Logz.io is an AWS Partner Network (APN) Advanced Technology Partner with AWS Competencies in DevOps, Security, and Data & Analytics. Logz.io offers a software as a service (SaaS) observability platform based on best-in-class open-source software solutions for log, metric, and tracing analytics. Customers are sending an increasing amount of data to Logz.io from various data sources to manage the health and performance of their applications and services. It can be overwhelming for new users who are looking to navigate across the various dashboards built over time, process different alert notifications, and connect the dots when troubleshooting production issues.
Mean time to detect (MTTD) and mean time to resolution (MTTR) are key metrics for our customers. They’re calculated by measuring the time a user in our platform starts to investigate an issue (such as production service down) to the point when they stop doing actions in the platform that are related to the specific investigation.
To help customers reduce MTTD and MTTR, Logz.io is turning to machine learning (ML) to provide recommendations for relevant dashboards and queries and perform anomaly detection via self-learning. As a result, the average user is equipped with the aggregated experience of their entire company, leveraging the wisdom of many. We found that our solution can reduce MTTR by up to 20%.
As MTTD decreases, users can identify the problem and resolve it faster. Our data semantic layer contains semantics for starting and stopping an investigation, and the popularity of each action the user is doing with respect to a specific alert.
In this post, we share how Logz.io used Amazon SageMaker to reduce the time and effort for our proof of concept (POC), experiments from research to production evaluation, and how we reduced our production inference cost.
The challenge
Until Logz.io used SageMaker, the time between research to POC testing and experiments on production was quite lengthy. This was because we needed to create Spark jobs to collect, clean, and normalize the data. DevOps required this work to read each data source. DevOps and data engineering skills aren’t part of our ML team, and this caused a high dependency between the teams.
Another challenge was to provide an ML inference service to our products while achieving optimal cost vs. performance ratio. Our optimal scenario is supporting as many models as possible for a computing unit, while providing high concurrency from customers with many models. We had flexibility on our inference time, because our initial window of the data stream for the inference service is 5 minutes bucket of logs.
Research phase
Data science is an iterative process that requires an interactive development environment for research, validating the data output on every iteration and data processing. Therefore, we encourage our ML researchers to use notebooks.
To accelerate the iteration cycle, we wanted to test our notebooks’ code on real production data, while running it at scale. Furthermore, we wanted to avoid the bottleneck of DevOps and data engineering during the initial test in production, while having the ability to view the outputs and trying to estimate the code runtime.
To implement this, we wanted to provide our data science team full control and end-to-end responsibility from research to initial test on production. We needed them to easily pull data, while preserving data access management and monitoring this access. They also needed to easily deploy their custom POC notebooks into production in a scalable manner, while monitoring the runtime and expected costs.
Evaluation phase
During this phase, we evaluated a few ML platforms in order to support both training and serving requirements. We found that SageMaker is the most appropriate for our use cases because it supports both training and inference. Furthermore, it’s customizable, so we can tailor it according to our preferred research process.
Initially, we started from local notebooks, testing various libraries. We ran into problems with pulling massive data from production. Later, we were stuck in a point of the modeling phase that took many hours on a local machine.
We evaluated many solutions and finally chose the following architecture:
-
DataPlate – The open-source version of DataPlate helped us pull and join our data easily by utilizing our Spark Amazon EMR clusters with a simple SQL, while monitoring the data access
-
SageMaker notebook instance and processing jobs – This helped us with the scalability of runtime and flexibility of machine types and ML frameworks, while collaborating our code via a Git connection
Research phase solution architecture
The following diagram illustrates the solution architecture of the research phase, and consists of the following components:
-
SageMaker notebooks – Data scientists use these notebooks to conduct their research.
-
AWS Lambda function – AWS Lambda is a serverless solution that runs a processing job on demand. The job uses a Docker container with the notebook we want to run during our experiment, together with all our common files that need to support the notebook (
requirements.txt
and the multi-processing functions code in a separate notebook).
