Analyzing real-world healthcare and life sciences (HCLS) data poses several practical challenges, such as distributed data silos, lack of sufficient data at a single site for rare events, regulatory guidelines that prohibit data sharing, infrastructure requirement, and cost incurred in creating a centralized data repository. Because they’re in a highly regulated domain, HCLS partners and customers seek privacy-preserving mechanisms to manage and analyze large-scale, distributed, and sensitive data.
To mitigate these challenges, we propose a federated learning (FL) framework, based on open-source FedML on AWS, which enables analyzing sensitive HCLS data. It involves training a global machine learning (ML) model from distributed health data held locally at different sites. It doesn’t require moving or sharing data across sites or with a centralized server during the model training process.
Deploying an FL framework on the cloud has several challenges. Automating the client-server infrastructure to support multiple accounts or virtual private clouds (VPCs) requires VPC peering and efficient communication across VPCs and instances. In a production workload, a stable deployment pipeline is needed to seamlessly add and remove clients and update their configurations without much overhead. Furthermore, in a heterogenous setup, clients may have varying requirements for compute, network, and storage. In this decentralized architecture, logging and debugging errors across clients can be difficult. Finally, determining the optimal approach to aggregate model parameters, maintain model performance, ensure data privacy, and improve communication efficiency is an arduous task. In this post, we address these challenges by providing a federated learning operations (FLOps) template that hosts a HCLS solution. The solution is agnostic to use cases, which means you can adapt it for your use cases by changing the model and data.
In this two-part series, we demonstrate how you can deploy a cloud-based FL framework on AWS. In the first post, we described FL concepts and the FedML framework. In this second part, we present a proof-of-concept healthcare and life sciences use case from a real-world dataset eICU. This dataset comprises a multi-center critical care database collected from over 200 hospitals, which makes it ideal to test our FL experiments.
HCLS use case
For the purpose of demonstration, we built an FL model on a publicly available dataset to manage critically ill patients. We used the eICU Collaborative Research Database, a multi-center intensive care unit (ICU) database, comprising 200,859 patient unit encounters for 139,367 unique patients. They were admitted to one of 335 units at 208 hospitals located throughout the US between 2014–2015. Due to the underlying heterogeneity and distributed nature of the data, it provides an ideal real-world example to test this FL framework. The dataset includes laboratory measurements, vital signs, care plan information, medications, patient history, admission diagnosis, time-stamped diagnoses from a structured problem list, and similarly chosen treatments. It is available as a set of CSV files, which can be loaded into any relational database system. The tables are de-identified to meet the regulatory requirements US Health Insurance Portability and Accountability Act (HIPAA). The data can be accessed via a PhysioNet repository, and details of the data access process can be found here [1].
The eICU data is ideal for developing ML algorithms, decision support tools, and advancing clinical research. For benchmark analysis, we considered the task of predicting the in-hospital mortality of patients [2]. We defined it as a binary classification task, where each data sample spans a 1-hour window. To create a cohort for this task, we selected patients with a hospital discharge status in the patient’s record and a length of stay of at least 48 hours, because we focus on prediction mortality during the first 24 and 48 hours. This created a cohort of 30,680 patients containing 1,164,966 records. We adopted domain-specific data preprocessing and methods described in [3] for mortality prediction. This resulted in an aggregated dataset comprising several columns per patient per record, as shown in the following figure. The following table provides a patient record in a tabular style interface with time in columns (5 intervals over 48 hours) and vital sign observations in rows. Each row represents a physiological variable, and each column represents its value recorded over a time window of 48 hours for a patient.
