Customers of every size and industry are innovating on AWS by infusing machine learning (ML) into their products and services. Recent developments in generative AI models have further sped up the need of ML adoption across industries. However, implementing security, data privacy, and governance controls are still key challenges faced by customers when implementing ML workloads at scale. Addressing those challenges builds the framework and foundations for mitigating risk and responsible use of ML-driven products. Although generative AI may need additional controls in place, such as removing toxicity and preventing jailbreaking and hallucinations, it shares the same foundational components for security and governance as traditional ML.
We hear from customers that they require specialized knowledge and investment of up to 12 months for building out their customized Amazon SageMaker ML platform implementation to ensure scalable, reliable, secure, and governed ML environments for their lines of business (LOBs) or ML teams. If you lack a framework for governing the ML lifecycle at scale, you may run into challenges such as team-level resource isolation, scaling experimentation resources, operationalizing ML workflows, scaling model governance, and managing security and compliance of ML workloads.
Governing ML lifecycle at scale is a framework to help you build an ML platform with embedded security and governance controls based on industry best practices and enterprise standards. This framework addresses challenges by providing prescriptive guidance through a modular framework approach extending an AWS Control Tower multi-account AWS environment and the approach discussed in the post Setting up secure, well-governed machine learning environments on AWS.
It provides prescriptive guidance for the following ML platform functions:
- Multi-account, security, and networking foundations – This function uses AWS Control Tower and well-architected principles for setting up and operating multi-account environment, security, and networking services.
- Data and governance foundations – This function uses a data mesh architecture for setting up and operating the data lake, central feature store, and data governance foundations to enable fine-grained data access.
- ML platform shared and governance services – This function enables setting up and operating common services such as CI/CD, AWS Service Catalog for provisioning environments, and a central model registry for model promotion and lineage.
- ML team environments – This function enables setting up and operating environments for ML teams for model development, testing, and deploying their use cases for embedding security and governance controls.
- ML platform observability – This function helps with troubleshooting and identifying the root cause for problems in ML models through centralization of logs and providing tools for log analysis visualization. It also provides guidance for generating cost and usage reports for ML use cases.
Although this framework can provide benefits to all customers, it’s most beneficial for large, mature, regulated, or global enterprises customers that want to scale their ML strategies in a controlled, compliant, and coordinated approach across the organization. It helps enable ML adoption while mitigating risks. This framework is useful for the following customers:
- Large enterprise customers that have many LOBs or departments interested in using ML. This framework allows different teams to build and deploy ML models independently while providing central governance.
- Enterprise customers with a moderate to high maturity in ML. They have already deployed some initial ML models and are looking to scale their ML efforts. This framework can help accelerate ML adoption across the organization. These companies also recognize the need for governance to manage things like access control, data usage, model performance, and unfair bias.
- Companies in regulated industries such as financial services, healthcare, chemistry, and the private sector. These companies need strong governance and audibility for any ML models used in their business processes. Adopting this framework can help facilitate compliance while still allowing for local model development.
- Global organizations that need to balance centralized and local control. This framework’s federated approach allows the central platform engineering team to set some high-level policies and standards, but also gives LOB teams flexibility to adapt based on local needs.
In the first part of this series, we walk through the reference architecture for setting up the ML platform. In a later post, we will provide prescriptive guidance for how to implement the various modules in the reference architecture in your organization.
