This post is co-authored by Salma Taoufiq and Harini Kannan from Sophos.
As a leader in next-generation cybersecurity, Sophos strives to protect more than 500,000 organizations and millions of customers across over 150 countries against evolving threats. Powered by threat intelligence, machine learning (ML), and artificial intelligence from Sophos X-Ops, Sophos delivers a broad and varied portfolio of advanced products and services to secure and defend users, networks, and endpoints against phishing, ransomware, malware, and the wide range of cyberattacks out there.
The Sophos Artificial Intelligence (AI) group (SophosAI) oversees the development and maintenance of Sophos’s major ML security technology. Security is a big-data problem. To evade detection, cybercriminals are constantly crafting novel attacks. This translates into colossal threat datasets that the group must work with to best defend customers. One notable example is the detection and elimination of files that were cunningly laced with malware, where the datasets are in terabytes.
In this post, we focus on Sophos’s malware detection system for the PDF file format specifically. We showcase how SophosAI uses Amazon SageMaker distributed training with terabytes of data to train a powerful lightweight XGBoost (Extreme Gradient Boosting) model. This allows their team to iterate over large training data faster with automatic hyperparameter tuning and without managing the underlying training infrastructure.
The solution is currently seamlessly integrated into the production training pipeline and the model deployed on millions of user endpoints via the Sophos endpoint service.
Use case context
Whether you want to share an important contract or preserve the fancy design of your CV, the PDF format is the most common choice. Its widespread use and the general perception that such documents are airtight and static have lulled users into a false sense of security. PDF has, therefore, become an infection vector of choice in attackers’ arsenal. Malicious actions using PDFs are most often achieved via embedding a JavaScript payload that is run by the PDF reader to download a virus from a URI, sabotage the user’s machine, or steal sensitive information.
Sophos detects malicious PDF files at various points of an attack using an ensemble of deterministic and ML models. One such approach is illustrated in the following diagram, where the malicious PDF file is delivered through email. As soon as a download attempt is made, it triggers the malicious executable script to connect to the attacker’s Command and Control server. SophosAI’s PDF detector blocks the download attempt after detecting that it’s malicious.
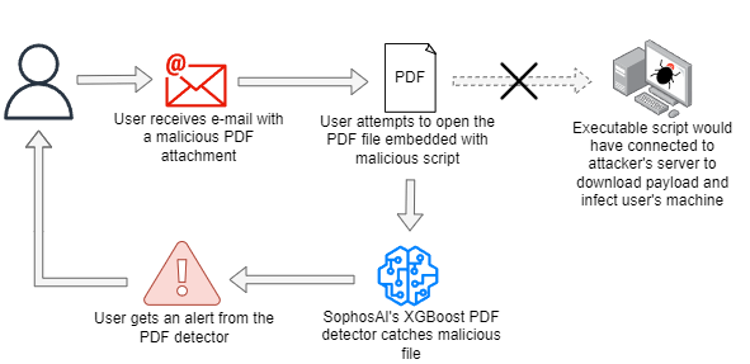
Other ways include blocking the PDF files in the endpoint, sending the malicious files to a sandbox (where it’s scored using multiple models), submitting the malicious file to a scoring infrastructure and generating a security report, and so on.
Motivation
To build a tree-based detector that can convict malicious PDFs with high confidence, while allowing for low endpoint computing power consumption and fast inference responses, the SophosAI team found the XGBoost algorithm to be a perfect candidate for the task. Such research avenues are important for Sophos for two reasons. Having powerful yet small models deployed at the level of customer endpoints has a high impact on the company’s product reviews by analysts. It also, and more importantly, provides a better user experience overall.
Technical challenge
Because the goal was to have a model with a smaller memory footprint than their existing PDF malware detectors (both on disk and in memory), SophosAI turned XGBoost, a classification algorithm with a proven record of producing drastically smaller models than neural networks while achieving impressive performance on tabular data. Before venturing into modeling XGBoost experiments, an important consideration was the sheer size of the dataset. Indeed, Sophos’s core dataset of PDF files is in terabytes.
Therefore, the main challenge was training the model with a large dataset without having to downsample. Because it’s crucial for the detector to learn to spot any PDF-based attacks — even needle-in-the-haystack and completely novel ones to better defend Sophos customers — it’s of the utmost importance to use all available diverse datasets.
Unlike neural networks, where you can train in batches, for XGBoost, we need the entire training dataset in memory. The largest training dataset for this project is over 1 TB, and there is no way to train on such a scale without utilizing the methodologies of a distributed training framework.