Physiologic Parameter |
Chart_Time_0 |
Chart_Time_1 |
Chart_Time_2 |
Chart_Time_3 |
Chart_Time_4 |
Glasgow Coma Score Eyes |
4 |
4 |
4 |
4 |
4 |
FiO2 |
15 |
15 |
15 |
15 |
15 |
Glasgow Coma Score Eyes |
15 |
15 |
15 |
15 |
15 |
Heart Rate |
101 |
100 |
98 |
99 |
94 |
Invasive BP Diastolic |
73 |
68 |
60 |
64 |
61 |
Invasive BP Systolic |
124 |
122 |
111 |
105 |
116 |
Mean arterial pressure (mmHg) |
77 |
77 |
77 |
77 |
77 |
Glasgow Coma Score Motor |
6 |
6 |
6 |
6 |
6 |
02 Saturation |
97 |
97 |
97 |
97 |
97 |
Respiratory Rate |
19 |
19 |
19 |
19 |
19 |
Temperature (C) |
36 |
36 |
36 |
36 |
36 |
Glasgow Coma Score Verbal |
5 |
5 |
5 |
5 |
5 |
admissionheight |
162 |
162 |
162 |
162 |
162 |
admissionweight |
96 |
96 |
96 |
96 |
96 |
age |
72 |
72 |
72 |
72 |
72 |
apacheadmissiondx |
143 |
143 |
143 |
143 |
143 |
ethnicity |
3 |
3 |
3 |
3 |
3 |
gender |
1 |
1 |
1 |
1 |
1 |
glucose |
128 |
128 |
128 |
128 |
128 |
hospitaladmitoffset |
-436 |
-436 |
-436 |
-436 |
-436 |
hospitaldischargestatus |
0 |
0 |
0 |
0 |
0 |
itemoffset |
-6 |
-1 |
0 |
1 |
2 |
pH |
7 |
7 |
7 |
7 |
7 |
patientunitstayid |
2918620 |
2918620 |
2918620 |
2918620 |
2918620 |
unitdischargeoffset |
1466 |
1466 |
1466 |
1466 |
1466 |
unitdischargestatus |
0 |
0 |
0 |
0 |
0 |
We used both numerical and categorical features and grouped all records of each patient to flatten them into a single-record time series. The seven categorical features (Admission diagnosis, Ethnicity, Gender, Glasgow Coma Score Total, Glasgow Coma Score Eyes, Glasgow Coma Score Motor, and Glasgow Coma Score Verbal were converted to one-hot encoding vectors) contained 429 unique values and were converted into one-hot embeddings. To prevent data leakage across training node servers, we split the data by hospital IDs and kept all records of a hospital on a single node.
Solution overview
The following diagram shows the architecture of multi-account deployment of FedML on AWS. This includes two clients (Participant A and Participant B) and a model aggregator.
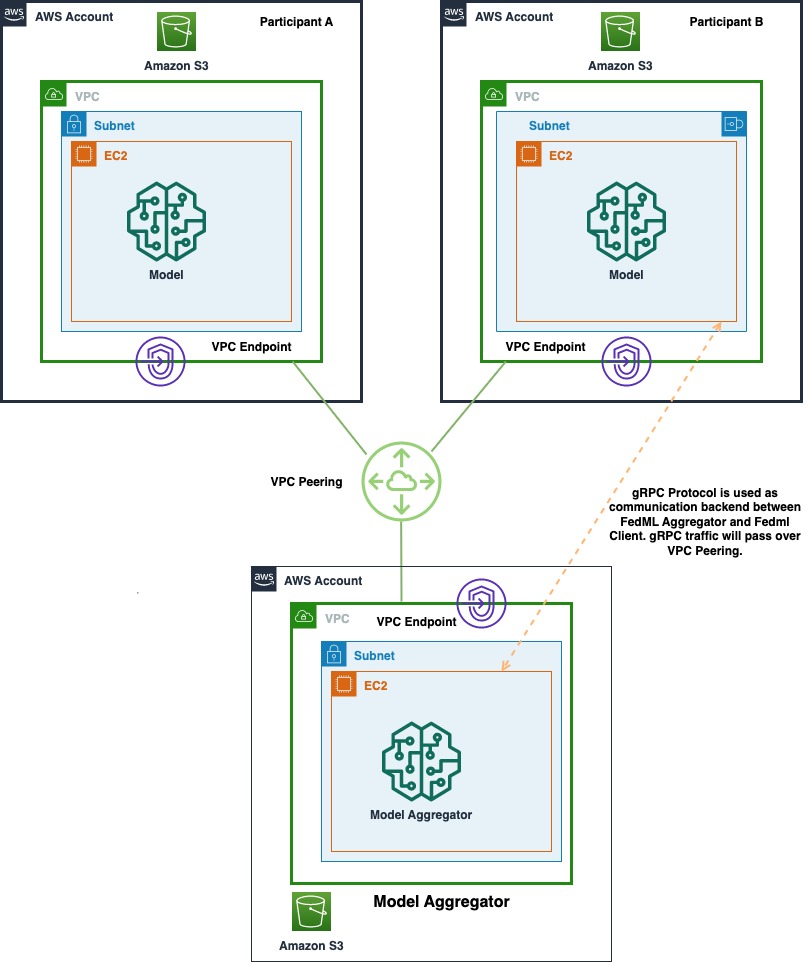
The architecture consists of three separate Amazon Elastic Compute Cloud (Amazon EC2) instances running in its own AWS account. Each of the first two instances is owned by a client, and the third instance is owned by the model aggregator. The accounts are connected via VPC peering to allow ML models and weights to be exchanged between the clients and aggregator. gRPC is used as communication backend for communication between model aggregator and clients. We tested a single account-based distributed computing setup with one server and two client nodes. Each of these instances were created using a custom Amazon EC2 AMI with FedML dependencies installed as per the FedML.ai installation guide.
