Using NVIDIA platforms, tools and libraries, European telecommunications institutions are accelerating efforts to develop 6G — the next generation of cellular technology, with AI woven in from the start.
6G will be an AI-native platform that fosters innovation, enables new services, enhances customer experiences and promotes sustainability. Since the launch of the NVIDIA 6G Developer Program last year, over 200 telecommunications organizations across 30+ European countries have used NVIDIA technologies to accelerate their work.
In the U.K., the Department for Science, Innovation and Technology announced a collaboration with NVIDIA to promote the nation’s goals for AI development in telecom. Leading U.K. universities will gain access to a suite of powerful AI tools, 6G research platforms and training resources — including NVIDIA AI Aerial and Sionna — to bolster research and development on AI-native wireless networks.
“This collaboration between the U.K. government and NVIDIA marks a pivotal step in our ambition to make the U.K. a global leader in the development of advanced connectivity technologies,” said Sir Chris Bryant, minister of state for data protection and telecoms of the U.K.“The use of AI in telecoms will make our networks more intelligent, efficient and reliable, and by equipping our world leading academia and researchers with cutting-edge AI tools and training, we will accelerate innovation that improves the everyday digital experience for people across the country.”
In Finland, the University of Oulu is conducting research for wireless channel estimation with a real-time network digital twin that taps into synthetic lidar data, using the NVIDIA Isaac Sim reference application for robotics simulation.
The project enables advanced development of AI and machine learning features for integrated sensing and communications, or ISAC, a capability that allows the network itself to act as a sensor of the physical world to enhance operations. The project also enables modeling of the 6G access system.
France-based OpenAirInterface (OAI) and NVIDIA are collaborating to advance AI-native wireless networks by integrating OAI’s open-source virtualized and open RAN stack with the GPU-accelerated NVIDIA AI Aerial– and NVIDIA Sionna-based systems. OAI provides the layer 2+ software for Aerial Commercial Testbed and full-stack O-RAN software for Sionna Research Kit, enabling researchers to innovate in 5G and 6G radio access networks using AI and machine learning at every layer.
In Germany, Fraunhofer HHI is conducting groundbreaking research on neuromorphic wireless cognition for robotic control using the NVIDIA AI Aerial suite of accelerated computing platforms and software for designing, simulating and operating wireless networks.
The research involves an event-based camera that senses and captures robotic movements, and forwards the information to a neuromorphic processor. Then, neural network models are used for decoding and gesture recognition to boost transmission over the base station, enhancing the quality of the connection.
Rohde & Schwarz, also based in Germany, is helping set new benchmarks in AI-powered wireless communication research with its latest milestone in neural receiver design and testing.
Showcased in March at Mobile World Congress in Barcelona, Rohde & Schwarz’s innovative proof of concept — developed in collaboration with NVIDIA — integrates advanced digital twin technology and high-fidelity ray tracing to create a robust framework for testing 5G-Advanced and 6G neural receivers under real-world radio environments. Tapping into simulations built with the NVIDIA Sionna library, this initiative paves the way for more efficient, accurate and reliable testing of next-generation receiver architectures.
ETH Zurich and NVIDIA are working on 6G projects related to the performance of AI-native 6G networks. This includes a new machine learning-based architecture, called DUIDD (Deep Unfolded Iterative Detector Decoder), which was developed using NVIDIA Sionna to improve the amount of data a base station can transmit or receive using information learned from its local environment. DUIDD is expected to be implemented on the real-time, over-the-air NVIDIA AI Aerial commercial testbed, dubbed ARC-OTA.
Other projects include a new approach to machine learning-assisted model training, machine learning approaches for positioning mobile devices using channel charting, and device identification based on their radio frequency signature, developed with NVIDIA 6G research tools.
The University of Leeds is developing an agentic architecture for integrating large language models into RAN operations to realize scalable, intelligent orchestration. The research involves creating standardized frameworks for deploying agent-based architectures, establishing key performance indicators for benchmarking performance and building templates for new agents to enhance performance and reduce operational costs.
Europe Key to Developing AI-Native 6G
Europe’s role in wireless networks dates back to the 1987 development of the Global System for Mobile Communications, or GSM, a widely used standard for digital cellular communication.
Today, the European Union continues to drive innovation through substantial governmental support and flagship initiatives such as the Smart Networks and Services Joint Undertaking, 6G SNS and the 6G Flagship project. These programs unite universities, research institutions and industry to create next-generation AI-native networks, pioneering work in AI integration, sustainability and security while educating future industry experts.
Major European telecommunications vendors play a vital role in shaping the vision and standards for 6G through their leadership and participation in major research consortia.
NVIDIA Technologies for AI-Native 6G Research and Development
For these European 6G researchers, the NVIDIA 6G research portfolio provides a three-computer solution for 1) developing and training AI algorithms, 2) simulating them and 3) deploying them into wireless stacks for AI-native 6G.