Solution overview
SageMaker is a fully managed ML service providing various tools to build, train, optimize, and deploy ML models. The SageMaker built-in libraries of algorithms consist of 21 popular ML algorithms, including XGBoost. (For more information, see Simplify machine learning with XGBoost and Amazon SageMaker.) With the XGBoost built-in algorithm, you can take advantage of the open-source SageMaker XGBoost Container by specifying a framework version greater than 1.0-1, which has improved flexibility, scalability, extensibility, and Managed Spot Training, and supports input formats like Parquet, which is the format used for the PDF dataset.
The main reason SophosAI chose SageMaker is the ability to benefit from the fully managed distributed training on multi-node CPU instances by simply specifying more than one instance. SageMaker automatically splits the data across nodes, aggregates the results across peer nodes, and generates a single model. The instances can be Spot Instances, thereby significantly reducing the training costs. With the built-in algorithm for XGBoost, you can do this without any additional custom script. Distributed versions of XGBoost also exist as open source, such as XGBoost-Ray and XGBoost4J-Spark, but their use requires building, securing, tuning, and self-managing distributed computing clusters, which represents significant effort additional to scientific development.
Additionally, SageMaker automatic model tuning, also known as hyperparameter tuning, finds the best version of a model by running many training jobs with ranges of hyperparameters that you specify. It then chooses the hyperparameter values that result in a model that performs the best, as measured by a metric for the given ML task.
The following diagram illustrates the solution architecture.
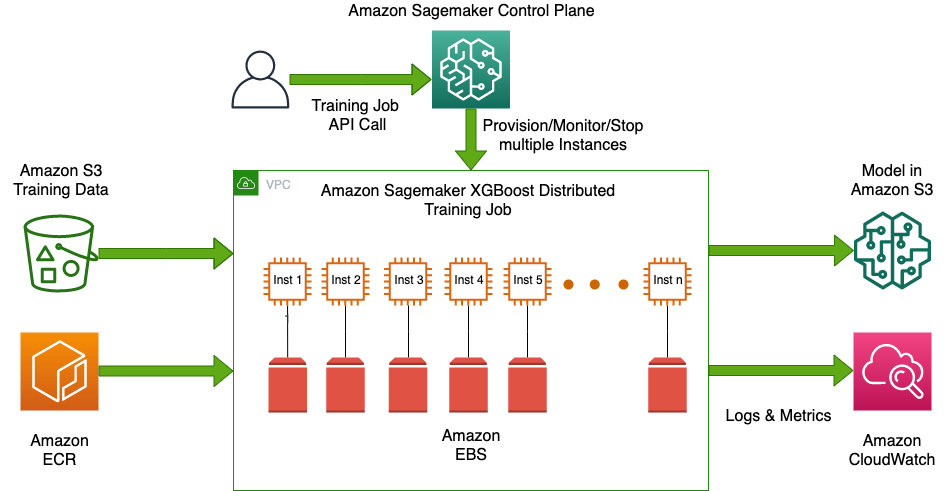
It’s worth noting that, when SophosAI started XGBoost experiments before turning to SageMaker, attempts were made to use large-memory Amazon Elastic Compute Cloud (Amazon EC2) instances (for example, r5a.24xlarge and x1.32xlarge) to train the model on as large of a sample of the data as possible. However, these attempts took more than 10 hours on average and usually failed due to running out of memory.
In contrast, by using the SageMaker XGBoost algorithm and a hassle-free distributed training mechanism, SophosAI could train a booster model at scale on the colossal PDF training dataset in a matter of 20 minutes. The team only had to store the data on Amazon Simple Storage Service (Amazon S3) as Parquet files of similar size, and choose an EC2 instance type and the desired number of instances, and SageMaker managed the underlying compute cluster infrastructure and distributed training between multiple nodes of the cluster. Under the hood, SageMaker splits the data across nodes using ShardedByS3Key to distribute the file objects equally between each instance and uses XGBoost implementation of the Rabit protocol (reliable AllReduce and broadcast interface) to launch distributed processing and communicate between primary and peer nodes. (For more details on the histogram aggregation and broadcast across nodes, refer to XGBoost: A Scalable Tree Boosting System.)
Beyond just training one model, with SageMaker, XGBoost hyperparameter tuning was also made quick and easy with the ability to run different experiments simultaneously to fine-tune the best combination of hyperparameters. The tunable hyperparameters include both booster-specific and objective function-specific hyperparameters. Two search strategies are offered: random or Bayesian. The Bayesian search strategy has proven to be valuable because it helps find better hyperparameters than a mere random search, in fewer experimental iterations.
Dataset information
SophosAI’s PDF malware detection modeling relies on a variety of features such as n-gram histograms and byte entropy features (For more information, refer to MEADE: Towards a Malicious Email Attachment Detection Engine). Metadata and features extracted from collected PDF files are stored in a distributed data warehouse. A dataset of over 3,500 features is then computed, further split based on time into training and test sets and stored in batches as Parquet files in Amazon S3 to be readily accessible by SageMaker for training jobs.
