Amazon SageMaker is a fully managed service that provides every machine learning (ML) developer and data scientist with the ability to build, train, and deploy ML models quickly. Amazon SageMaker Studio is a web-based, integrated development environment (IDE) for ML that lets you build, train, debug, deploy, and monitor your ML models. Amazon SageMaker Studio provides all the tools you need to take your models from experimentation to production while boosting your productivity. You can write code, track experiments, visualize data, and perform debugging and monitoring within a single, integrated visual interface.
This post outlines how to configure access control for teams or groups within Amazon SageMaker Studio using attribute-based access control (ABAC). ABAC is a powerful approach that you can utilize to configure Studio so that different ML and data science teams have complete isolation of team resources.
We provide guidance on how to configure Amazon SageMaker Studio access for both AWS Identity and Access Management (IAM) and AWS Single Sign-On (AWS SSO) authentication methods. This post helps you set up IAM policies for users and roles using ABAC principals. To demonstrate the configuration, we set up two teams as shown in the following diagram and showcase two use cases:
- Use case 1 – Only User A1 can access their studio environment; User A2 can’t access User A1’s environment, and vice versa
- Use case 2 – Team B users cannot access artifacts (experiments, etc.) created by Team A members
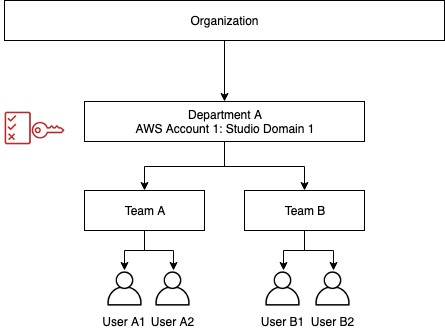
You can configure policies according to your needs. You can even include a project tag in case you want to further restrict user access by projects within a team. The approach is very flexible and scalable.
Authentication
Amazon SageMaker Studio supports the following authentication methods for onboarding users. When setting up Studio, you can pick an authentication method that you use for all your users:
- IAM – Includes the following:
- IAM users – Users managed in IAM
- AWS account federation – Users managed in an external identity provider (IdP)
- AWS SSO – Users managed in an external IdP federated using AWS SSO
Data science user personas
The following table describes two different personas that interact with Amazon SageMaker Studio resources and the level of access they need to fulfill their duties. We use this table as a high-level requirement to model IAM roles and policies to establish desired controls based on resource ownership at the team and user level.
User Personas |
Permissions |
Admin User |
Create, modify, delete any IAM resource.
Create Amazon SageMaker Studio user profiles with a tag.
Sign in to the Amazon SageMaker console.
Read and describe Amazon SageMaker resources.
|
Data Scientists or Developers |
Launch an Amazon SageMaker Studio IDE assigned to a specific IAM or AWS SSO user.
Create Amazon SageMaker resources with necessary tags. For this post, we use the team tag.
Update, delete, and run resources created with a specific tag.
Sign in to the Amazon SageMaker console if an IAM user.
Read and describe Amazon SageMaker resources.
|
Solution overview
We use the preceding requirements to model roles and permissions required to establish controls. The following flow diagram outlines the different configuration steps:
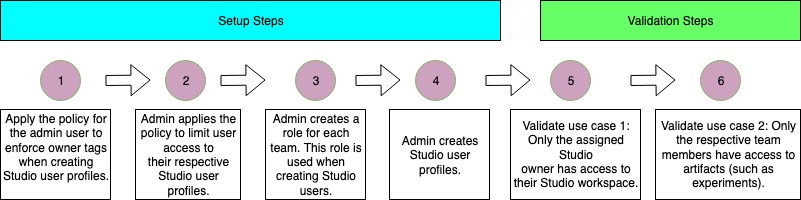
Applying your policy to the admin user
You should apply the following policy to the admin user who creates Studio user profiles. This policy requires the admin to include the studiouserid
tag. You could use a different name for the tag if need be. The Studio console doesn’t allow you to add tags when creating user profiles, so we use the AWS Command Line Interface (AWS CLI).
