Today we are excited to announce that Stable Diffusion XL 1.0 (SDXL 1.0) is available for customers through Amazon SageMaker JumpStart. SDXL 1.0 is the latest image generation model from Stability AI. SDXL 1.0 enhancements include native 1024-pixel image generation at a variety of aspect ratios. It’s designed for professional use, and calibrated for high-resolution photorealistic images. SDXL 1.0 offers a variety of preset art styles ready to use in marketing, design, and image generation use cases across industries. You can easily try out these models and use them with SageMaker JumpStart, a machine learning (ML) hub that provides access to algorithms, models, and ML solutions so you can quickly get started with ML.
In this post, we walk through how to use SDXL 1.0 models via SageMaker JumpStart.
What is Stable Diffusion XL 1.0 (SDXL 1.0)
SDXL 1.0 is the evolution of Stable Diffusion and the next frontier for generative AI for images. SDXL is capable of generating stunning images with complex concepts in various art styles, including photorealism, at quality levels that exceed the best image models available today. Like the original Stable Diffusion series, SDXL is highly customizable (in terms of parameters) and can be deployed on Amazon SageMaker instances.
The following image of a lion was generated using SDXL 1.0 using a simple prompt, which we explore later in this post.
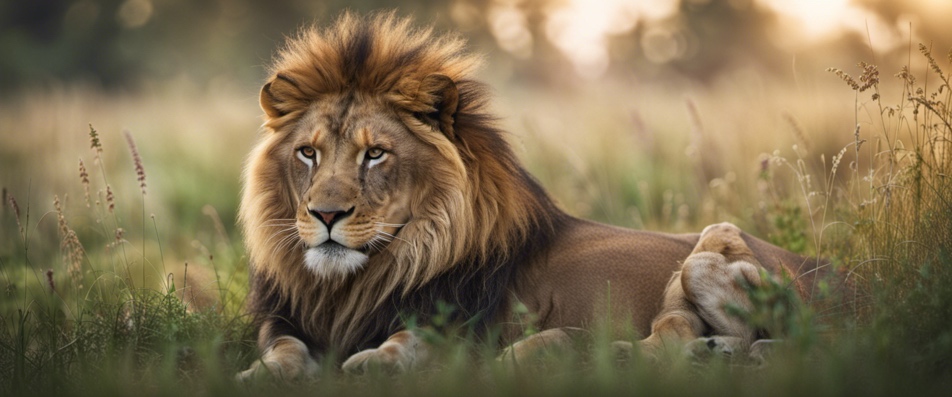
The SDXL 1.0 model includes the following highlights:
- Freedom of expression – Best-in-class photorealism, as well as an ability to generate high-quality art in virtually any art style. Distinct images are made without having any particular feel that is imparted by the model, ensuring absolute freedom of style.
- Artistic intelligence – Best-in-class ability to generate concepts that are notoriously difficult for image models to render, such as hands and text, or spatially arranged objects and people (for example, a red box on top of a blue box).
- Simpler prompting – Unlike other generative image models, SDXL requires only a few words to create complex, detailed, and aesthetically pleasing images. No more need for paragraphs of qualifiers.
- More accurate – Prompting in SDXL is not only simple, but more true to the intention of prompts. SDXL’s improved CLIP model understands text so effectively that concepts like “The Red Square” are understood to be different from “a red square.” This accuracy allows much more to be done to get the perfect image directly from text, even before using the more advanced features or fine-tuning that Stable Diffusion is famous for.
What is SageMaker JumpStart
With SageMaker JumpStart, ML practitioners can choose from a broad selection of state-of-the-art models for use cases such as content writing, image generation, code generation, question answering, copywriting, summarization, classification, information retrieval, and more. ML practitioners can deploy foundation models to dedicated SageMaker instances from a network isolated environment and customize models using SageMaker for model training and deployment. The SDXL model is discoverable today in Amazon SageMaker Studio and, as of this writing, is available in us-east-1
, us-east-2
, us-west-2
, eu-west-1
, ap-northeast-1
, and ap-southeast-2
Regions.
Solution overview
In this post, we demonstrate how to deploy SDXL 1.0 to SageMaker and use it to generate images using both text-to-image and image-to-image prompts.
