Amazon researchers optimize the distributed-training tool to run efficiently on the Elastic Fabric Adapter network interface.Read More
NVIDIA Omniverse Upgrade Delivers Extraordinary Benefits to 3D Content Creators
At GTC, NVIDIA announced significant updates for millions of creators using the NVIDIA Omniverse real-time 3D design collaboration platform.
The announcements kicked off with updates to the Omniverse apps Create, Machinima and Showroom, with an immement View release. Powered by GeForce RTX and NVIDIA RTX GPUs, they dramatically accelerate 3D creative workflows.
New Omniverse Connections are expanding the ecosystem and are now available in beta: Unreal Engine 5 Omniverse Connector and the Adobe Substance 3D Material Extension, with the Adobe Substance 3D Painter Omniverse Connector very close behind.
Maxon’s Cinema 4D now has Universal Scene Description (USD) support. Unlocking Cinema 4D workflows via OmniDrive brings deeper integration and flexibility to the Omniverse ecosystem.
Leveraging the hydra render delegate feature, artists can now use Pixar HDStorm, Chaos V-Ray, Maxon Redshift and OTOY Octane Hydra render delegates within the viewport of all Omniverse apps, with Blender Cycles coming soon.
Whether refining 3D scenes or exporting final projects, artists can switch between the lightning-fast Omniverse RTX Renderer or their preferred renderer, giving them ultimate freedom to create however they like.
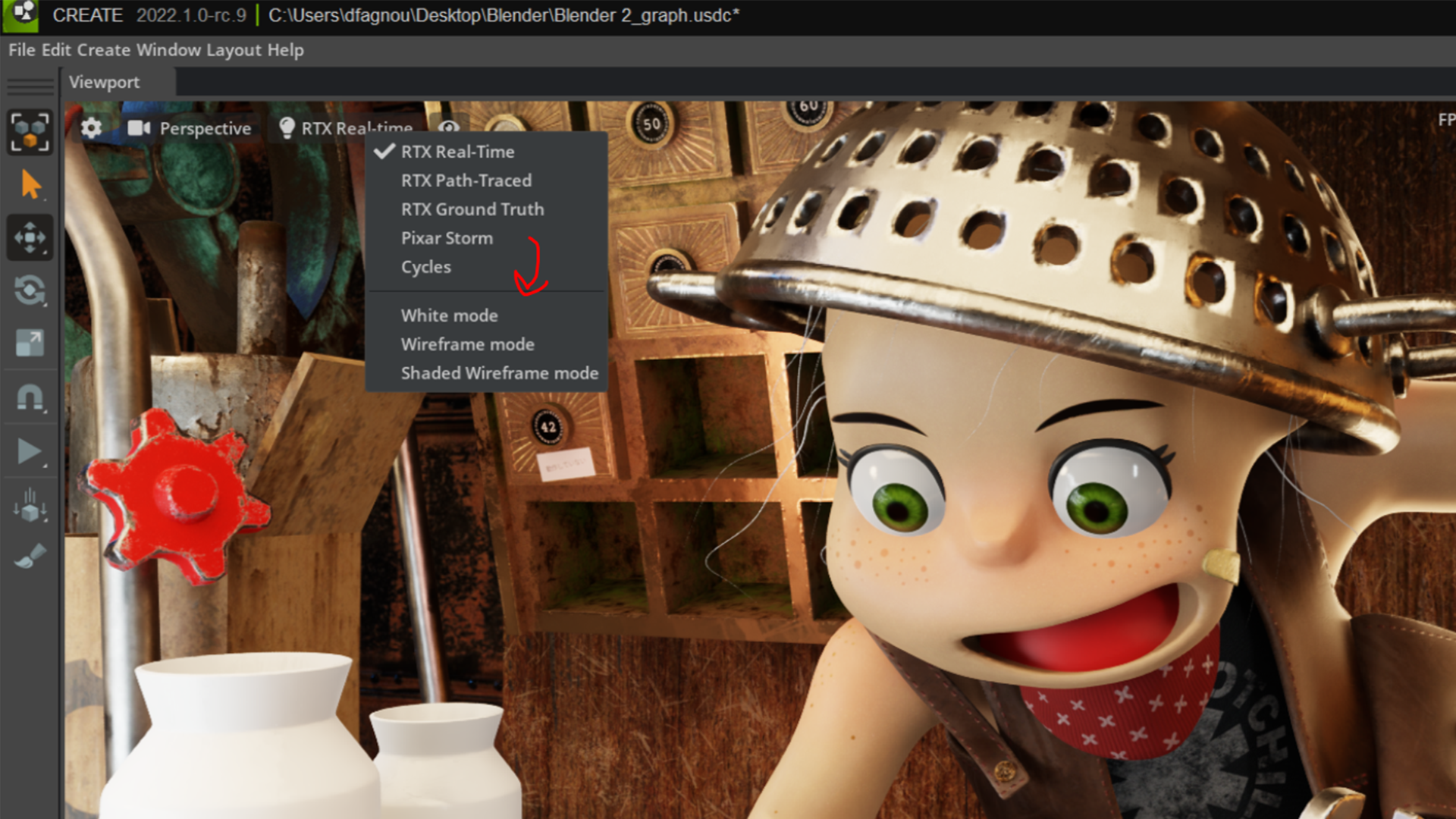
These updates and more are available today in the Omniverse launcher, free to download, alongside the March NVIDIA Studio Driver release.
To celebrate the Machinima app update, we’re kicking off the #MadeInMachinima contest, in which artists can remix iconic characters from Squad, Mount & Blade II: Bannerlord and Mechwarrior 5 into a cinematic short in Omniverse Machinima to win NVIDIA Studio laptops. The submission window opens on March 29 and runs through June 27. Visit the contest landing page for details.
Can’t Wait to Create
Omniverse Create allows users to interactively assemble full-fidelity scenes by connecting to their favorite creative apps. Artists can add lighting, simulate physically accurate scenes and choose to render with Omniverse’s advanced RTX Renderer, or their favorite Hydra Render delegate.
Create version 2022.1 includes USD support for NURBS curves, a type of curve modeling useful for hair, particles and more. Scenes can now be rendered in passes with arbitrary output variables, or AOVs, delivering more control to artists during the compositing stage.
Animation curve editing is now possible with the addition of a graph editor. The feature helps animators feel comfortable working in creative apps such as Autodesk Maya and Blender. They can iterate simpler, faster and more intuitively.
The new ActionGraph feature unlocks keyboard shortcuts and user-interface buttons to trigger complex events simultaneously.
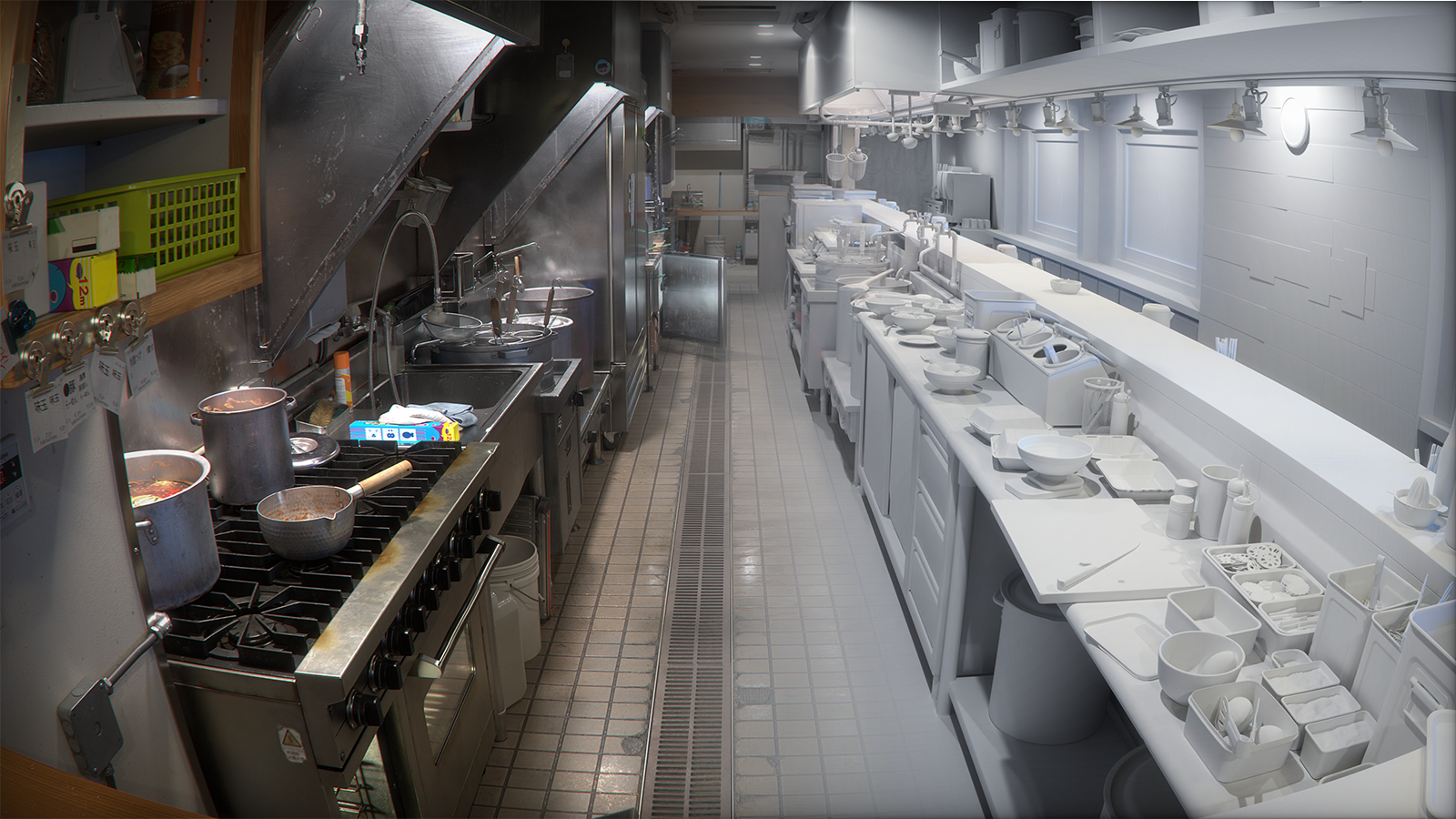
NVIDIA PhysX 5.0 updates provide soft and deformable body support for objects such as fabric, jelly and balloons, adding further realism to scenes with no animation necessary.