-
Amazon ECR – Amazon Elastic Container Registry (Amazon ECR) stores our Docker container.
-
SageMaker Processing job – We can run this data processing job on any ML machine, and it runs our notebook with parameters.
-
DataPlate – This service helps us use SQL and join several data sources easily. It translates it to Spark code and optimizes it, while monitoring data access and helping reduce data breaches. The Xtra version provided even more capabilities.
-
Amazon EMR – This service runs our data extractions as workloads over Spark, contacting all our data resources.
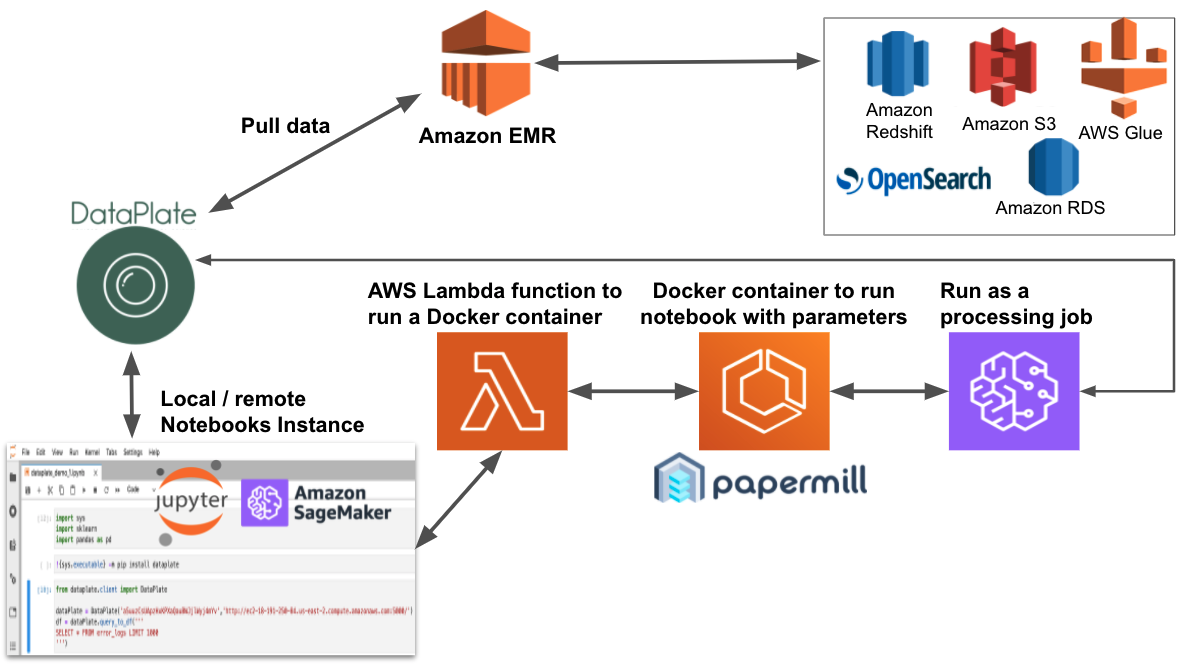
With the SageMaker notebook instance lifecycle, we can control the maximum notebook instance runtime, using the autostop.py
template script.
After testing the ML frameworks, we chose the SageMaker MXNet kernel for our clustering and ranking phases.
To test the notebook code on our production data, we ran the notebook by encapsulating it via Docker in Amazon ECS and ran it as a processing job to validate the maximum runtime on different types of machines.
The Docker container also helps us share resources among notebooks’ tests. In some cases, a notebook calls other notebooks to utilize a multi-process by splitting big data frames into smaller data frames, which can run simultaneously on each vCPU in a large machine type.
