FORMULA 1 (F1) turns 70 years old in 2020 and is one of the few sports that combines real-time skill with engineering and technical prowess. Technology has always played a central role in F1; where the evolution of the rules and tools is built into the DNA of F1. This keeps fans engaged and drivers and teams always pushing as races are won and lost in tenths of a second.
With pit stops from well over a minute to under 2 seconds, 5g cornering and braking, speeds up to 375 KPH, and racing in 22 countries, no sport has been as dynamic in its evolution and embrace of new technology. FORMULA 1 seeks to innovate continuously and some of the latest innovations are going to enhance the experiences of its growing base of over a half a billion fans, and an improved understanding of what happens on and off the track through the power of data and analytics, by bringing the split-second decisions made by drivers and teams to the viewers.
With 300 sensors on each race car generating 1.1M data points per second transmitted from the car to the pit, the fan experience has downshifted from reactive to real time, which accelerates the action on the track. F1 can pinpoint how a driver is performing and whether they are pushing the car over the limit by using cloud-native technologies, such as machine learning (ML) models created in Amazon SageMaker and hosted on AWS Lambda. As a result, they can predict the outcome of an overtake or pit stop battle. They can share these insights immediately with fans all over the world through broadcast partners and digital platforms.
This post takes a deep dive into how the Amazon ML Solutions Lab and Professional Services Teams worked with F1 to build a real-time race strategy prediction application using AWS technology that brings “Pit-Wall” decisions to the viewer and resulted in the Pit Strategy Battle graphic. The post discusses race strategies and how to translate them into application logic, all while working backwards from a concept with multiple teams in parallel. You can also learn how a serverless architecture can provide ML predictions with minimal latency across the globe, and how to get started on your own ML journey.
To pit or not to pit
To a fan, 20 drivers and 10 teams on the race track can feel like chaos. But drivers and engineers employ different strategies to get more out of their race cars and get an edge over their competitors. While some are well-calculated risks and others are wild gambles, all are critical to a race outcome, sometimes coming down to split seconds, and all contribute to the spectacular adrenaline rush that keeps fans coming back for more. F1 wants to pull back the curtain for their fans to provide a glimpse into how they make these decisions and their impact on battles as they unfold.
Tire condition is a critical factor that affects the performance of a race car. It is not possible for a driver to stay competitive and finish a race on a single set of tires. Teams choose between varying tire compounds that balance performance and resilience. Softer compounds provide superior grip and handling in exchange for faster degradation, and harder compounds provide superior durability but limit cornering speed and traction. Drivers and teams decide when and how often to pit, but the rules require that drivers make a pit stop at least once per grand prix.
A fresh set of tires can significantly boost a vehicle’s performance, thus increasing the driver’s chance of overtaking another car. However, this comes at a cost—around 20 seconds on average to make a pit stop. Careful planning and execution of when to pit relative to your opponents may give the advantage that delivers victory.
Imagine a battle between two drivers: driver 1 and driver 2. Driver 1 leads and is trying to defend his position, with driver 2 gaining ground to attempt an overtake, which already proves challenging despite his faster pace. Considering that both drivers need to change tires at least once, driver 2 might choose to pit first to get a performance advantage. By pitting early, driver 2 now has the upper hand to close the gap between the cars because driver 1’s tire degradation limits his performance. If driver 2 catches back up to driver 1 after pitting, he can overtake when driver 1 is finally forced to pit. This strategy is called an undercut.