VMaterials 2.0, a curated collection of MDL materials and lights, now has over 900 physical materials for artists to apply physically accurate, real-world materials to their scenes with just a double click, no shader writing necessary.
Several new Create features are also available in beta:
- AnimGraph based on OmniGraph brings characters to life with a new graph editor for simple, no-code, realistic animations.
- New animation retargeting allows artists to map animations from one character to another, automating complex animation tasks such as joint mapping, reference post matching and previewing. When used with AnimGraph, artists can automate character rigging, saving artists countless hours of manual, tedious work.
- Users can drag and drop assets they own, or click on others to purchase directly from the asset’s product page. Nearly 1 million assets from TurboSquid by Shutterstock, Sketchfab and Reallusion ActorCore are directly searchable in the Omniverse asset browser.
This otherworldly set of features is Create-ing infectious excitement for 3D workflows.
Machinima Magic
Omniverse Machinima 2022.1 beta provides tools for artists to remix, recreate and redefine animated video game storytelling through immersive visualization, collaborative design and photorealistic rendering.
The integration of NVIDIA Maxine’s body pose estimation feature gives users the ability to track and capture motion in real time using a single camera — without requiring a MoCap suit — with live conversion from a 2D camera capture to a 3D model.
Prerecorded videos can now be converted to animations with a new easy-to-use interface.
The retargeting feature applies these captured animations to custom-built skeletons, providing an easy way to animate a character with a webcam. No fancy, expensive device necessary, just a webcam.
Sequencer functionality updates include a new user interface for easier navigation; new tools including splitting, looping, hold and scale; more drag-and-drop functionality to simplify pipelines; and a new audio graph display.
Stitching and building cinematics is now as intuitive as editing video projects.
Step Into the Showroom
Omniverse Showroom 2022.1 includes seven new scenes that invite the newest of users to get started and embrace the incredible possibilities and technology within the platform.
Artists can engage with tech demos showcasing PhysX, rigid and soft body dynamics, flow, combustible fluid, smoke and fire, and blast, featuring destruction and fractures.
Enjoy the View
Omniverse View 2022.1 will enable non-technical project reviewers to collaboratively and interactively review 3D design projects in stunning photorealism, with several astonishing new features.
Markup gives artists the ability to add 2D feedback based on their viewpoint, including shapes and scribbles, for 3D feedback in the cloud.
Turntable places an interactive scene on a virtual table that can be rotated to see how realistic lighting conditions affect the scene in real time, advantageous for high-end movie production and architects.
Teleport and Waypoints allow artists to easily jump around their scenes and preset fully interactive views of Omniverse scenes for sharing.
Omniverse Ecosystem Expansion Continues
New beta Omniverse Connectors and extensions add variety and versatility to 3D creative workflows.
Now available, an Omniverse Connector for Unreal Engine 5 allows live-sync workflows.
The Adobe Substance 3D Material extension is now available, with a beta Substance 3D Painter Omniverse Connector coming soon, enabling artists to achieve more seamless, live-sync texture and material workflows.
Maxon’s Cinema4D now supports USD and is compatible with OmniDrive, unlocking Omniverse workflows for visualization specialists.
Finally, a new CAD importer enables product designers to convert 26 popular CAD formats into Omniverse USD scenes.
More Machinima Magic — With Prizes
The #MadeInMachinima contest asks participants to build scenes and assets — composed of characters from Squad, Mount & Blade II: Bannerlord and Mechwarrior 5 — using Omniverse Machinima.
Legendary Halo Red vs. Blue Studio, Rooster Teeth, produced this magnificent cinematic short in Machinima. Take a look to see what’s possible.
Machinima expertise, while welcome, is not required; this contest is for creators of all levels. Three talented winners will get an NVIDIA Studio laptop, powerful and purpose-built with vivid color displays and blazing-fast memory and storage, to boost future Omniverse sessions.
Machinima will be prominently featured at the Game Developers Conference, where game artists, producers, developers and designers come together to exchange ideas, educate and inspire. At the show, we also launched Omniverse for Developers, providing a more collaborative environment for the creation of virtual worlds.
NVIDIA offers sessions at GDC to assist content creators featuring virtual worlds and AI, real-time ray tracing, and developer tools. Check out the complete list.
Launch or download Omniverse today.
The post NVIDIA Omniverse Upgrade Delivers Extraordinary Benefits to 3D Content Creators appeared first on NVIDIA Blog.
At GTC: NVIDIA RTX Professional Laptop GPUs Debut, New NVIDIA Studio Laptops, a Massive Omniverse Upgrade and NVIDIA Canvas Update
Digital artists and creative professionals have plenty to be excited about at NVIDIA GTC.
Impressive NVIDIA Studio laptop offerings from ASUS and MSI launch with upgraded RTX GPUs, providing more options for professional content creators to elevate and expand creative possibilities.
NVIDIA Omniverse gets a significant upgrade — including updates to the Omniverse Create, Machinima and Showroom apps; with an upcoming, imminent, View release. A new Unreal Engine Omniverse Connector beta is out now with our Adobe Substance 3D Painter Connector close behind.
Omniverse artists can now use Pixar HDStorm, Chaos V-Ray, Maxon Redshift and OTOY Octane Hydra render delegates within the viewport of all Omniverse apps, bringing more freedom and choice to 3D creative workflows, with Blender Cycles coming soon. Read our Omniverse blog for more details.
NVIDIA Canvas, the beta app sensation using advanced AI to quickly turn simple brushstrokes into realistic landscape images, has received a stylish update.
The March Studio Driver, available for download today, optimizes the latest creative app updates, featuring Blender Cycles 3.1, all with the stability and reliability NVIDIA Studio delivers.
To celebrate, NVIDIA is kicking off the #MadeInMachinima contest. Artists can remix iconic characters from Squad, Mount & Blade II: Bannerlord and Mechwarrior 5 into a cinematic short in Omniverse Machinima to win NVIDIA Studio laptops. The submission window opens on March 29 and runs through June 27. Visit the contest landing page for details.
New NVIDIA RTX Laptop GPUs Unlock Endless Creative Possibilities
Professionals on the go have powerful new laptop GPUs to choose from, with faster speeds and larger memory options: RTX A5500, RTX A4500 , RTX A3000 12GB, RTX A2000 8GB and NVIDIA RTX A1000. These GPUs incorporate the latest RTX and Max-Q technology, are available in thin and light laptops, and deliver extraordinary performance.
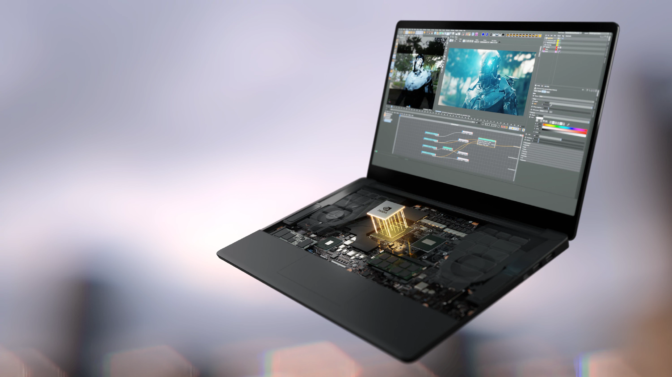
Our new flagship laptop GPU, the NVIDIA RTX A5500 with 16GB of memory, is capable of handling the most challenging 3D and video workloads; with up to double the rendering performance of the previous generation RTX 5000.
The most complex, advanced, creative workflows have met their match.
NVIDIA Studio Laptop Drop
Three extraordinary Studio laptops are available for purchase today.
The ASUS ProArt Studiobook 16 is capable of incredible performance, and is configurable with a wide-range of professional and consumer GPUs. It’s rich with creative features: certified color-accurate 16-inch 120 Hz 3.2K OLED wide-view 16:10 display, a three-button touchpad for 3D designers, ASUS dial for video editing and an enlarged touchpad for stylus support.
MSI’s Creator Z16P and Z17 sport an elegant and minimalist design, featuring up to an NVIDIA RTX 3080 Ti or RTX A5500 GPU, and boast a factory-calibrated True Pixel display with QHD+ resolution and 100 percent DCI-P3 color.
NVIDIA Studio laptops are tested and validated for maximum performance and reliability. They feature the latest NVIDIA technologies that deliver real-time ray tracing, AI-enhanced features and time-saving rendering capabilities. These laptops have access to the exclusive Studio suite of software — including best-in-class Studio Drivers, NVIDIA Omniverse, Canvas, Broadcast and more.
In the weeks ahead, ASUS and GIGABYTE will make it even easier for new laptop owners to enjoy one of the Studio benefits. Upgraded livestreams, voice chats and video calls — powered by AI — will be available immediately with the NVIDIA Broadcast app preinstalled in their Pro Art and AERO product lines.
To Omniverse and Beyond
New Omniverse Connections are expanding the ecosystem and are now available in beta: Unreal Engine 5 Omniverse Connector and the Adobe Substance 3D Material Extension, with the Adobe Substance 3D Painter Omniverse Connector very close behind, allowing users to enjoy seamless, live-edit texture and material workflows.
