Processing large documents like PDFs and static images is a cornerstone of today’s highly regulated industries. From healthcare information like doctor-patient visits and bills of health, to financial documents like loan applications, tax filings, research reports, and regulatory filings, these documents are integral to how these industries conduct business. The mechanisms by which these documents are processed and analyzed, however, are often manual, time-consuming, error-prone, expensive, and not easily scalable.
Fortunately, recent innovations in this space are helping companies improve these methods. Machine learning (ML) techniques such as optical character recognition (OCR) and natural language processing (NLP) enable firms to digitize and extract text from millions of documents and understand the content, including contextual nuances of the language within them. Furthermore, you can then transform the extracted text by merging it with supplemental data to produce additional business insights.
This step-by-step method is called a document processing pipeline. The pipeline includes various components to extract, transform, enrich, and conform the data. New data domains are often introduced and used for numerous downstream business purposes. For example, in financial services, you could be identifying connected financial events, calculating environmental risk scores, and developing risk models. Because these documents help inform or even dictate such important data-driven decisions, it’s imperative for regulated industry companies to establish and maintain a robust data governance framework as part of these document processing pipelines. Without governance, pipelines become a dumping ground where documents are inconsistently stored, duplicated, and processed, and the business is unable to explain to potential auditors where the data that fed their decisions came from, or what that data was used for.
A data governance framework is made up of people, processes, and technology. It enables business users to work collaboratively with technologists to drive clean, certified, and trusted data. It consists of several components including data quality, data catalog, data ownership, data lineage, operation, and compliance. In this post, we discuss data catalog, data ownership, and data lineage, and how they tie together with building document processing pipelines for regulated industries.
For more information about design patterns on data quality, see How to Architect Data Quality on the AWS Cloud.
Data lineage
Data lineage is the part of data governance that refers to the practice of providing GPS services for data. At any point in time, it can explain where the data originated, what happened to it, what its latest status is, and where it’s headed from this point on.
It provides visibility while simplifying the ability to trace financial numbers back to their origin, and provides transparency on potential errors and their root cause analyses.
Furthermore, you can use data lineage captured over time as analytical inputs to drive accuracy scores.
It’s imperative for a document processing pipeline to have a well-defined data lineage framework. The framework should include an end-to-end lifecycle, responsibility model, and the technology to enable data transformation transparency. Without lineage, the data can’t be trusted.
To illustrate this end-to-end data lineage concept, we walk you through creating an NLP-powered document search engine with built-in lineage at each step. Every object and piece of data processed by this ML pipeline can be traced back to the original document.
Each processing component can be replaced by your choice of tooling or bespoke ML model. Furthermore, you can customize the solution to include other use cases, such as central document data lakes or supplemental tabular data feed to an online transaction processing (OLTP) application.
The solution follows an event-driven architecture in which the completion of each stage within the pipeline triggers the next step, while providing self-service lineage for traceability and monitoring. In addition, hooks have been included to provide capabilities to extend the pipeline to additional workloads.
This design uses the following AWS services (you can also follow along in the GitHub repo):
- Amazon Comprehend – An NLP service that uses ML to find insights and relationships in text, and can do so in multiple languages.
- Amazon DynamoDB – A key-value and document database that delivers single-digit millisecond performance at any scale.
- Amazon DynamoDB Streams – A change data capture (CDC) service. It captures an ordered flow of information about changes to items in a DynamoDB table. When you enable a stream on a table, DynamoDB captures information about every modification to data items in the table.
- Amazon Elasticsearch Service (Amazon ES) – A fully managed service that makes it possible for you to deploy, secure, and run Elasticsearch cost-effectively and at scale. You can build, monitor, and troubleshoot your applications using the tools you love, at the scale you need.
- AWS Lambda – A serverless compute service that runs code in response to triggers such as changes in data, shifts in system state, or user actions. Because Amazon S3 can directly trigger a Lambda function, you can build a variety of real-time serverless data-processing systems.