The capabilities of the ML platform are grouped into four categories, as shown in the following figure. These capabilities form the foundation of the reference architecture discussed later in this post:
- Build ML foundations
- Scale ML operations
- Observable ML
- Secure ML
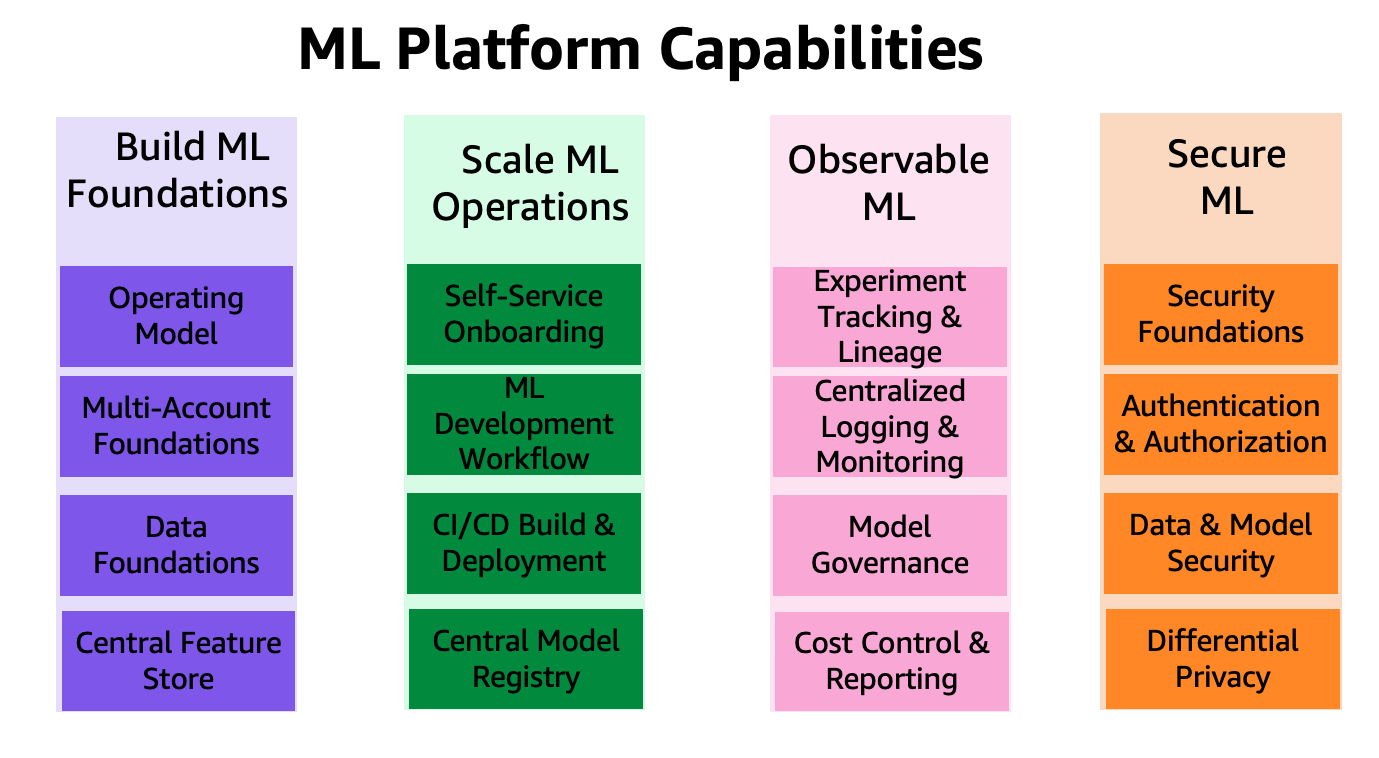
Solution overview
The framework for governing ML lifecycle at scale framework enables organizations to embed security and governance controls throughout the ML lifecycle that in turn help organizations reduce risk and accelerate infusing ML into their products and services. The framework helps optimize the setup and governance of secure, scalable, and reliable ML environments that can scale to support an increasing number of models and projects. The framework enables the following features:
- Account and infrastructure provisioning with organization policy compliant infrastructure resources
- Self-service deployment of data science environments and end-to-end ML operations (MLOps) templates for ML use cases
- LOB-level or team-level isolation of resources for security and privacy compliance
- Governed access to production-grade data for experimentation and production-ready workflows
- Management and governance for code repositories, code pipelines, deployed models, and data features
- A model registry and feature store (local and central components) for improving governance
- Security and governance controls for the end-to-end model development and deployment process
In this section, we provide an overview of prescriptive guidance to help you build this ML platform on AWS with embedded security and governance controls.
The functional architecture associated with the ML platform is shown in the following diagram. The architecture maps the different capabilities of the ML platform to AWS accounts.
