Have you ever thought about how artificial intelligence could be used to detect events during live sports broadcasts? With machine learning (ML) techniques, we introduce a scalable multimodal solution for event detection on sports video data. Recent developments in deep learning show that event detection algorithms are performing well on sports data [1]; however, they’re dependent upon the quality and amount of data used in model development. This post explains a deep learning-based approach developed by the Amazon Machine Learning Solutions Lab for sports event detection using Amazon SageMaker. This approach minimizes the impact of low-quality data in terms of labeling and image quality while improving the performance of event detection. Our solution uses a multimodal architecture utilizing video, static images, audio, and optical flow data to develop and fine-tune a model, followed by boosting and a postprocessing algorithm.
We used sports video data that included static 2D images and frames over time and audio data, which enabled us to train separate models in parallel. The outlined approach also enhances the performance of event detection by consolidating the models’ outcomes into one decision-maker using a boosting technique.
In this post, we first give an overview of the data. We then explain the preprocessing workflow, modeling strategy, postprocessing, and present the results.
Dataset
In this exploratory research study, we used the Sports-1 Million dataset [2], which includes 400 classes of short video clips of sports. The videos include the audio channel, enabling us to extract audio samples for multimodal model development. Among the sports in the dataset, we selected the most frequently occurring sports based on their number of data samples, resulting in 89 sports.
We then consolidated the sports in similar categories, resulting in 25 overall classes. The final list of selected sports for modeling is:
['americanfootball', 'athletics', 'badminton', 'baseball', 'basketball', 'bowling', 'boxing', 'cricket', 'cycling', 'fieldhockey', 'football', 'formula1', 'golf', 'gymnastics', 'handball', 'icehockey', 'lacrosse', 'rugby', 'skiing', 'soccer', 'swimming', 'tabletennis', 'tennis', 'volleyball', 'wrestling']
The following graph shows the number of video samples per sports category. Each video is cut into 1-second intervals.
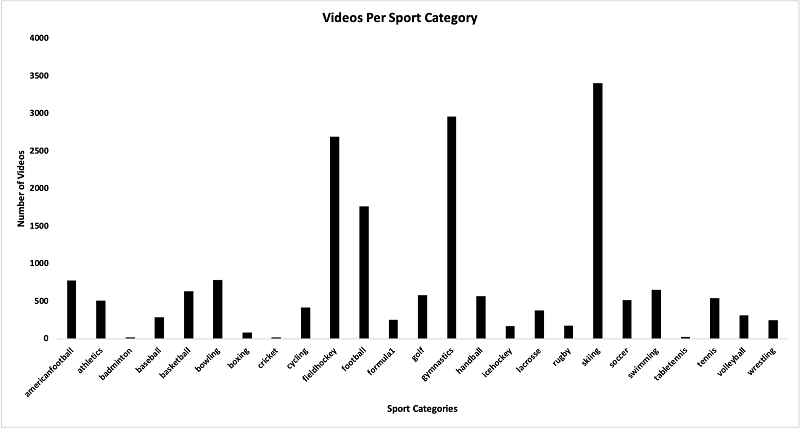
Data processing pipeline
The temporal modeling in this solution uses video clips with 1-second-long durations. Therefore, we first extracted 1-second length video clips from each data example. The average length of videos in the dataset is around 20 seconds, resulting in approximately 190,000 1-second video clips. We passed each second-level video clip through a frame extraction pipeline and, depending on the frames per second (fps) of the video clip, extracted the corresponding number of frames, and stored them in an Amazon Simple Storage Service (Amazon S3) bucket. The total number of frames extracted was around 3.8 million. We performed multi-processing on a SageMaker notebook using an Amazon Elastic Compute Cloud (Amazon EC2) ml.c5.large instance with 64 cores to parallelize the I/O heavy clip-extraction process. Parallelization reduced the clip extraction from hours to minutes.
To train the ML algorithms, we split the data using stratified sampling on the original clips, which prevented potential information leakage down the pipeline. In a classification setting, stratifying helps ensure that the training, validation, and test sets have approximately the same percentage of samples of each target class as the complete set. We split the data into 80/10/10 portions for training, validation, and test sets, respectively. We then reflected this splitting pattern on the 1-second video clips level and the corresponding extracted frames level.
Next, we fine-tuned the ResNet50 architecture using the extracted frames. Additionally, we trained a ResNet50 architecture using dense optical flow features extracted from the frames for each 1-second clip. Finally, we extracted audio features from 1-second clips and implemented an audio model. Each approach represented a modality in the final multimodal technique. The following diagram illustrates the architecture of the data processing and pipeline.
