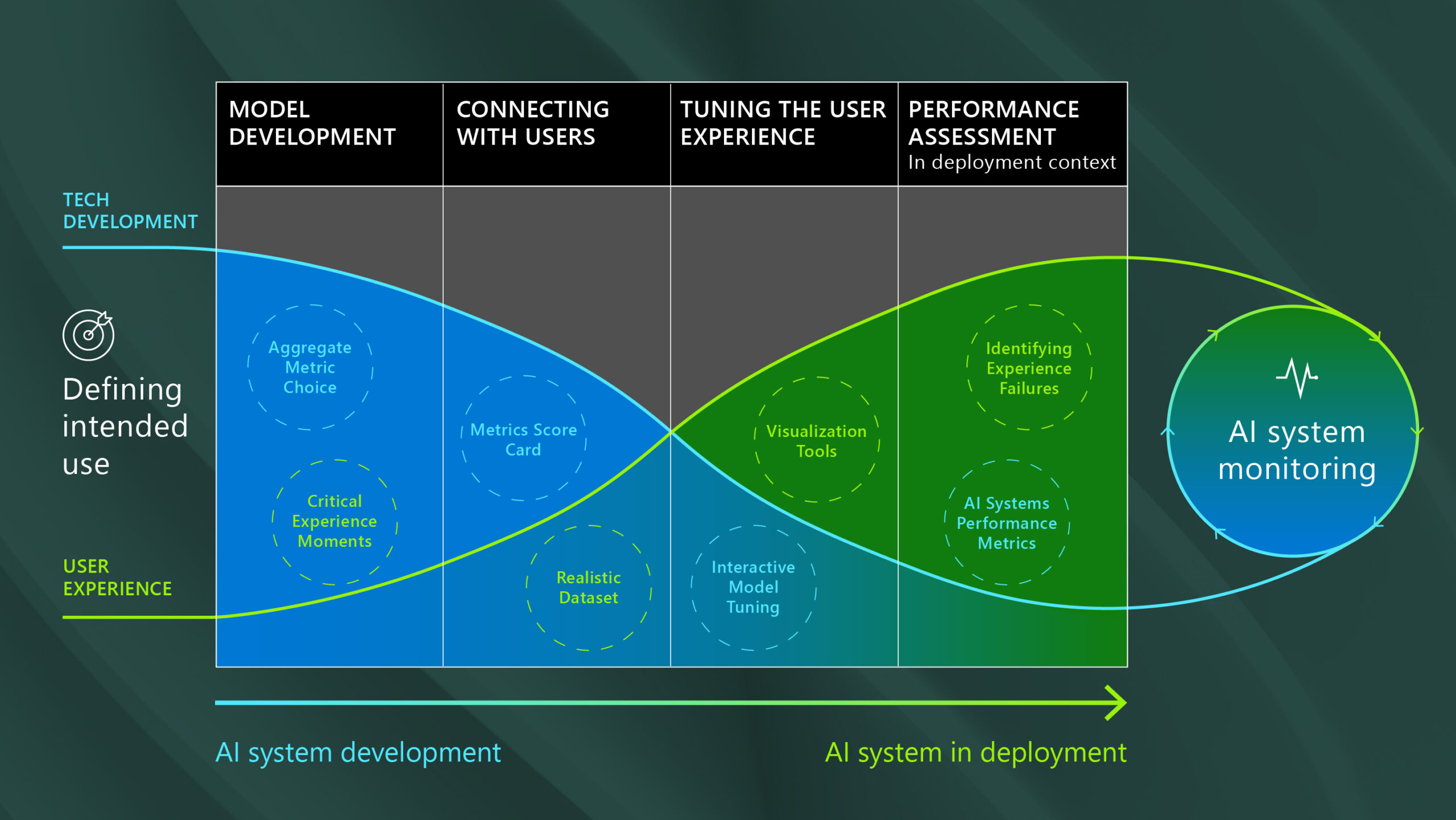
AI systems are becoming increasingly complex as we move from visionary research to deployable technologies such as self-driving cars, clinical predictive models, and novel accessibility devices. Unlike singular AI models, it is more difficult to assess whether these more complex AI systems are performing consistently and as intended to realize human benefit.
-
- Real-world contexts for which the data might be noisy or different from training data;
- Multiple AI components interact with each other, creating unanticipated dependencies and behaviors;
- Human-AI feedback loops that come from repeated engagements between people and AI system.