SageMaker Studio is a web-based integrated development environment (IDE) for ML that lets you build, train, debug, deploy, and monitor your ML models. For more details on how to get started and set up SageMaker Studio, refer to Amazon SageMaker Studio.
Once you are in the SageMaker Studio UI, access SageMaker JumpStart and search for Stable Diffusion XL
. Choose the SDXL 1.0 model card, which will open up an example notebook. This means you will be only be responsible for compute costs. There is no associated model cost. Closed weight SDXL 1.0 offers SageMaker optimized scripts and container with faster inference time and can be run on smaller instance compared to the open weight SDXL 1.0. The example notebook will walk you through steps, but we also discuss how to discover and deploy the model later in this post.
In the following sections, we show how you can use SDXL 1.0 to create photorealistic images with shorter prompts and generate text within images. Stable Diffusion XL 1.0 offers enhanced image composition and face generation with stunning visuals and realistic aesthetics.
Stable Diffusion XL 1.0 parameters
The following are the parameters used by SXDL 1.0:
- cfg_scale – How strictly the diffusion process adheres to the prompt text.
- height and width – The height and width of image in pixel.
- steps – The number of diffusion steps to run.
- seed – Random noise seed. If a seed is provided, the resulting generated image will be deterministic.
- sampler – Which sampler to use for the diffusion process to denoise our generation with.
- text_prompts – An array of text prompts to use for generation.
- weight – Provides each prompt a specific weight
For more information, refer to the Stability AI’s text to image documentation.
The following code is a sample of the input data provided with the prompt:
{
"cfg_scale": 7,
"height": 1024,
"width": 1024,
"steps": 50,
"seed": 42,
"sampler": "K_DPMPP_2M",
"text_prompts": [
{
"text": "A photograph of fresh pizza with basil and tomatoes, from a traditional oven",
"weight": 1
}
]
}
All examples in this post are based on the sample notebook for Stability Diffusion XL 1.0, which can be found on Stability AI’s GitHub repo.
Generate images using SDXL 1.0
In the following examples, we focus on the capabilities of Stability Diffusion XL 1.0 models, including superior photorealism, enhanced image composition, and the ability to generate realistic faces. We also explore the significantly improved visual aesthetics, resulting in visually appealing outputs. Additionally, we demonstrate the use of shorter prompts, enabling the creation of descriptive imagery with greater ease. Lastly, we illustrate how the text in images is now more legible, further enriching the overall quality of the generated content.
The following example shows using a simple prompt to get detailed images. Using only a few words in the prompt, it was able to create a complex, detailed, and aesthetically pleasing image that resembles the provided prompt.
text = "photograph of latte art of a cat"
output = deployed_model.predict(GenerationRequest(text_prompts=[TextPrompt(text=text)],
seed=5,
height=640,
width=1536,
sampler="DDIM",
))
decode_and_show(output)
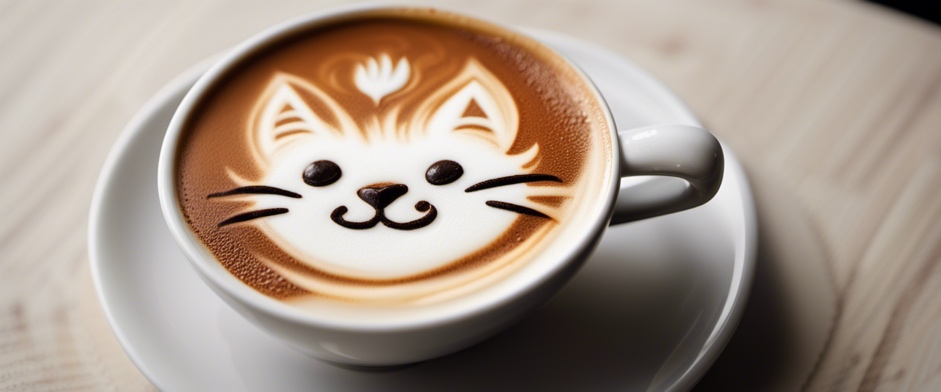
Next, we show the use of the style_preset
input parameter, which is only available on SDXL 1.0. Passing in a style_preset
parameter guides the image generation model towards a particular style.