Set up VPC peering
After you launch the three instances in their respective AWS accounts, you establish VPC peering between the accounts via Amazon Virtual Private Cloud (Amazon VPC). To set up a VPC peering connection, first create a request to peer with another VPC. You can request a VPC peering connection with another VPC in your account, or with a VPC in a different AWS account. To activate the request, the owner of the VPC must accept the request. For the purpose of this demonstration, we set up the peering connection between VPCs in different accounts but the same Region. For other configurations of VPC peering, refer to Create a VPC peering connection.
Before you begin, make sure that you have the AWS account number and VPC ID of the VPC to peer with.
Request a VPC peering connection
To create the VPC peering connection, complete the following steps:
- On the Amazon VPC console, in the navigation pane, choose Peering connections.
- Choose Create peering connection.
- For Peering connection name tag, you can optionally name your VPC peering connection.Doing so creates a tag with a key of the name and a value that you specify. This tag is only visible to you; the owner of the peer VPC can create their own tags for the VPC peering connection.
- For VPC (Requester), choose the VPC in your account to create the peering connection.
- For Account, choose Another account.
- For Account ID, enter the AWS account ID of the owner of the accepter VPC.
- For VPC (Accepter), enter the VPC ID with which to create the VPC peering connection.
- In the confirmation dialog box, choose OK.
- Choose Create peering connection.
Accept a VPC peering connection
As mentioned earlier, the VPC peering connection needs to be accepted by the owner of the VPC the connection request has been sent to. Complete the following steps to accept the peering connection request:
- On the Amazon VPC console, use the Region selector to choose the Region of the accepter VPC.
- In the navigation pane, choose Peering connections.
- Select the pending VPC peering connection (the status is
pending-acceptance
), and on the Actions menu, choose Accept Request.
- In the confirmation dialog box, choose Yes, Accept.
- In the second confirmation dialog, choose Modify my route tables now to go directly to the route tables page, or choose Close to do this later.
Update route tables
To enable private IPv4 traffic between instances in peered VPCs, add a route to the route tables associated with the subnets for both instances. The route destination is the CIDR block (or portion of the CIDR block) of the peer VPC, and the target is the ID of the VPC peering connection. For more information, see Configure route tables.
Update your security groups to reference peer VPC groups
Update the inbound or outbound rules for your VPC security groups to reference security groups in the peered VPC. This allows traffic to flow across instances that are associated with the referenced security group in the peered VPC. For more details about setting up security groups, refer to Update your security groups to reference peer security groups.
Configure FedML
After you have the three EC2 instances running, connect to each of them and perform the following steps:
- Clone the FedML repository.
- Provide topology data about your network in the config file
grpc_ipconfig.csv
.
This file can be found at FedML/fedml_experiments/distributed/fedavg
in the FedML repository. The file includes data about the server and clients and their designated node mapping, such as FL Server – Node 0, FL Client 1 – Node 1, and FL Client 2 – Node2.
- Define the GPU mapping config file.
This file can be found at FedML/fedml_experiments/distributed/fedavg
in the FedML repository. The file gpu_mapping.yaml
consists of configuration data for client server mapping to the corresponding GPU, as shown in the following snippet.
After you define these configurations, you’re ready to run the clients. Note that the clients must be run before kicking off the server. Before doing that, let’s set up the data loaders for the experiments.
Customize FedML for eICU
To customize the FedML repository for eICU dataset, make the following changes to the data and data loader.
Data
Add data to the pre-assigned data folder, as shown in the following screenshot. You can place the data in any folder of your choice, as long as the path is consistently referenced in the training script and has access enabled. To follow a real-world HCLS scenario, where local data isn’t shared across sites, split and sample the data so there’s no overlap of hospital IDs across the two clients. This ensures the data of a hospital is hosted on its own server. We also enforced the same constraint to split the data into train/test sets within each client. Each of the train/test sets across the clients had a 1:10 ratio of positive to negative labels, with roughly 27,000 samples in training and 3,000 samples in test. We handle the data imbalance in model training with a weighted loss function.
