Amazon Forecast is a fully managed service that uses statistical and machine learning (ML) algorithms to deliver highly accurate time-series forecasts. Recently, based on Amazon Forecast, we helped one of our retail customers achieve accurate demand forecasting, within 8 weeks. The solution improved the manual forecast by an average of 10% in regards to the WAPE metric. This leads to a direct savings of 16 labor hours monthly. In addition, we estimated that by fulfilling the correct number of items, sales could increase by up to 11.8%. In this post, we present the workflow and the critical elements to implement—from proof of concept (POC) to production—a demand forecasting system with Amazon Forecast, focused on challenges in the retail industry.
Background and current challenges of demand forecasting in the retail industry
The goal of demand forecasting is to estimate future demand from historical data, and to help store replenishment and capacity allocation. With demand forecasting, retailers are able to position the right amount of inventory at each location in their network to meet demand. Therefore, an accurate forecasting system can drive a wide range of benefits across different business functions, such as:
- Increasing sales from better product availability and reducing the effort of inter-store transfer waste
- Providing more reliable insight to improve capacity utilization and proactively avoid bottlenecks in capacity provisioning
- Minimizing inventory and production costs and improve inventory turnover
- Presenting an overall better customer experience
ML techniques demonstrate great value when a large volume of good quality data is present. Today, experience-based replenishment management or demand forecast is still the mainstream for most retailers. With the goal to improve the customer experience, more and more retailers are willing to replace experience-based demand forecasting systems with ML-based forecasts. However, retailers face multiple challenges when implementing ML-based demand forecasting systems into production. We summarize the different challenges into three categories: data challenges, ML challenges, and operational challenges.
Data challenges
A large volume of clean, quality data is a key requirement for driving accurate ML-based predictions. Quality data, including historical sales and sales-related data (such as inventory, item pricing, and promotions), needs to be collected and consolidated. The diversity of data from multiple resources requires a modern data platform to unite data silos. In addition, access to data in a timely manner is necessary for frequent and fine-grained demand forecasts.
ML challenges
Developing advanced ML algorithms requires expertise. Implementing the right algorithms for the right problem needs both in-depth domain knowledge and ML competences. In addition, learning from large available datasets requires a scalable ML infrastructure. Moreover, maintaining ML algorithms in production requires ML competences in order to analyze the root cause of model degradation and correctly retrain the model.
To solve practical business problems, producing accurate forecasts is only part of the story. Decision-makers need probabilistic forecasts at different quantiles make important customer experience vs. financial results trade-off decisions. They also need to explain predictions to stakeholders, and perform what-if analyses to investigate how different scenarios might affect forecast results.
Operational challenges
Reducing the operational effort of maintaining a cost-effective forecasting system is the third principal challenge. In a common scenario of demand forecasting, each item at each location has its own forecast. A system that can manage hundreds of thousands of forecasts at any time is required. In addition, business end-users need the forecasting system to be integrated into existing downstream systems, such as existing supply chain management platforms, so that they can use ML-based systems without modifying existing tools and processes.
These challenges are especially acute when business are large, dynamic, and growing. To address these challenges, we share a customer success story that reduces the efforts to quickly validate the potential business gain. This is achieved through prototyping with Amazon Forecast—a fully managed service that provides accurate forecasting results without the need to manage underlying infrastructure resources and algorithms.
Rapid prototyping for an ML-based forecasting system with Amazon Forecast
Based on our experience, we often see that retail customers are willing to initiate a proof of concept on their sales data. This can be done within a range of a few days to a few weeks for rapid prototyping, depending on the data complexity and available resources to iterate through the model tuning process. During prototyping, we suggest using sprints to effectively manage the process, and separating the POC into data exploration, iterative improvement, and automation phases.
Data exploration
Data exploration often involves intense discussion with data scientists or business intelligence analysts to get familiar with the historical sales dataset and available data sources that can potentially impact forecast results, such as inventory and historical promotional events. One of the most efficient ways is to consolidate the sales data, as the target dataset, from the data warehouse at the early stage of the project. This is based on the fact that forecast results are often dominated by the target dataset patterns. Data warehouses often store day-to-day business data, and an exhaustive understanding within a short period of time is difficult and time consuming. Our suggestion is to concentrate on generating the target dataset and make sure this dataset is correct. These data exploration and baseline results can often be achieved within a few days, and this can determine if the target data can be accurately forecasted. We discuss data forecastability later in this post.