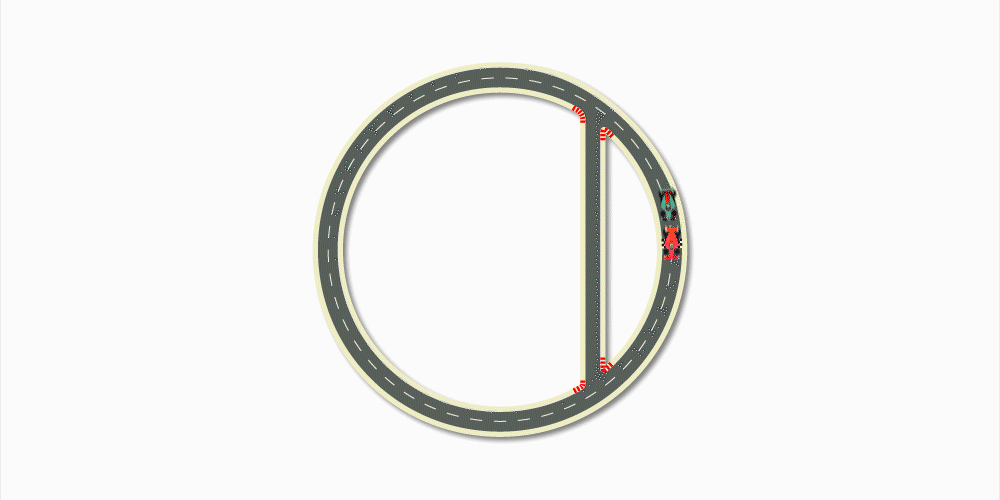
While this may seem obvious, the opposite strategy, an overcut, is sometimes also the case. Driver 2 may decide to push his car as far as he can, hoping that driver 1 pits first, possibly gambling that driver 1’s tires are wearing faster. The calculation here is that having no traffic ahead might be the advantage that driver 2 needs to get ahead. When executed well, the chaser overtakes the leader after an eventual pit stop. With more than two drivers on the track, this gets complex fairly quickly. A given driver is a chaser to some and a leader to others, and such battles may take multiple laps to unfold. For spectators during the chaos of the race, it is nearly impossible to track which drivers have the advantage and which strategies teams employ. Even the most die-hard F1 fan benefits from data analytics to make the complex simple.
F1 partnered with AWS to build new F1 insights, working backwards to build ML models to track pit battles and improve the viewing experience.
Working backwards
AWS starts with the customer and works backwards, which forces us to validate ideas against your values. A Working Backwards document includes three parts: a press release using customer-centric language to describe an idea at a high level, frequently asked questions that customers and internal stakeholders may ask, and visuals to help communicate the idea. When weighing the merits of an idea, it is important to sketch out all possible experience outcomes. It might be a whiteboard sketch, a workflow diagram, or a wire-frame. The following was the initial view for the Pit Strategy Battle use case:
This conceptual illustration allows stakeholders to align on a diverse set of outcomes and goals—graphics applications, application development, ML models, and more—and you can test it with a small user group to verify the desired outcomes. Also, it allows teams to break up the work in chunks to handle in parallel, such as the development of different graphic wire-frames (graphics), collection of data (operations), translation of race logic into application logic (development team), and building the ML models (ML team).
The Working Backwards model provided a clear vision from the outset. We aligned with F1’s broadcast partners on the types of messages and formats used, and illustrators created a video as a proof of concept for the on-screen graphics team.
We used Amazon SageMaker notebooks to do exploratory analysis and visualize large quantities of timing, tire, and weather data uploaded to Amazon S3 to understand how the race looks from an algorithm’s point of view. We determined what strategies were used during past races and what factors determined outcomes, and endlessly replayed races to see what historical features we could extract for our ML models and how to extract those features during a live race.
Having extracted and cleaned the relevant data from various sources, we started on ML tasks. When you start an ML project, you are rarely certain of the best possible outcome that you can achieve. To experiment and iterate quickly, we set two key performance indicators (KPIs):
- Business KPIs – These are designed to communicate the progress to all relevant stakeholders, such as the percentage of predictions within a certain boundary.
- Technical KPIs – These are used to optimize the model, such as root mean square error.
You can use these KPIs, technical requirements, and a set output format in validation code that allows for quick experimentation with feature engineering and various algorithms to optimize for prediction error.
Implementing the architecture
When we were designing how the application architecture would look, we faced many requirements, some of which seemed contradictory at first glance. We achieved our goals by using cloud-native AWS services while focusing on what mattered and spending little overhead on maintenance. And the pay-as-you-go model allowed us to keep costs relatively low.