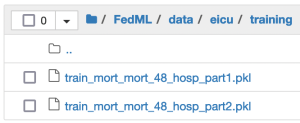
Data loader
Each of the FedML clients loads the data and converts it into PyTorch tensors for efficient training on GPU. Extend the existing FedML nomenclature to add a folder for eICU data in the data_processing
folder.
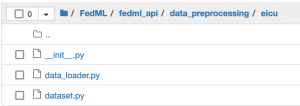
The following code snippet loads the data from the data source. It preprocesses the data and returns one item at a time through the __getitem__
function.
import logging
import pickle
import random
import numpy as np
import torch.utils.data as data
class eicu_truncated(data.Dataset):
def __init__(self, file_path, dataidxs=None, transform=None, target_transform=None,
task='mort', ohe=True, cat=True, num=True, n_cat_class=429):
<code to initialize class variables>
def _load_data(self, file_path):
<code to load data files for each client>
def __getitem__(self, index):
<code to process data and return input and labels>
return x.astype(np.float32), y
def __len__(self):
return len(self.data)
Training ML models with a single data point at a time is tedious and time-consuming. Model training is typically done on a batch of data points at each client. To implement this, the data loader in the data_loader.py
script converts NumPy arrays into Torch tensors, as shown in the following code snippet. Note that FedML provides dataset.py
and data_loader.py
scripts for both structured and unstructured data that you can use for data-specific alterations, as in any PyTorch project.
import logging
import numpy as np
import torch
import torch.utils.data as data
import torchvision.transforms as transforms
from .dataset import eicu_truncated #load the dataset.py file mentioned above
.
.
.
.
# Use standard FedML functions for data distribution and split here
.
.
.
.
# Invoke load_partition_data function for model training. Adapt this function for your dataset
def load_partition_data_eicu(dataset, train_file, test_file, partition_method, partition_alpha, client_number, batch_size):
<code to partition eicu data and its aggregated statistics>
return train_data_num, test_data_num, train_data_global, test_data_global,
data_local_num_dict, train_data_local_dict, test_data_local_dict, class_num, net_dataidx_map
Import the data loader into the training script
After you create the data loader, import it into the FedML code for ML model training. Like any other dataset (for example, CIFAR-10 and CIFAR-100), load the eICU data to the main_fedavg.py
script in the path FedML/fedml_experiments/distributed/fedavg/
. Here, we used the federated averaging (fedavg
) aggregation function. You can follow a similar method to set up the main
file for any other aggregation function.
from fedml_api.data_preprocessing.cifar100.data_loader import load_partition_data_cifar100
from fedml_api.data_preprocessing.cinic10.data_loader import load_partition_data_cinic10
# For eicu
from fedml_api.data_preprocessing.eicu.data_loader import load_partition_data_eicu
We call the data loader function for eICU data with the following code:
elif dataset_name == "eicu":
logging.info("load_data. dataset_name = %s" % dataset_name)
args.client_num_in_total = 2
train_data_num, test_data_num, train_data_global, test_data_global,
train_data_local_num_dict, train_data_local_dict, test_data_local_dict,
class_num, net_dataidx_map = load_partition_data_eicu(dataset=dataset_name, train_file=args.train_file,
test_file=args.test_file, partition_method=args.partition_method, partition_alpha=args.partition_alpha,
client_number=args.client_num_in_total, batch_size=args.batch_size)
Define the model
FedML supports several out-of-the-box deep learning algorithms for various data types, such as tabular, text, image, graphs, and Internet of Things (IoT) data. Load the model specific for eICU with input and output dimensions defined based on the dataset. For this proof of concept development, we used a logistic regression model to train and predict the mortality rate of patients with default configurations. The following code snippet shows the updates we made to the main_fedavg.py
script. Note that you can also use custom PyTorch models with FedML and import it into the main_fedavg.py
script.
if model_name == "lr" and args.dataset == "mnist":
logging.info("LogisticRegression + MNIST")
model = LogisticRegression(28 * 28, output_dim)
elif model_name == "lr" and args.dataset == "eicu":
logging.info("LogisticRegression + eicu")
model = LogisticRegression(22100, output_dim)
elif model_name == "rnn" and args.dataset == "shakespeare":
logging.info("RNN + shakespeare")
model = RNN_OriginalFedAvg()
Run and monitor FedML training on AWS
The following video shows the training process being initialized in each of the clients. After both the clients are listed for the server, create the server training process that performs federated aggregation of models.