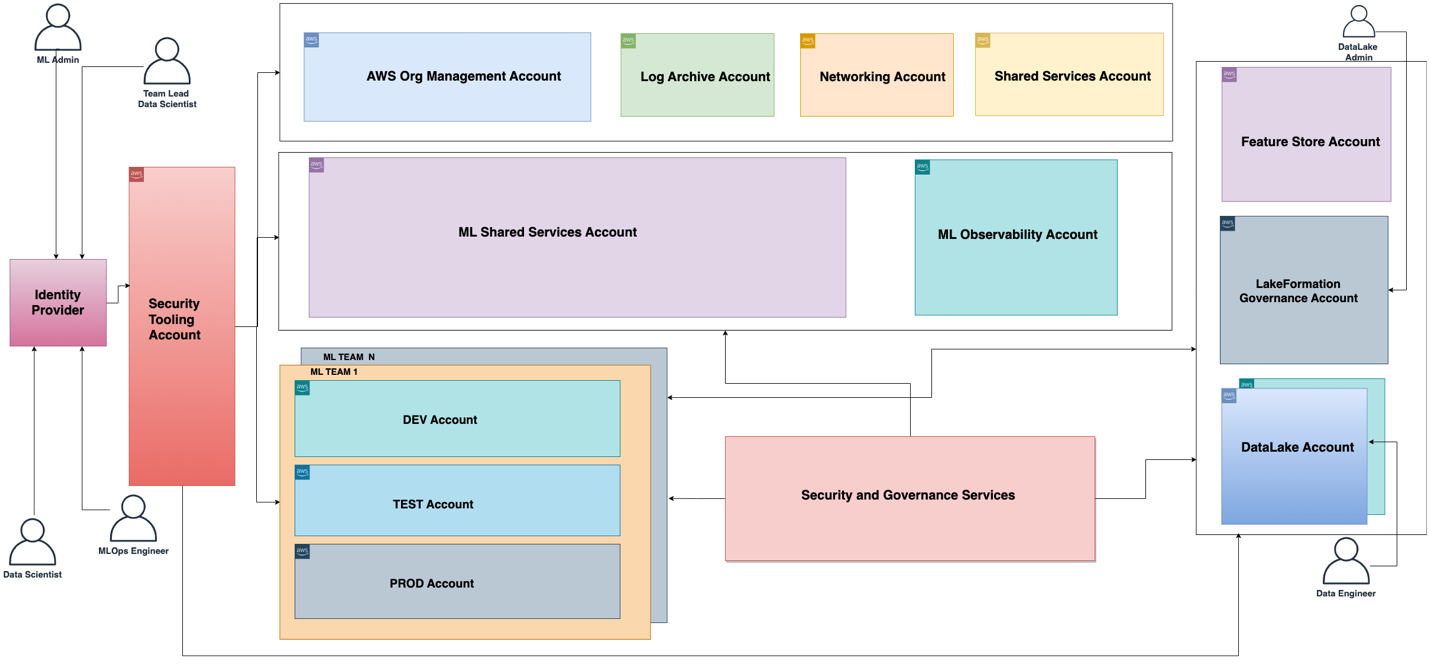
The functional architecture with different capabilities is implemented using a number of AWS services, including AWS Organizations, SageMaker, AWS DevOps services, and a data lake. The reference architecture for the ML platform with various AWS services is shown in the following diagram.
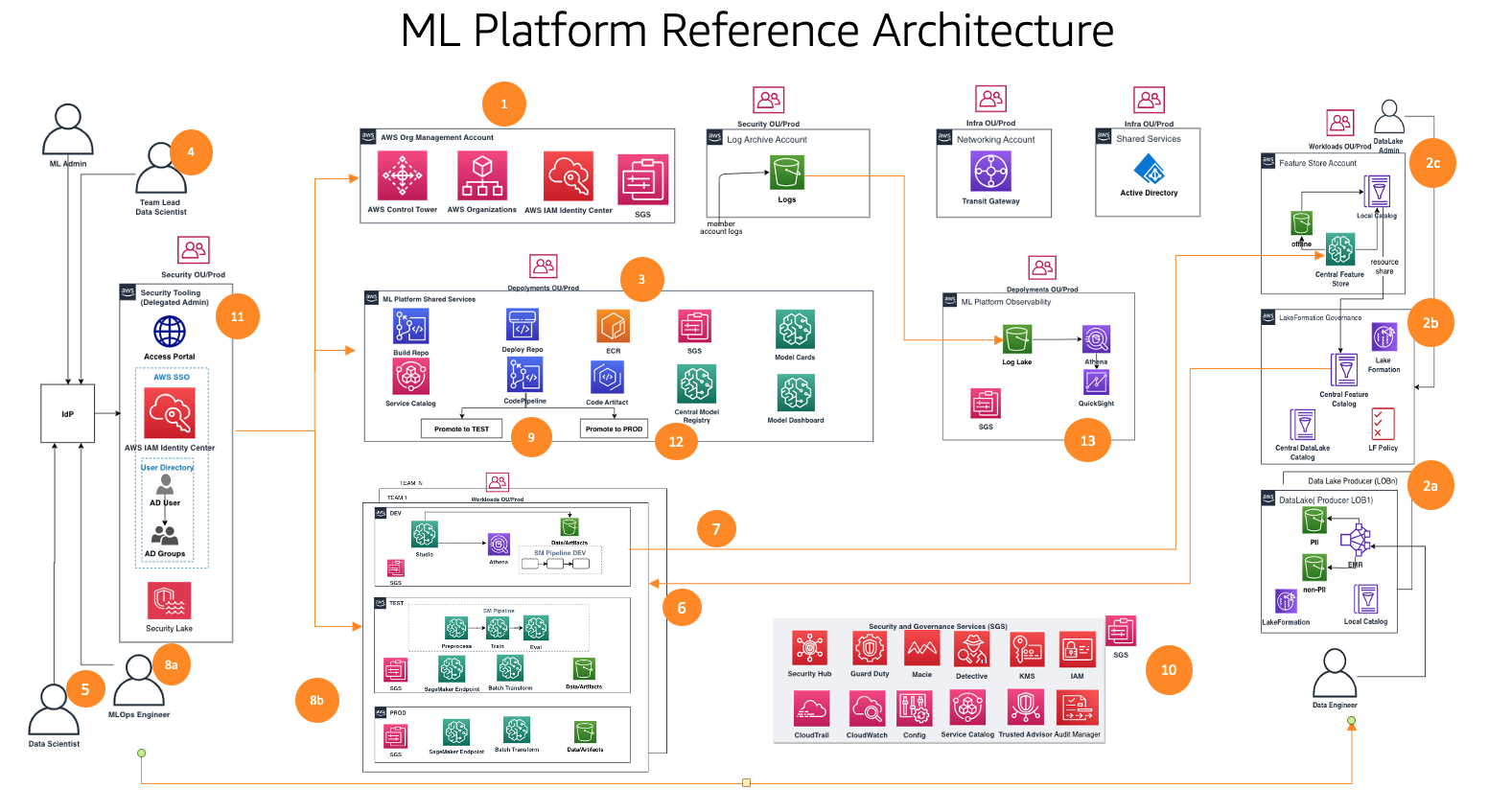
This framework considers multiple personas and services to govern the ML lifecycle at scale. We recommend the following steps to organize your teams and services:
- Using AWS Control Tower and automation tooling, your cloud administrator sets up the multi-account foundations such as Organizations and AWS IAM Identity Center (successor to AWS Single Sign-On) and security and governance services such as AWS Key Management Service (AWS KMS) and Service Catalog. In addition, the administrator sets up a variety of organization units (OUs) and initial accounts to support your ML and analytics workflows.
- Data lake administrators set up your data lake and data catalog, and set up the central feature store working with the ML platform admin.
- The ML platform admin provisions ML shared services such as AWS CodeCommit, AWS CodePipeline, Amazon Elastic Container Registry (Amazon ECR), a central model registry, SageMaker Model Cards, SageMaker Model Dashboard, and Service Catalog products for ML teams.
- The ML team lead federates via IAM Identity Center, uses Service Catalog products, and provisions resources in the ML team’s development environment.
- Data scientists from ML teams across different business units federate into their team’s development environment to build the model pipeline.
- Data scientists search and pull features from the central feature store catalog, build models through experiments, and select the best model for promotion.
- Data scientists create and share new features into the central feature store catalog for reuse.
- An ML engineer deploys the model pipeline into the ML team test environment using a shared services CI/CD process.
- After stakeholder validation, the ML model is deployed to the team’s production environment.