Maxon’s Cinema4D now supports USD and is compatible with OmniDrive, unlocking Omniverse workflows for visualization specialists.
Artists can now use Pixar HD Storm, Chaos V-Ray, Maxon Redshift and OTOY Octane renderers within the viewport of all Omniverse apps, with Blender Cycles coming soon. Be it refining 3D scenes or exporting final projects, artists can switch between the lightning-fast Omniverse RTX Renderer, or their preferred renderer with advantageous features.
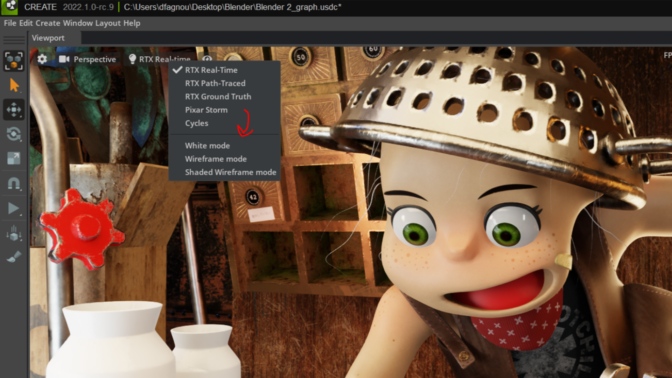
CAD designers can now directly import 26 popular CAD formats into Omniverse USD scenes.
The integration of NVIDIA Maxine’s body pose estimation feature in the Omniverse Machinima app gives users the ability to track and capture motion in real time using a single camera — without requiring a MoCap suit — with live conversion from a 2D camera capture to a 3D model.
Read more about Omniverse for content creators here.
And if you haven’t downloaded Omniverse, now’s the time.
Your Canvas, Never Out of Style
Styles in Canvas — preset filters that modify the look and feel of the painting — can now be modified in up to 10 different variations.
More style variations enhance artist creativity while providing additional options within the theme of their selected style.
Check out style variations; and if you haven’t already, download Canvas, which is free for RTX owners.
3D Creative App Updates Backed by March NVIDIA Studio Driver
In addition to supporting the latest updates for NVIDIA Omniverse and NVIDIA Canvas, the March Studio Driver also supports a host of other recent creative app and renderer updates.
The highly anticipated Blender 3.1 update adds USD preview surface material export support, making it easier to move assets between USD-supported apps, including Omniverse.
Blender artists equipped with NVIDIA RTX GPUs maintain performance advantages over Mac. Midrange GeForce RTX 3060 Studio laptops deliver 3.5x faster rendering than the fastest M1 Max Macbooks per Blender’s benchmark testing.
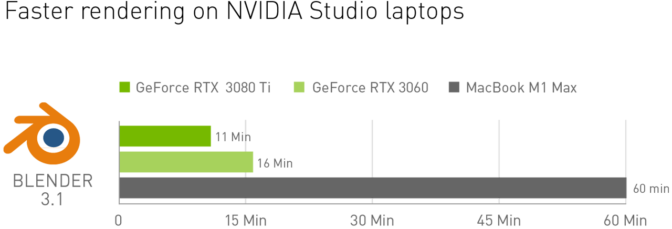
Luxion Keyshot 11 brings several updates: GPU-accelerated 3D paint features, physical simulation using NVIDIA PhysX, and NVIDIA Optix shader enhancements, speeding up animation workflows by up to 3x.
GPU Audio Inc., with an eye on the future, taps into parallel processing power for audio solutions, introducing an NVIDIA GPU-based VST filter to remove extreme frequencies and improve sound quality — an audio production game changer.
Download the March Studio Driver today.
On-Demand Sessions for Creators
Join the first GTC breakout session dedicated to the creative community.
“NVIDIA Studio and Omniverse for the Next Era of Creativity” will include artists and directors from NVIDIA’s creative team. Network with fellow 3D artists and get Omniverse feature support to enhance 3D workflows. Join this free session on Wednesday, March 23, from 7-8 a.m. Pacific.
It’s just one of many Omniverse sessions available to watch live or on demand, including the featured sessions below:
- Creating a Real-Time, Photoreal Ramen Shop in Omniverse with Gabriele Leone, senior art director at NVIDIA, and Andrew Averkin, senior environmental artist at NVIDIA.
- New Approach to Fashion Design With Adobe Substance 3D & Omniverse with Rob Bryant, creative 3D artist at NVIDIA.
- A Primer on Materials for NVIDIA Omniverse with Jan Jordan, senior software product manager for MDL at NVIDIA.
Themed GTC sessions and demos covering visual effects, virtual production and rendering, AI art galleries, and building and infrastructure design are also available to help realize your creative ambition.
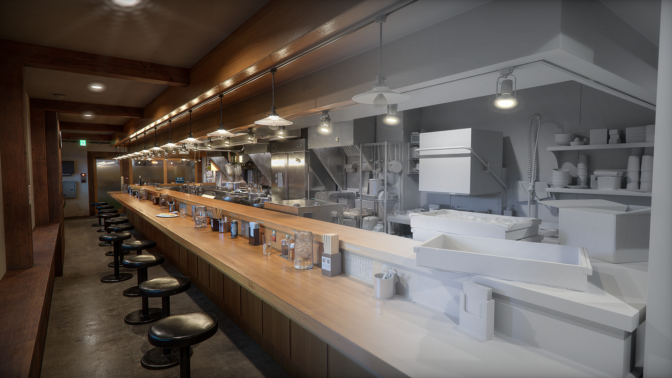
Also this week, game artists, producers, developers and designers are coming together for the annual Game Developers Conference where NVIDIA launched Omniverse for Developers, providing a more collaborative environment for the creation of virtual worlds.
At GDC, NVIDIA sessions to assist content creators in the gaming industry will feature virtual worlds and AI, real-time ray tracing, and developer tools. Check out the complete list.
To boost your creativity throughout the year, follow NVIDIA Studio on Facebook, Twitter and Instagram. There you’ll find the latest information on creative app updates, new Studio apps, creator contests and more. Get updates directly to your inbox by subscribing to the Studio newsletter.
The post At GTC: NVIDIA RTX Professional Laptop GPUs Debut, New NVIDIA Studio Laptops, a Massive Omniverse Upgrade and NVIDIA Canvas Update appeared first on NVIDIA Blog.
Powering the next generation of trustworthy AI in a confidential cloud using NVIDIA GPUs
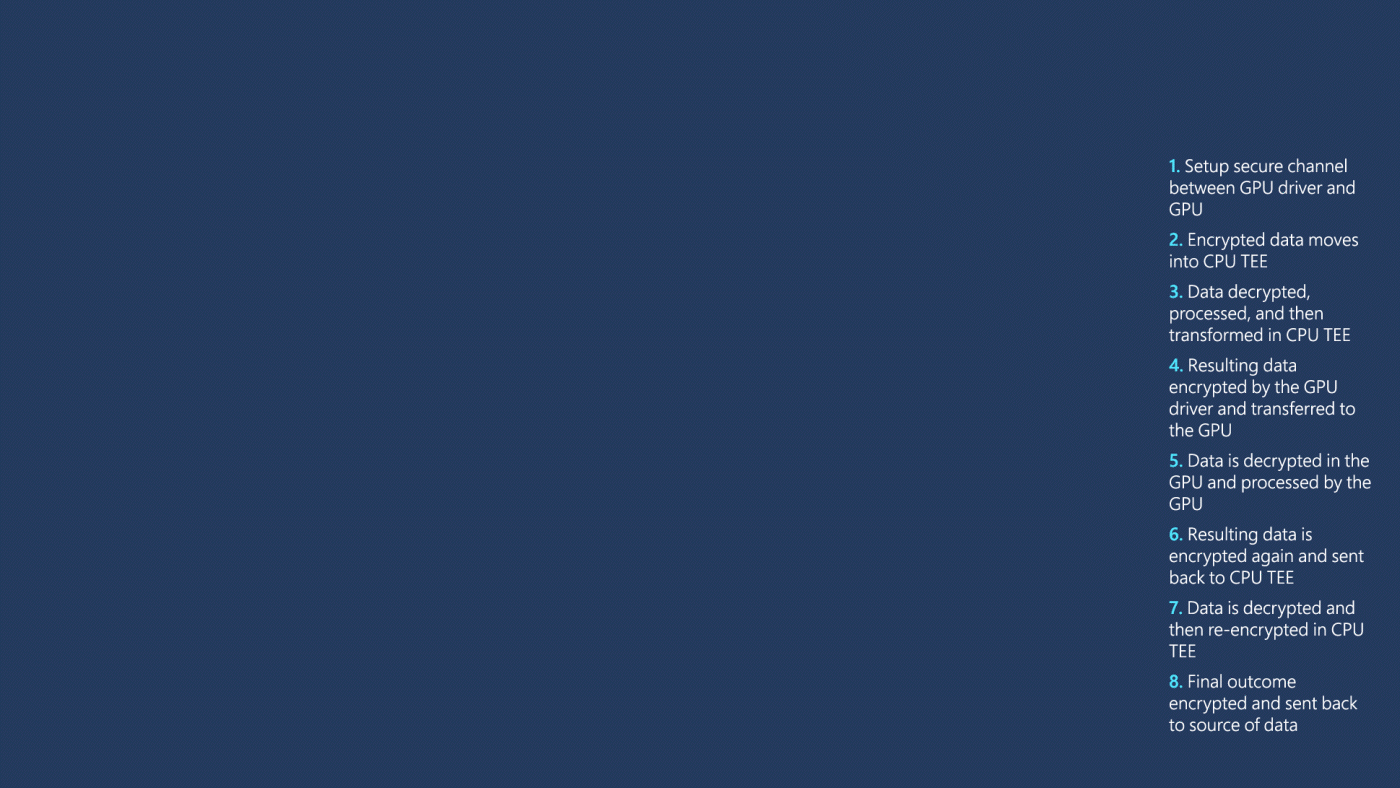
Cloud computing is powering a new age of data and AI by democratizing access to scalable compute, storage, and networking infrastructure and services. Thanks to the cloud, organizations can now collect data at an unprecedented scale and use it to train complex models and generate insights.