- Amazon Simple Notification Service (Amazon SNS) – An AWS managed service for application-to-application communications, with a pub/sub model enabling high-throughput, low-latency message relaying.
- Amazon Simple Queue Service (Amazon SQS) – A fully managed message queuing service that enables you to decouple and scale microservices, distributed systems, and serverless applications.
- Amazon Simple Storage Service (Amazon S3) – An object storage service to stores your documents and allows for central management with fine-tuned access controls.
- Amazon Textract – A fully managed ML service that automatically extracts printed text, handwriting, and other data from scanned documents that goes beyond OCR to identify, understand, and extract data from forms and tables.
Architecture
The overall design is grouped into five segments:
- Metadata services module
- Ingestion module
- OCR module
- NLP module
- Analytics module
All components interact via asynchronous events to allow for scalability. The following diagram illustrates the conceptual design.
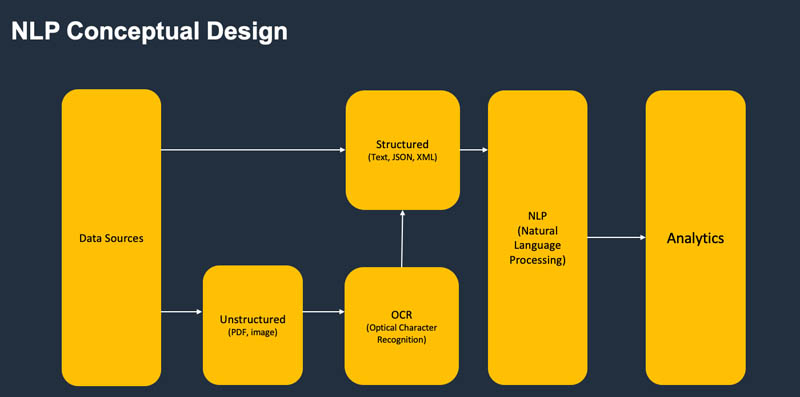
The following diagram illustrates the physical design.
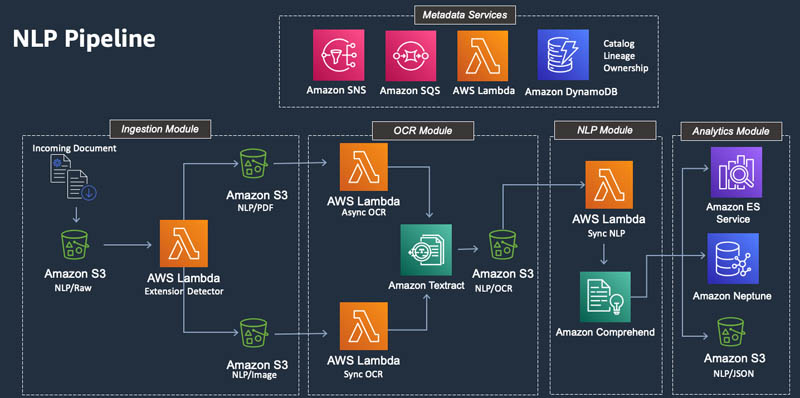
Metadata services
This is an encapsulated module to register, track, and trace incoming documents. It’s designed to be used across many different document processing pipelines. In your organization, one team might decide to use the OCR and NLP modules designed in this post. Another team might decide to use a different pipeline. However, governance practices of each pipeline should be consistent, and documents should be registered one time with full transparency on movement and downstream usage. Each document can be processed several times. You can extend the catalog and lineage services designed in this post to keep track of many pipelines, from multiple sources of data.
At the core, the metadata services module contains four reference tables, an SNS topic, three SQS queues, and three self-contained Lambda functions. Tables are created in DynamoDB, and schemas can be easily extended to include additional data attributes deemed important for your pipeline.
In addition, you can extend this design to include additional data governance components such as data quality.
The tables are defined as follows.
