2: Generating AWS credentials
This step generates a session token for the pipeline execution role in the specified scientist AWS account. It then writes a credentials file to the PVC in a way that allows boto3 to access it as a configuration. Downstream pipeline components mount the PVC as a volume and use the credentials file to perform operations against SageMaker.
This credential generation step is required for KubeFlow to operate across multiple AWS accounts in Bayer’s environment. This is because all pipelines run in the same namespace and run using the generic KubeFlow Pipeline Runner IAM role from the Kubeflow AWS account. Each pipeline in Bayer’s Kubeflow environment has a dedicated IAM role associated with it that has a trust relationship with the Kubeflow Pipeline Runner IAM role. For this deployment workflow, the KSageMaker Deployment Master Pipeline Executor IAM role is assumed by the KubeFlow Pipeline Runner IAM role, and then the appropriate deployment role within the data scientist account is assumed by that role in turn. This keeps the trust relationships for the deployment process as self-contained as possible. See the following code:
import os
credentials_file_path = "/tmp/aws_credentials"
if os.path.exists(credentials_file_path):
os.remove(credentials_file_path)
import argparse
import sts_ops
parser = argparse.ArgumentParser()
parser.add_argument("--account_id", help="AWS Account Id", required=True)
parser.add_argument("--master_pipeline_role", help="ARN of master pipeline role", required=True)
args = parser.parse_args()
master_session = sts_ops.assume_master_pipeline_role(args.master_pipeline_role)
creds = sts_ops.generate_deploy_session_credentials(master_session, args.account_id)
credentials_output = """[default]
aws_access_key_id = {}
aws_secret_access_key = {}
aws_session_token = {}
""".format(creds["AccessKeyId"], creds["SecretAccessKey"], creds["SessionToken"])
open("/tmp/aws_credentials", "w").write(credentials_output)
open("/tmp/aws_credentials_location.txt", "w").write(credentials_file_path)
Step 3: Generating resource tags
Within Bayer, a standard set of tags are used to help identify Amazon resources. These tags are specified in an S3 path and applied to the model and endpoints via parameters in the corresponding SageMaker components.
Step 4: (Optional) Transferring a Docker image to Amazon ECR
If the model training and inference images are not stored in a SageMaker-compatible Docker repository, this step copies them into Amazon ECR using a custom KubeFlow component.
Step 5: Training the model
The SageMaker Training KubeFlow Pipelines component creates a SageMaker training job and outputs a path to the eventual model artifact for downstream use. See the following code:
train_model_op = kfp.components. load_component_from_url('https://raw.githubusercontent.com/kubeflow/pipelines/cb36f87b727df0578f4c1e3fe9c24a30bb59e5a2/components/aws/sagemaker/train/component.yaml')
train_model_step = apply_environment_variables(train_model_op(
algorithm_name=training_algorithm_name,
hyperparameters=training_hyperparameters,
image=training_docker_image,
instance_type=training_instance_type,
channels=training_channels,
region=aws_region,
instance_count=training_instance_count,
role="arn:aws:iam::{}:role/sagemaker-deploy-model-execution-role".format(account_id),
model_artifact_path=training_s3_output_path,
network_isolation=False
), sgm_volume, create_secret_step.output
Step 6: Generating a model artifact
The SageMaker Create Model KubeFlow Pipelines component generates a .tar.gz file containing the model configuration and trained parameters for downstream use. If a model artifact already exists in the specified S3 location, this step deletes it before generating a new one. See the following code:
sagemaker_create_model_op = components.load_component_from_url('https://raw.githubusercontent.com/kubeflow/pipelines/cb36f87b727df0578f4c1e3fe9c24a30bb59e5a2/components/aws/sagemaker/model/component.yaml')
sagemaker_create_model_step = sagemaker_create_model_op(
region=aws_region,
model_name=model_name,
image=image,
role="arn:aws:iam::{}:role/sagemaker-deploy-model-execution-role".format(account_id),
model_artifact_url=model_artifact_url,
network_isolation=False,
environment=environment,
tags=tags
)
Step 7: Deploying the model on SageMaker hosting services
The SageMaker Create Endpoint KubeFlow Pipelines component creates an endpoint configuration and HTTPS endpoint. This process can take some time because the component pauses until the endpoint is in a ready state. See the following code:
Sagemaker_deploy_op = components.load_component_from_url('https://raw.githubusercontent.com/kubeflow/pipelines/cb36f87b727df0578f4c1e3fe9c24a30bb59e5a2/components/aws/sagemaker/deploy/component.yaml')
create_endpoint_step = apply_environment_variables(sagemaker_deploy_op(
region=aws_region,
endpoint_config_name=full_model_name,
model_name_1=full_model_name,
instance_type_1=endpoint_instance_type,
endpoint_name=full_model_name,
endpoint_config_tags=generate_tags_step.output,
endpoint_tags=generate_tags_step.output
), sgm_volume, create_secret_step.output)
create_endpoint_step.after(create_model_step)
Step 8: Performing Bayer-specific postprocessing
Finally, the pipeline generates an Amazon API Gateway deployment and other Bayer-specific resources required for other applications within the Bayer network to use the model.
Conclusion
Data science is complex enough without asking data scientists to take on additional engineering responsibilities. By integrating open-source tools like KubeFlow with the power of Amazon SageMaker, the science@scale team at Bayer Crop Science is making it easier to develop and share advanced ML models. The MLOps workflow described in this post gives data scientists a self-service method to deploy scalable inference endpoints in the same notebooks they use for exploratory data analysis and model development. The result is rapid iteration, more successful data science products, and ultimately greater value for our farmer customers.
In the future, we’re looking forward to adding additional SageMaker components for hyperparameter optimization and data labeling to our pipeline. We’re also looking at ways to recommend instance types, configure endpoint autoscaling, and support multi-model endpoints. These additions will allow us to further standardize our ML workflows.
About the Authors
Thomas Kantowski is a cloud engineer at Bayer Crop Science. He received his master’s degree from the University of Oklahoma.
Brian Loyal leads science@scale, the enterprise ML engineering team at Bayer Crop Science.
Bhaskar Dutta is a data scientist at Bayer Crop Science. He designs machine learning models using deep neural networks and Bayesian statistics.
Read More