While this increasing demand for data has unlocked new possibilities, it also raises concerns about privacy and security, especially in regulated industries such as government, finance, and healthcare. One area where data privacy is crucial is patient records, which are used to train models to aid clinicians in diagnosis. Another example is in banking, where models that evaluate borrower creditworthiness are built from increasingly rich datasets, such as bank statements, tax returns, and even social media profiles. This data contains very personal information, and to ensure that it’s kept private, governments and regulatory bodies are implementing strong privacy laws and regulations to govern the use and sharing of data for AI, such as the General Data Protection Regulation (GDPR) and the proposed EU AI Act. You can learn more about some of the industries where it’s imperative to protect sensitive data in this Microsoft Azure Blog post.
Commitment to a confidential cloud
Microsoft recognizes that trustworthy AI requires a trustworthy cloud—one in which security, privacy, and transparency are built into its core. A key component of this vision is confidential computing—a set of hardware and software capabilities that give data owners technical and verifiable control over how their data is shared and used. Confidential computing relies on a new hardware abstraction called trusted execution environments (TEEs). In TEEs, data remains encrypted not just at rest or during transit, but also during use. TEEs also support remote attestation, which enables data owners to remotely verify the configuration of the hardware and firmware supporting a TEE and grant specific algorithms access to their data.
At Microsoft, we are committed to providing a confidential cloud, where confidential computing is the default for all cloud services. Today, Azure offers a rich confidential computing platform comprising different kinds of confidential computing hardware (Intel SGX, AMD SEV-SNP), core confidential computing services like Azure Attestation and Azure Key Vault managed HSM, and application-level services such as Azure SQL Always Encrypted, Azure confidential ledger, and confidential containers on Azure. However, these offerings are limited to using CPUs. This poses a challenge for AI workloads, which rely heavily on AI accelerators like GPUs to provide the performance needed to process large amounts of data and train complex models.
-
Publication
Graviton: Trusted Execution Environments on GPUs
The Confidential Computing group at Microsoft Research identified this problem and defined a vision for confidential AI powered by confidential GPUs, proposed in two papers, “Oblivious Multi-Party Machine Learning on Trusted Processors” and “Graviton: Trusted Execution Environments on GPUs.” In this post, we share this vision. We also take a deep dive into the NVIDIA GPU technology that’s helping us realize this vision, and we discuss the collaboration among NVIDIA, Microsoft Research, and Azure that enabled NVIDIA GPUs to become a part of the Azure confidential computing ecosystem.
Vision for confidential GPUs
Today, CPUs from companies like Intel and AMD allow the creation of TEEs, which can isolate a process or an entire guest virtual machine (VM), effectively eliminating the host operating system and the hypervisor from the trust boundary. Our vision is to extend this trust boundary to GPUs, allowing code running in the CPU TEE to securely offload computation and data to GPUs.
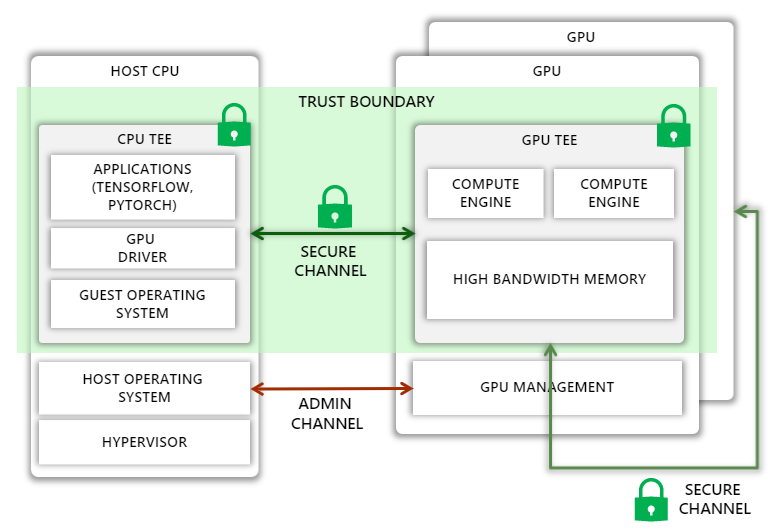
Unfortunately, extending the trust boundary is not straightforward. On the one hand, we must protect against a variety of attacks, such as man-in-the-middle attacks where the attacker can observe or tamper with traffic on the PCIe bus or on a NVIDIA NVLink connecting multiple GPUs, as well as impersonation attacks, where the host assigns an incorrectly configured GPU, a GPU running older versions or malicious firmware, or one without confidential computing support for the guest VM. At the same time, we must ensure that the Azure host operating system has enough control over the GPU to perform administrative tasks. Furthermore, the added protection must not introduce large performance overheads, increase thermal design power, or require significant changes to the GPU microarchitecture.
Our research shows that this vision can be realized by extending the GPU with the following capabilities:
- A new mode where all sensitive state on the GPU, including GPU memory, is isolated from the host
- A hardware root-of-trust on the GPU chip that can generate verifiable attestations capturing all security sensitive state of the GPU, including all firmware and microcode
- Extensions to the GPU driver to verify GPU attestations, set up a secure communication channel with the GPU, and transparently encrypt all communications between the CPU and GPU
- Hardware support to transparently encrypt all GPU-GPU communications over NVLink
- Support in the guest operating system and hypervisor to securely attach GPUs to a CPU TEE, even if the contents of the CPU TEE are encrypted
Confidential computing with NVIDIA A100 Tensor Core GPUs
NVIDIA and Azure have taken a significant step toward realizing this vision with a new feature called Ampere Protected Memory (APM) in the NVIDIA A100 Tensor Core GPUs. In this section, we describe how APM supports confidential computing within the A100 GPU to achieve end-to-end data confidentiality.
APM introduces a new confidential mode of execution in the A100 GPU. When the GPU is initialized in this mode, the GPU designates a region in high-bandwidth memory (HBM) as protected and helps prevent leaks through memory-mapped I/O (MMIO) access into this region from the host and peer GPUs. Only authenticated and encrypted traffic is permitted to and from the region.
In confidential mode, the GPU can be paired with any external entity, such as a TEE on the host CPU. To enable this pairing, the GPU includes a hardware root-of-trust (HRoT). NVIDIA provisions the HRoT with a unique identity and a corresponding certificate created during manufacturing. The HRoT also implements authenticated and measured boot by measuring the firmware of the GPU as well as that of other microcontrollers on the GPU, including a security microcontroller called SEC2. SEC2, in turn, can generate attestation reports that include these measurements and that are signed by a fresh attestation key, which is endorsed by the unique device key. These reports can be used by any external entity to verify that the GPU is in confidential mode and running last known good firmware.
When the NVIDIA GPU driver in the CPU TEE loads, it checks whether the GPU is in confidential mode. If so, the driver requests an attestation report and checks that the GPU is a genuine NVIDIA GPU running known good firmware. Once confirmed, the driver establishes a secure channel with the SEC2 microcontroller on the GPU using the Security Protocol and Data Model (SPDM)-backed Diffie-Hellman-based key exchange protocol to establish a fresh session key. When that exchange completes, both the GPU driver and SEC2 hold the same symmetric session key.
The GPU driver uses the shared session key to encrypt all subsequent data transfers to and from the GPU. Because pages allocated to the CPU TEE are encrypted in memory and not readable by the GPU DMA engines, the GPU driver allocates pages outside the CPU TEE and writes encrypted data to those pages. On the GPU side, the SEC2 microcontroller is responsible for decrypting the encrypted data transferred from the CPU and copying it to the protected region. Once the data is in high bandwidth memory (HBM) in cleartext, the GPU kernels can freely use it for computation.
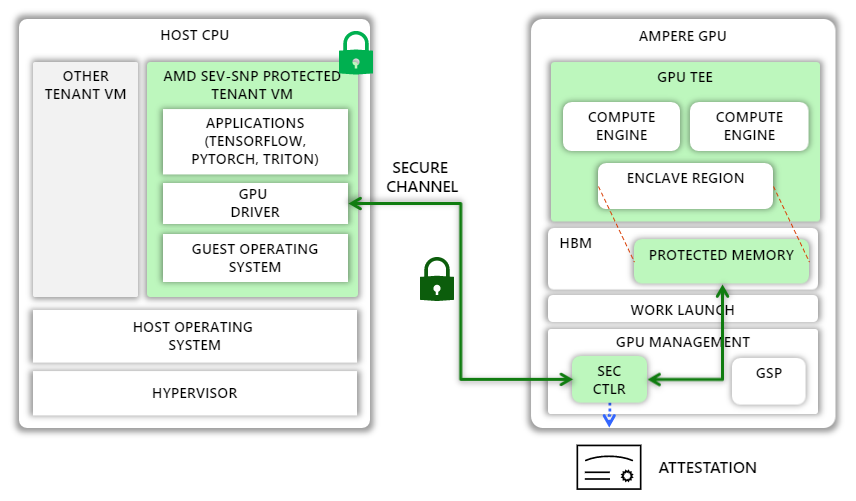
Accelerating innovation with confidential AI
The implementation of APM is an important milestone toward achieving broader adoption of confidential AI in the cloud and beyond. APM is the foundational building block of Azure Confidential GPU VMs, now in private preview. These VMs, designed in collaboration with NVIDIA, Azure, and Microsoft Research, feature up to four A100 GPUs with 80 GB of HBM and APM technology and enable users to host AI workloads on Azure with a new level of security.