For admin users managed in IAM, attach the following policy to the user. For admin users managed in an external IdP, add the following policy to the rule that the user assumes upon federation. The following policy enforces the studiouserid
tag to be present when the sagemaker:CreateUserProfile
action is invoked.
{
"Version": "2012-10-17",
"Statement": [
{
"Sid": "CreateSageMakerStudioUserProfilePolicy",
"Effect": "Allow",
"Action": "sagemaker:CreateUserProfile",
"Resource": "*",
"Condition": {
"ForAnyValue:StringEquals": {
"aws:TagKeys": [
"studiouserid"
]
}
}
}
]
}
AWS SSO doesn’t require this policy; it performs the identity check.
Assigning the policy to Studio users
The following policy limits Studio access to the respective users by requiring the resource tag to match the user name for the sagemaker:CreatePresignedDomainUrl
action. When a user tries to access the Amazon SageMaker Studio launch URL, this check is performed.
For IAM users, attach the following policy to the user. Use the user name for the studiouserid tag value.
{
"Version": "2012-10-17",
"Statement": [
{
"Sid": "AmazonSageMakerPresignedUrlPolicy",
"Effect": "Allow",
"Action": [
"sagemaker:CreatePresignedDomainUrl"
],
"Resource": "*",
"Condition": {
"StringEquals": {
"sagemaker:ResourceTag/studiouserid": "${aws:username}"
}
}
}
]
}
For AWS account federation, attach the following policy to role that the user assumes after federation:
{
"Version": "2012-10-17",
"Statement": [
{
"Sid": "AmazonSageMakerPresignedUrlPolicy",
"Effect": "Allow",
"Action": [
"sagemaker:CreatePresignedDomainUrl"
],
"Resource": "*",
"Condition": {
"StringEquals": {
"sagemaker:ResourceTag/studiouserid": "${aws:PrincipalTag/studiouserid}"
}
}
}
]
}
Add the following statement to this policy in the Trust Relationship section. This statement defines the allowed transitive tag.
"Statement": [
{
--Existing statements
},
{
"Sid": "IdentifyTransitiveTags",
"Effect": "Allow",
"Principal": {
"Federated": "arn:aws:iam::<account id>:saml-provider/<identity provider>"
},
"Action": "sts:TagSession",
"Condition": {
"ForAllValues:StringEquals": {
"sts:TransitiveTagKeys": [
"studiouserid"
]
}
}
]
For users managed in AWS SSO, this policy is not required. AWS SSO performs the identity check.
Creating roles for the teams
To create roles for your teams, you must first create the policies. For simplicity, we use the same policies for both teams. In most cases, you just need one set of policies for all teams, but you have the flexibility to create different policies for different teams. In the second step, you create a role for each team, attach the policies, and tag the roles with appropriate team tags.
Creating the policies
Create the following policies. For this post, we split them into three policies for more readability, but you can create them according to your needs.
Policy 1: Amazon SageMaker read-only access
The following policy gives privileges to List and Describe Amazon SageMaker resources. You can customize this policy according to your needs.