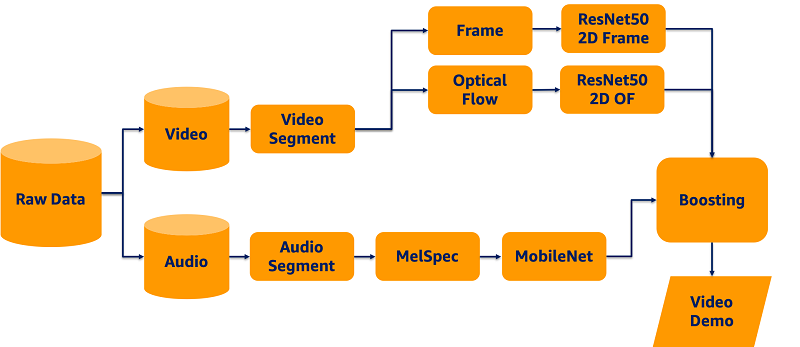
The rest of this section details each modality.
Computer vision
We used two separate computer vision-based approaches to fit the data. First, we used the ResNet50 architecture to fine-tune the multi-class classification algorithm using RBG frames. Second, we used the ResNet50 architecture with the same fine-tuning strategy against optical flow frames. ResNet50 is one of the best classifiers for image data and has been remarkably successful in developing business applications.
We used a two-step fine-tuning approach: we first unfroze the last layer, added two flattened layers to the network, and fine-tuned the results for 10 epochs; we then saved the weights of this model, unfroze all the layers, and trained the entire network on the preceding sports data for 30 epochs. We used TensorFlow with Horovod for training on AWS Deep Learning AMI (DLAMI) instances. You can also use SageMaker Pipe mode to set up Horovod.
Horovod, an open-source framework for distributed deep learning, is available for use with most popular deep learning toolkits, like TensorFlow, Keras, PyTorch, and Apache MXNet. It uses the all-reduce algorithm for fast distributed training rather than using a parameter server approach, and it includes multiple optimization methods to make distributed training faster.
Since completing this project, SageMaker has introduced a new data parallelism library optimized for AWS, which allows you to use existing Horovod APIs. For more information, see New – Managed Data Parallelism in Amazon SageMaker Simplifies Training on Large Datasets.
Optical flow
For the second modality, we used an optical flow approach. The implementations of a classifier, such as ResNet50, on image data only addresses relationships of objects within the same frame, disregarding time information. A model trained this way assumes that frames are independent and unrelated.
To capture the relationships between consecutive frames, such as for recognizing human actions, we can use optical flow. Optical flow is the motion of objects between consecutive frames of sequence caused by the relative movement between the object and camera. We performed a dense optical flow algorithm on the images extracted from each 1-second video. We used OpenCV’s Gunner Farnebäck’s algorithm, which is explained in Farnebäck’s 2003 article “Two-Frame Motion Estimation Based on Polynomial Expansion” [3].
Audio event detection
ML-based audio modeling formed the third stream of our multimodal event detection solution, where audio samples were extracted from 1-second videos, resulting in audio segments in M4A format.
To explore the performance of audio models, two types of features broadly used in digital signal processing were extracted from the audio samples: Mel Spectrogram (MelSpec) and Mel-Frequency Cepstrum coefficient (MFCC). A modified version of MobileNet, a state-of-the-art architecture for audio data classification, was employed for the model development [4].
The audio processing pipeline consists of three steps, including MelSpec and MFCC features and MobileNetV2 model development:
- First, MelSpec refers to the fast Fourier transformation of an audio segment known as spectrogram while considering Mel-Scale. Research has shown that human auditory systems are non-linearly distinguishable between certain frequencies so that the Mel-Scale equalizes the distance between frequency bands audible to a human. For our use case, MelSpec features with 128 points were calculated for model development.
- Second, MFCC is a similar feature to MelSpec, where a linear cosine transformation is applied to the MelSpec feature as research has revealed that such a transformation can improve the performance of classification for audible sound. MFCC features with 33 points were extracted from the audio data; however, the performance of a model based on this feature was unable to compete with MelSpec, suggesting that MFCC often performs better with sequence models.