But this is just the beginning. We look forward to taking our collaboration with NVIDIA to the next level with NVIDIA’s Hopper architecture, which will enable customers to protect both the confidentiality and integrity of data and AI models in use. We believe that confidential GPUs can enable a confidential AI platform where multiple organizations can collaborate to train and deploy AI models by pooling together sensitive datasets while remaining in full control of their data and models. Such a platform can unlock the value of large amounts of data while preserving data privacy, giving organizations the opportunity to drive innovation.
A real-world example involves Bosch Research, the research and advanced engineering division of Bosch, which is developing an AI pipeline to train models for autonomous driving. Much of the data it uses includes personal identifiable information (PII), such as license plate numbers and people’s faces. At the same time, it must comply with GDPR, which requires a legal basis for processing PII, namely, consent from data subjects or legitimate interest. The former is challenging because it is practically impossible to get consent from pedestrians and drivers recorded by test cars. Relying on legitimate interest is challenging too because, among other things, it requires showing that there is a no less privacy-intrusive way of achieving the same result. This is where confidential AI shines: Using confidential computing can help reduce risks for data subjects and data controllers by limiting exposure of data (for example, to specific algorithms), while enabling organizations to train more accurate models.
At Microsoft Research, we are committed to working with the confidential computing ecosystem, including collaborators like NVIDIA and Bosch Research, to further strengthen security, enable seamless training and deployment of confidential AI models, and help power the next generation of technology.
About confidential computing at Microsoft Research
The Confidential Computing team at Microsoft Research Cambridge conducts pioneering research in system design that aims to guarantee strong security and privacy properties to cloud users. We tackle problems around secure hardware design, cryptographic and security protocols, side channel resilience, and memory safety. We are also interested in new technologies and applications that security and privacy can uncover, such as blockchains and multiparty machine learning. Please visit our careers page to learn about opportunities for both researchers and engineers. We’re hiring.
Related GTC Conference sessions
The post Powering the next generation of trustworthy AI in a confidential cloud using NVIDIA GPUs appeared first on Microsoft Research.
Microsoft Translator enhanced with Z-code Mixture of Experts models

Translator, a Microsoft Azure Cognitive Service, is adopting Z-code Mixture of Experts models, a breakthrough AI technology that significantly improves the quality of production translation models. As a component of Microsoft’s larger XYZ-code initiative to combine AI models for text, vision, audio, and language, Z-code supports the creation of AI systems that can speak, see, hear, and understand. This effort is a part of Azure AI and Project Turing, focusing on building multilingual, large-scale language models that support various production teams. Translator is using NVIDIA GPUs and Triton Inference Server to deploy and scale these models efficiently for high-performance inference. Translator is the first machine translation provider to introduce this technology live for customers.
Z-code MoE boosts efficiency and quality
Z-code models utilize a new architecture called Mixture of Experts (MoE), where different parts of the models can learn different tasks. The models learn to translate between multiple languages at the same time. The Z-code MoE model utilizes more parameters while dynamically selecting which parameters to use for a given input. This enables the model to specialize a subset of the parameters (experts) during training. At runtime, the model uses the relevant experts for the task, which is more computationally efficient than utilizing all model’s parameters.

Newly introduced Z-code MoE models leverage transfer learning, which enables efficient knowledge sharing across similar languages. Moreover, the models utilize both parallel and monolingual data during the training process. This opens the way to high quality machine translation beyond the high-resource languages and improves the quality of low-resource languages that lack significant training data. This approach can provide a positive impact on AI fairness, since both high-resource and low-resource languages see improvements.
We have trained translation systems for research purposes with 200 billion parameters supporting 100 language pairs. Though such large systems significantly improved the translation quality, this also introduced challenges to deploy them in a production environment cost effectively. For our production model deployment, we opted for training a set of 5 billion parameter models, which are 80 times larger than our currently deployed models. We trained a multilingual model per set of languages, where each model can serve up to 20 language pairs and therefore replace up to 20 of the current systems. This enabled our model to maximize the transfer learning among languages while being deployable with effective runtime cost. We compared the quality improvements of the new MoE to the current production system using human evaluation. The figure below shows the results of the models on various language pairs. The Z-code-MoE systems outperformed individual bilingual systems, with average improvements of 4%. For instance, the models improved English to French translations by 3.2 percent, English to Turkish by 5.8 percent, Japanese to English by 7.6 percent, English to Arabic by 9.3 percent, and English to Slovenian by 15 percent.

Training large models with billions of parameters is challenging. The Translator team collaborated with Microsoft DeepSpeed to develop a high-performance system that helped train massive scale Z-code MoE models, enabling us to efficiently scale and deploy Z-code models for translation.
We partnered with NVIDIA to optimize faster engines that can be used at runtime to deploy the new Z-code/MoE models on GPUs. NVIDIA developed custom CUDA kernels and leveraged the CUTLASS and FasterTransformer libraries to efficiently implement MoE layers on a single V100 GPU. This implementation achieved up to 27x throughput improvements over standard GPU (PyTorch) runtimes. We used NVIDIA’s open source Triton Inference Server to serve Z-code MoE models. We used Triton’s dynamic batching feature to pool several requests into a big batch for higher throughput that enabled us to ship large models with relatively low runtime costs.
How can you use the new Z-code models?
Z-code models are available now by invitation to customers using Document Translation, a feature that translates entire documents, or volumes of documents, in a variety of different file formats preserving their original formatting. Z-code models will be made available to all customers and to other Translator products in phases. Please fill out this form to request access to Document Translation using Z-code models.
Learn more
- New Z-code Mixture of Experts models improve quality, efficiency in Translator and Azure AI
- Cognitive Services—APIs for AI Solutions | Microsoft Azure
- Scalable and Efficient MoE Training for Multitask Multilingual Models
- Fast and Scalable AI Model Deployment with NVIDIA Triton Inference Server | NVIDIA Technical Blog
Acknowledgements
The following people contributed to this work: Abdelrahman Abouelenin, Ahmed Salah, Akiko Eriguchi, Alex Cheng, Alex Muzio, Amr Hendy, Arul Menezes, Brad Ballinger, Christophe Poulain, Evram Narouz, Fai Sigalov, Hany Hassan Awadalla, Hitokazu Matsushita, Mohamed Afify, Raffy Bekhit, Rohit Jain, Steven Nguyen, Vikas Raunak, Vishal Chowdhary, and Young Jin Kim.
The post Microsoft Translator enhanced with Z-code Mixture of Experts models appeared first on Microsoft Research.
How Amazon Search achieves low-latency, high-throughput T5 inference with NVIDIA Triton on AWS
Amazon Search’s vision is to enable customers to search effortlessly. Our spelling correction helps you find what you want even if you don’t know the exact spelling of the intended words. In the past, we used classical machine learning (ML) algorithms with manual feature engineering for spelling correction. To make the next generational leap in spelling correction performance, we are embracing a number of deep-learning approaches, including sequence-to-sequence models. Deep learning (DL) models are compute-intensive both in training and inference, and these costs have historically made DL models impractical in a production setting at Amazon’s scale. In this post, we present the results of an inference optimization experimentation where we overcome those obstacles and achieve 534% inference speed-up for the popular Hugging Face T5 Transformer.
Challenge
The Text-to-Text Transfer Transformer (T5, Exploring the Limits of Transfer Learning with a Unified Text-to-Text Transformer, Reffel et al) is the state-of-the-art natural language processing (NLP) model architecture. T5 is a promising architecture for spelling correction, that we found to perform well in our experiments. T5 models are easy to research, develop, and train, thanks to open-source deep learning frameworks and ongoing academic and enterprise research.
However, it’s difficult to achieve production-grade, low-latency inference with a T5. For example, a single inference with a PyTorch T5 takes 45 milliseconds on one of the four NVIDIA V100 Tensor Core GPUs equipping an Amazon Elastic Compute Cloud (EC2) p3.8xlarge instance. (All inference numbers reported are for an input of 9 tokens and output of 11 tokens. The latency of T5 architectures is sensitive to both input and output lengths.)
Low-latency, cost-efficient T5 inference at scale is a known difficulty that has been reported by several AWS customers beyond Amazon Search, which boosts our motivation to contribute this post. To go from an offline, scientific achievement to a customer-facing production service, Amazon Search faces the following challenges:
- Latency – How to realize T5 inference in less than 50-millisecond P99 latency
- Throughput – How to handle large-scale concurrent inference requests
- Cost efficiency – How to keep costs under control
In the rest of this post, we explain how the NVIDIA inference optimization stack—namely the NVIDIA TensorRT compiler and the open source NVIDIA Triton Inference Server—solves those challenges. Read NVIDIA’s press release to learn about the updates.
NVIDIA TensorRT: Reducing costs and latency with inference optimization
Deep learning frameworks are convenient to iterate fast on the science, and come with numerous functionalities for scientific modeling, data loading, and training optimization. However, most of those tools are suboptimal for inference, which only requires a minimal set of operators for matrix multiplication and activation functions. Therefore, significant gains can be realized by using a specialized, prediction-only application instead of running inference in the deep learning development framework.
NVIDIA TensorRT is an SDK for high-performance deep learning inference. TensorRT delivers both an optimized runtime, using low-level optimized kernels available on NVIDIA GPUs, and an inference-only model graph, which rearranges inference computation in an optimized order.
In the following section, we will talk about the details happening behind TensorRT and how it speeds performance.
- Reduced Precision maximizes throughput with FP16 or INT8 by quantizing models while maintaining correctness.
- Layer and Tensor Fusion optimizes use of GPU memory and bandwidth by fusing nodes in a kernel to avoid kernel launch latency.