Some of the available style_preset
parameters are enhance
, anime
, photographic
, digital-art
, comic-book
, fantasy-art
, line-art
, analog-film
, neon-punk
, isometric
, low-poly
, origami
, modeling-compound
, cinematic
, 3d-mode
, pixel-art
, and tile-texture
. This list of style presets is subject to change; refer to the latest release and documentation for updates.
For this example, we use a prompt to generate a teapot with a style_preset
of origami
. The model was able to generate a high-quality image in the provided art style.
output = deployed_model.predict(GenerationRequest(text_prompts=[TextPrompt(text="teapot")],
style_preset="origami",
seed = 3,
height = 1024,
width = 1024
))
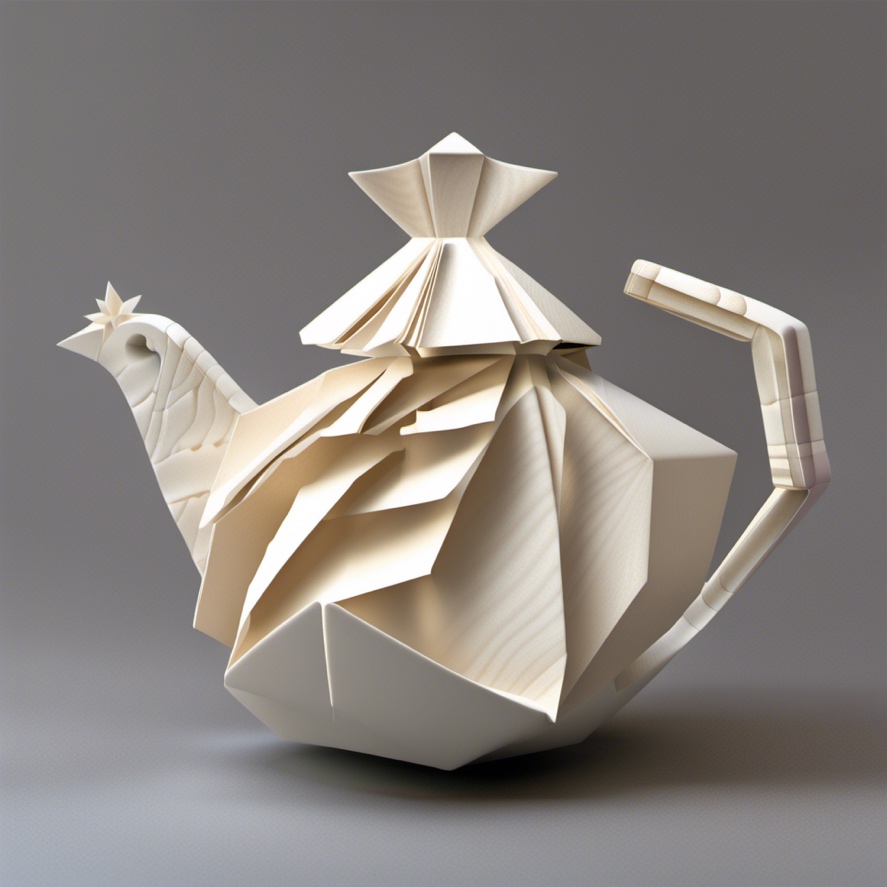
Let’s try some more style presets with different prompts. The next example shows a style preset for portrait generation using style_preset="photographic"
with the prompt “portrait of an old and tired lion real pose.”
text = "portrait of an old and tired lion real pose"
output = deployed_model.predict(GenerationRequest(text_prompts=[TextPrompt(text=text)],
style_preset="photographic",
seed=111,
height=640,
width=1536,
))
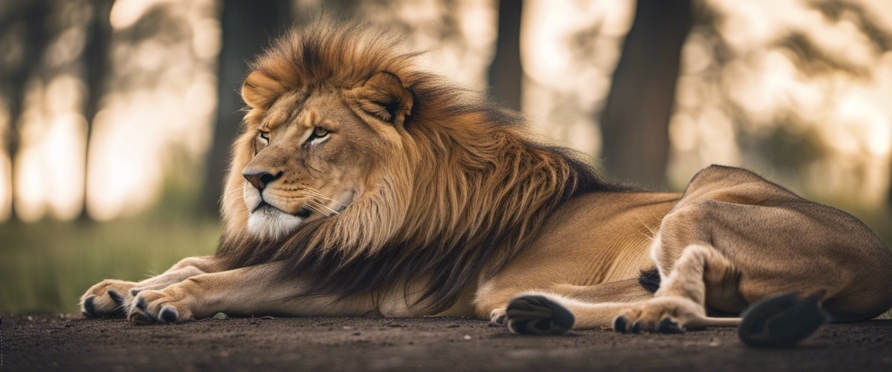
Now let’s try the same prompt (“portrait of an old and tired lion real pose”) with modeling-compound as the style preset. The output image is a distinct image made without having any particular feel that is imparted by the model, ensuring absolute freedom of style.
