Amazon Research Award recipient Yezhou Yang is studying how to make autonomous systems more robust.Read More
RECON: Learning to Explore the Real World with a Ground Robot
An example of our method deployed on a Clearpath Jackal ground robot (left) exploring a suburban environment to find a visual target (inset). (Right) Egocentric observations of the robot.
Imagine you’re in an unfamiliar neighborhood with no house numbers and I give you a photo that I took a few days ago of my house, which is not too far away. If you tried to find my house, you might follow the streets and go around the block looking for it. You might take a few wrong turns at first, but eventually you would locate my house. In the process, you would end up with a mental map of my neighborhood. The next time you’re visiting, you will likely be able to navigate to my house right away, without taking any wrong turns.
Such exploration and navigation behavior is easy for humans. What would it take for a robotic learning algorithm to enable this kind of intuitive navigation capability? To build a robot capable of exploring and navigating like this, we need to learn from diverse prior datasets in the real world. While it’s possible to collect a large amount of data from demonstrations, or even with randomized exploration, learning meaningful exploration and navigation behavior from this data can be challenging – the robot needs to generalize to unseen neighborhoods, recognize visual and dynamical similarities across scenes, and learn a representation of visual observations that is robust to distractors like weather conditions and obstacles. Since such factors can be hard to model and transfer from simulated environments, we tackle these problems by teaching the robot to explore using only real-world data.
3 Questions: Blending computing with other disciplines at MIT
The demand for computing-related training is at an all-time high. At MIT, there has been a remarkable tide of interest in computer science programs, with heavy enrollment from students studying everything from economics to life sciences eager to learn how computational techniques and methodologies can be used and applied within their primary field.
Launched in 2020, the Common Ground for Computing Education was created through the MIT Stephen A. Schwarzman College of Computing to meet the growing need for enhanced curricula that connect computer science and artificial intelligence with different domains. In order to advance this mission, the Common Ground is bringing experts across MIT together and facilitating collaborations among multiple departments to develop new classes and approaches that blend computing topics with other disciplines.
Dan Huttenlocher, dean of the MIT Schwarzman College of Computing, and the chairs of the Common Ground Standing Committee — Jeff Grossman, head of the Department of Materials Science and Engineering and the Morton and Claire Goulder and Family Professor of Environmental Systems; and Asu Ozdaglar, deputy dean of academics for the MIT Schwarzman College of Computing, head of the Department of Electrical Engineering and Computer Science, and the MathWorks Professor of Electrical Engineering and Computer Science — discuss here the objectives of the Common Ground, pilot subjects that are underway, and ways they’re engaging faculty to create new curricula for MIT’s class of “computing bilinguals.”
Q: What are the objectives of the Common Ground and how does it fit into the mission of the MIT Schwarzman College of Computing?
Huttenlocher: One of the core components of the college mission is to educate students who are fluent in both the “language” of computing and that of other disciplines. Machine learning classes, for example, attract a lot of students outside of electrical engineering and computer science (EECS) majors. These students are interested in machine learning for modeling within the context of their fields of interest, rather than inner workings of machine learning itself as taught in Course 6. So, we need new approaches to how we develop computing curricula in order to provide students with a thorough grounding in computing that is relevant to their interests, to not just enable them to use computational tools, but understand conceptually how they can be developed and applied in their primary field, whether it be science, engineering, humanities, business, or design.
The core goals of the Common Ground are to infuse computing education throughout MIT in a coordinated manner, as well as to serve as a platform for multi-departmental collaborations. All classes and curricula developed through the Common Ground are intended to be created and offered jointly by multiple academic departments to meet ‘common’ needs. We’re bringing the forefront of rapidly-changing computer science and artificial intelligence fields together with the problems and methods of other disciplines, so the process has to be collaborative. As much as computing is changing thinking in the disciplines, the disciplines are changing the way people develop new computing approaches. It can’t be a stand-alone effort — otherwise it won’t work.
Q: How is the Common Ground facilitating collaborations and engaging faculty across MIT to develop new curricula?
Grossman: The Common Ground Standing Committee was formed to oversee the activities of the Common Ground and is charged with evaluating how best to support and advance program objectives. There are 29 members on the committee — all are faculty experts in various computing areas, and they represent 18 academic departments across all five MIT schools and the college. The structure of the committee very much aligns with the mission of the Common Ground in that it draws from all parts of the Institute. Members are organized into subcommittees currently centered on three primary focus areas: fundamentals of computational science and engineering; fundamentals of programming/computational thinking; and machine learning, data science, and algorithms. The subcommittees, with extensive input from departments, framed prototypes for what Common Ground subjects would look like in each area, and a number of classes have already been piloted to date.
