Editor’s note: This post is part of our weekly In the NVIDIA Studio series, which celebrates featured artists, offers creative tips and tricks, and demonstrates how NVIDIA Studio technology accelerates creative workflows.
Creativity heats up In the NVIDIA Studio as the July NVIDIA Studio Driver, available now, accelerates the recent Chaos V-Ray 6 for 3ds Max release.
Plus, this week’s In the NVIDIA Studio 3D artist, Brian Lai, showcases his development process for Afternoon Coffee and Waffle, a piece that went from concept to completion faster with NVIDIA RTX acceleration in Chaos V-Ray rendering software.
Chaos V-Ray Powered by NVIDIA Studio Offers a July to Remember
Visualization and computer graphics software company Chaos this month released an update to the all-in-one photorealistic rendering software, V-Ray 6 for 3ds Max. The July Studio Driver gives creators on NVIDIA GPUs the best experience.
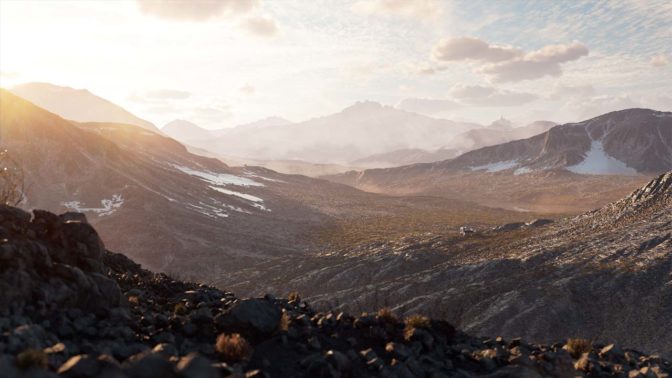
This release is a major upgrade that gives artists powerful new world-building and workflow tools to quickly distribute 3D objects, generate detailed 3D surfaces and add procedural clouds to create beautiful custom skies.
V-Ray GPU software — which already benefits from high-performance final-frame rendering with RTX-accelerated ray tracing and accelerated interactive rendering with AI-powered denoising — gets a performance bump, in addition to the new features.
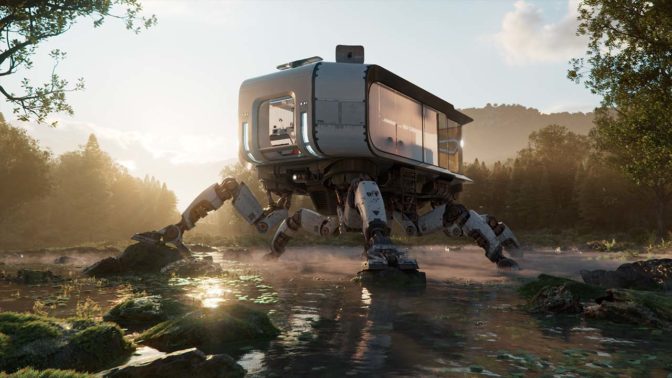
3D artists benefit from speedups in multiple ways with V-Ray 6. GPU improvements include support for nearly all new V-Ray 6 features, and enable faster Light Cache and a new Device Selector to assign rendering devices to tasks. By allowing users to specify use of the GPU for the AI denoiser, rendering performance is nearly doubled.
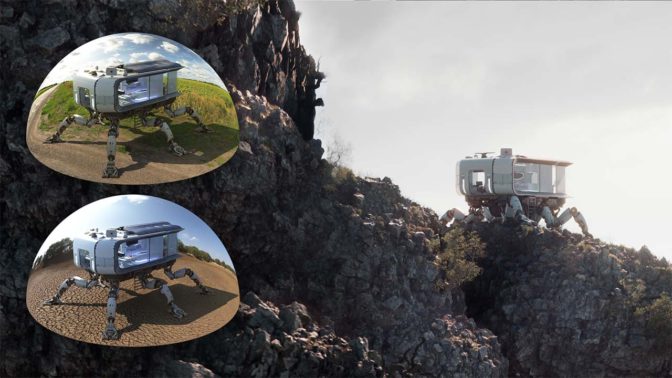
Additional key new features include:
- Chaos Scatter — easily populate scenes with millions of 3D objects to produce natural-looking landscapes and environments without adjusting objects by hand.
- Procedural Clouds — simulate a variety of cloud types and weather conditions, from partly cloudy to overcast.
- Improved trace-depth workflow — simplifies the setting of trace-depth overrides for reflections and refractions.
- Shading improvements — includes new energy-preserving GGX shader, thin film rollout in the V-Ray material for bubbles and fabrics, and improved V-Ray dirt functionality.
And there’s much more to explore. Creators can join a free V-Ray 6 for 3ds Max webinar on Thursday, July 28, to see how the new features are already helping 3D artists create fantastic visuals.