Iteration
After we have the baseline results, we can continue adding more related data to see how these can impact accuracy. This is often done through a deep dive into additional datasets; for more information, refer to Using Related Time Series Datasets and Using Item Metadata Datasets.
In some cases, it may be possible to improve accuracy in Amazon Forecast by training the models with similarly behaving subsets of the dataset, or by removing the sparse data from the dataset. During this iterative improvement phase, the challenging part—true for all ML projects—is that the current iteration depends on the previous iteration’s key findings and insights, so rigorous analysis and reporting is key for success.
Analysis can be done quantitatively and empirically. The quantitative aspect refers to evaluation during the backtesting and comparing the accuracy metric, such as WAPE. The empirical aspect refers to visualizing the prediction curve and actual target data, and using the domain knowledge to incorporate potential factors. These analyses help you iterate faster to bridge the gap between forecasted results and target data. In addition, presenting such results via a weekly report can often provide confidence to business end-users.
Automation
The final step often involves the discussion of POC to production procedure and automation. Because the ML project is constrained by the total project duration, we might not have enough time to explore every possibility. Therefore, indicating the potential area throughout the findings during the project can often earn trust. In addition, automation can help business end-users evaluate Forecast for a longer period, because they can use an existing predictor to generate forecasts with the updated data.
The success criteria can be evaluated with generated results, both from technical and business perspectives. During the evaluation period, we can estimate potential benefits for the following:
- Increasing the forecast accuracy (technical) – Compute the prediction accuracy with regards to actual sales data, and compare with the existing forecast system, including manual forecasts
- Reducing waste (business) – Reduce over-forecasting in order to reduce waste
- Improving in-stock rates (business) – Reduce under-forecasting in order to improve in-stock rates
- Estimating the increase of gross profit (business) – Reduce wastage and improve in-stock rates in order to increase gross profit
We summarize the development workflow in the following diagram.
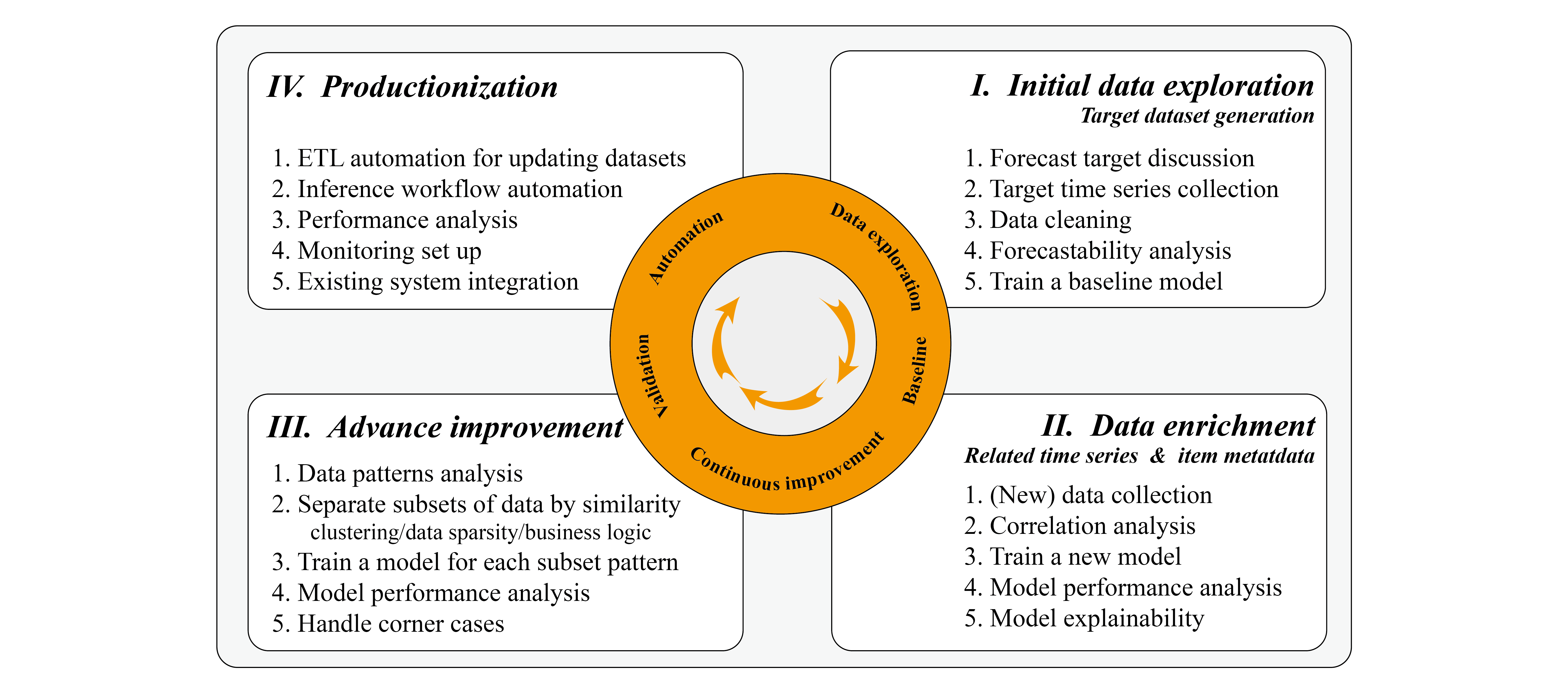
In the following sections, we discuss the important elements to take into consideration during the implementation.