- Kernel Auto-Tuning selects best data layers and algorithms based on the target GPU platform and data kernel shapes.
- Dynamic Tensor Memory minimizes memory footprint by freeing unnecessary memory consumption of intermediate results and reuses memory for tensors efficiently.
- Multi-Stream Execution uses a scalable design to process multiple input streams in parallel with dedicated CUDA streams.
- Time Fusion optimizes recurrent neural networks over time steps with dynamically generated kernels.
T5 uses transformer layers as building blocks for its architectures. The latest release of NVIDIA TensorRT 8.2 introduces new optimizations for the T5 and GPT-2 models for real-time inference. In the following table, we can see the speedup with TensorRT on some public T5 models running on Amazon EC2G4dn instances, powered by NVIDIA T4 GPUs and EC2 G5 instances, powered by NVIDIA A10G GPUs.
Model | Instance | Baseline Pytorch Latency (ms) | TensorRT 8.2 Latency (ms) | Speedup vs. the HF baseline | ||||||||
FP32 | FP32 | FP16 | FP32 | FP16 | ||||||||
Encoder | Decoder | End to End | Encoder | Decoder | End to End | Encoder | Decoder | End to End | End to End | End to End | ||
t5-small | g4dn.xlarge | 5.98 | 9.74 | 30.71 | 1.28 | 2.25 | 7.54 | 0.93 | 1.59 | 5.91 | 407.40% | 519.34% |
g5.xlarge | 4.63 | 7.56 | 24.22 | 0.61 | 1.05 | 3.99 | 0.47 | 0.80 | 3.19 | 606.66% | 760.01% | |
t5-base | g4dn.xlarge | 11.61 | 19.05 | 78.44 | 3.18 | 5.45 | 19.59 | 3.15 | 2.96 | 13.76 | 400.48% | 569.97% |
g5.xlarge | 8.59 | 14.23 | 59.98 | 1.55 | 2.47 | 11.32 | 1.54 | 1.65 | 8.46 | 530.05% | 709.20% |
For more information about optimizations and replication of the attached performance, refer to Optimizing T5 and GPT-2 for Real-Time Inference with NVIDIA TensorRT.
It is important to note that compilation preserves model accuracy, as it operates on the inference environment and the computation scheduling, leaving the model science unaltered – unlike weight removal compression such as distillation or pruning. NVIDIA TensorRT allows to combine compilation with quantization for further gains. Quantization has double benefits on recent NVIDIA hardware: it reduces memory usage, and enables the use of NVIDIA Tensor Cores, DL-specific cells that run a fused matrix-multiply-add in mixed precision.
In the case of the Amazon Search experimentation with Hugging Face T5 model, replacing PyTorch with TensorRT for model inference increases speed by 534%.
NVIDIA Triton: Low-latency, high-throughput inference serving
Modern model serving solutions can transform offline trained models into customer-facing ML-powered products. To maintain reasonable costs at such a scale, it’s important to keep serving overhead low (HTTP handling, preprocessing and postprocessing, CPU-GPU communication), and fully take advantage of the parallel processing ability of GPUs.
NVIDIA Triton is an inference serving software proposing wide support of model runtimes (NVIDIA TensorRT, ONNX, PyTorch, XGBoost among others) and infrastructure backends, including GPUs, CPU and AWS Inferentia.
ML practitioners love Triton for multiple reasons. Its dynamic batching ability allows to accumulate inference requests during a user-defined delay and within a maximal user-defined batch size, so that GPU inference is batched, amortizing the CPU-GPU communication overhead. Note that dynamic batching happens server-side and within very short time frames, so that the requesting client still has a synchronous, near-real-time invocation experience. Triton users also enjoy its concurrent model execution capacity. GPUs are powerful multitaskers that excel in executing compute-intensive workloads in parallel. Triton maximize the GPU utilization and throughput by using CUDA streams to run multiple model instances concurrently. These model instances can be different models from different frameworks for different use cases, or a direct copy of the same model. This translates to direct throughput improvement when you have enough idle GPU memory. Also, as Triton is not tied to a specific DL development framework, it allows scientist to fully express themselves, in the tool of their choice.
With Triton on AWS, Amazon Search expects to better serve Amazon.com customers and meet latency requirements at low cost. The tight integration between the TensorRT runtime and the Triton server facilitates the development experience. Using AWS cloud infrastructure allows to scale up or down in minutes based on throughput requirements, while maintaining the bar high or reliability and security.
How AWS lowers the barrier to entry
While Amazon Search conducted this experiment on Amazon EC2 infrastructure, other AWS services exist to facilitate the development, training and hosting of state-of-the-art deep learning solutions.
For example, AWS and NVIDIA have collaborated to release a managed implementation of Triton Inference Server in Amazon SageMaker ; for more information, see Deploy fast and scalable AI with NVIDIA Triton Inference Server in Amazon SageMaker. AWS also collaborated with Hugging Face to develop a managed, optimized integration between Amazon SageMaker and Hugging Face Transformers, the open-source framework from which Amazon Search T5 model is derived ; read more at https://aws.amazon.com/machine-learning/hugging-face/.
We encourage customers with latency-sensitive CPU and GPU deep learning serving applications to consider NVIDIA TensorRT and Triton on AWS. Let us know what you build!
Passionate about deep learning and building deep learning-based solutions for Amazon Search? Check out our careers page.
About the Authors
RJ is an engineer in Search M5 team leading the efforts for building large scale deep learning systems for training and inference. Outside of work he explores different cuisines of food and plays racquet sports.
Hemant Pugaliya is an Applied Scientist at Search M5. He works on applying latest natural language processing and deep learning research to improve customer experience on Amazon shopping worldwide. His research interests include natural language processing and large-scale machine learning systems. Outside of work, he enjoys hiking, cooking and reading.
Andy Sun is a Software Engineer and Technical Lead for Search Spelling Correction. His research interests include optimizing deep learning inference latency, and building rapid experimentation platforms. Outside of work, he enjoys filmmaking, and acrobatics.
Le Cai is a Software Engineer at Amazon Search. He works on improving Search Spelling Correction performance to help customers with their shopping experience. He is focusing on high-performance online inference and distributed training optimization for deep learning model. Outside of work, he enjoys skiing, hiking and cycling.
Anthony Ko is currently working as a software engineer at Search M5 Palo Alto, CA. He works on building tools and products for model deployment and inference optimization. Outside of work, he enjoys cooking and playing racquet sports.
Olivier Cruchant is a Machine Learning Specialist Solutions Architect at AWS, based in France. Olivier helps AWS customers – from small startups to large enterprises – develop and deploy production-grade machine learning applications. In his spare time, he enjoys reading research papers and exploring the wilderness with friends and family.
Anish Mohan is a Machine Learning Architect at NVIDIA and the technical lead for ML and DL engagements with its customers in the greater Seattle region.
Jiahong Liu is a Solution Architect on the Cloud Service Provider team at NVIDIA. He assists clients in adopting machine learning and AI solutions that leverage NVIDIA accelerated computing to address their training and inference challenges. In his leisure time, he enjoys origami, DIY projects, and playing basketball.
Eliuth Triana is a Developer Relations Manager at NVIDIA. He connects Amazon and AWS product leaders, developers, and scientists with NVIDIA technologists and product leaders to accelerate Amazon ML/DL workloads, EC2 products, and AWS AI services. In addition, Eliuth is a passionate mountain biker, skier, and poker player.
Keynote Wrap Up: Turning Data Centers into ‘AI Factories,’ NVIDIA CEO Intros Hopper Architecture, H100 GPU, New Supercomputers, Software
Promising to transform trillion-dollar industries and address the “grand challenges” of our time, NVIDIA founder and CEO Jensen Huang Tuesday shared a vision of an era where intelligence is created on an industrial scale and woven into real and virtual worlds.
Kicking off NVIDIA’s GTC conference, Huang introduced new silicon — including the new Hopper GPU architecture and new H100 GPU, new AI and accelerated computing software and powerful new data-center-scale systems.
”Companies are processing, refining their data, making AI software, becoming intelligence manufacturers,” Huang said, speaking from a virtual environment in the NVIDIA Omniverse real-time 3D collaboration and simulation platform as he described how AI is “racing in every direction.”
And all of it will be brought together by Omniverse to speed collaboration between people and AIs, better model and understand the real world, and serve as a proving ground for new kinds of robots, “the next wave of AI.”
Huang shared his vision with a gathering that has become one of the world’s most important AI conferences, bringing together leading developers, scientists and researchers.
The conference features more 1,600 speakers including from companies such as American Express, DoorDash, LinkedIn, Pinterest, Salesforce, ServiceNow, Snap and Visa, as well as 200,000 registered attendees.
Huang’s presentation began with a spectacular flythrough of NVIDIA’s new campus, rendered in Omniverse, including buzzing labs working on advanced robotics projects.
He shared how the company’s work with the broader ecosystem is saving lives by advancing healthcare and drug discovery, and even helping save our planet.
“Scientists predict that a supercomputer a billion times larger than today’s is needed to effectively simulate regional climate change,” Huang said.
“NVIDIA is going to tackle this grand challenge with our Earth-2, the world’s first AI digital twin supercomputer, and invent new AI and computing technologies to give us a billion-X before it’s too late,” he said.
New Silicon — NVIDIA H100: A “New Engine of the World’s AI Infrastructure”
To power these ambitious efforts, Huang introduced the NVIDIA H100 built on the Hopper architecture, as the “new engine of the world’s AI infrastructures.”
AI applications like speech, conversation, customer service and recommenders are driving fundamental changes in data center design, he said.
“AI data centers process mountains of continuous data to train and refine AI models,” Huang said. “Raw data comes in, is refined, and intelligence goes out — companies are manufacturing intelligence and operating giant AI factories.”