The following table provides information about the training and test data.
Dataset |
Number of Samples |
Number of Parquet Files |
Total Size |
Training |
70,391,634 |
5,500 |
~1010 GB |
Test |
1,242,283 |
98 |
~18 GB |
The data sizes have been computed following the formula:
Data Size = N × (nF + nL) × 4
The formula has the following parameters:
-
N is the number of samples in the dataset
-
nF is the number of features, with nF = 3585
-
nL is the number of ground truth labels, with nL = 1
- 4 is the number of bytes needed for the features’ data type:
float32
Additionally, the following pie charts provide the label distribution of both the training and test sets, eliciting the class imbalance faced in the PDF malware detection task.
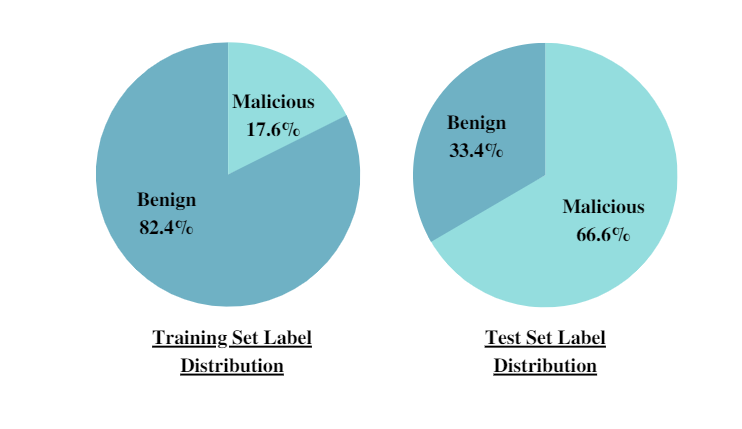
The distribution shifts from the training set to the One-month test set. A time-based split of the dataset into training and testing is applied in order to simulate the real-life deployment scenario and avoid temporal snooping. This strategy also allowed SophosAI to evaluate the model’s true generalization capabilities when faced with previously unseen brand-new PDF attacks, for example.
Experiments and results
To kickstart experiments, the SophosAI team trained a baseline XGBoost model with default parameters. Then they started performing hyperparameter fine-tuning with SageMaker using the Bayesian strategy, which is as simple as specifying the hyperparameters to be tuned and the desired range of values, the evaluation metric (ROC (Receiver Operating Characteristic) AUC in this case) and the training and validation sets. For the PDF malware detector, SophosAI prioritized hyperparameters including the number of boosting rounds (num_round
), the maximum tree depth (max_depth
), the learning rate (eta
), and the columns sampling ratio when building trees (colsample_bytree
). Eventually, the best hyperparameters were obtained and used to train a model on the full dataset, and finally evaluated on the holdout test set.
The following plot shows the objective metric (ROC AUC) vs. the 15 training jobs run within the tuning job. The best hyperparameters are those corresponding to the ninth training job.
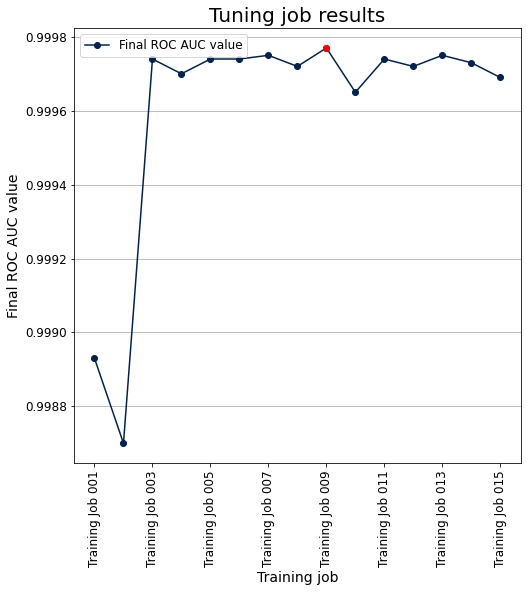
At the beginning of SophosAI’s experiments on SageMaker, an especially important question to answer was: what type of instances and how many of them are needed to train XGBoost on the data on hand? This is crucial because using the wrong number or type of instance can be a waste of time and money; the training is bound to fail due to running out of memory, or, if using too many too-large instances, this can become unnecessarily expensive.
XGBoost is a memory-bound (as opposed to compute-bound) algorithm. So, a general-purpose compute instance (for example, M5) is a better choice than a compute-optimized instance (for example, C4). To make an informed decision, there is a simple SageMaker guideline for picking the number of instances required to run training on the full dataset:
Total Training Data Size × Safety Factor(*) < Instance Count × Instance Type’s Total Memory
In this case: Total Training Data Size × Safety Factor (12) = 12120 GB
The following table summarizes the requirements when the chosen instance type is ml.m5.24xlarge.