Architecture overview
The following diagram shows the architecture in detail:
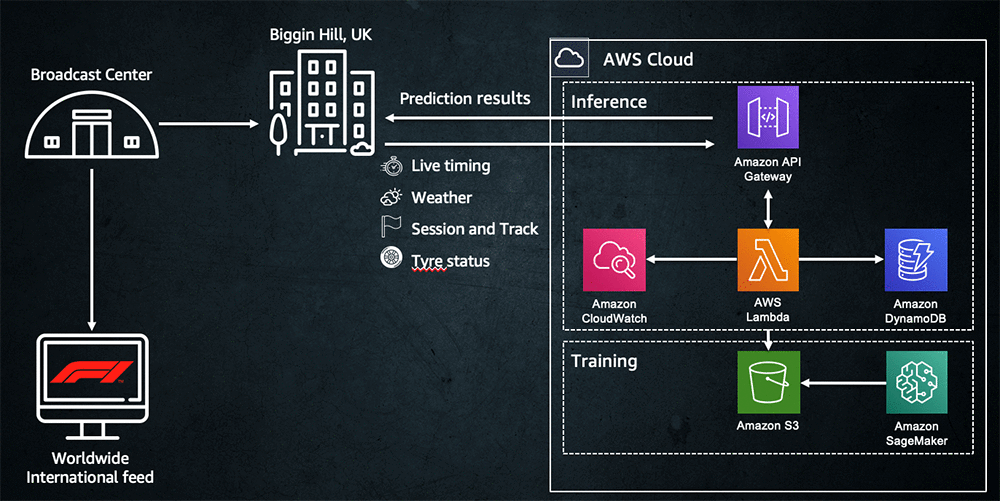
When a signal is captured at the race track, it begins its journey, first passing via F1 infrastructure, then as an HTTP call to the AWS Cloud. Amazon API Gateway acts as the entry point to the application, which is hosted as a function in Lambda, which implements the race logic. When the function receives the incoming message, it updates the race state stored in Amazon DynamoDB (for example, a change in driver position). After the update is finished, the function evaluates whether this is a trigger for prediction. If so, it uses the model trained in Amazon SageMaker to make the prediction. The prediction is sent back as a response to the call and ingested back to the F1 infrastructure. It returns to the broadcasting center and is ready for the race director to use. We needed the whole process to complete in less than 500 milliseconds.
Picking the right tools
The first challenge was that we didn’t know in advance what approaches would work, especially given the tight deadlines. We had to pick a set of tools that would enable us to prototype fast, validate, and experiment, and enable us to move quickly from a proof of concept to a production-ready application. We used serverless products offered by AWS, such as Lambda, API Gateway, DynamoDB, Amazon CloudWatch, and S3. For example, we hosted a prototype on Lambda with zero operational overhead, and when we were satisfied with the results, we could move the application into production with a simple script. We didn’t have to worry about provisioning infrastructure because Lambda automatically scales up your resources when the rate of requests increases. When the race finished, the resources were released without the need for manual actions. Because the predictions are made live, it is critical to have an infrastructure with high availability. Traditionally, building such an infrastructure would require a dedicated skilled team of system engineers. Lambda readily offers highly available infrastructure for any applications.
When the application received a message from the track, the content of a single message was never enough to trigger a prediction. For example, a position change of one driver doesn’t tell much about the whole situation on the track. Because predictions take comprehensive inputs that include past and present situations on the track, we had to employ a database to store and manage the state of the race. DynamoDB was a crucial tool for storing the race state, timing data, the strategies that we were monitoring, and features for ML models. DynamoDB provides single-digit millisecond performance regardless of the number of rows in a table, with no operational overhead. We didn’t have to spend time spinning up and managing database clusters or worrying about uptime.
To automate our iterations from prototype to production, we used CI/CD tools, including AWS CodePipeline and AWS CodeBuild, to segregate environments and move the code to production when it was ready. We used AWS CloudFormation to implement an approach called infrastructure as code (IaC) to provision environments and have predictable deployments.
We used most of these resources only during live races or tests, so we wanted to pay for only the consumed resources. To avoid paying for over-provisioning, we would need to manually start and stop components. The services that we used offer a pay-as-you-go model; the bill included only the exact amount of storage that we used, and the number of calls determined the charges for computational resources. This was possible because we hosted the model on Lambda which is an alternative to hosting models on SageMaker end-points. For more detailed information about hosting models on Lambda you can take a look at this blog post.