The factory operation is 24/7 and intense, Huang said. Minor improvements in quality drive a significant increase in customer engagement and company profits, Huang explained.
H100 will help these factories move faster. The “massive” 80 billion transistor chip uses TSMC’s 4N process.
“Hopper H100 is the biggest generational leap ever — 9x at-scale training performance over A100 and 30x large-language-model inference throughput,” Huang said.
Hopper is packed with technical breakthroughs, including a new Transformer Engine to speed up these networks 6x without losing accuracy.
“Transformer model training can be reduced from weeks to days” Huang said.
H100 is in production, with availability starting in Q3, Huang announced.
Huang also announced the Grace CPU Superchip, NVIDIA’s first discrete data center CPU for high-performance computing.
It comprises two CPU chips connected over a 900 gigabytes per second NVLink chip-to-chip interconnect to make a 144-core CPU with 1 terabyte per second of memory bandwidth, Huang explained.
“Grace is the ideal CPU for the world’s AI infrastructures,” Huang said.
Huang also announced new Hopper GPU-based AI supercomputers — DGX H100, H100 DGX POD and DGX SuperPOD.
To connect it all, NVIDIA’s new NVLink high-speed interconnect technology will be coming to all future NVIDIA chips — CPUs, GPUs, DPUs and SOCs, Huang said.
He also announced NVIDIA will make NVLink available to customers and partners to build companion chips.
“NVLink opens a new world of opportunities for customers to build semi-custom chips and systems that leverage NVIDIA’s platforms and ecosystems,” Huang said.
New Software — AI Has “Fundamentally Changed” Software
Thanks to acceleration unleashed by accelerated computing, the progress of AI is “stunning,” Huang declared.
“AI has fundamentally changed what software can make and how you make software,” Huang said.
Transformers, Huang explained, have opened self-supervised learning and unblocked the need for human-labeled data. As a result, Transformers are being unleashed in a growing array of fields.
“Transformers made self-supervised learning possible, and AI jumped to warp speed,” Huang said.
Google BERT for language understanding, NVIDIA MegaMolBART for drug discovery, and DeepMind AlphaFold2 are all breakthroughs traced to Transformers, Huang said.
Huang walked through new deep learning models for natural language understanding, physics, creative design, character animation and even — with NVCell — chip layout.
“AI is racing in every direction — new architectures, new learning strategies, larger and more robust models, new science, new applications, new industries — all at the same time,” Huang said.
NVIDIA is “all hands on deck” to speed new breakthroughs in AI and speed the adoption of AI and machine learning to every industry, Huang said.
The NVIDIA AI platform is getting major updates, Huang said, including Triton Inference Server, the NeMo Megatron 0.9 framework for training large language models, and the Maxine framework for audio and video quality enhancement.
The platform includes NVIDIA AI Enterprise 2.0, an end-to-end, cloud-native suite of AI and data analytics tools and frameworks, optimized and certified by NVIDIA and now supported across every major data center and cloud platform.
“We updated 60 SDKs at this GTC,” Huang said. “For our 3 million developers, scientists and AI researchers, and tens of thousands of startups and enterprises, the same NVIDIA systems you run just got faster.”
NVIDIA AI software and accelerated computing SDKs are now relied on by some of the world’s largest companies.
- Microsoft Translator accelerates global communications with real-time translation capabilities powered by NVIDIA Triton.
- AT&T accelerates their data science teams with NVIDIA RAPIDS software that makes it easier to process trillions of records.
“NVIDIA SDKs serve healthcare, energy, transportation, retail, finance, media and entertainment — a combined $100 trillion of industries,” Huang said.
‘The Next Evolution’: Omniverse for Virtual Worlds
Half a century ago, the Apollo 13 lunar mission ran into trouble. To save the crew, Huang said, NASA engineers created a model of the crew capsule back on Earth to “work the problem.”
“Extended to vast scales, a digital twin is a virtual world that’s connected to the physical world,” Huang said. “And in the context of the internet, it is the next evolution.”
NVIDIA Omniverse software for building digital twins, and new data-center-scale NVIDIA OVX systems, will be integral for “action-oriented AI.”
“Omniverse is central to our robotics platforms,” Huang said, announcing new releases and updates for Omniverse. “And like NASA and Amazon, we and our customers in robotics and industrial automation realize the importance of digital twins and Omniverse.”
OVX will run Omniverse digital twins for large-scale simulations with multiple autonomous systems operating in the same space-time, Huang explained.
The backbone of OVX is its networking fabric, Huang said, announcing the NVIDIA Spectrum-4 high-performance data networking infrastructure platform.
The world’s first 400Gbps end-to-end networking platform, NVIDIA Spectrum-4 consists of the Spectrum-4 switch family, NVIDIA ConnectX-7 SmartNIC, NVIDIA BlueField-3 DPU and NVIDIA DOCA data center infrastructure software.
And to make Omniverse accessible to even more users, Huang announced Omniverse Cloud. Now, with just a few clicks, collaborators can connect through Omniverse on the cloud.
Huang showed how this works with a demo of four designers, one an AI, collaborating to build a virtual world.
He also showed how Amazon uses Omniverse Enterprise “to design and optimize their incredible fulfillment center operations.”
“Modern fulfillment centers are evolving into technical marvels — facilities operated by humans and robots working together,” Huang said.
The ‘Next Wave of AI’: Robots and Autonomous Vehicles
New silicon, new software and new simulation capabilities will unleash “the next wave of AI,” Huang said, robots able to “devise, plan and act.”
NVIDIA Avatar, DRIVE, Metropolis, Isaac and Holoscan are robotics platforms built end to end and full stack around “four pillars”: ground-truth data generation, AI model training, the robotics stack and Omniverse digital twins, Huang explained.
The NVIDIA DRIVE autonomous vehicle system is essentially an “AI chauffeur,” Huang said.
And Hyperion 8 — NVIDIA’s hardware architecture for self-driving cars on which NVIDIA DRIVE is built — can achieve full self-driving with a 360-degree camera, radar, lidar and ultrasonic sensor suite.
Hyperion 8 will ship in Mercedes-Benz cars starting in 2024, followed by Jaguar Land Rover in 2025, Huang said.
Huang announced that NVIDIA Orin, a centralized AV and AI computer that acts as the engine of new-generation EVs, robotaxis, shuttles, and trucks started shipping this month.
And Huang announced Hyperion 9, featuring the coming DRIVE Atlan SoC for double the performance of the current DRIVE Orin-based architecture, which will ship starting in 2026.
BYD, the second-largest EV maker globally, will adopt the DRIVE Orin computer for cars starting production in the first half of 2023, Huang announced.
Overall, NVIDIA’s automotive pipeline has increased to over $11 billion over the next six years.
Clara Holoscan puts much of the real-time computing muscle used in DRIVE to work supporting medical instruments and real-time sensors, such as RF ultrasound, 4K surgical video, high-throughput cameras and lasers.
Huang showed a video of Holoscan accelerating images from a light-sheet microscope — which creates a “movie” of cells moving and dividing.
It typically takes an entire day to process the 3TB of data these instruments produce in an hour.
At the Advanced Bioimaging Center at UC Berkeley, however, researchers using Holoscan are able to process this data in real-time, enabling them to auto-focus the microscope while experiments are running.
Holoscan development platforms are available for early access customers today, generally available in May, and medical-grade readiness in the first quarter of 2023.
NVIDIA is also working with thousands of customers and developers who are building robots for manufacturing, retail, healthcare, agriculture, construction, airports and entire cities, Huang said.
NVIDIA’s robotics platforms consist of Metropolis and Isaac — Metropolis is a stationary robot tracking moving things, while Isaac is a platform for things that move, Huang explained.
To help robots navigate indoor spaces — like factories and warehouses — NVIDIA announced Isaac Nova Orin, built on Jetson AGX Orin, a state-of-the-art compute and sensor reference platform to accelerate autonomous mobile robot development and deployment.
In a video, Huang showed how PepsiCo uses Metropolis and an Omniverse digital twin together.
Four Layers, Five Dynamics
Huang ended by tying all the technologies, product announcements and demos back into a vision of how NVIDIA will drive forward the next generation of computing.
NVIDIA announced new products across its four-layer stack: hardware, system software and libraries, software platforms NVIDIA HPC, NVIDIA AI, and NVIDIA Omniverse; and AI and robotics application frameworks, Huang explained.
Huang also ticked through the five dynamics shaping the industry: million-X computing speedups, transformers turbocharging AI, data centers becoming AI factories, which is exponentially increasing demand for robotics systems, and digital twins for the next era of AI.
“Accelerating across the full stack and at data center scale, we will strive for yet another million-X in the next decade,” Huang said, concluding his talk. “I can’t wait to see what the next million-X brings.”
Noting that Omniverse generated “every rendering and simulation you saw today,” Huang then introduced a stunning video put together by NVIDIA’s creative team featuring viewers “on one more trip into Omniverse” for a surprising musical jazz number set in the heart of NVIDIA’s campus featuring a cameo from Huang’s digital counterpart, Toy Jensen.
The post Keynote Wrap Up: Turning Data Centers into ‘AI Factories,’ NVIDIA CEO Intros Hopper Architecture, H100 GPU, New Supercomputers, Software appeared first on NVIDIA Blog.
Unlimited Data, Unlimited Possibilities: UF Health and NVIDIA Build World’s Largest Clinical Language Generator
The University of Florida’s academic health center, UF Health, has teamed up with NVIDIA to develop a neural network that generates synthetic clinical data — a powerful resource that researchers can use to train other AI models in healthcare.
Trained on a decade of data representing more than 2 million patients, SynGatorTron is a language model that can create synthetic patient profiles that mimic the health records it’s learned from. The 5 billion-parameter model is the largest language generator in healthcare.