- Security and governance controls are embedded into every layer of this architecture using services such as AWS Security Hub, Amazon GuardDuty, Amazon Macie, and more.
- Security controls are centrally managed from the security tooling account using Security Hub.
- ML platform governance capabilities such as SageMaker Model Cards and SageMaker Model Dashboard are centrally managed from the governance services account.
- Amazon CloudWatch and AWS CloudTrail logs from each member account are made accessible centrally from an observability account using AWS native services.
Next, we dive deep into the modules of the reference architecture for this framework.
Reference architecture modules
The reference architecture comprises eight modules, each designed to solve a specific set of problems. Collectively, these modules address governance across various dimensions, such as infrastructure, data, model, and cost. Each module offers a distinct set of functions and interoperates with other modules to provide an integrated end-to-end ML platform with embedded security and governance controls. In this section, we present a short summary of each module’s capabilities.
Multi-account foundations
This module helps cloud administrators build an AWS Control Tower landing zone as a foundational framework. This includes building a multi-account structure, authentication and authorization via IAM Identity Center, a network hub-and-spoke design, centralized logging services, and new AWS member accounts with standardized security and governance baselines.
In addition, this module gives best practice guidance on OU and account structures that are appropriate for supporting your ML and analytics workflows. Cloud administrators will understand the purpose of the required accounts and OUs, how to deploy them, and key security and compliance services they should use to centrally govern their ML and analytics workloads.
A framework for vending new accounts is also covered, which uses automation for baselining new accounts when they are provisioned. By having an automated account provisioning process set up, cloud administrators can provide ML and analytics teams the accounts they need to perform their work more quickly, without sacrificing on a strong foundation for governance.