Step-by-step workflow for developing a forecasting system
Target dataset generation
The first step is to generate the target dataset for Forecast. In the retail industry, this refers to the historical time series demand and sales data for retail items (SKUs). When preparing the dataset, one important aspect is granularity. We should consider the data granularity from both business requirements and technical requirements.
The business defines how forecasting results in the production system:
- Horizon – The number of time steps being forecasted. This depends on the underlying business problem. If we want to refill the stock level each week, then a weekly forecast or daily forecast seems appropriate.
- Granularity – The granularity of your forecasts: time frequency such as daily or weekly, different store locations, and different sizes of the same item. In the end, the prediction can be a combination of each store SKU, with daily data points.
Although the aforementioned forecast horizon and granularity should be defined to prioritize the business requirement, we might need to make trade-offs between requirements and feasibility. Take the footwear business as one example. If we want to predict sales of each shoe size at each store level, the data soon becomes sparse and the pattern is hard to find. However, to refill stock, we need to estimate this granularity. To do this, alternative solutions might require estimating a ratio between different shoe sizes and using this ratio to calculate fine-grained results.
We often need to balance the business requirement and the data pattern that can be learned and used for forecasting. To provide a quantitative qualification of the data patterns, we propose using data forecastability.
Data forecastability and data pattern classification
One of the key insights that we can collect from the target dataset is its ability to produce quality forecasts. This can be analyzed at the very early phase of the ML project. Forecast shines when data shows seasonality, trends, and cyclical patterns.
To determine forecastability, there are two major coefficients: variability in demand timing and variability in demand quantity. Variability in demand timing means the interval between two instances of demand, and it measures the demand regularity in time. Variability in demand quantity means variation in quantities. The following figure illustrates some different patterns. Forecast accuracy strongly depends on product forecastability. For more information, refer to Demand classification: why forecastability matters.
It’s worth noting that this forecastability analysis is for each fine-grained item (for example, SKU-Store-Color-Size). It’s quite common that in a demand forecasting production system, different items follow different patterns. Therefore, it’s important to separate the items following different data patterns. One typical example is fast-moving and slow-moving items; another example would be dense and sparse data. In addition, a fine-grained item has more chances of yielding a lumpy pattern. For example, in a clothing store, the sales of one popular item can be quite smooth daily, but if we further separate the sales of the item for each color and size, it soon becomes sparse. Therefore, reducing the granularity from SKU-Store-Color-Size to SKU-Store can change the data pattern from lumpy to smooth, and vice versa.
Moreover, not all items contribute to sales equally. We have observed that item contribution often follows the Pareto distribution, in which top items contribute most of the sales. The sales of these top items are often smooth. Items with a lower sales record are often lumpy and erratic, and therefore hard to estimate. Adding these items might actually decrease the accuracy of top sales items. Based on these observations, we can separate the items into different groups, train the Forecast model on top sales items, and handle the lower sales items as corner cases.