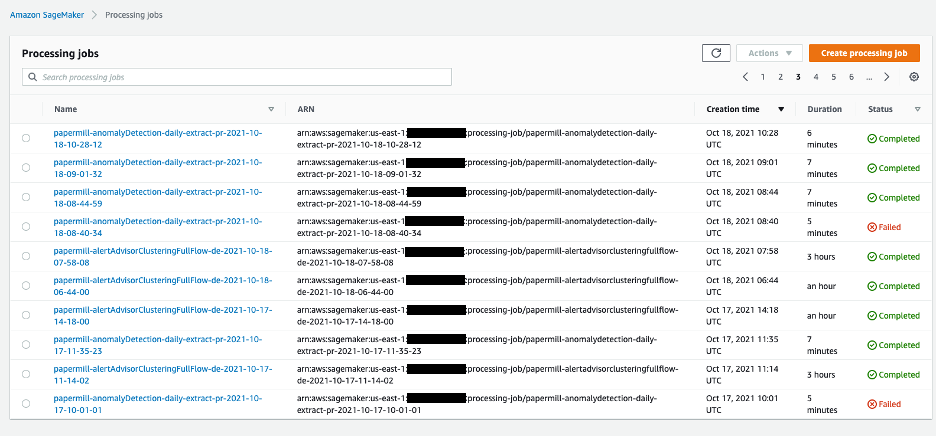
The real-time production inference solution
In the research phase, we used Parquet Amazon Simple Storage Service (Amazon S3) files to maintain our recommendations. These are consumed once a day from our engineering pipeline to attach the recommendations to our alerts’ mechanism.
However, our roadmap requires a higher refresh rate solution and pulling once a day isn’t enough in the long term, because we want to provide recommendations even during the investigation.
To implement this solution at scale, we tested most of the SageMaker endpoint solutions in our anomaly-detection research. We tested 500 of the pre-built models with a single endpoint machine of various types and used concurrent multi-threaded clients to perform requests to the endpoint. We measured the response time, CPU, memory, and other metrics (for more information, see Monitor Amazon SageMaker with Amazon CloudWatch). We found that the multi-model endpoint is a perfect fit for our use cases.
A multi-model endpoint can reduce our costs dramatically in comparison to a single endpoint or even Kubernetes to use Flask (or other Python) web services. Our first assumption was that we must provide a single endpoint, using a 4-vCPU small machine, for each customer, and on average query four dedicated models, because each vCPU serves one model. With the multi-model endpoint, we could aggregate more customers on a single multi-endpoint machine.
We had a model and encoding files per customer, and after doing load tests, we determined that we could serve 50 customers, each using 10 models and even using the smallest ml.t2.medium instance for our solutions.
In this stage, we considered using multi-model endpoints. Multi-model endpoints provide a scalable and cost-effective solution to deploy a large number of models, enabling you to host multiple models with a single inference container. This reduces hosting costs by improving endpoint utilization compared to using multiple small single-model endpoints that each serve a single customer. It also reduces deployment overhead because SageMaker manages loading models in memory and scaling them based on the traffic patterns to them.
Furthermore, the multi-model endpoint advantage is that if you have a high inference rate from specific customers, its framework preserves the last serving models in memory for better performance.
After we estimated costs using multi-model endpoints vs. standard endpoints, we found out that it could potentially lead to cost reduction of approximately 80%.
The outcome
In this section, we review the steps and the outcome of the process.
We use the lifecycle notebook configuration to enable running the notebooks as processing jobs, by encapsulating the notebook in a Docker container in order to validate the code faster and use the autostop mechanism:
#!/bin/bash
# Copyright Amazon.com, Inc. or its affiliates. All Rights Reserved.
#
# Licensed under the Apache License, Version 2.0 (the "License"). You
# may not use this file except in compliance with the License. A copy of
# the License is located at
#
# http://aws.amazon.com/apache2.0/
#
# or in the "license" file accompanying this file. This file is
# distributed on an "AS IS" BASIS, WITHOUT WARRANTIES OR CONDITIONS OF
# ANY KIND, either express or implied. See the License for the specific
# language governing permissions and limitations under the License.
set -e
# OVERVIEW
# This script installs the sagemaker_run_notebook extension package in SageMaker Notebook Instance
#
# There are two parameters you need to set:
# 1. S3_LOCATION is the place in S3 where you put the extension tarball
# 2. TARBALL is the name of the tar file that you uploaded to S3. You should just need to check
# that you have the version right.
sudo -u ec2-user -i <<'EOF'
# PARAMETERS
VERSION=0.18.0
EXTENSION_NAME=sagemaker_run_notebook
# Set up the user setting and workspace directories
mkdir -p /home/ec2-user/SageMaker/.jupyter-user/{workspaces,user-settings}
# Run in the conda environment that the Jupyter server uses so that our changes are picked up
source /home/ec2-user/anaconda3/bin/activate JupyterSystemEnv
# Install the extension and rebuild JupyterLab so it picks up the new UI
aws s3 cp s3://aws-emr-resources-11111111-us-east-1/infra-sagemaker/sagemaker_run_notebook-0.18.0-Logz-latest.tar.gz ./sagemaker_run_notebook-0.18.0-Logz-latest.tar.gz
pip install sagemaker_run_notebook-0.18.0-Logz-latest.tar.gz
jupyter lab build
source /home/ec2-user/anaconda3/bin/deactivate
EOF
# sudo -u ec2-user -i <<'EOF'
# PARAMETERS
for PACKAGE in pandas dataplate awswrangler==2.0.0 ipynb==0.5.1 prison==0.1.3 PyMySQL==0.10.1 requests==2.25.0 scipy==1.5.4 dtaidistance joblib sagemaker_run_notebook-0.18.0-Logz-latest.tar.gz fuzzywuzzy==0.18.0; do
echo $PACKAGE
# Note that "base" is special environment name, include it there as well.
for env in base /home/ec2-user/anaconda3/envs/*; do
source /home/ec2-user/anaconda3/bin/activate $(basename "$env")
if [ $env = 'JupyterSystemEnv' ]; then
continue
fi
pip install --upgrade "$PACKAGE"
source /home/ec2-user/anaconda3/bin/deactivate
done
done
jupyter lab build
# Tell Jupyter to use the user-settings and workspaces directory on the EBS
# volume.
echo "export JUPYTERLAB_SETTINGS_DIR=/home/ec2-user/SageMaker/.jupyter-user/user-settings" >> /etc/profile.d/jupyter-env.sh
echo "export JUPYTERLAB_WORKSPACES_DIR=/home/ec2-user/SageMaker/.jupyter-user/workspaces" >> /etc/profile.d/jupyter-env.sh
# The Jupyter server needs to be restarted to pick up the server part of the
# extension. This needs to be done as root.
initctl restart jupyter-server --no-wait
# OVERVIEW
# This script stops a SageMaker notebook once it's idle for more than 2 hour (default time)
# You can change the idle time for stop using the environment variable below.
# If you want the notebook the stop only if no browsers are open, remove the --ignore-connections flag
#
# Note that this script will fail if either condition is not met
# 1. Ensure the Notebook Instance has internet connectivity to fetch the example config
# 2. Ensure the Notebook Instance execution role permissions to SageMaker:StopNotebookInstance to stop the notebook
# and SageMaker:DescribeNotebookInstance to describe the notebook.
# PARAMETERS
IDLE_TIME=3600
echo "Fetching the autostop script"
wget https://raw.githubusercontent.com/aws-samples/amazon-sagemaker-notebook-instance-lifecycle-config-samples/master/scripts/auto-stop-idle/autostop.py
echo "Starting the SageMaker autostop script in cron"
(crontab -l 2>/dev/null; echo "*/5 * * * * /usr/bin/python $PWD/autostop.py --time $IDLE_TIME --ignore-connections") | crontab -
We clone the sagemaker-run-notebook GitHub project, and add the following to the container:
- Our pip requirements
- The ability to run notebooks from within a notebook, which enables us multi-processing behavior to utilize all the ml.m5.12xlarge instance cores
This enables us to run workflows that consist of many notebooks running as processing jobs in a line of code, while defining the instance type to run on.