Accuracy and performance
When it came to ML models, we based our requirements on accuracy and runtime performance. To achieve accuracy, we needed tools that would enable us to test approaches fast, experiment, and iterate. To train the models, we used Amazon SageMaker; its built-in algorithm XGBoost is a popular and efficient open-source implementation of the gradient boosted trees algorithm. We carefully analyzed racing data and model predictions to extract features that are available in the race data. After we finished the optimal design of the model and input features, we trained the models on historical race data using training jobs in Amazon SageMaker. The benefit of this feature is that it fully implements provisioning and de-provisioning of the resources, while the data scientists can focus on the optimization of the model. In addition, SageMaker allows you to control the instance types and number of instances that you use for training. This is particularly useful when training large data sets.
Although the training time of the algorithms was fairly straightforward, inference had to happen in real time. F1 serves a live stream to hundreds of millions of viewers around the world; for a sport that is being decided in milliseconds, data that is even a few seconds old is obsolete. To meet the required response time, we loaded the model trained in Amazon SageMaker into the application hosted on Lambda and implemented the inference in the function code. Because the model stayed loaded in memory right next to the running code, we could cut the invocation overhead to a bare minimum. We used the built-in open-source algorithm XGBoost to train the model. We recorded the model into a smaller and higher performing format using an additional open-source package, which boosted inference speed and reduced deployment size. Because we hosted the application and models in Lambda, we could scale the infrastructure elastically and easily keep up with the varying prediction rates during the race without operational interventions.
The choice of tools and services is fundamental to a project’s success. Thanks to the breadth and depth of services offered by AWS, we could pick the best-suited tools for our requirements and operational model. And serverless technologies freed up time spent on infrastructure upkeep so we could focus on what mattered most.
Results
The Pit Strategy Battle insight was released on March 17, 2019, at the Australian Grand Prix at the official start of the 2019 F1 season. To show the Pit Strategy Battle graphic used to its fullest potential, we traveled to Bahrain on March 31 for the Bahrain Grand Prix. The Grand Prix was one of the most exhilarating races in the 2019 season, and it was also the stage for a top-class display of Mercedes performing the undercut strategy. The following short clip shows Hamilton chasing down Vettel on fresh new tires from his pit stop one lap earlier, attempting to overtake Vettel while he is making his pit stop on lap 14.
The video shows how Hamilton pulled off a successful undercut. The graphic was used to build the suspense and help the viewer understand what was happening on the track. The application provided live predictions for both the predicted time gap and the overtake probability by using ML models trained on historical data, all within 500 milliseconds.
Summary
Despite F1’s history of technical innovation, we’re just getting started with the volume of data we can now collect—over 300 sensors in each race car produces over 1.1M data points per second. This post showed how the AWS Professional Services team worked with F1 to take this data and apply ML and analytics to help fans get insights and better understand the race. Multiple teams created a shared understanding and had clarity on the end goal by working backwards, which allowed us to work in parallel. This can greatly accelerate a project and remove bottlenecks.
Much like other businesses, F1 is trying to make sense of chaos. You can apply the higher-level services and underlying principles we used to any industry. The use of Lambda for application hosting, DynamoDB for storage, and Amazon SageMaker for model training allows developers and data scientists to focus on what matters. Rather than spending time building and maintaining infrastructure or worrying about uptime and costs, you can focus on translating business knowledge to application logic, experimenting, and iterating quickly.
Whether it’s a company building websites that wants to offer personalized products, factories that want to run more efficiently, or farms that want to increase yield, you can benefit from using data in your respective businesses to develop faster and scale quicker. AWS Professional Services are ready to supplement your team with specialized skills and experience to help you achieve your business outcomes. For more information, see AWS Professional Services, or reach out through your account manager to get in touch.
About the authors
Luuk Figdor is a data scientist in the AWS Professional Services team. He works with clients across industries to help them tell stories with data using machine learning. In his spare time he likes to learn all about the mind and the intersection between psychology, economics and AI.
Andrey Syschikov is a full-stack technologist in the AWS Professional Services team. He helps customers to fulfil their ideas into innovative cloud-based applications. In the rare moments when Andrey is not next to a computer, he enjoys audiobooks, playing piano, and hiking.
Read More