It has been wonderful working with colleagues from different departments. The level of commitment that everyone on the committee has put into this effort has truly been amazing to see, and I share their enthusiasm for pursuing opportunities in computing education.
Q: Can you tell us more about the subjects that are already underway?
Ozdaglar: So far, we have four offerings for students to choose from: in the fall, there’s Linear Algebra and Optimization with the Department of Mathematics and EECS, and Programming Skills and Computational Thinking in-Context with the Experimental Study Group and EECS; Modeling with Machine Learning: From Algorithms to Applications in the spring, with disciplinary modules developed by multiple engineering departments and MIT Supply Chain Management; and Introduction to Computational Science and Engineering during both semesters, which is a collaboration between the Department of Aeronautics and Astronautics and the Department of Mathematics.
We have had students from a range of majors take these classes, including mechanical engineering, physics, chemical engineering, economics, and management, among others. The response has been very positive. It is very exciting to see MIT students having access to these unique offerings. Our goal is to enable them to frame disciplinary problems using a rich computational framework, which is one of the objectives of the Common Ground.
We are planning to expand Common Ground offerings in the years to come and welcome ideas for new subjects. Some ideas that we currently have in the works include classes on causal inference, creative programming, and data visualization with communication. In addition, this fall, we put out a call for proposals to develop new subjects. We invited instructors from all across the campus to submit ideas for pilot computing classes that are useful across a range of areas and support the educational mission of individual departments. The selected proposals will receive seed funding from the Common Ground to assist in the design, development, and staffing of new, broadly-applicable computing subjects and revision of existing subjects in alignment with the Common Ground’s objectives. We are looking explicitly to facilitate opportunities in which multiple departments would benefit from coordinated teaching.
AWS team wins best-paper award for work on automated reasoning
SOSP paper describes lightweight formal methods for validating new S3 data storage service.Read More
Reducing city transport emissions with Maps and AI
City transportation is crucial to connecting residents to education, employment and essential services. At the same time, the transportation sector is where global greenhouse gas (GHG) emissions are rising the quickest.
In 2018, we launched the Environmental Insights Explorer (EIE) in collaboration with the Global Covenant of Mayors for Climate & Energy (GCoM). As part of Google’s most ambitious decade of climate action, we’ve committed to helping more than 500 cities and local governments reduce an aggregate of 1 gigaton of carbon emissions per year by 2030 and beyond. With EIE, cities have free access to Google’s unique mapping data and insights so they can make sustainable decisions regarding cleaner transport policies and infrastructure programs. Since launching EIE, we’ve seen more cities and governments set ambitious climate targets. This week, 120 world governments will gather in Glasgow at COP26 to report their progress toward these commitments and set a path forward to address climate change. EIE can help cities and governments translate these targets into concrete action.
In pursuit of helping more cities take action against climate change, we will make transportation insights available in EIE for over 20,000 cities and regional governments by the end of the year, making it one of the largest ever collections of high-quality, globally consistent environmental data sources. This expansion will double the number of geographies represented in EIE, accounting for the majority of the world’s transport emissions.
As the window continues to narrow on implementing policies and plans to reduce emissions, we’re unifying around a single mission: to foster sustainability at scale. To help, we’re partnering with the C40 Cities Climate Leadership Group, a network of megacities committed to addressing climate change. Our work with C40 will help us better support the needs of cities while making data accessible to city projects that are working on climate solutions. Together we can provide higher-quality transportation activity data to measure and track GHG emissions at a global scale, while also giving state and local governments resources to better understand what’s working at a local level.
The need for action is now, and we need to rise to the challenge quickly. Google technology is unlocking our ability to generate climate-related insights and impact at a global scale. Here are a few of the latest ways we’re using AI and Google Maps data in EIE.
Taking inventory of yearly progress
More cities, states and regions are committing to comprehensive climate plans to decarbonize transportation by 2040. These next two decades of ambitious action will require regular progress reports to assess what is and isn’t working.
Using AI, our systems analyze transportation trends in a city by mode, helping local governments take stock of their progress in tackling GHG emissions. GHG inventory processes traditionally take months and multiple data sources to compile, and are now streamlined, allowing government staff to reduce the cost and personnel burden of reporting.