Data lake foundations
This module helps data lake admins set up a data lake to ingest data, curate datasets, and use the AWS Lake Formation governance model for managing fine-grained data access across accounts and users using a centralized data catalog, data access policies, and tag-based access controls. You can start small with one account for your data platform foundations for a proof of concept or a few small workloads. For medium-to-large-scale production workload implementation, we recommend adopting a multi-account strategy. In such a setting, LOBs can assume the role of data producers and data consumers using different AWS accounts, and the data lake governance is operated from a central shared AWS account. The data producer collects, processes, and stores data from their data domain, in addition to monitoring and ensuring the quality of their data assets. Data consumers consume the data from the data producer after the centralized catalog shares it using Lake Formation. The centralized catalog stores and manages the shared data catalog for the data producer accounts.
ML platform services
This module helps the ML platform engineering team set up shared services that are used by the data science teams on their team accounts. The services include a Service Catalog portfolio with products for SageMaker domain deployment, SageMaker domain user profile deployment, data science model templates for model building and deploying. This module has functionalities for a centralized model registry, model cards, model dashboard, and the CI/CD pipelines used to orchestrate and automate model development and deployment workflows.
In addition, this module details how to implement the controls and governance required to enable persona-based self-service capabilities, allowing data science teams to independently deploy their required cloud infrastructure and ML templates.
ML use case development
This module helps LOBs and data scientists access their team’s SageMaker domain in a development environment and instantiate a model building template to develop their models. In this module, data scientists work on a dev account instance of the template to interact with the data available on the centralized data lake, reuse and share features from a central feature store, create and run ML experiments, build and test their ML workflows, and register their models to a dev account model registry in their development environments.
Capabilities such as experiment tracking, model explainability reports, data and model bias monitoring, and model registry are also implemented in the templates, allowing for rapid adaptation of the solutions to the data scientists’ developed models.
ML operations
This module helps LOBs and ML engineers work on their dev instances of the model deployment template. After the candidate model is registered and approved, they set up CI/CD pipelines and run ML workflows in the team’s test environment, which registers the model into the central model registry running in a platform shared services account. When a model is approved in the central model registry, this triggers a CI/CD pipeline to deploy the model into the team’s production environment.
Centralized feature store
After the first models are deployed to production and multiple use cases start to share features created from the same data, a feature store becomes essential to ensure collaboration across use cases and reduce duplicate work. This module helps the ML platform engineering team set up a centralized feature store to provide storage and governance for ML features created by the ML use cases, enabling feature reuse across projects.
Logging and observability
This module helps LOBs and ML practitioners gain visibility into the state of ML workloads across ML environments through centralization of log activity such as CloudTrail, CloudWatch, VPC flow logs, and ML workload logs. Teams can filter, query, and visualize logs for analysis, which can help enhance security posture as well.
Cost and reporting
This module helps various stakeholders (cloud admin, platform admin, cloud business office) to generate reports and dashboards to break down costs at ML user, ML team, and ML product levels, and track usage such as number of users, instance types, and endpoints.
Customers have asked us to provide guidance on how many accounts to create and how to structure those accounts. In the next section, we provide guidance on that account structure as reference that you can modify to suit your needs according to your enterprise governance requirements.
Reference account structure
In this section, we discuss our recommendation for organizing your account structure. We share a baseline reference account structure; however, we recommend ML and data admins work closely with their cloud admin to customize this account structure based on their organization controls.
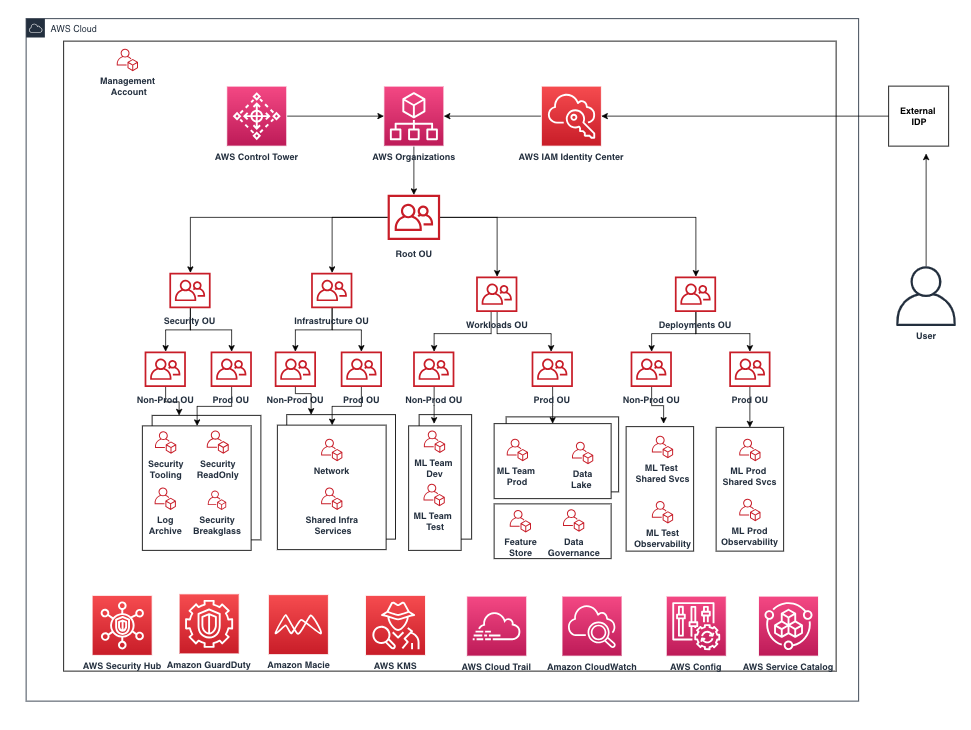
We recommend organizing accounts by OU for security, infrastructure, workloads, and deployments. Furthermore, within each OU, organize by non-production and production OU because the accounts and workloads deployed under them have different controls. Next, we briefly discuss those OUs.