Because we can add parameters to the notebook, we can scale our processing by running simultaneously at different hours, days, or months to pull and process data.
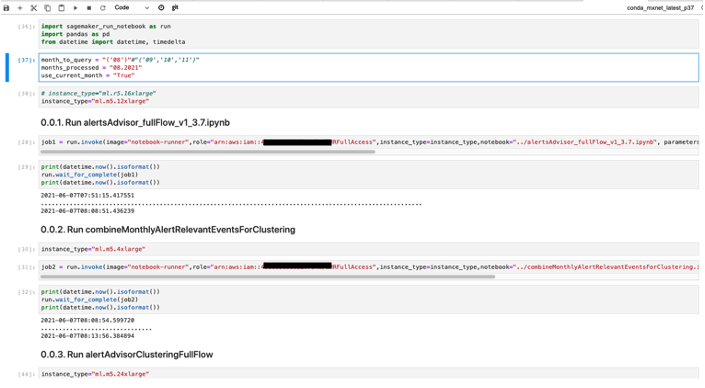
We can also create scheduling jobs that run notebooks (and even limit the run time).
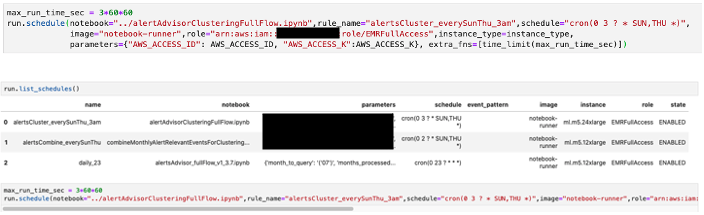
We also can observe the last runs and their details, such as processing time.
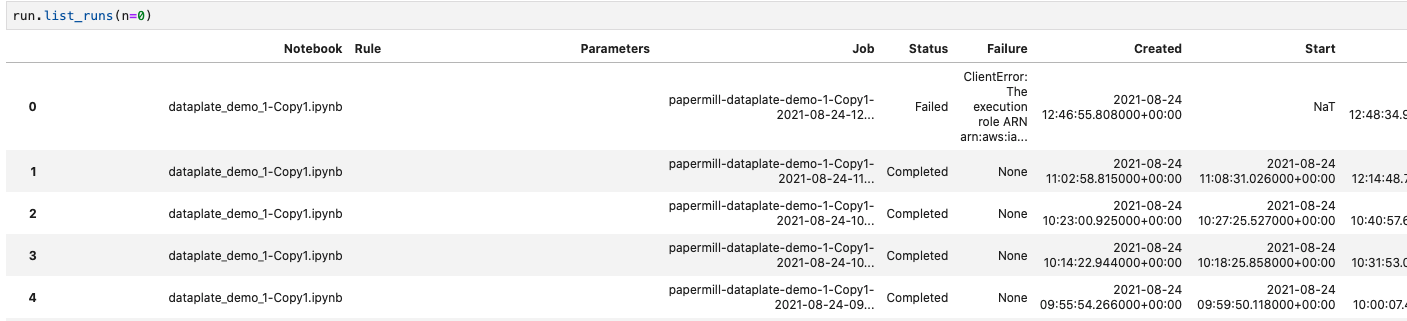
With the papermill that is used in the container, we can view the output of every run, which helps us debug in production.

Our notebook output review is in the form of a standard read-only notebook.
