Next time socks, cereal or sandpaper shows up in hours delivered to your doorstep, consider the behind-the-scenes logistics acrobatics that help get them there so fast.
Order fulfillment is a massive industry of moving parts. Heavily supported by autonomous mobile robots (AMRs), warehouses can span 1 million square feet, expanding and reconfiguring to meet demands. It’s an obstacle course of workers and bottlenecks for hospitals, retailers, airports, manufacturers and others.
To accelerate development of these AMRs, we’ve introduced Isaac Nova Orin, a state-of-the-art compute and sensor reference platform. It’s built on the powerful new NVIDIA Jetson AGX Orin edge AI system, available today. The platform includes the latest sensor technologies and high-performance AI compute capability.
New Isaac Software Arrives for AMR Ecosystem
In addition to Nova Orin, which will be available later this year, we’re delivering new software and simulation capabilities to accelerate AMR deployments — including hardware-accelerated modules, or Isaac ROS GEMs, that are essential for enabling robots to visually navigate. That’s key for mobile robots to better perceive their environment to safely avoid obstacles and efficiently plan paths.
New simulation capabilities, available in the NVIDIA Isaac Sim April release, will help save time when building virtual environments to test and train AMRs. Using 3D building blocks, developers can rapidly create realistic complex warehouse scenes and configurations to validate the robot’s performance on a breadth of logistics tasks.
Isaac Nova Orin Key Features
Nova Orin comes with all of the compute and sensor hardware needed to design, build and test autonomy in AMRs.
Its two Jetson AGX Orin units provide upto 550 TOPS of AI compute for perception, navigation and human-machine interaction. These modules process data in real time from the AMR’s central nervous system — essentially the sensor suite comprising up to six cameras, three lidars and eight ultrasonic sensors.
Nova Orin includes tools necessary to simulate the robot in Isaac Sim on Omniverse, as well as support for numerous ROS software modules designed to accelerate perception and navigation tasks. Tools are also provided for accurately mapping the robots’ environment using NVIDIA DeepMap.
The entire platform is calibrated and tested to work out of the box and give developers valuable time to innovate on new features and capabilities.
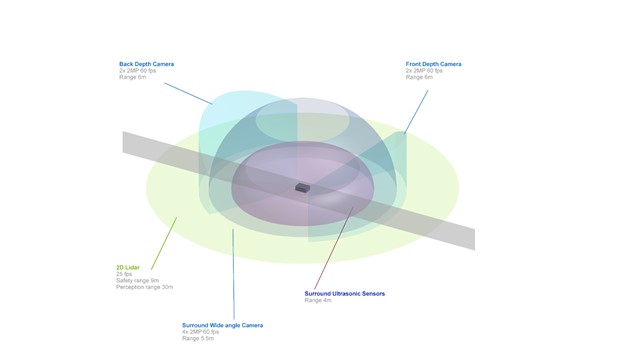
Enabling the Future
Much is at stake in intralogistics for AMRs, a market expected to top $46 billion by 2030, up from under $8 billion in 2021, according to estimates from ABI Research.
The old method of designing the AMR compute and sensor stack from the ground up is too costly in time and effort. Tapping into an existing platform allows manufacturers to focus on building the right software stack for the right robot application.
Improving productivity for factories and warehouses will depend on AMRs working safely and efficiently side by side at scale. High levels of autonomy driven by 3D perception from Nova Orin will help drive that revolution.
As AMRs evolve, the need for secure deployment and management of the critical AI software on board is paramount. Over-the-air software management support is already preintegrated in Nova Orin.
Learn more about Nova Orin and the complete Isaac for AMR platform.
The post NVIDIA Unveils Isaac Nova Orin to Accelerate Development of Autonomous Mobile Robots appeared first on NVIDIA Blog.