Security OU
The accounts in this OU are managed by the organization’s cloud admin or security team for monitoring, identifying, protecting, detecting, and responding to security events.
Infrastructure OU
The accounts in this OU are managed by the organization’s cloud admin or network team for managing enterprise-level infrastructure shared resources and networks.
We recommend having the following accounts under the infrastructure OU:
- Network – Set up a centralized networking infrastructure such as AWS Transit Gateway
- Shared services – Set up centralized AD services and VPC endpoints
Workloads OU
The accounts in this OU are managed by the organization’s platform team admins. If you need different controls implemented for each platform team, you can nest other levels of OU for that purpose, such as an ML workloads OU, data workloads OU, and so on.
We recommend the following accounts under the workloads OU:
- Team-level ML dev, test, and prod accounts – Set this up based on your workload isolation requirements
- Data lake accounts – Partition accounts by your data domain
- Central data governance account – Centralize your data access policies
- Central feature store account – Centralize features for sharing across teams
Deployments OU
The accounts in this OU are managed by the organization’s platform team admins for deploying workloads and observability.
We recommend the following accounts under the deployments OU because the ML platform team can set up different sets of controls at this OU level to manage and govern deployments:
- ML shared services accounts for test and prod – Hosts platform shared services CI/CD and model registry
- ML observability accounts for test and prod – Hosts CloudWatch logs, CloudTrail logs, and other logs as needed
Next, we briefly discuss organization controls that need to be considered for embedding into member accounts for monitoring the infrastructure resources.
AWS environment controls
A control is a high-level rule that provides ongoing governance for your overall AWS environment. It’s expressed in plain language. In this framework, we use AWS Control Tower to implement the following controls that help you govern your resources and monitor compliance across groups of AWS accounts:
- Preventive controls – A preventive control ensures that your accounts maintain compliance because it disallows actions that lead to policy violations and are implemented using a Service Control Policy (SCP). For example, you can set a preventive control that ensures that CloudTrail is not deleted or stopped in AWS accounts or Regions.
- Detective controls – A detective control detects noncompliance of resources within your accounts, such as policy violations, provides alerts through the dashboard, and is implemented using AWS Config rules. For example, you can create a detective control to detects whether public read access is enabled to the Amazon Simple Storage Service (Amazon S3) buckets in the log archive shared account.
- Proactive controls – A proactive control scans your resources before they are provisioned and makes sure that the resources are compliant with that control and are implemented using AWS CloudFormation hooks. Resources that aren’t compliant will not be provisioned. For example, you can set a proactive control that checks that direct internet access is not allowed for a SageMaker notebook instance.
Interactions between ML platform services, ML use cases, and ML operations
Different personas, such as the head of data science (lead data scientist), data scientist, and ML engineer, operate modules 2–6 as shown in the following diagram for different stages of ML platform services, ML use case development, and ML operations along with data lake foundations and the central feature store.