- Very large AI models (e.g., transformer models)
- AI models that interact with other parts of a system (e.g., user interface or heuristic algorithm)
How do we know when these more advanced systems are ‘good enough’ for their intended use? When assessing the performance of AI models, we often rely on aggregate performance metrics like percentage of accuracy. But this ignores the many, often human elements, that make up an AI system.
Our research on what it takes to build forward-looking, inclusive AI experiences has demonstrated that getting to ‘good enough’ requires multiple performance assessment approaches at different stages of the development lifecycle, based upon realistic data and key user needs (figure 1).
Shifting emphasis gradually from iterative adjustments in the AI models themselves toward approaches that improve the AI system as a whole has implications not only in terms of how performance is assessed, but who should be involved in the performance assessment process. Engaging (and training) non-technical domain experts earlier (i.e., for choosing test data or defining experience metrics) and in a larger capacity throughout the development lifecycle can enhance relevance, usability, and reliability of the AI system.
Performance assessment best practices from the PeopleLens
The PeopleLens (figure 2) is a new Microsoft technology designed to enable children who are born blind to experience social agency and build up the range of social attention skills needed to initiate and maintain social interactions. Running on smart glasses, it provides the wearer with continuous, real-time information about the people around them through spatial audio, helping them build up a dynamic map of the whereabouts of others. Its underlying technology is a complex AI system using several computer vision algorithms to calculate, pose, identify registered people, and track those entities over time.
The PeopleLens offers a useful illustration of the wide range of performance assessment methods and people necessary to comprehensively gauge its efficacy.
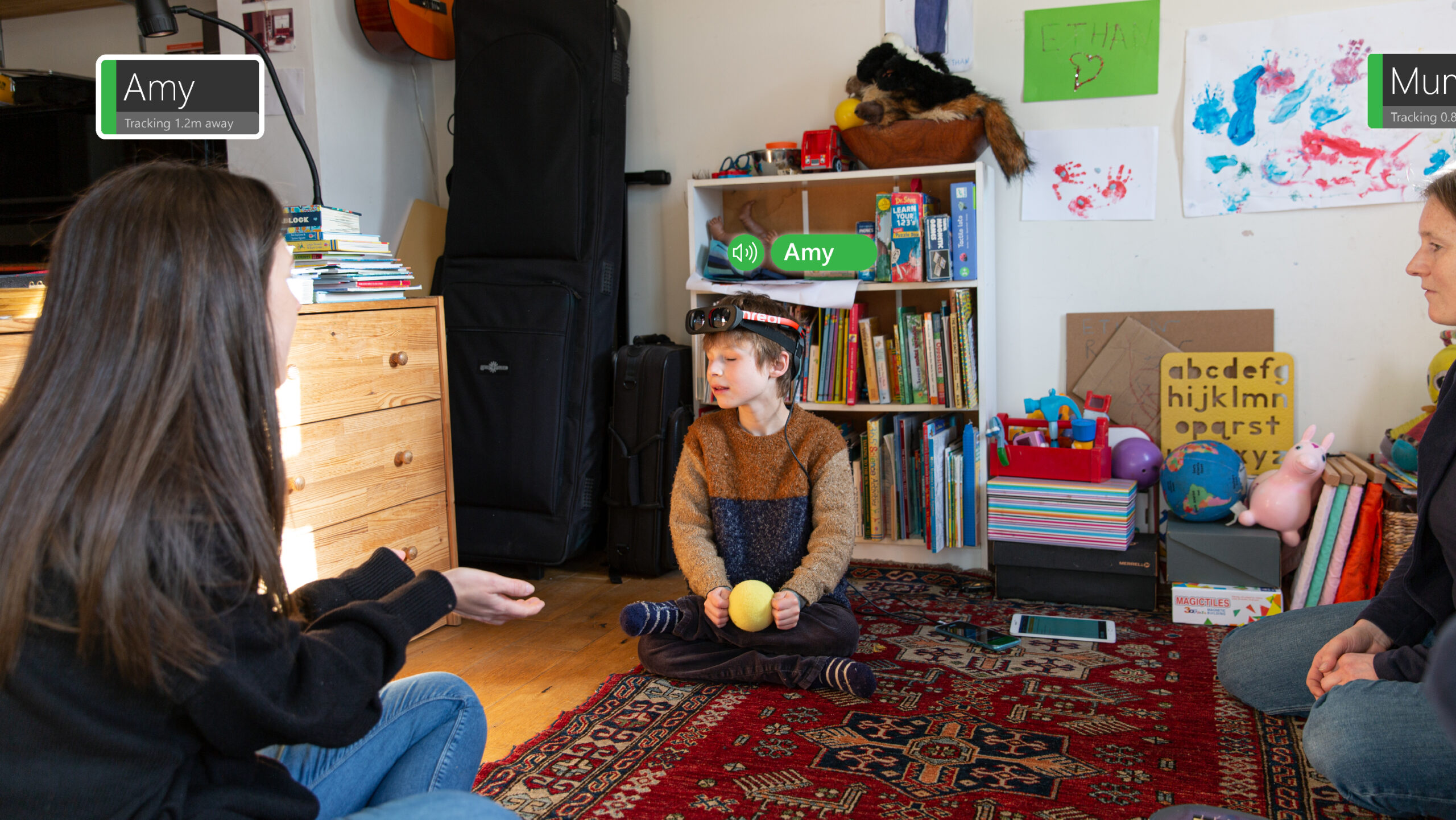
Getting started: AI model or AI system performance?