- Finally, the audio model MobileNetV2 was adopted for our data and trained for 100 epochs with preloaded ImageNet weights. MobileNetV2 [5] is a convolutional neural network architecture that seeks to perform well on mobile devices. It’s based on an inverted residual structure, where the residual connections occur between the bottleneck layers.
Postprocessing
The objective of the postprocessing step employs a boosting algorithm to do the following:
- Obtain video-level performance from the frame level
- Incorporate three models of output into the decision-making process
- Enhance the model performance in prediction using a defined class-model strategy obtained from validation sets and applied to test sets
First, the postprocessing module generated 1-second-level predicted classes and their probabilities for RGB, optical flow, and audio models. We then used a majority voting algorithm to assign the predicted class at the 1-second-level during inference.
Next, the 1-second-level computer vision and audio labels were converted to video-level performance. The results on the validations sets were compared to create a table of classes based on the model-class performance strategy for multimodal prediction against testing sets.
In the final stage, testing sets were passed through the prediction module, resulting in three labels and probabilities.
In this work, the RGB models resulted in the highest performance for all classes except badminton
, where the audio model gave the best performance. The optical flow models didn’t compete with the other two models, although some research has shown that optical flow-based models could generate better results for certain datasets. The final prediction was performed by incorporating all three labels based on the predefined table to output the most probable classes.
The boosting algorithm of the prediction module is described as follows:
- Split videos into 1-second segments.
- Extract frames and audio signals.
- Prepare RGB frames and MelSpec features.
- Pass RGB frames through the trained ResNet50 by RGB samples and obtain prediction labels per frame.
- Pass MelSpec features through the trained MobileNet by audio samples and obtain prediction labels for each 1-second audio sample.
- Calculate 1-second-level RGB labels and probabilities.
- Use a predefined table (obtained from validation results).
- If the
badminton
class is found among two labels associated with a 1-second sample, vote for the audio model (get the label and probability from the audio model). Otherwise, vote for the RBG model (get the label and probability from the RGB model).
Results
The following graph shows the averaged frame-level F1 scores of the three models against two validation datasets; the error bars represent the standard deviations.
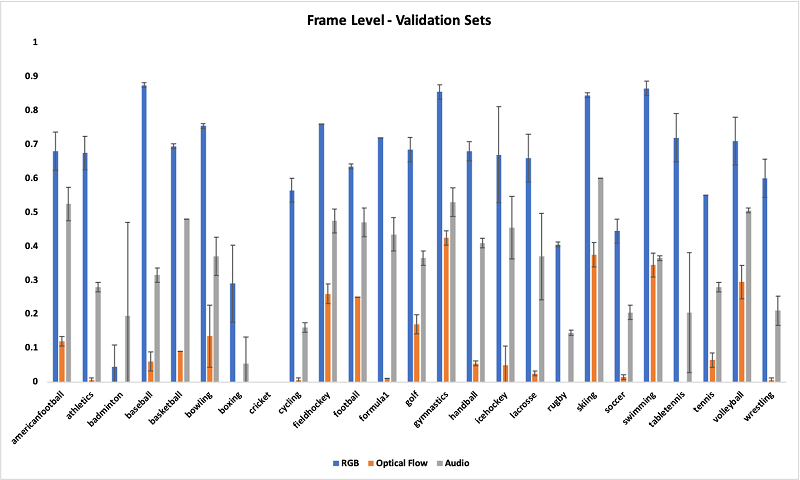
Similarly, the following graph compares the F1 scores for three models per class measured for two testing datasets before postprocessing (average and standard deviation as error bars).
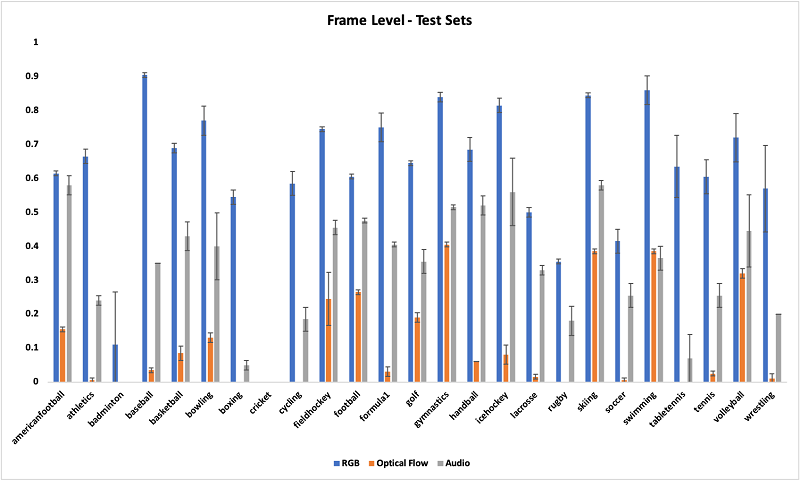
After applying the multimodal prediction module to the testing datasets to convert frame-level and 1-second-level predictions, the postprocessed video-level metrics were produced (see the following graph) and showed a significant improvement from the frame-level single modality to the video-level multimodal outputs.