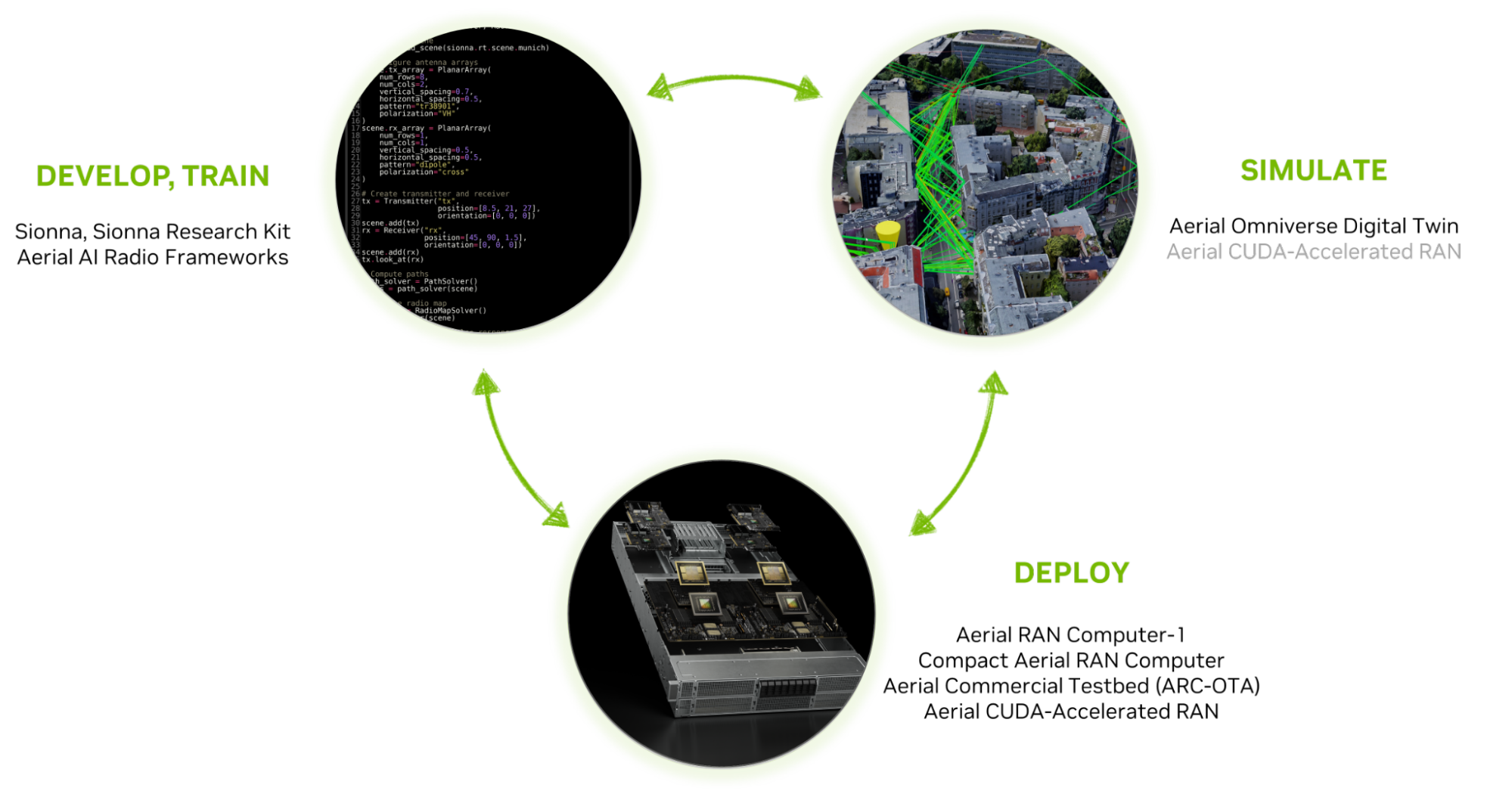
The portfolio includes accelerated compute infrastructure, as well as software libraries, including NVIDIA AI Aerial, Sionna and NVIDIA CUDA-X for accelerating workloads. NVIDIA provides the world’s only 6G research portfolio with open-source and source-code offerings, cloud and on-premises options, and full-stack systems or components-level options for researchers to choose the best tool for their mission.
In addition, the NVIDIA Deep Learning Institute provides training on skills essential to 6G development, such as simulating physical environments.
The NVIDIA 6G Developer Program offers early access to advanced tools, technical support and a global community of innovators. So far, more than 2,000 researchers across 85 countries have joined the program, leading to over 190,000 downloads of NVIDIA tools and 350+ citations in technical papers and journals.
Learn more about the latest AI advancements for telecom and other industries at NVIDIA GTC Paris, running June 11-14 at VivaTech, including a special address from Ronnie Vasishta, senior vice president of telecom at NVIDIA.
Watch the NVIDIA GTC Paris keynote from NVIDIA founder and CEO Jensen Huang at VivaTech 2025, and explore GTC Paris sessions.
See notice regarding software product information.