Sweet, Rendery Waffles
This week In the NVIDIA Studio, Brian Lai, a computer graphics craftsman, details the sweet, buttery-smooth creative process for Afternoon Coffee and Waffle.
Lai enjoyed the sweet satisfaction of creating with a GeForce RTX 3090 GPU and NVIDIA Studio benefits.
Lai’s journey into 3D art started at an early age, inspired by his photographer father — who Lai worked for until getting accepted into Malaysia’s top art college, The One Academy. He finds inspiration in real-world environments, observing what’s around him.
“I was always obsessed with optics and materials, so I just look at things around me, and then I want to replicate them into 3D form,” Lai said. “It’s satisfying to recreate a thing or environment to confuse my audience about whether it’s a real or 3D rendered image.”
It’s no wonder that Afternoon Coffee and Waffle takes on a picturesque quality, as typically exhibited by a social media image showing off a food adventure.
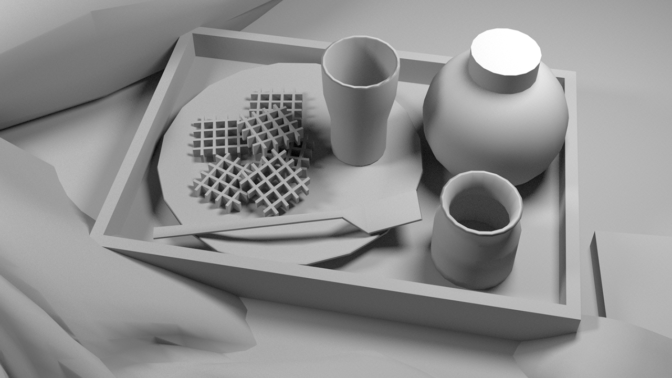
After finding a reference shot online, Lai started his creative process with basic 3D model blocking, which helped him set a direction for the final image, as well as find a good focal length and position for the camera. Then, he finalized each 3D model, turning them into high-resolution models and texturing each separately. This allowed him to focus on the props’ details.
Lai called his experience creating with a GeForce RTX 3090 “butter smooth throughout the process.”
RTX-accelerated ray tracing happens lightning quick in V-Ray GPU with AI-powered denoising. Lai prefers V-Ray “mainly because it utilizes the power from RT and Tensor Cores on the graphics card,” he said. “I don’t really feel limited by the software.”
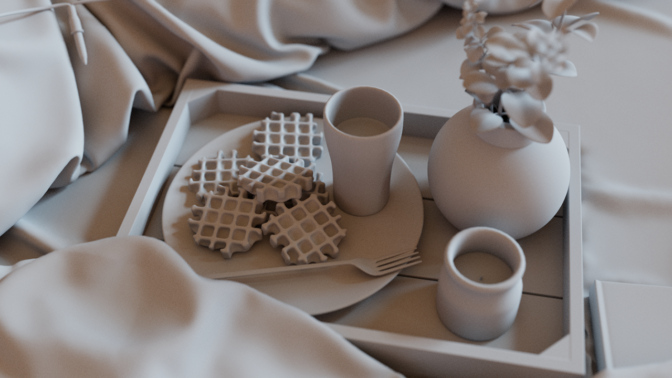
The artist also used his GPU for simulation in Autodesk Maya to get the best-looking fabrics possible, noting that “texturing in 4K preview is so satisfying.” He then finalized the models with normal map folds in Adobe Substance Painter.
Once all the models were ready, Lai gathered everything into one scene. In the above tutorial, he shows how each shader was constructed in Autodesk Maya’s Hypershade window.
The very last — and creatively infinite — step was to look dev. Here, Lai had to self-contain his tinkering as he “can do endless improvement in this stage,” he said. Ultimately, he reached the extent of realism he was aiming for and called the piece complete.
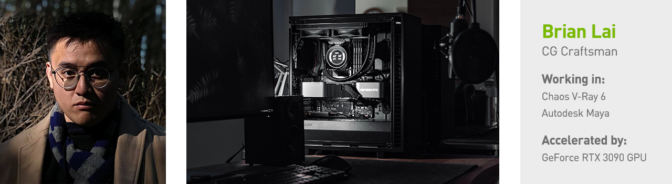
Find more work from Lai, who first made his name discovering invert art, or negative drawing, on his Instagram.
Join the #ExtendtheOmniverse contest, running through Friday, Aug. 19. Perform something akin to magic by making your own NVIDIA Omniverse Extension for a chance to win an NVIDIA RTX GPU. Winners will be announced in September at GTC.
Follow NVIDIA Studio on Instagram, Twitter and Facebook. Access tutorials on the Studio YouTube channel and get updates directly in your inbox by subscribing to the NVIDIA Studio newsletter.
The post July NVIDIA Studio Driver Improves Performance for Chaos V-Ray 6 for 3ds Max appeared first on NVIDIA Blog.