Training Size × Safety Factor (12) |
Instance Memory ml.m5.24xlarge |
Minimum Instance Count Required for Training |
12120 GB |
384 GB |
32 |
*Due to the nature of XGBoost distributed training, which requires the entire training dataset to be loaded into a DMatrix object before training and additional free memory, a safety factor of 10–12 is recommended.
To take a closer look at the memory utilization for a full SageMaker training of XGBoost on the provided dataset, we provide the corresponding graph obtained from the training’s Amazon CloudWatch monitoring. For this training job, 40 ml.m5.24xlarge instances were used and maximum memory utilization reached around 62 %.
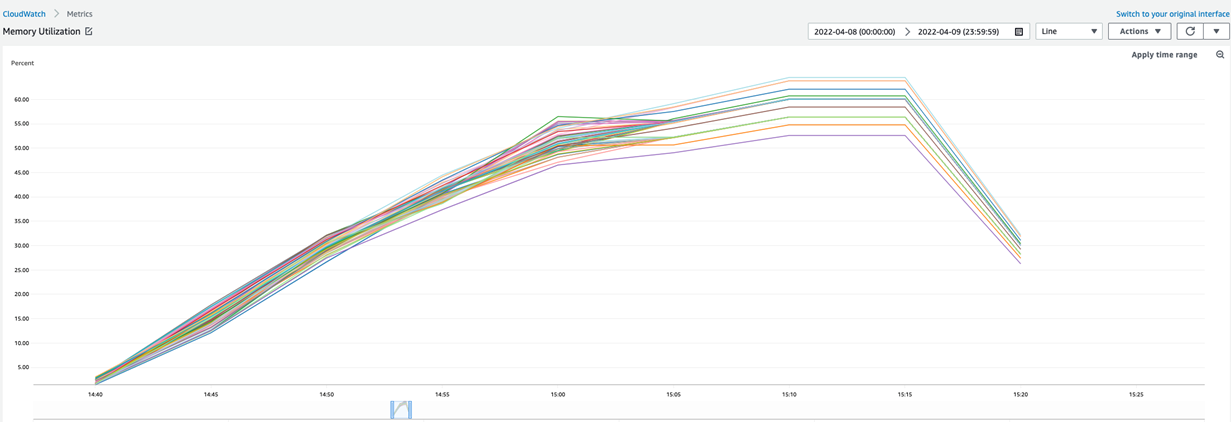
The engineering cost saved by integrating a managed ML service like SageMaker into the data pipeline is around 50%. The option to use Spot Instances for training and hyperparameter tuning jobs cut costs by an additional 63%.
Conclusion
With SageMaker, the SophosAI team could successfully resolve a complex high-priority project by building a lightweight PDF malware detection XGBoost model that is much smaller on disk (up to 25 times smaller) and in-memory (up to 5 times smaller) than its detector predecessor. It’s a small but mighty malware detector with ~0.99 AUC and a true positive rate of 0.99 and a false positive rate of
. This model can be quickly retrained, and its performance can be easily monitored over time, because it takes less than 20 minutes to train it on more than 1 TB of data.
You can leverage SageMaker built-in algorithm XGBoost for building models with your tabular data at scale. Additionally, you can also try the new built-in Amazon SageMaker algorithms LightGBM, CatBoost, AutoGluon-Tabular and Tab Transformer as described in this blog.
About the authors
Salma Taoufiq is a Senior Data Scientist at Sophos, working at the intersection of machine learning and cybersecurity. With an undergraduate background in computer science, she graduated from the Central European University with a MSc. in Mathematics and Its Applications. When not developing a malware detector, Salma is an avid hiker, traveler, and consumer of thrillers.
Harini Kannan is a Data Scientist at SophosAI. She has been in security data science for ~4 years. She was previously the Principal Data Scientist at Capsule8, which got acquired by Sophos. She has given talks at CAMLIS, BlackHat (USA), Open Data Science Conference (East), Data Science Salon, PyData (Boston), and Data Connectors. Her areas of research include detecting hardware-based attacks using performance counters, user behavior analysis, interpretable ML, and unsupervised anomaly detection.
Hasan Poonawala is a Senior AI/ML Specialist Solutions Architect at AWS, based in London, UK. Hasan helps customers design and deploy machine learning applications in production on AWS. He has over 12 years of work experience as a data scientist, machine learning practitioner and software developer. In his spare time, Hasan loves to explore nature and spend time with friends and family.
Digant Patel is an Enterprise Support Lead at AWS. He works with customers to design, deploy and operate in cloud at scale. His areas of interest are MLOps and DevOps practices and how it can help customers in their cloud journey. Outside of work, he enjoys photography, playing volleyball and spending time with friends and family.
Read More