Calculating aggregate performance metrics on open-source benchmarked datasets may demonstrate the capability of an individual AI model, but may be insufficient when applied to an entire AI system. It can be tempting to believe a single aggregate performance metric (such as accuracy) can be sufficient to validate multiple AI models individually. But the performance of two AI models in a system cannot be comprehensively measured by simple summation of each model’s aggregate performance metric.
We used two AI models to test the accuracy of the PeopleLens to locate and identify people: the first was a benchmarked, state-of-the-art pose model used to indicate the location of people in an image. The second was a novel facial recognition algorithm previously demonstrated to have greater than 90% accuracy. Despite strong historical performance of these two models, when applied to the PeopleLens, the AI system recognized only 10% of people from a realistic dataset in which people were not always facing the camera.
This finding illustrates that multi-algorithm systems are more than a sum of their parts, requiring specific performance assessment approaches.
Connecting to the human experience: Metric scorecards and realistic data
Metrics scorecards, calculated on a realistic reference dataset, offer one way to connect to the human experience while the AI system is still undergoing significant technical iteration. A metrics scorecard can combine several metrics to measure aspects of the system that are most important to users.
We used ten metrics in the development of PeopleLens. The most valuable two metrics were time-to-first-identification, which measured how long it took from the time a person was seen in a frame to the user hearing the name of that person, and number of repeat false positives, which measured how often a false positive occurred in three frames or more in a row within the reference dataset.
The first metric captured the core value proposition for the user: having the social agency to be the first to say hello when someone approaches. The second was important because the AI system would self-correct single misidentifications, while repeated mistakes would lead to a poor user experience. This measured the ramifications of that accuracy throughout the system, rather than just on a per-frame basis.
Beyond metrics: Using visualization tools to finetune the user experience
While metrics play a critical role in the development of AI systems, a wider range of tools is needed to finetune the intended user experience. It is essential for development teams to test on realistic datasets to understand how the AI system generates the actual user experience. This is especially important with complex systems, where multiple models, human-AI feedback loops, or unpredictable data (e.g., user-controlled data capture) can cause the AI system to respond unpredictably.
Visualization tools can enhance the top-down statistical tools of data scientists, helping domain experts contribute to system development. In the PeopleLens, we used custom-built visualization tools to compare side-by-side renditions of the experience with different model parameters (figure 3). We leveraged these visualizations to enable domain experts—in this case parents and teachers—to spot patterns of odd system behavior across the data.