To configure the FL server and clients, complete the following steps:
- Run Client 1 and Client 2.
To run a client, enter the following command with its corresponding node ID. For instance, to run Client 1 with node ID 1, run from the command line:
> sh run_fedavg_cross_zone_eICU.sh 1
- After both the client instances are started, start the server instance using the same command and the appropriate node ID per your configuration in the
grpc_ipconfig.csv file
. You can see the model weights being passed to the server from the client instances.
- We train FL model for 50 epochs. As you can see in the below video, the weights are transferred between nodes 0, 1, and 2, indicating the training is progressing as expected in a federated manner.
- Finally, monitor and track the FL model training progression across different nodes in the cluster using the weights and biases (wandb) tool, as shown in the following screenshot. Please follow the steps listed here to install wandb and setup monitoring for this solution.
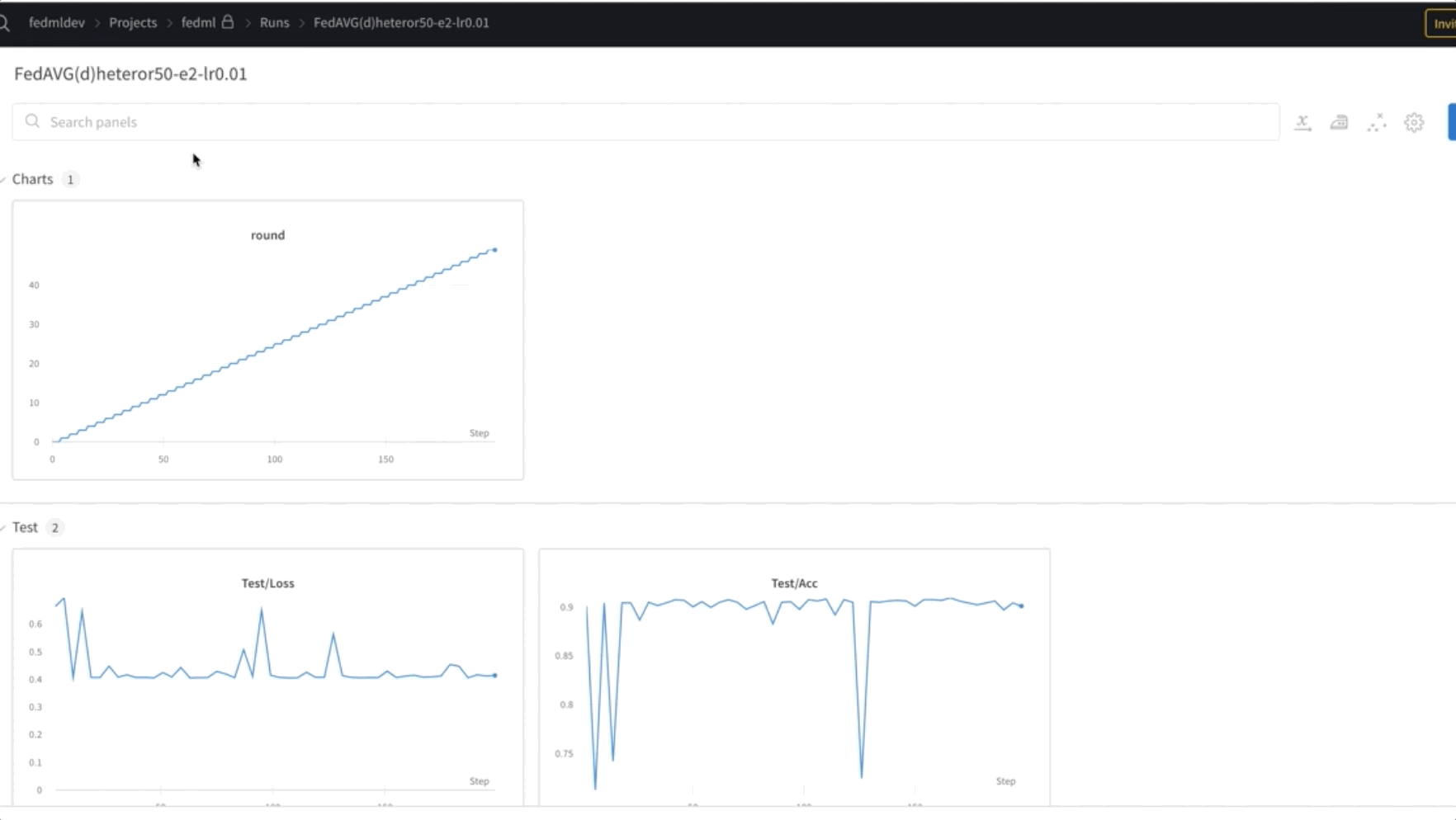
The following video captures all these steps to provide an end-to-end demonstration of FL on AWS using FedML:
Conclusion
In this post, we showed how you can deploy an FL framework, based on open-source FedML, on AWS. It allows you to train an ML model on distributed data, without the need to share or move it. We set up a multi-account architecture, where in a real-world scenario, hospitals or healthcare organizations can join the ecosystem to benefit from collaborative learning while maintaining data governance. We used the multi-hospital eICU dataset to test this deployment. This framework can also be applied to other use cases and domains. We will continue to extend this work by automating deployment through infrastructure as code (using AWS CloudFormation), further incorporating privacy-preserving mechanisms, and improving interpretability and fairness of the FL models.