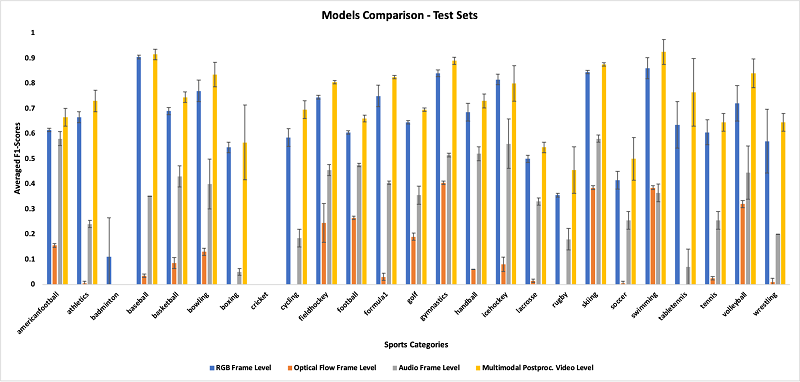
As previously mentioned, the class-model table was prepared using the comparison of three models for validation sets.
The analysis demonstrated that the multimodal approach could improve the performance of multi-class event detection by 5.10%, 55.68%, and 34.2% for single RGB, optical flow, and audio models, respectively. In addition, the confusion matrices for postprocessed testing datasets, shown in the following figures, indicated that the multimodal approach could predict most classes in a challenging 25-class event detection task.
The following figure shows the video-level confusion matrix of the first testing dataset after postprocessing.
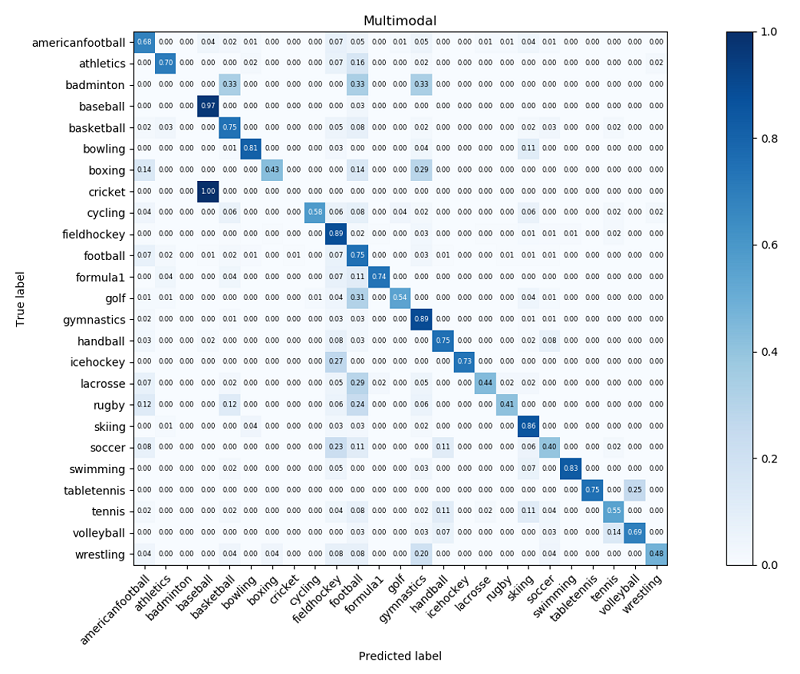
The following figure shows the video-level confusion matrix of the second testing dataset after postprocessing.