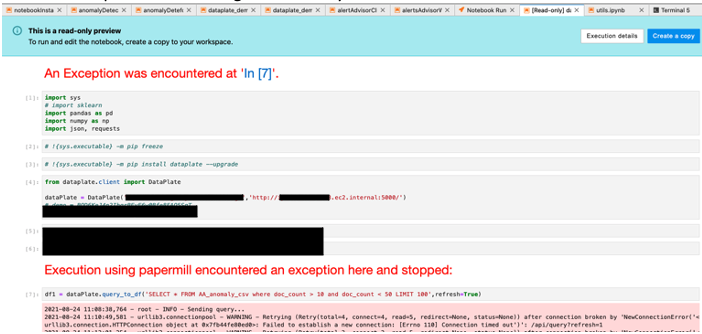
Multi-processing utilization helps us scale on each notebook processing and utilize all its cores. We generated functions in other notebooks that can do heavy processing, such as the following:
- Explode JSONs
- Find relevant rows in a DataFrame while the main notebook splits the DataFrame in
#cpu-cores
elements
- Run clustering per alert type actions simultaneously
We then add these functional notebooks into the container that runs the notebook as a processing job. See the following Docker file (notice the COPY commands):
ARG BASE_IMAGE=need_an_image
FROM $BASE_IMAGE
ENV JUPYTER_ENABLE_LAB yes
ENV PYTHONUNBUFFERED TRUE
COPY requirements.txt /tmp/requirements.txt
RUN pip install papermill jupyter nteract-scrapbook boto3 requests==2.20.1
RUN pip install -r /tmp/requirements.txt
ENV PYTHONUNBUFFERED=TRUE
ENV PATH="/opt/program:${PATH}"
# Set up the program in the image
COPY multiprocessDownloadNormalizeFunctions.ipynb /tmp/multiprocessDownloadNormalizeFunctions.ipynb
COPY multiprocessFunctions.ipynb /tmp/multiprocessFunctions.ipynb
COPY run_notebook execute.py /opt/program/
ENTRYPOINT ["/bin/bash"]
# because there is a bug where you have to be root to access the directories
USER root
Results
During the research phase, we evaluated the option to run our notebooks as is to experiment and evaluate how our code performs on all our relevant data, not just a sample of data. We found that encapsulating our notebooks using processing jobs can be a great fit for us, because we don’t need to rewrite code and we can utilize the power of AWS compute optimized and memory optimized instances and follow the status of the process easily.
During the inference assessment, we evaluated various SageMaker endpoint solutions. We found that using a multi-model endpoint can help us serve approximately 50 customers, each having multiple (approximately 10) models in a single instance, which can meet our low-latency constraints, and therefore save us up to 80% of the cost.
With this solution architecture, we were able to reduce the MTTR of our customers, which is a main metric for measuring success using our platform. It reduces the total time from the point of responding to our alert link, which describes an issue in your systems, to when you’re done investigating the problem using our platform. During the investigation phase, we measure the users’ actions with and without our ML recommendation solution. This helps us provide recommendations for the best action to resolve the specific issue faster and pinpoint anomalies to identify the actual cause of the problem.
Conclusion and next steps
In this post, we shared how Logz.io used SageMaker to improve MTTD and MTTR.
As a next step, we’re considering expanding the solution with the following features:
We encourage you to try out SageMaker notebooks. For more examples, check out the SageMaker examples GitHub repo.
About the Authors
Amit Gross is leading the Research department of Logz.io, which is responsible for the AI solutions of all Logz.io products, from the research phase to the integration phase. Prior to Logz.io Amit has managed both Data Science and Security Research Groups at Here inc. and Cellebrite inc. Amit has M.Sc in computer science from Tel-Aviv University.
Yaniv Vaknin is a Machine Learning Specialist at Amazon Web Services. Prior to AWS, Yaniv held leadership positions with AI startups and Enterprise including co-founder and CEO of Dipsee.ai. Yaniv works with AWS customers to harness the power of Machine Learning to solve real world tasks and derive value. In his spare time, Yaniv enjoys playing soccer with his boys.
Eitan Sela is a Machine Learning Specialist Solutions Architect with Amazon Web Services. He works with AWS customers to provide guidance and technical assistance, helping them build and operate machine learning solutions on AWS. In his spare time, Eitan enjoys jogging and reading the latest machine learning articles.
Read More