{
"Version": "2012-10-17",
"Statement": [
{
"Sid": "AmazonSageMakerDescribeReadyOnlyPolicy",
"Effect": "Allow",
"Action": [
"sagemaker:Describe*",
"sagemaker:GetSearchSuggestions"
],
"Resource": "*"
},
{
"Sid": "AmazonSageMakerListOnlyPolicy",
"Effect": "Allow",
"Action": [
"sagemaker:List*"
],
"Resource": "*"
},
{
"Sid": "AmazonSageMakerUIandMetricsOnlyPolicy",
"Effect": "Allow",
"Action": [
"sagemaker:*App",
"sagemaker:Search",
"sagemaker:RenderUiTemplate",
"sagemaker:BatchGetMetrics"
],
"Resource": "*"
},
{
"Sid": "AmazonSageMakerEC2ReadOnlyPolicy",
"Effect": "Allow",
"Action": [
"ec2:DescribeDhcpOptions",
"ec2:DescribeNetworkInterfaces",
"ec2:DescribeRouteTables",
"ec2:DescribeSecurityGroups",
"ec2:DescribeSubnets",
"ec2:DescribeVpcEndpoints",
"ec2:DescribeVpcs"
],
"Resource": "*"
},
{
"Sid": "AmazonSageMakerIAMReadOnlyPolicy",
"Effect": "Allow",
"Action": [
"iam:ListRoles"
],
"Resource": "*"
}
]
}
Policy 2: Amazon SageMaker access for supporting services
The following policy gives privileges to create, read, update, and delete access to Amazon Simple Storage Service (Amazon S3), Amazon Elastic Container Registry (Amazon ECR), and Amazon CloudWatch, and read access to AWS Key Management Service (AWS KMS). You can customize this policy according to your needs.
{
"Version": "2012-10-17",
"Statement": [
{
"Sid": "AmazonSageMakerCRUDAccessS3Policy",
"Effect": "Allow",
"Action": [
"s3:PutObject",
"s3:GetObject",
"s3:AbortMultipartUpload",
"s3:DeleteObject",
"s3:CreateBucket",
"s3:ListBucket",
"s3:PutBucketCORS",
"s3:ListAllMyBuckets",
"s3:GetBucketCORS",
"s3:GetBucketLocation"
],
"Resource": "<S3 BucketName>"
},
{
"Sid": "AmazonSageMakerReadOnlyAccessKMSPolicy",
"Effect": "Allow",
"Action": [
"kms:DescribeKey",
"kms:ListAliases"
],
"Resource": "*"
},
{
"Sid": "AmazonSageMakerCRUDAccessECRPolicy",
"Effect": "Allow",
"Action": [
"ecr:Set*",
"ecr:CompleteLayerUpload",
"ecr:Batch*",
"ecr:Upload*",
"ecr:InitiateLayerUpload",
"ecr:Put*",
"ecr:Describe*",
"ecr:CreateRepository",
"ecr:Get*",
"ecr:StartImageScan"
],
"Resource": "*"
},
{
"Sid": "AmazonSageMakerCRUDAccessCloudWatchPolicy",
"Effect": "Allow",
"Action": [
"cloudwatch:Put*",
"cloudwatch:Get*",
"cloudwatch:List*",
"cloudwatch:DescribeAlarms",
"logs:Put*",
"logs:Get*",
"logs:List*",
"logs:CreateLogGroup",
"logs:CreateLogStream",
"logs:ListLogDeliveries",
"logs:Describe*",
"logs:CreateLogDelivery",
"logs:PutResourcePolicy",
"logs:UpdateLogDelivery"
],
"Resource": "*"
}
]
}
Policy 3: Amazon SageMaker Studio developer access
The following policy gives privileges to create, update, and delete Amazon SageMaker Studio resources.
It also enforces the team tag requirement during creation. In addition, it enforces start, stop, update, and delete actions on resources restricted only to the respective team members.
The team tag validation condition in the following code makes sure that the team tag value matches the principal’s team. Refer to the bolded code for specifcs.