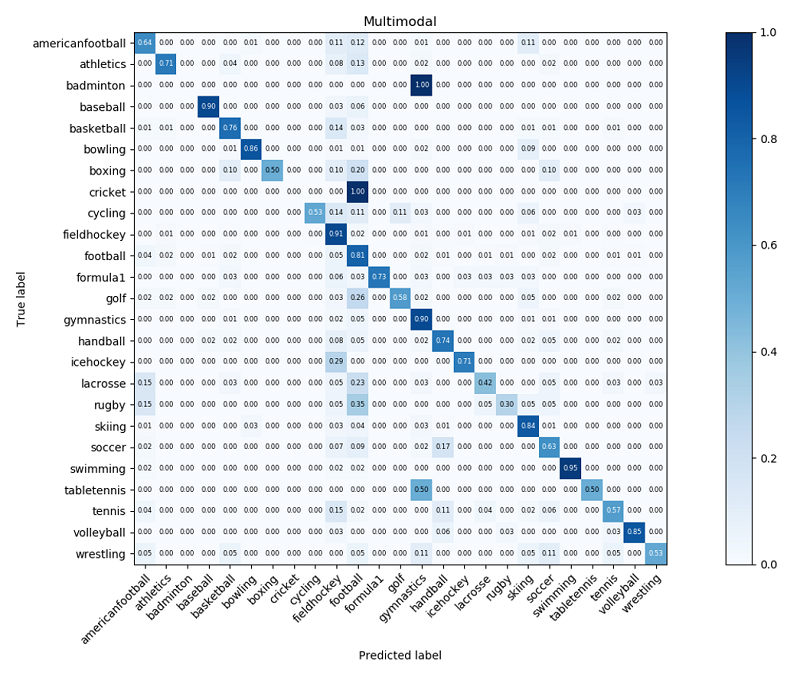
The modeling workflow explained in this post assumes that the data examples in the dataset are all relevant, are all labeled correctly, and have similar distributions among each class. However, the authors’ manual observation of the data sometimes found substantial differences in video footage from one sample to another in the same class. Therefore, one of the areas of improvement that can have great impact on the performance of the model is to further prune the dataset to only include the relevant training examples and provide better labeling.
We used the multimodal model prediction against the testing dataset to generate the following demo for 25 sports, where the bars demonstrate the probability of each class per second (we called it 1-second-level prediction).
Conclusion
This post outlined a multimodal event detection approach using a combination of RGB, optical flow, and audio models through robust ResNet50 and MobileNet architectures implemented on SageMaker. The results of this study demonstrated that, by using a parallel model development, multimodal event detection improved the performance of a challenging 25-class event detection task in sports.
A dynamic postprocessing module enables you to update predictions after new training to enhance the model’s performance against new data.
About Amazon ML Solutions Lab
The Amazon ML Solutions Lab pairs your team with ML experts to help you identify and implement your organization’s highest value ML opportunities. If you’d like help accelerating your use of ML in your products and processes, please contact the Amazon ML Solutions Lab.
Disclaimer
Editor’s note: The dataset used in this post is for non-commercial demonstration and exploratory research.
References
[1] Vats, Kanav, Mehrnaz Fani, Pascale Walters, David A. Clausi, and John Zelek. “Event Detection in Coarsely Annotated Sports Videos via Parallel Multi-Receptive Field 1D Convolutions.” In
Proceedings of the IEEE/CVF Conference on Computer Vision and Pattern Recognition Workshops, pp. 882-883. 2020.
[2] Karpathy, Andrej, George Toderici, Sanketh Shetty, Thomas Leung, Rahul Sukthankar, and Li Fei-Fei. “Large-scale video classification with convolutional neural networks.” In
Proceedings of the IEEE Conference on Computer Vision and Pattern Recognition, pp. 1725-1732. 2014.
[3] Farnebäck, Gunnar. “Two-frame motion estimation based on polynomial expansion.” In
Scandinavian Conference on Image Analysis, pp. 363-370. Springer, Berlin, Heidelberg, 2003.
[4] Adapa, Sainath. “Urban sound tagging using convolutional neural networks.”
arXiv preprint arXiv:1909.12699 (2019).
[5] Sandler, Mark, Andrew Howard, Menglong Zhu, Andrey Zhmoginov, and Liang-Chieh Chen. “Mobilenetv2: Inverted residuals and linear bottlenecks.” In
Proceedings of the IEEE Conference on Computer Vision and Pattern Recognition, pp. 4510-4520. 2018.
About the Authors
Saman Sarraf is a Data Scientist at the Amazon ML Solutions Lab. His background is in applied machine learning including deep learning, computer vision, and time series data prediction.
Mehdi Noori is a Data Scientist at the Amazon ML Solutions Lab, where he works with customers across various verticals, and helps them to accelerate their cloud migration journey and solve their ML problems using state-of-the-art solutions and technologies.
Read More