Table Name |
Purpose |
DynamoDB Stream Enabled? |
Data Governance component |
Sample Use |
Document Registry |
Keeps track of all incoming documents. Each document is assigned a unique document ID and registered one time in this table. |
Yes |
Catalog |
Provides the ability to quickly look up and understand the document source and context metadata. |
Document Ownership |
Covers responsibility model of the data governance in which each document acquired to the pipeline has a defined owner. |
No |
Ownership |
Provides notification services and can be extended to manage data quality controls. |
Document Lineage |
Keeps track of all data movements. It provides detailed lineage info that includes the source S3 bucket name, destination S3 bucket name, source file name, target file name, ARN ID of the AWS service that processed the document, and timestamp. |
No |
|
A simple PartiQL query against this table based on the document ID provides a list of all steps the original document has taken. Query output can include the following columns:
· Document ID
· Original document name
· Timestamp
· Source S3 bucket
· Source file name
· Destination S3 bucket
· Destination file name
|
Pipeline Operations |
Keeps a record of all pipeline actions taken on a document ID, including the current pipeline stage and its status, and keeps a timeline of the stages in chronological order. |
Yes |
|
An operational query on a document ID to determine where in the pipeline the current document processing is. |
DynamoDB Streams allows downstream application code to react to updates to objects in DynamoDB. It provides a mechanism to keep an event-based microservices architecture in place by triggering subsequent steps of a workflow whenever new documents are written to our Document Registry table, and subsequently when new document references are created in the Pipeline Operations table.
In addition, DynamoDB Streams provides developer teams with an efficient way of connecting your application logic to various updates in the tables (for example, to keep track of a particular document ID based on owner tags, or alert when certain unexpected problems arise while processing some documents).
The Lambda functions provide microservices API call capabilities for the document pipeline to self-register its movements and actions undertaken by the pipeline code:
- Document Arrival Register API – Registers the incoming document’s metadata and location within Document Registry table
- Document Lineage API – Registers the lineage information within Document Lineage table
- Pipeline Operations API – Provides up-to-date information on the state of the pipeline
The SNS topic is used as a sink for incoming messages from all pipeline movements and document registrations. It disseminates the messages to each downstream subscribed SQS queue according to what type of message was received. In this model, the number of consumers of the messages coming through the SNS topic could be greatly expanded as needed, and all messages are guaranteed to stay in order, because both the SNS topics and SQS queues are created in a First-In-First-Out (FIFO) configuration to prevent duplicates and maintain single-threaded processing in the pipeline.
Using Amazon SNS in the design provides scalability by creating a pub/sub architecture. A pub/sub architecture design is a pattern that provides a framework to decouple the services that produce an event from services that process the event. Many subscribers can subscribe to the same event and trigger different pipelines. For example, this design can easily be extended to process incoming XML file formats by subscribing an additional XML process pipeline for the same event.
The following table provides schema information. The document ID is identical and unique for each document and is part of the composite primary key used to identify movement of each document within the pipeline.
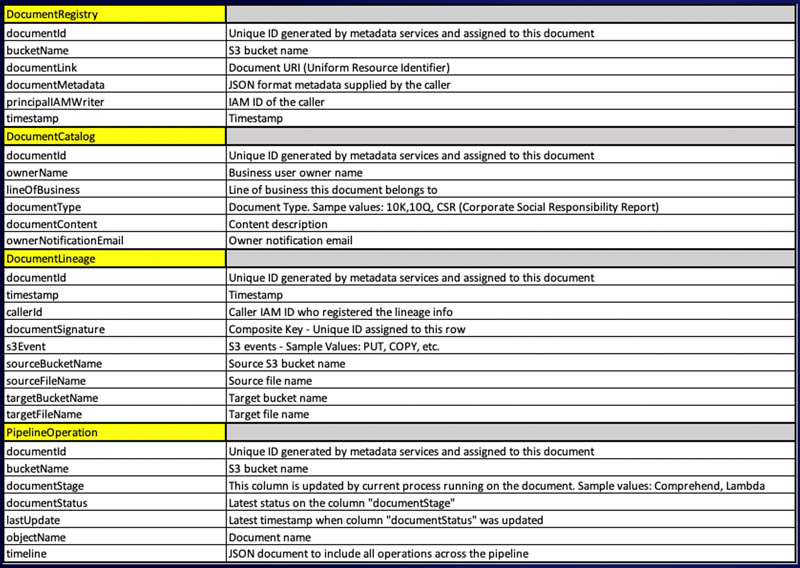
The following diagram shows the architecture of our metadata services.