{
"Version": "2012-10-17",
"Statement": [
{
"Sid": "AmazonSageMakerStudioCreateApp",
"Effect": "Allow",
"Action": [
"sagemaker:CreateApp"
],
"Resource": "*"
},
{
"Sid": "AmazonSageMakerStudioIAMPassRole",
"Effect": "Allow",
"Action": [
"iam:PassRole"
],
"Resource": "*"
},
{
"Sid": "AmazonSageMakerInvokeEndPointRole",
"Effect": "Allow",
"Action": [
"sagemaker:InvokeEndpoint"
],
"Resource": "*"
},
{
"Sid": "AmazonSageMakerAddTags",
"Effect": "Allow",
"Action": [
"sagemaker:AddTags"
],
"Resource": "*"
},
{
"Sid": "AmazonSageMakerCreate",
"Effect": "Allow",
"Action": [
"sagemaker:Create*"
],
"Resource": "*",
"Condition": { "ForAnyValue:StringEquals": { "aws:TagKeys": [ "team" ] }, "StringEqualsIfExists": { "aws:RequestTag/team": "${aws:PrincipalTag/team}" } }
},
{
"Sid": "AmazonSageMakerUpdateDeleteExecutePolicy",
"Effect": "Allow",
"Action": [
"sagemaker:Delete*",
"sagemaker:Stop*",
"sagemaker:Update*",
"sagemaker:Start*",
"sagemaker:DisassociateTrialComponent",
"sagemaker:AssociateTrialComponent",
"sagemaker:BatchPutMetrics"
],
"Resource": "*",
"Condition": { "StringEquals": { "aws:PrincipalTag/team": "${sagemaker:ResourceTag/team}" } }
}
]
}
Creating and configuring the roles
You can now create a role for each team with these policies. Tag the roles on the IAM console or with the CLI command. The steps are the same for all three authentication types. For example, tag the role for Team A with the tag key= team and value
= “<Team Name>”.
Creating the Amazon SageMaker Studio user profile
In this step, we add the studiouserid
tag when creating Studio user profiles. The steps are slightly different for each authentication type.
IAM users
For IAM users, you create Studio user profiles for each user by including the role that was created for the team the user belongs to. The following code is a sample CLI command. As of this writing, including a tag when creating a user profile is available only through AWS CLI.
aws sagemaker create-user-profile --domain-id <domain id> --user-profile-name <unique profile name> --tags Key=studiouserid,Value=<aws user name> --user-settings ExecutionRole=arn:aws:iam::<account id>:role/<Team Role Name>
AWS account federation
For AWS account federation, you create a user attribute (studiouserid
) in an external IdP with a unique value for each user. The following code shows how to configure the attribute in Okta:
Example below shows how to add “studiouserid” attribute in OKTA. In OKTA’s SIGN ON METHODS screen, configure following SAML 2.0 attributes, as shown in the image below.
Attribute 1:
Name: https://aws.amazon.com/SAML/Attributes/PrincipalTag:studiouserid
Value: user.studiouserid
Attribute 2:
Name: https://aws.amazon.com/SAML/Attributes/TransitiveTagKeys
Value: {"studiouserid"}
The following screenshot shows the attributes on the Okta console.
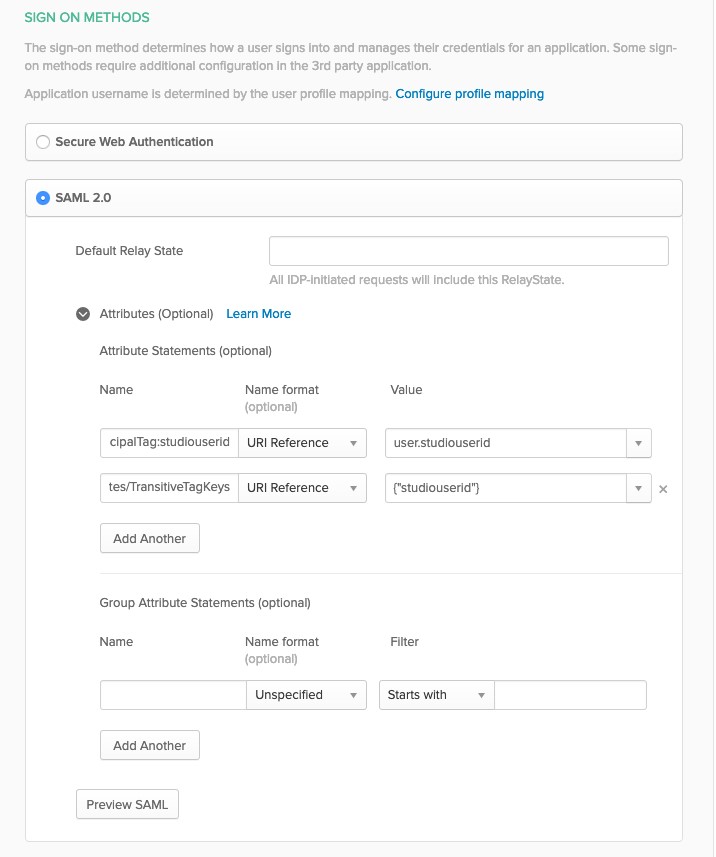
Next, create the user profile using the following command. Use the user attribute value in the preceding step for the studiouserid
tag value.