Data enrichment and additional dataset selection
When we want to use additional datasets to improve the performance of forecast results, we can rely on time series datasets and metadata datasets. In the retail domain, based on intuition and domain knowledge, features such as inventory, price, promotion, and winter or summer seasons could be imported as the related time series. The simplest way to identify usefulness of features is via feature importance. In Forecast, this is done by explainability analysis. Forecast Predictor Explainability helps us better understand how the attributes in the datasets impact forecasts for the target. Forecast uses a metric called impact scores to quantify the relative impact of each attribute and determine whether they increase or decrease forecast values. If one or more attributes have an impact score of zero, then these attributes have no significant impact on forecast values. This way, we can quickly remove the features that have less impact and add the potential ones iteratively. It’s important to note that impact scores measure the relative impact of attributes, which are normalized together with impact scores of all other attributes.
Like all ML projects, improving accuracy with additional features requires iterative experiments. You need to experiment with multiple combinations of datasets, while observing the impact of incremental changes on model accuracy. You can try to run multiple Forecast experiments via the Forecast console or with Python notebooks with Forecast APIs. In addition, you can onboard with AWS CloudFormation, which deploys AWS provided ready-made solutions for common use cases (for example, the Improving Forecast Accuracy with Machine Learning solution). Forecast automatically separates the dataset and produces accuracy metrics to evaluate predictors. For more information, see Evaluating Predictor Accuracy. This helps data scientists iterate faster to achieve the best performing model.
Advanced improvement and handling corner cases
We mentioned that forecast algorithms can learn seasonality, trends, and cyclical features from data. For items with these characteristics, and the appropriate data density and volume, we can use Forecast to generate estimations. However, when facing lumpy data patterns, especially when the data volume is small, we might need to handle them differently, such as with empirical estimation based on a ruleset.
For dense SKUs, we further improve Forecast accuracy by training the models with similarly behaving subsets of the time series dataset. The subset separation strategies that we used are business logic, product type, data density, and patterns learned by the algorithm. After the subsets are generated, we can train multiple Forecast models for the different subsets. For one such example, refer to Cluster time series data for use with Amazon Forecast.
Toward production: Updating the dataset, monitoring, and retraining
Let’s explore an example architecture with Forecast, as shown in the following diagram. Each time an end-user consolidates a new dataset on Amazon Simple Storage Service (Amazon S3), it triggers AWS Step Functions to orchestrate different components, including creating the dataset import job, creating an auto predictor, and generating forecasts. After the forecast results are generated, the Create Forecast Export step exports them to Amazon S3 for downstream consumers. For more information about how to provision this automated pipeline, refer to Automating with AWS CloudFormation. It uses a CloudFormation stack to automatically deploy datasets to an S3 bucket and trigger a Forecast pipeline. You can use the same automation stack to generate forecasts with your own datasets.