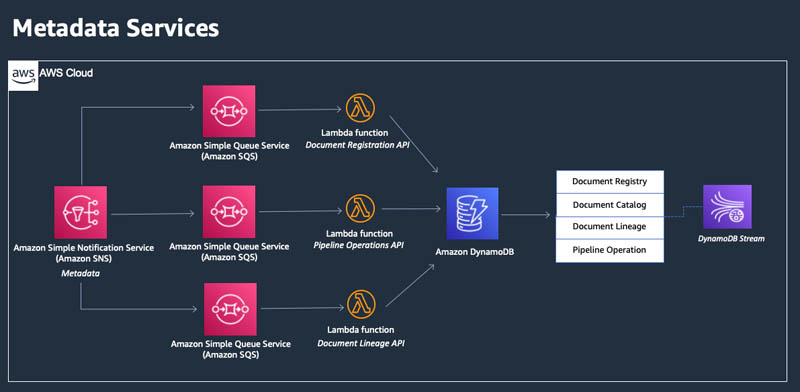
Ingestion module
The ingestion workload is triggered when a new document is uploaded to the NLP/Raw S3 bucket (or the bucket where raw documents are placed from users or front-end applications).
The ingestion module follows a four-step process (as shown in the following diagram):
- A document is uploaded to the NLP/Raw S3 bucket.
- The Document Registrar Lambda function is invoked, which calls the metadata services API to register the document and receive a unique ID. This ID is added to the document as a tag, and the metadata is registered within the DynamoDB table Document Registry.
- After the document metadata is registered with Metadata Services, the DynamoDB Document Registration stream is invoked to start the Document Classification Lambda function. This function examines the metadata registered on the document and determines if the downstream OCR segment should be invoked on this document. The result of this examination is written back to the metadata services.
- The metadata registration of the previous step invokes a DynamoDB Pipeline Operations Stream, which invokes the Document Extension Detector Lambda function. This function examines the incoming file formats and separates the images files from PDF documents.
All steps are registered in metadata services. The red dotted lines in the following diagram represent the metadata asynchronous API calls.
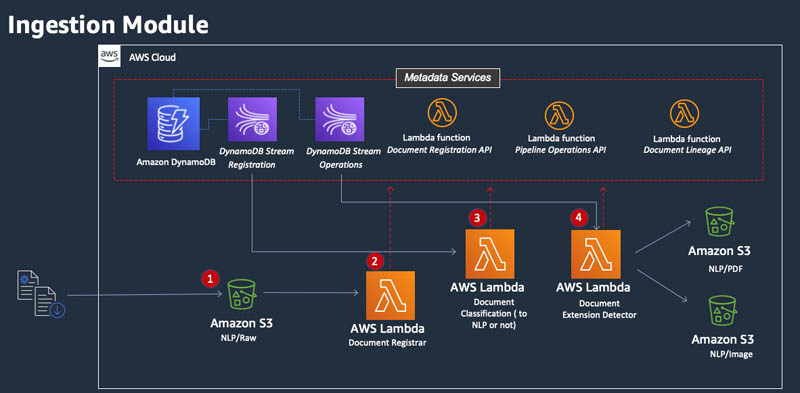
OCR module
This module detects the incoming file format and uses Amazon Textract in this implementation to convert the incoming documents into text. Amazon Textract can process image files synchronously, and PDF and other documents asynchronously, to allow time for the service to complete its analysis.