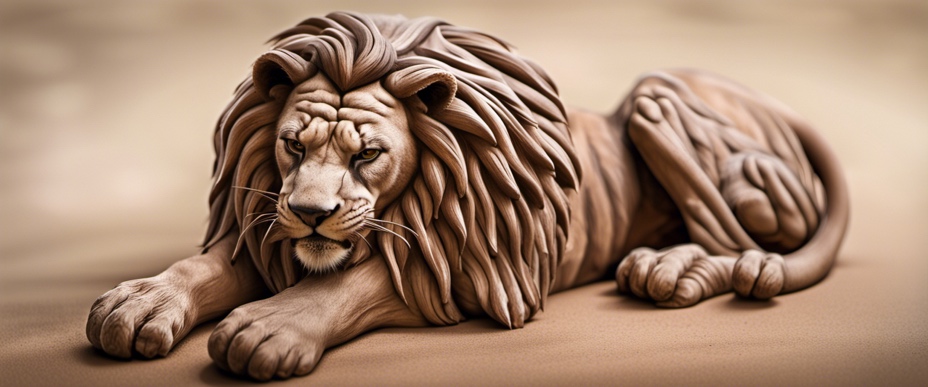
Multi-prompting with SDXL 1.0
As we have seen, one of the core foundations of the model is the ability to generate images via prompting. SDXL 1.0 supports multi-prompting. With multi-prompting, you can mix concepts together by assigning each prompt a specific weight. As you can see in the following generated image, it has a jungle background with tall bright green grass. This image was generated using the following prompts. You can compare this to a single prompt from our earlier example.
text1 = "portrait of an old and tired lion real pose"
text2 = "jungle with tall bright green grass"
output = deployed_model.predict(GenerationRequest(
text_prompts=[TextPrompt(text=text1),
TextPrompt(text=text2, weight=0.7)],
style_preset="photographic",
seed=111,
height=640,
width=1536,
))
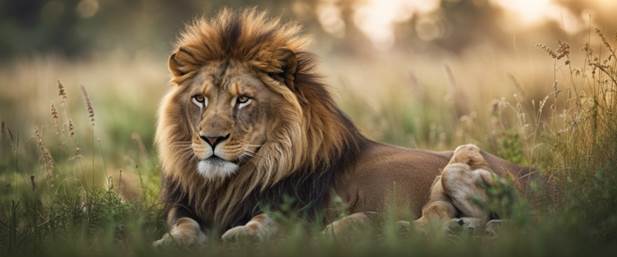
Spatially aware generated images and negative prompts
Next, we look at poster design with a detailed prompt. As we saw earlier, multi-prompting allows you to combine concepts to create new and unique results.
In this example, the prompt is very detailed in terms of subject position, appearance, expectations, and surroundings. The model is also trying to avoid images that have distortion or are poorly rendered with the help of a negative prompt. The image generated shows spatially arranged objects and subjects.
text = “A cute fluffy white cat stands on its hind legs, peering curiously into an ornate golden mirror. But in the reflection, the cat sees not itself, but a mighty lion. The mirror illuminated with a soft glow against a pure white background.”
text = "A cute fluffy white cat stands on its hind legs, peering curiously into an ornate golden mirror. But in the reflection, the cat sees not itself, but a mighty lion. The mirror illuminated with a soft glow against a pure white background."