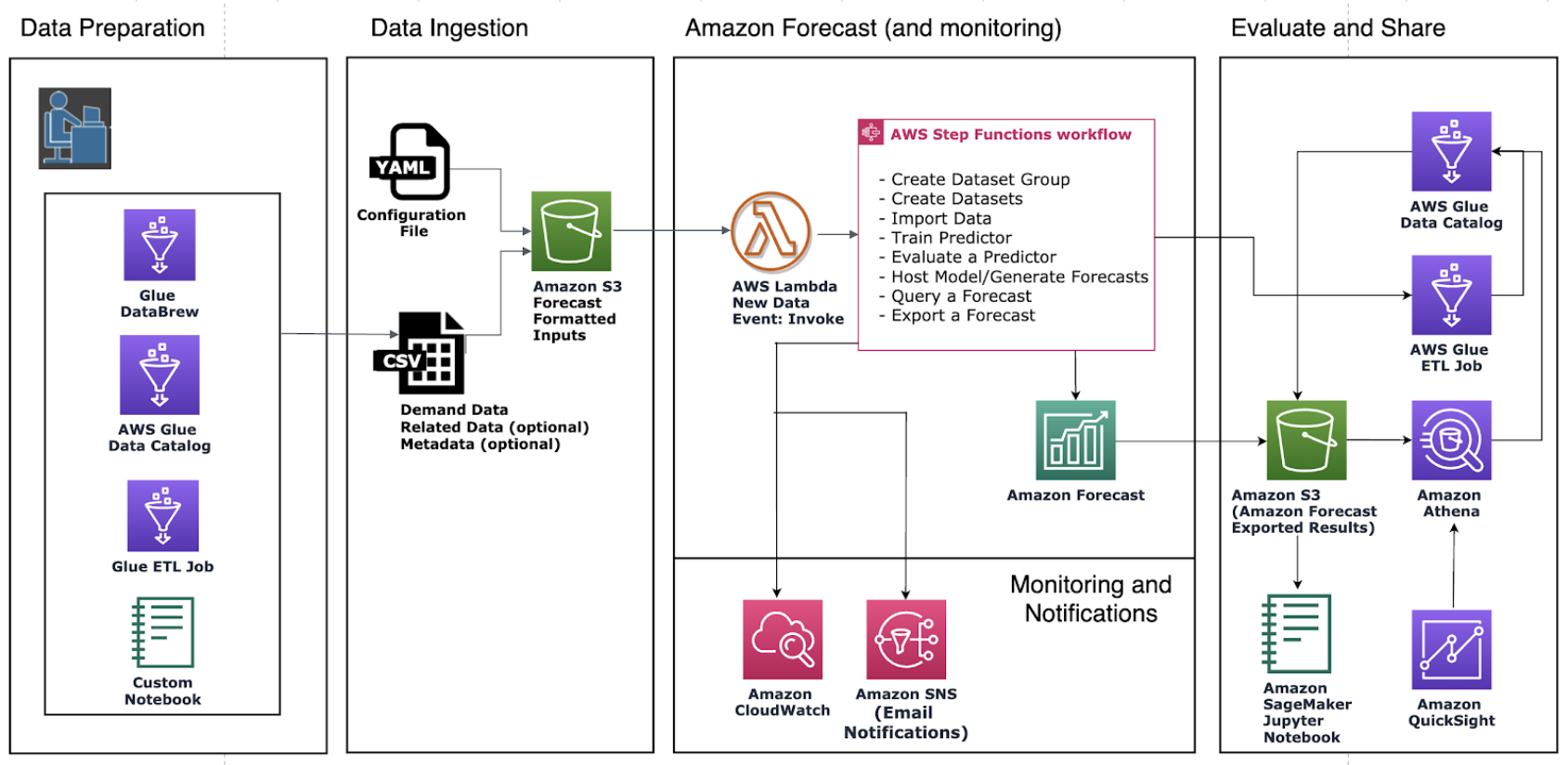
There are two ways to incorporate recent trends into the forecasting system: updating data or retraining the predictor.
To generate the forecast with updated data reflecting recent trends, you need to upload the updated input data file to an S3 bucket (the updated input data should still contain all of your existing data). Forecast doesn’t automatically retrain a predictor when you import an updated dataset. You can generate forecasts as you usually do. Forecast predicts the forecast horizon starting from the last day in the updated input data. Therefore, recent trends are incorporated into any new inferences produced by Forecast.
However, if you want your predictor to be trained off of the new data, you must create a new predictor. You might need to consider retraining the model when data patterns (seasonality, trends, or cycles) change. As mentioned in Continuously monitor predictor accuracy with Amazon Forecast, the performance of a predictor will fluctuate over time, due to factors such as changes in the economic environment or in consumer behavior. Therefore, the predictor may need to be retrained, or a new predictor may need to be created to ensure highly accurate predictions continue to be made. With the help of predictor monitoring, Forecast can track the quality of your predictors, allowing you to reduce operational efforts, while helping you make more informed decisions about keeping, retraining, or rebuilding your predictors.
Conclusion
Amazon Forecast is a time series forecasting service based on ML and built for business metrics analysis. We can integrate demand forecasting prediction with high accuracy by combining historical sales and other relevant information such as inventory, promotions, or season. Within 8 weeks, we helped one of our retail customers achieve accurate demand forecasting—10% improvement in comparison with the manual forecast. This leads to a direct savings of 16 labor hours monthly and estimated sales increase by up to 11.8%.
This post shared common practices for bringing your forecasting project from proof of concept to production. Get started now with Amazon Forecast to achieve highly accurate forecasts for your business.
About the Authors
Yanwei Cui, PhD, is a Machine Learning Specialist Solutions Architect at AWS. He started machine learning research at IRISA (Research Institute of Computer Science and Random Systems), and has several years of experience building artificial intelligence powered industrial applications in computer vision, natural language processing and online user behavior prediction. At AWS, he shares the domain expertise and helps customers to unlock business potentials, and to drive actionable outcomes with machine learning at scale. Outside of work, he enjoys reading and traveling.
Gordon Wang is a Senior Data Scientist on the Professional Services team at Amazon Web Services. He supports customers in many industries, including media, manufacturing, energy, retail, and healthcare. He is passionate about computer vision, deep learning, and MLOps. In his spare time, he loves running and hiking.
Read More