The OCR module consists of the following process, as illustrated in the architecture diagram:
- Image files are uploaded to the NLP/image S3 bucket and the Sync Processor Lambda function is invoked. The function synchronously points Amazon Textract to the S3 location of the image file, and waits for a response.
- Amazon Textract transforms the format to text and deposits the text output in the NLP/Textract. This step concludes OCR processing of the image file types.
- PDF files are placed within the NLP/PDF S3 bucket. This bucket invokes the Async Processor Lambda function. This function feeds the document to Amazon Textract and completes its state, registering as such with the metadata services.
- When the Amazon Textract document analysis is complete, an SNS message is sent to a specified SNS topic, notifying downstream consumers of the job completion. In this implementation, an SQS queue captures that message.
- The SQS queue message is the event that triggers the Result Processor Lambda function.
- The function extracts the results of document analysis from Amazon Textract and formats it according to the type of text it analyzed (forms, tables, and raw text).
- The results are pushed to the NLP/Textract S3 bucket, page by page for every type of text, and as a complete JSON response.
All the progress is registered in metadata services. The red dotted lines in the diagram represent the metadata asynchronous API calls.
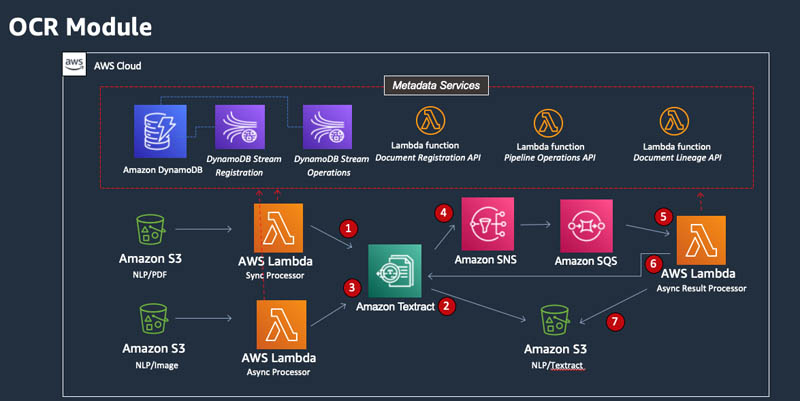
NLP module
This module detects key phrases and entities within the document by using the text output from the OCR module. A key phrase is a string containing a noun phrase that describes a particular thing. It generally consists of a noun and the modifiers that distinguish it. For example, “day” is a noun; “a beautiful day” is a noun phrase that includes an article (“a”) and an adjective (“beautiful”).
Once key phrases are understood, it’s quite likely that indexing them in an analytical tool would let you find this article quickly and accurately. For example, if you want to analyze corporate social responsibility (CSR) reports, you can find attributes such as “reducing carbon footprints,” “improving labor policies,” “participating in fair-trade,” and “charitable giving” by indexing results of this module.
We use Amazon Comprehend to perform this function in this pipeline. However, as we explained earlier, you can easily swap the tooling used for this design with your preferred tool. For example, you can replace Amazon Comprehend with an Amazon SageMaker custom model as an alternative to extract key phrases and entities in a more domain-focused way. SageMaker is an ML service that you can use to build, train, and deploy ML models for virtually any use case.
Amazon Comprehend is called on a synchronous basis to extract key phrases in the following steps (as illustrated in the following diagram):
- The incoming text file uploaded to the NLP/Textract S3 bucket invokes the Sync Comprehend Processor Lambda function.
- The function feeds the incoming file to Amazon Comprehend for processing.
- The results from Amazon Comprehend, in JSON format, are deposited in the NLP/JSON S3 bucket.
- The results from Amazon Comprehend are sent to Amazon ES, the service we incorporate as our document search engine.
All steps are being registered in metadata services. The red dotted lines in the diagram represent the metadata asynchronous API calls.