Please review the presentation at re:MARS 2022 focused on “Managed Federated Learning on AWS: A case study for healthcare” for a detailed walkthrough of this solution.
Reference
[1] Pollard, Tom J., et al. “The eICU Collaborative Research Database, a freely available multi-center database for critical care research.”
Scientific data 5.1 (2018): 1-13.
[2] Yin, X., Zhu, Y. and Hu, J., 2021. A comprehensive survey of privacy-preserving federated learning: A taxonomy, review, and future directions.
ACM Computing Surveys (CSUR),
54(6), pp.1-36.
[3] Sheikhalishahi, Seyedmostafa, Vevake Balaraman, and Venet Osmani. “Benchmarking machine learning models on multi-centre eICU critical care dataset.”
Plos one 15.7 (2020): e0235424.
About the Authors
Vidya Sagar Ravipati is a Manager at the Amazon ML Solutions Lab, where he leverages his vast experience in large-scale distributed systems and his passion for machine learning to help AWS customers across different industry verticals accelerate their AI and cloud adoption. Previously, he was a Machine Learning Engineer in Connectivity Services at Amazon who helped to build personalization and predictive maintenance platforms.
Olivia Choudhury, PhD, is a Senior Partner Solutions Architect at AWS. She helps partners, in the Healthcare and Life Sciences domain, design, develop, and scale state-of-the-art solutions leveraging AWS. She has a background in genomics, healthcare analytics, federated learning, and privacy-preserving machine learning. Outside of work, she plays board games, paints landscapes, and collects manga.
Wajahat Aziz is a Principal Machine Learning and HPC Solutions Architect at AWS, where he focuses on helping healthcare and life sciences customers leverage AWS technologies for developing state-of-the-art ML and HPC solutions for a wide variety of use cases such as Drug Development, Clinical Trials, and Privacy Preserving Machine Learning. Outside of work, Wajahat likes to explore nature, hiking, and reading.
Divya Bhargavi is a Data Scientist and Media and Entertainment Vertical Lead at the Amazon ML Solutions Lab, where she solves high-value business problems for AWS customers using Machine Learning. She works on image/video understanding, knowledge graph recommendation systems, predictive advertising use cases.
Ujjwal Ratan is the leader for AI/ML and Data Science in the AWS Healthcare and Life Science Business Unit and is also a Principal AI/ML Solutions Architect. Over the years, Ujjwal has been a thought leader in the healthcare and life sciences industry, helping multiple Global Fortune 500 organizations achieve their innovation goals by adopting machine learning. His work involving the analysis of medical imaging, unstructured clinical text and genomics has helped AWS build products and services that provide highly personalized and precisely targeted diagnostics and therapeutics. In his free time, he enjoys listening to (and playing) music and taking unplanned road trips with his family.
Chaoyang He is Co-founder and CTO of FedML, Inc., a startup running for a community building open and collaborative AI from anywhere at any scale. His research focuses on distributed/federated machine learning algorithms, systems, and applications. He received his Ph.D. in Computer Science from the University of Southern California, Los Angeles, USA.
Salman Avestimehr is Co-founder and CEO of FedML, Inc., a startup running for a community building open and collaborative AI from anywhere at any scale. Salman Avestimehr is a world-renowned expert in federated learning with over 20 years of R&D leadership in both academia and industry. He is a Dean’s Professor and the inaugural director of the USC-Amazon Center on Trustworthy Machine Learning at the University of Southern California. He has also been an Amazon Scholar in Amazon. He is a United States Presidential award winner for his profound contributions in information technology, and a Fellow of IEEE.
Read More