negative_prompts = ['distorted cat features', 'distorted lion features', 'poorly rendered']
output = deployed_model.predict(GenerationRequest(
text_prompts=[TextPrompt(text=text)],
style_preset="enhance",
seed=43,
height=640,
width=1536,
steps=100,
cfg_scale=7,
negative_prompts=negative_prompts
))
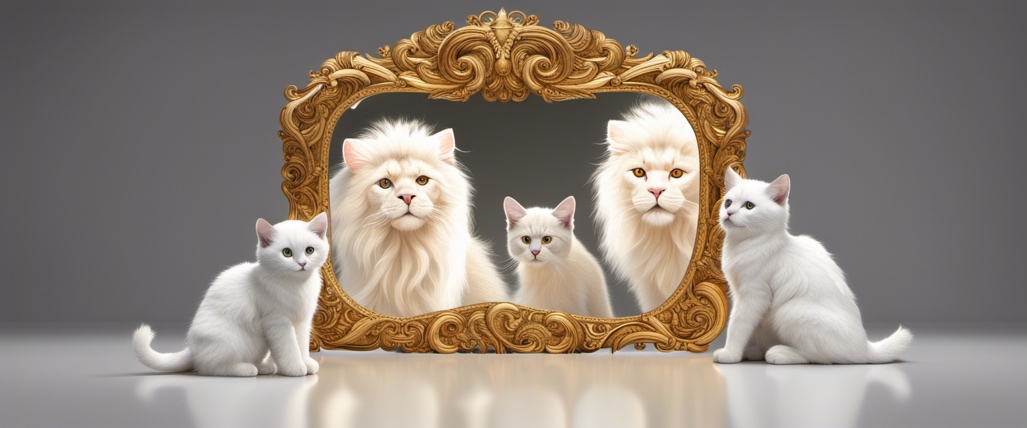
Let’s try another example, where we keep the same negative prompt but change the detailed prompt and style preset. As you can see, the generated image not only spatially arranges objects, but also changes the style presets with attention to details like the ornate golden mirror and reflection of the subject only.
text = "A cute fluffy white cat stands on its hind legs, peering curiously into an ornate golden mirror. In the reflection the cat sees itself."
negative_prompts = ['distorted cat features', 'distorted lion features', 'poorly rendered']
output = deployed_model.predict(GenerationRequest(
text_prompts=[TextPrompt(text=text)],
style_preset="neon-punk",
seed=4343434,
height=640,
width=1536,
steps=150,
cfg_scale=7,
negative_prompts=negative_prompts
))
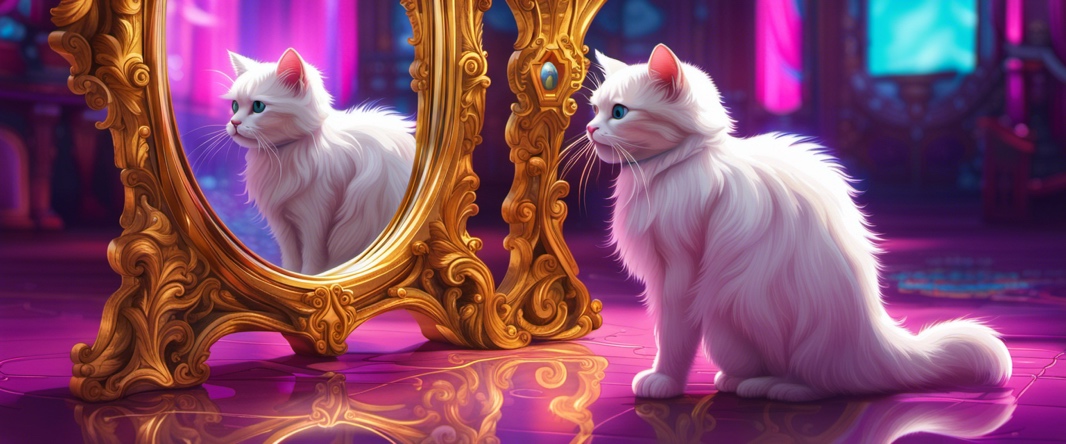
Face generation with SDXL 1.0
In this example, we show how SDXL 1.0 creates enhanced image composition and face generation with realistic features such as hands and fingers. The generated image is of a human figure created by AI with clearly raised hands. Note the details in the fingers and the pose. An AI-generated image such as this would otherwise have been amorphous.
text = "Photo of an old man with hands raised, real pose."