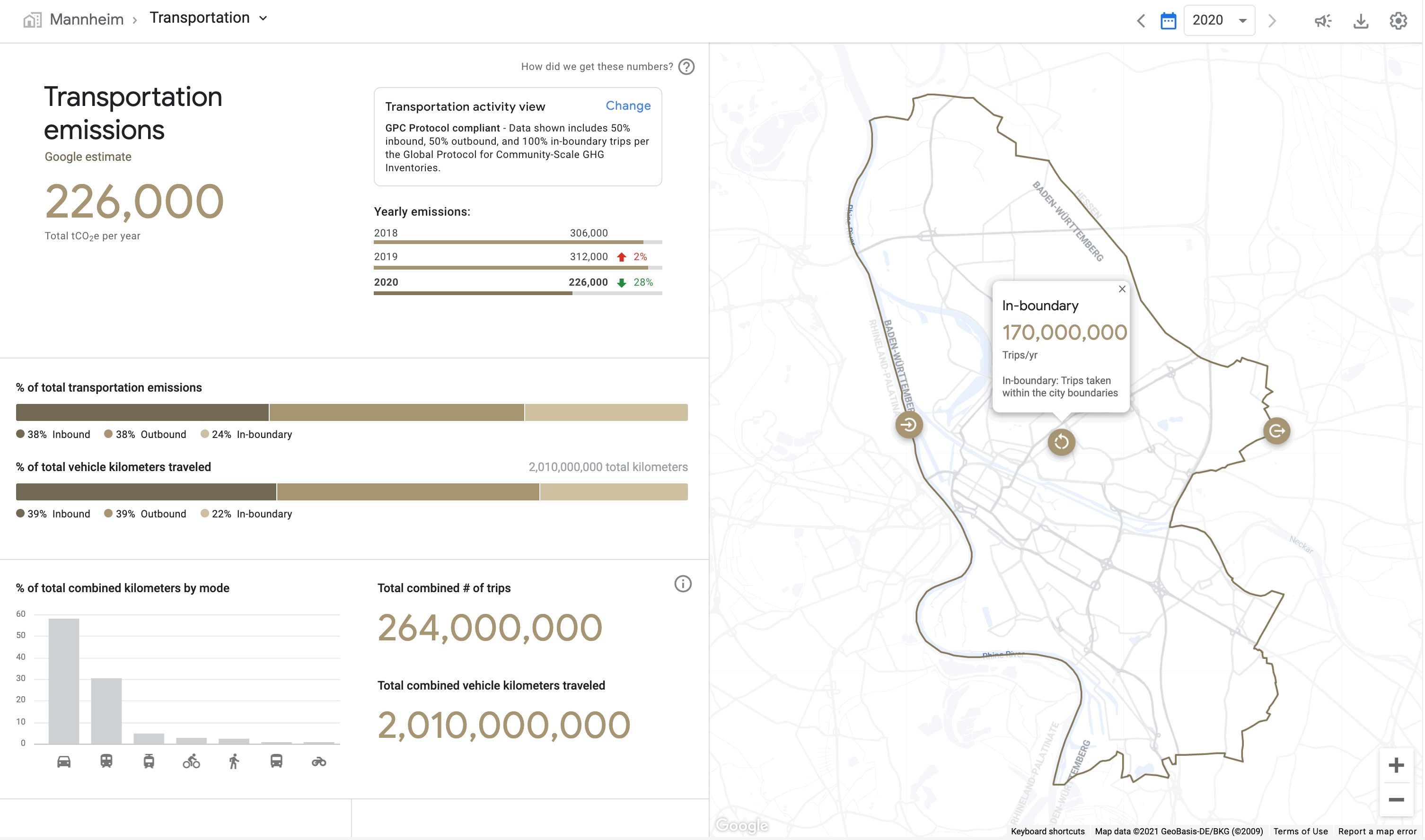
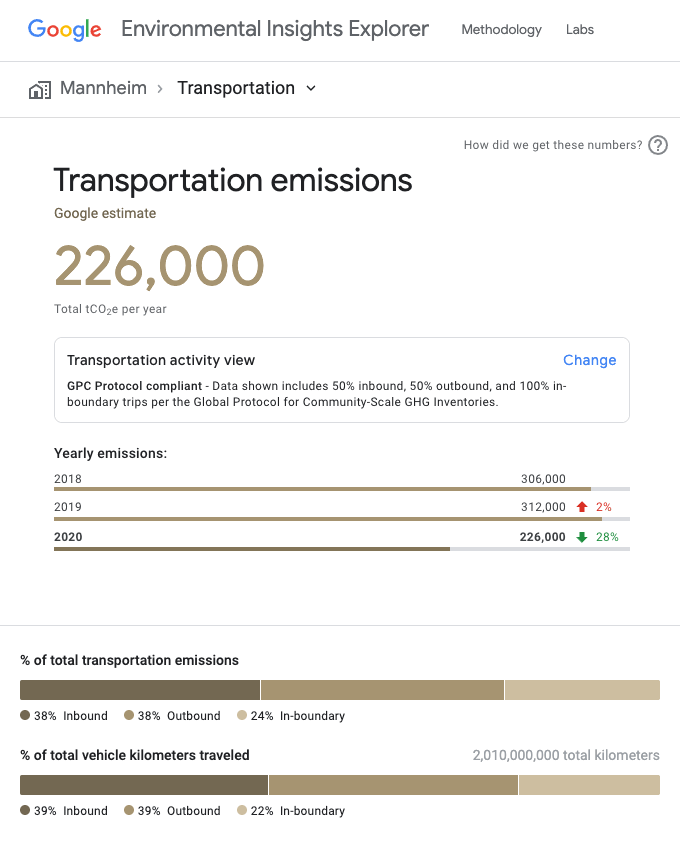
Plan eco-friendly mobility interventions
To accelerate data-driven decisions aimed at reducing transportation emissions and boosting infrastructure investments, EIE summarizes critical insights across which modes of transport to tackle. EIE characterizes trips traveled within and across city boundaries and applies city-specific scaling factors based on overall population. EIE’s multimodal insights allow government data practitioners to ask questions that inform transportation decisions, such as the extent to which city investments in different modes of transportation can shift behaviors.
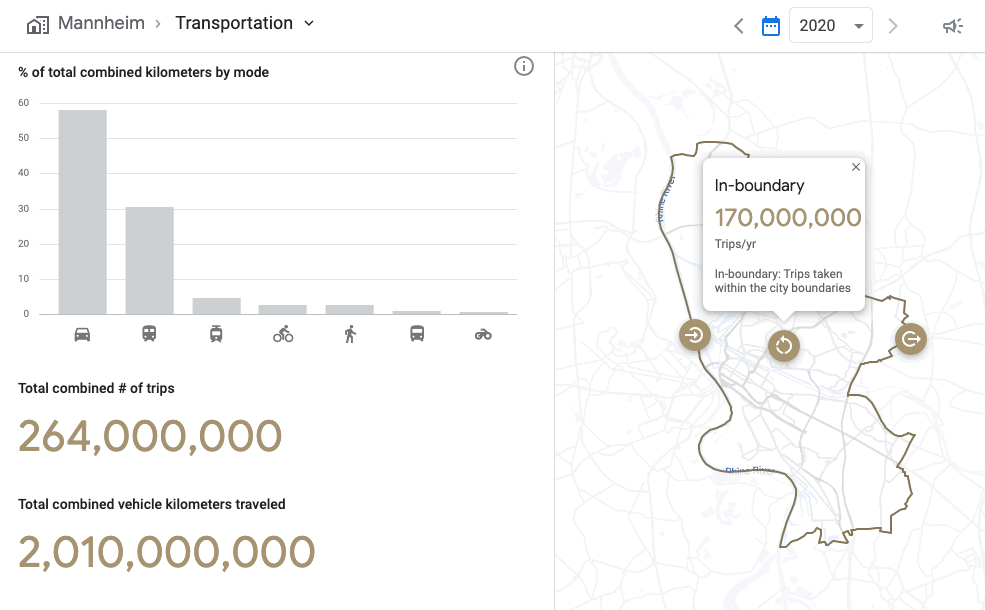
Looking forward
Modeling transportation flows is complex. With EIE, cities, states, regional policymakers, consultants can better understand the impact sustainable changes are making on global greenhouse gas emissions.
To learn more about how cities are using EIE, view our EIE 2021 City Impact report. If you’re part of a local government and interested in what EIE can do for your community, fill out this form to get in touch with our team.
Real-world challenges for AGI
When people picture a world with artificial general intelligence (AGI), robots are more likely to come to mind than enabling solutions to society’s most intractable problems. But I believe the latter is much closer to the truth. AI is already enabling huge leaps in tackling fundamental challenges: from solving protein folding to predicting accurate weather patterns, scientists are increasingly using AI to deduce the rules and principles that underpin highly complex real-world domains – ones they might never have discovered unaided. Advances in AGI research will supercharge society’s ability to tackle and manage climate change – not least because of its urgency but also due to its complex and multifaceted nature.Read More
Real-World Challenges for AGI
Koray Kavukcuoglu, VP of Research, discusses why addressing real-world challenges now helps advance the development of true AGI.Read More
Amazon SCOT employees participate in innovation and diversity panel discussion
Watch the replay of the October 28 discussion featuring four Amazon leaders.Read More