aws sagemaker create-user-profile --domain-id <domain id> --user-profile-name <unique profile name> --tags Key=studiouserid,Value=<user attribute value> --user-settings ExecutionRole=arn:aws:iam::<account id>:role/<Team Role Name>
AWS SSO
For instructions on assigning users in AWS SSO, see Onboarding Amazon SageMaker Studio with AWS SSO and Okta Universal Directory.
Update the Studio user profile to include the appropriate execution role that was created for the team that the user belongs to. See the following CLI command:
aws sagemaker update-user-profile --domain-id <domain id> --user-profile-name <user profile name> --user-settings ExecutionRole=arn:aws:iam::<account id>:role/<Team Role Name> --region us-west-2
Validating that only assigned Studio users can access their profiles
When a user tries to access a Studio profile that doesn’t have studiouserid
tag value matching their user name, an AccessDeniedException
error occurs. You can test this by copying the link for Launch Studio on the Amazon SageMaker console and accessing it when logged in as a different user. The following screenshot shows the error message.

Validating that only respective team members can access certain artifacts
In this step, we show how to configure Studio so that members of a given team can’t access artifacts that another team creates.
In our use case, a Team A user creates an experiment and tags that experiment with the team tag. This limits access to this experiment to Team A users only. See the following code:
import sys
!{sys.executable} -m pip install sagemaker
!{sys.executable} -m pip install sagemaker-experiments
import time
import sagemaker
from smexperiments.experiment import Experiment
demo_experiment = Experiment.create(experiment_name = "USERA1TEAMAEXPERIMENT1",
description = "UserA1 experiment",
tags = [{'Key': 'team', 'Value': 'TeamA'}])
If a user who is not in Team A tries to delete the experiment, Studio denies the delete action. See the following code:
#command run from TeamB User Studio Instance
import time
from smexperiments.experiment import Experiment
experiment_to_cleanup = Experiment.load(experiment_name="USERA1TEAMAEXPERIMENT1")
experiment_to_cleanup.delete()
[Client Error]
An error occurred (AccessDeniedException) when calling the DeleteExperiment operation: User: arn:aws:sts:: :<AWS Account ID>::assumed-role/ SageMakerStudioDeveloperTeamBRole/SageMaker is not authorized to perform: sagemaker:DeleteExperiment on resource: arn:aws:sagemaker:us-east-1:<AWS Account ID>:experiment/usera1teamaexperiment1
Conclusion
In this post, we demonstrated how to isolate Amazon SageMaker Studio access using the ABAC technique. We showcased two use cases: restricting access to a Studio profile to only the assigned user (using the studiouserid
tag) and restricting access to Studio artifacts to team members only. We also showed how to limit access to experiments to only the members of the team using the team tag. You can further customize policies by applying more tags to create more complex hierarchical controls.
Try out this solution for isolating resources by teams or groups in Amazon SageMaker Studio. For more information about using ABAC as an authorization strategy, see What is ABAC for AWS?
About the Authors
Vikrant Kahlir is Senior Solutions Architect in the Solutions Architecture team. He works with AWS strategic customers product and engineering teams to help them with technology solutions using AWS services for Managed Databases, AI/ML, HPC, Autonomous Computing, and IoT.
Rakesh Ramadas is an ISV Solution Architect at Amazon Web Services. His focus areas include AI/ML and Big Data.
Rama Thamman is a Software Development Manager with the AI Platforms team, leading the ML Migrations team.
Read More