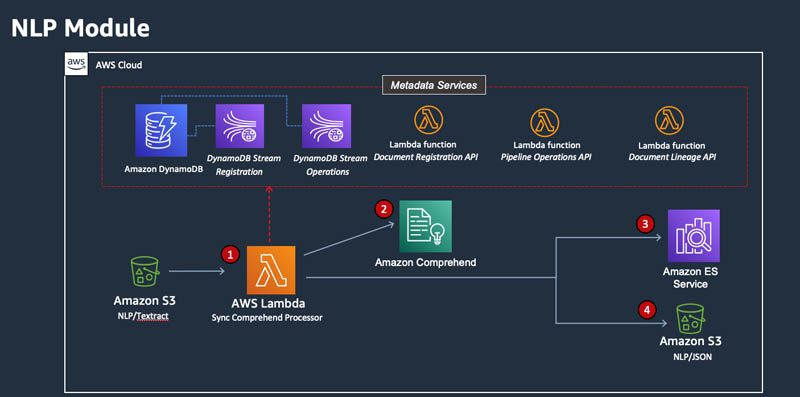
Analytics module
This module is responsible for the consumption and analytics segment of the pipeline. The steps are illustrated in the following diagram:
- The output from Amazon Comprehend, in JSON format, is fed to Amazon Neptune. Neptune allows end users to discover relationships across documents. This is an example of a downstream analytics application that is not implemented in this post.
- The end users have access to the original document in four formats (CSV, JSON, original, text), and can search key phrases using Amazon ES. They can identify relationships using Neptune. A JSON version of the document is available in the NLP/JSON S3 bucket. The original document is available in the NLP/Raw S3 bucket.
- Full lineage can be obtained from the Document Lineage table in DynamoDB.
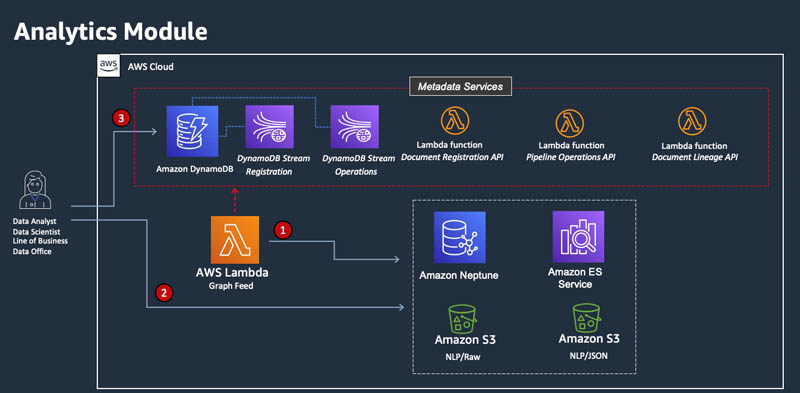
The analytics module has many potential implementations. For example, you can use a relational datastore like Amazon Relational Database (Amazon RDS) or Amazon Aurora to analyze extracted tabular data using SQL.
Conclusion
In this post, we architected an end-to-end document processing pipeline using AWS managed ML services. In addition, we introduced metadata services to help organizations create a centralized document repository to store documents one time but process multiple times. A data governance framework as illustrated in this design provides you with necessary guardrails to ensure documents are governed in a standard fashion across the organization, while providing lines of business with autonomy to decide your NLP and OCR models and choice of tooling.
The architecture discussed in this post has been coded and is available for deployment in the GitHub repo. You can download the code and create your pipeline within a few days.
About the Authors
David Kheyman is a Solutions Architect at Amazon Web Services based out of New York City, where he designs and implements repeatable AWS architecture patterns and solutions for large organizations.
Mojgan Ahmadi is a Principal Solutions Architect with Amazon Web Services based in New York, where she guides global financial services customers to build highly secure, scalable, reliable, and cost-efficient applications on the cloud. She brings over 20 years of technology experience on Software Development and Architecture, Data Governance and Engineering, and IT Management.
Anirudh Menon is a Solutions Architect with Amazon Web Services based in New York, where he helps financial services customers drive innovation with AWS solutions and industry-specific patterns.
Read More