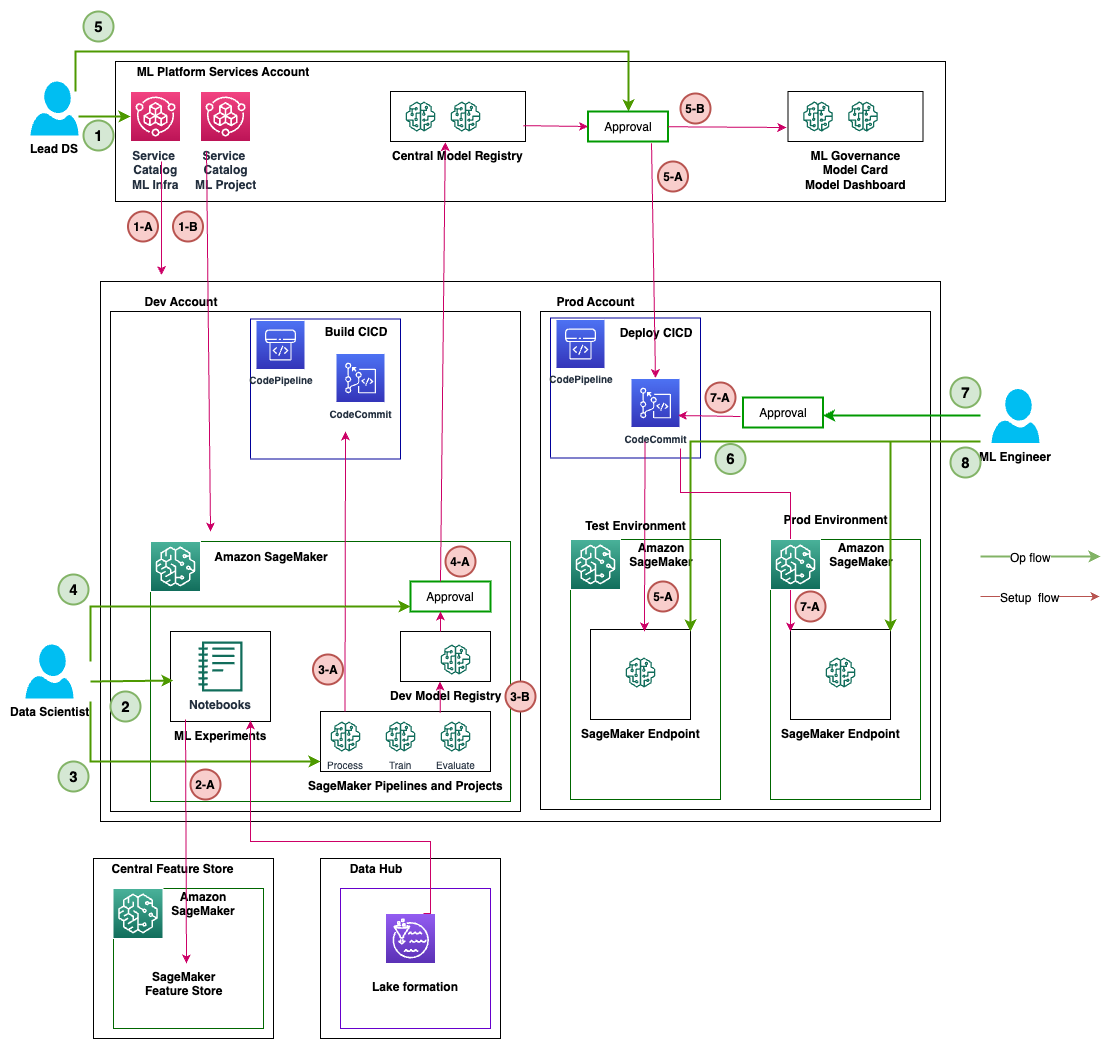
The following table summarizes the ops flow activity and setup flow steps for different personas. Once a persona initiates a ML activity as part of ops flow, the services run as mentioned in setup flow steps.
Persona |
Ops Flow Activity – Number |
Ops Flow Activity – Description |
Setup Flow Step – Number |
Setup Flow Step – Description |
Lead Data Science or ML Team Lead |
1
|
Uses Service Catalog in the ML platform services account and deploys the following:
-
- ML infrastructure
- SageMaker projects
- SageMaker model registry
|
1-A
|
- Sets up the dev, test, and prod environments for LOBs
- Sets up SageMaker Studio in the ML platform services account
|
1-B
|
- Sets up SageMaker Studio with the required configuration
|
Data Scientist |
2
|
Conducts and tracks ML experiments in SageMaker notebooks |
2-A
|
- Uses data from Lake Formation
- Saves features in the central feature store
|
3
|
Automates successful ML experiments with SageMaker projects and pipelines |
3-A
|
-
- Initiates SageMaker pipelines (preprocess, train, evaluate) in the dev account
- Initiates the build CI/CD process with CodePipeline in the dev account
|
3-B
|
After the SageMaker pipelines run, saves the model in the local (dev) model registry |
Lead Data Scientist or ML Team Lead |
4
|
Approves the model in the local (dev) model registry |
4-A
|
Model metadata and model package writes from the local (dev) model registry to the central model registry |
5
|
Approves the model in the central model registry |
5-A
|
Initiates the deployment CI/CD process to create SageMaker endpoints in the test environment |
5-B
|
Writes the model information and metadata to the ML governance module (model card, model dashboard) in the ML platform services account from the local (dev) account |
ML Engineer |
6
|
Tests and monitors the SageMaker endpoint in the test environment after CI/CD |
. |
7
|
Approves deployment for SageMaker endpoints in the prod environment |
7-A
|
Initiates the deployment CI/CD process to create SageMaker endpoints in the prod environment |
8
|
Tests and monitors the SageMaker endpoint in the test environment after CI/CD |
. |
Personas and interactions with different modules of the ML platform
Each module caters to particular target personas within specific divisions that utilize the module most often, granting them primary access. Secondary access is then permitted to other divisions that require occasional use of the modules. The modules are tailored towards the needs of particular job roles or personas to optimize functionality.