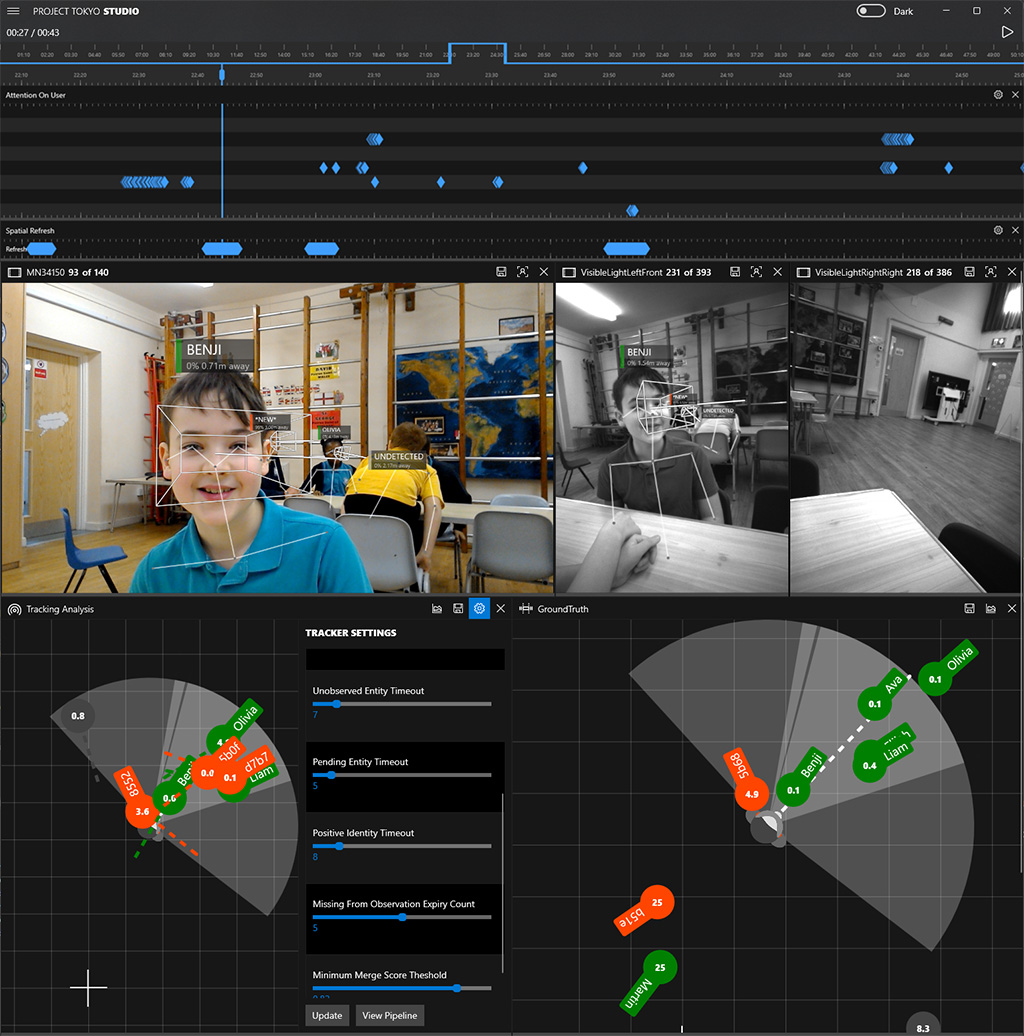
AI system performance in the context of the user experience
A user experience can only be as good as the underlying AI system. Testing the AI system in a realistic context, measuring things that matter to the users, is a critical stage before wide-spread deployment. We know, for example, that improving AI system performance does not necessarily correspond to improved performance of AI teams (reference).
We also know that human-to-AI feedback loops can make it difficult to measure an AI system’s performance. Essentially repeated interactions between AI system and user, these feedback loops can surface (and intensify) errors. They can also, through good intelligibility, be repaired by the user.
The PeopleLens system gave users feedback about the people’s locations and their faces. A missed identification (e.g., because the person is looking at a chest rather than a face) can be resolved once the user responds to feedback (e.g., by looking up). This example shows us that we do not need to focus on missed identification as they will be resolved by the human-AI feedback loop. However, users were very perplexed by the identification of people who were no longer present, and therefore performance assessments needed to focus on these false positive misidentifications.
-
- Multiple performance assessment methods should be used in AI system development. In contrast to developing individual AI models, general aggregate performance metrics are a small component, relevant primarily in the earliest stages of development.
- Documenting AI system performance should include a range of approaches, from metrics scorecards to system performance metrics for a deployed user experience, to visualization tools.
- Domain experts play an important role in performance assessment, beginning early in the development lifecycle. Domain experts are often not prepared or skilled for the in-depth participation optimal in AI system development.
- Visualization tools are as important as metrics in creating and documenting an AI system for a particular intended use. It is critical that domain experts have access to these tools as key decision-makers in AI system deployment.
Bringing it all together
For complex AI systems, performance assessment methods change across the development lifecycle in ways that differ from individual AI models. Shifting performance assessment techniques from rapid technical innovation requiring easy-to-calculate aggregate metrics at the beginning of the development process, to the performance metrics that reflect critical AI system attributes that make up the user experience toward the end of development helps every type of stakeholder precisely and collectively define what is ‘good enough’ to achieve the intended use.
It is useful for developers to remember performance assessment is not an end goal in itself; it is a process that defines how the system has reached its best state and whether that state is ready for deployment. The performance assessment process must include a broad range of stakeholders, including domain experts, who may need new tools to fulfill critical (sometimes unexpected) roles in the development and deployment of an AI system.
The post Assessing AI system performance: thinking beyond models to deployment contexts appeared first on Microsoft Research.