“Synthetic data isn’t actually linked to a real human being, but it has similar characteristics to real patients,” said Dr. Duane Mitchell, an assistant vice president for research and director of the UF Clinical and Translational Science Institute. “SynGatorTron can, for example, create health records of digital diabetes patients that have features just like a real population.”
Using this synthetic data, researchers can create tools, models and tasks without risks or privacy concerns. These can then be used on real data to ask clinical questions, look for associations and even explore patient outcomes.
Working with synthetic data also makes it easier for different research institutions to collaborate and share models. And since the amount of data that can be synthesized is virtually limitless, researchers can use SynGatorTron-generated data to augment small datasets of rare disease patients or minority populations to reduce model bias.
SynGatorTron was developed using the open-source NVIDIA Megatron-LM and NeMo frameworks. It’s based on UF Health’s GatorTron model, announced last year at NVIDIA GTC. The models were trained on HiPerGator-AI, the university’s in-house NVIDIA DGX SuperPOD system, which ranks among the world’s top 30 supercomputers.
GatorTron-S, a BERT-style transformer model trained on synthetic data generated by SynGatorTron, will be available for developers next month on the NGC software hub.
SynGatorTron Opens Gate to Robust Training Data
To a doctor, an AI-generated doctor’s note can appear impractical at first glance — it doesn’t represent a real patient and won’t read as logical to an expert eye. So a clinician can’t make a direct analysis or diagnosis from it. But to an untrained AI, real and synthetic clinical data are both highly valuable.
“SynGatorTron’s generative capability is a great enabler of natural language processing for medicine,” said Dr. Mona Flores, global head of medical AI at NVIDIA. “Synthesizing different types of clinical records will democratize the ability to create all sorts of applications dependent on such data by addressing data sparsity and privacy.”
Once it’s available, research institutions outside UF Health could fine-tune the pretrained SynGatorTron model with their own localized data and apply it to their AI projects. For example, if a given condition or a patient population is underrepresented in a health system’s clinical data, SynGatorTron can be prompted to generate additional data with characteristics of that disease or population.
These AI-generated records could then be used to supplement and balance out real healthcare datasets used to train other neural networks, so that they better represent the population.
Since synthetic training datasets mimic real medical notes without being associated with specific patients, they can also be more readily shared across research institutions without raising privacy concerns.
“When you have the ability to mimic population characteristics without being tethered to real patients, it opens the imagination to see if we can generate realistic datasets that allow us to answer questions we couldn’t otherwise, due to constraints on access to data or limited information on patients of interest,” Mitchell said.
One potential application is in clinical trials, which often divide patients into treatment and control groups to measure the effectiveness of a new medication. An application derived from SynGatorTron-generated data could parse through real records and create a digital twin of patient records. These records could then be used as the control group in a clinical trial, instead of having a control group derived by giving real patients a placebo treatment.
Researchers developing a deep learning model to study a rare disease, or the effects of a treatment on a specific population, could also use SynGatorTron for data augmentation, generating more training data to supplement the limited amount of real medical records available.
Healthcare at GTC
Register free for GTC, running online March 21-24, to discover the latest in AI and healthcare. Hear from SynGatorTron collaborators in the session “A Next-Generation Clinical Language Model,” taking place March 23 at 7 a.m. Pacific.
Watch the replay of NVIDIA founder and CEO Jensen Huang’s keynote address below:
The post Unlimited Data, Unlimited Possibilities: UF Health and NVIDIA Build World’s Largest Clinical Language Generator appeared first on NVIDIA Blog.
New NVIDIA RTX GPUs Tackle Demanding Professional Workflows and Hybrid Work, Enabling Creation From Anywhere
Remote work and hybrid workplaces are the new normal for professionals in many industries. Teams spread throughout the world are expected to create and collaborate while maintaining top productivity and performance.
Businesses use the NVIDIA RTX platform to enable their workers to keep up with the most demanding workloads, from anywhere. And today, NVIDIA is expanding its RTX offerings with seven new NVIDIA Ampere architecture GPUs for laptops and desktops.
The new NVIDIA RTX A500, RTX A1000, RTX A2000 8GB, RTX A3000 12GB, RTX A4500 and RTX A5500 laptop GPUs expand access to AI and ray-tracing technology, delivering breakthrough performance no matter where you work. The laptops include the latest RTX and Max-Q technology, giving professionals the ability to take their workflows to the next level.
The new NVIDIA RTX A5500 desktop GPU combines the latest-generation RT Cores, Tensor Cores and CUDA cores with 24GB of memory for incredible rendering, AI, graphics and compute performance. Its ray-traced rendering is 2x faster than the previous generation and its motion blur rendering performance is up to 9x faster.
With the new NVIDIA RTX GPUs, artists can create photorealistic immersive digital experiences, scientists can make the latest groundbreaking discoveries, and engineers can develop innovative technology to advance us into the future.
Customer Adoption
Among the first to use the NVIDIA RTX A5500 is M4 Engineering, a leading aerospace engineering firm focused on conceptual aircraft design, analysis and development.
“The multi-app product development workflows we use at M4 are well-served by the NVIDIA RTX A5500 and its 24GB of memory,” said Brian Rotty, senior engineer at M4 Engineering. “My team can handle larger CAD and CAE datasets than before and, critically, we can interact with and iterate these larger datasets simultaneously by making use of the extra GPU memory headroom and compute capabilities of this new card.”
“The performance we get with the NVIDIA RTX A5500 is unprecedented,” said Hiram Rodriguez, design technology specialist at AS+GG Architecture. “Previously, our point cloud processing took too long and created a bottleneck for designing and analyzing current site conditions. Using the NVIDIA RTX A5500, in less than 20 minutes we can integrate a fully processed, geolocated, classified point cloud into our 3D models.”
“The new NVIDIA RTX A5500 gives us the ability to load highly detailed environments, while maintaining high frame rates and smooth camera motion to explore and develop the scenes,” said Yiotis Katsambas, executive director of Technology at Sony Pictures Animation. “By combining the A5500 with NVIDIA Omniverse Enterprise and our own FlixiVerse software, our artists and directors can immerse themselves in our virtual worlds and collaborate in real-time.”
Next-Generation RTX Technology
The new NVIDIA RTX GPUs feature the latest generation of NVIDIA RTX technology to accelerate graphics, AI and ray-tracing performance.
These GPUs are part of the comprehensive NVIDIA RTX platform, which includes NVIDIA GPU-accelerated software development kits, toolkits, frameworks and enterprise management tools.
These GPUs also take advantage of the accelerations of the NVIDIA Studio platform, including optimizations to leverage RTX hardware in 75 of the top creative apps, exclusive tools like NVIDIA Broadcast and Canvas, and the advanced real-time 3D design and collaboration platform, NVIDIA Omniverse.
NVIDIA RTX GPUs are certified by independent software vendors of over 50+ professional applications. Certification provides users with a reliable and dependable graphics and computing experience through testing and development.
NVIDIA RTX laptop GPUs include:
- The latest NVIDIA RTX technology: 2nd gen RT Cores, 3rd gen Tensor Cores and NVIDIA Ampere architecture streaming multiprocessor, which provide up to 2x the throughput of the previous-generation architecture to tackle demanding rendering, ray tracing and AI workflows from anywhere.
- NVIDIA Max-Q technology: AI-based system optimizations make thin and light laptops perform quieter, faster and more efficiently with Dynamic Boost, CPU Optimizer, Rapid Core Scaling, WhisperMode, Battery Boost, Resizable BAR and NVIDIA DLSS technology.
- Up to 16GB of GPU memory: For the largest models, scenes and assemblies. The RTX A2000 8GB, RTX A3000 12GB and RTX A4500 now feature 2x the memory of their previous-generation counterparts for working with larger models, datasets and multi-app workflows.
- Rich suite of NVIDIA software technology: Users can tap into unique benefits ranging from tetherless VR to collaborative 3D design with a wide variety of software tools, including NVIDIA CloudXR, NVIDIA Omniverse, NVIDIA Canvas, NVIDIA Broadcast, NVIDIA NGC, NVIDIA RTX Experience and more.
The NVIDIA RTX A5500 features the latest technologies in the NVIDIA Ampere architecture:
- Second-generation RT Cores: Up to 2x the throughput of the first generation with the ability to run concurrent ray tracing, shading and denoising tasks.
- Third-generation Tensor Cores: Up to 12x the training throughput over the previous generation, with support for new TF32 and Bfloat16 data formats.
- CUDA Cores: Up to 3x the single-precision floating point throughput over the previous generation.
- Up to 48GB of GPU memory: The RTX A5500 features 24GB of GDDR6 memory with ECC (error correction code). The RTX A5500 is expandable up to 48GB of memory using NVIDIA NVLink to connect two GPUs.
- Virtualization: The RTX A5500 supports NVIDIA RTX Virtual Workstation (vWS) software for multiple high-performance virtual workstation instances that enable remote users to share resources to drive high-end design, AI and compute workloads.
- PCIe Gen 4: Doubles the bandwidth of the previous generation and speeds up data transfers for data-intensive tasks such as AI, data science and creating 3D models.
Availability
The new NVIDIA RTX A5500 desktop GPUs are available today from channel partners and through global system builders starting in Q2.
The new NVIDIA RTX laptop GPUs will be available in mobile workstations from global OEM partners including Acer, ASUS, BOXX Technologies, Dell Technologies, HP, Lenovo, and MSI starting this spring. Contact these partners for specific system configuration details and availability.
To learn more about NVIDIA RTX, watch the GTC 2022 keynote from Jensen Huang. Register for GTC 2022 for free to attend sessions with NVIDIA and industry leaders.
The post New NVIDIA RTX GPUs Tackle Demanding Professional Workflows and Hybrid Work, Enabling Creation From Anywhere appeared first on NVIDIA Blog.