We discuss the following teams:
- Central cloud engineering – This team operates at the enterprise cloud level across all workloads for setting up common cloud infrastructure services, such as setting up enterprise-level networking, identity, permissions, and account management
- Data platform engineering – This team manages enterprise data lakes, data collection, data curation, and data governance
- ML platform engineering – This team operates at the ML platform level across LOBs to provide shared ML infrastructure services such as ML infrastructure provisioning, experiment tracking, model governance, deployment, and observability
The following table details which divisions have primary and secondary access for each module according to the module’s target personas.
Module Number |
Modules |
Primary Access |
Secondary Access |
Target Personas |
Number of accounts |
1
|
Multi-account foundations |
Central cloud engineering |
Individual LOBs |
- Cloud admin
- Cloud engineers
|
Few |
2
|
Data lake foundations |
Central cloud or data platform engineering |
Individual LOBs |
- Data lake admin
- Data engineers
|
Multiple |
3
|
ML platform services |
Central cloud or ML platform engineering |
Individual LOBs |
- ML platform Admin
- ML team Lead
- ML engineers
- ML governance lead
|
One |
4
|
ML use case development |
Individual LOBs |
Central cloud or ML platform engineering |
- Data scientists
- Data engineers
- ML team lead
- ML engineers
|
Multiple |
5
|
ML operations |
Central cloud or ML engineering |
Individual LOBs |
- ML Engineers
- ML team leads
- Data scientists
|
Multiple |
6
|
Centralized feature store |
Central cloud or data engineering |
Individual LOBs |
- Data engineer
- Data scientists
|
One |
7
|
Logging and observability |
Central cloud engineering |
Individual LOBs |
|
One |
8
|
Cost and reporting |
Individual LOBs |
Central platform engineering |
- LOB executives
- ML managers
|
One |
Conclusion
In this post, we introduced a framework for governing the ML lifecycle at scale that helps you implement well-architected ML workloads embedding security and governance controls. We discussed how this framework takes a holistic approach for building an ML platform considering data governance, model governance, and enterprise-level controls. We encourage you to experiment with the framework and concepts introduced in this post and share your feedback.
About the authors
Ram Vittal is a Principal ML Solutions Architect at AWS. He has over 3 decades of experience architecting and building distributed, hybrid, and cloud applications. He is passionate about building secure, scalable, reliable AI/ML and big data solutions to help enterprise customers with their cloud adoption and optimization journey to improve their business outcomes. In his spare time, he rides motorcycle and walks with his three-year old sheep-a-doodle!
Sovik Kumar Nath is an AI/ML solution architect with AWS. He has extensive experience designing end-to-end machine learning and business analytics solutions in finance, operations, marketing, healthcare, supply chain management, and IoT. Sovik has published articles and holds a patent in ML model monitoring. He has double masters degrees from the University of South Florida, University of Fribourg, Switzerland, and a bachelors degree from the Indian Institute of Technology, Kharagpur. Outside of work, Sovik enjoys traveling, taking ferry rides, and watching movies.
Maira Ladeira Tanke is a Senior Data Specialist at AWS. As a technical lead, she helps customers accelerate their achievement of business value through emerging technology and innovative solutions. Maira has been with AWS since January 2020. Prior to that, she worked as a data scientist in multiple industries focusing on achieving business value from data. In her free time, Maira enjoys traveling and spending time with her family someplace warm.
Ryan Lempka is a Senior Solutions Architect at Amazon Web Services, where he helps his customers work backwards from business objectives to develop solutions on AWS. He has deep experience in business strategy, IT systems management, and data science. Ryan is dedicated to being a lifelong learner, and enjoys challenging himself every day to learn something new.
Sriharsh Adari is a Senior Solutions Architect at Amazon Web Services (AWS), where he helps customers work backwards from business outcomes to develop innovative solutions on AWS. Over the years, he has helped multiple customers on data platform transformations across industry verticals. His core area of expertise include Technology Strategy, Data Analytics, and Data Science. In his spare time, he enjoys playing sports, binge-watching TV shows, and playing Tabla.
Read More