output = deployed_model.predict(GenerationRequest(
text_prompts=[TextPrompt(text=text)],
style_preset="photographic",
seed=11111,
height=640,
width=1536,
steps=100,
cfg_scale=7,
))
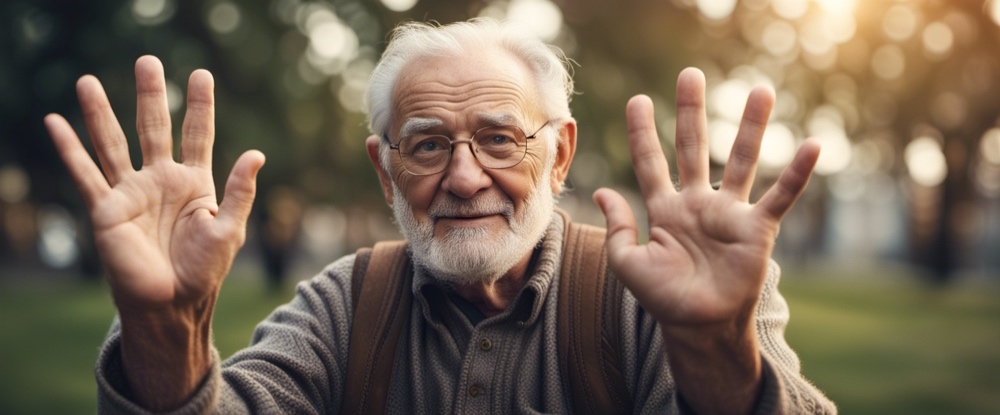
Text generation using SDXL 1.0
SDXL is primed for complex image design workflows that include generation of text within images. This example prompt showcases this capability. Observe how clear the text generation is using SDXL and notice the style preset of cinematic.
text = "Write the following word: Dream"
output = deployed_model.predict(GenerationRequest(text_prompts=[TextPrompt(text=text)],
style_preset="cinematic",
seed=15,
height=640,
width=1536,
sampler="DDIM",
steps=32,
))
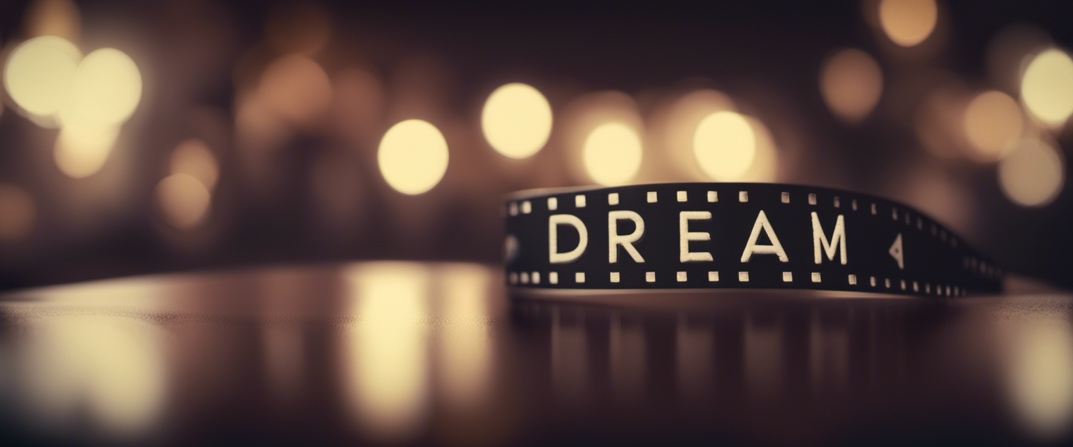
Discover SDXL 1.0 from SageMaker JumpStart
SageMaker JumpStart onboards and maintains foundation models for you to access, customize, and integrate into your ML lifecycles. Some models are open weight models that allow you to access and modify model weights and scripts, whereas some are closed weight models that don’t allow you to access them to protect the IP of model providers. Closed weight models require you to subscribe to the model from the AWS Marketplace model detail page, and SDXL 1.0 is a model with closed weight at this time. In this section, we go over how to discover, subscribe, and deploy a closed weight model from SageMaker Studio.
You can access SageMaker JumpStart by choosing JumpStart under Prebuilt and automated solutions on the SageMaker Studio Home page.
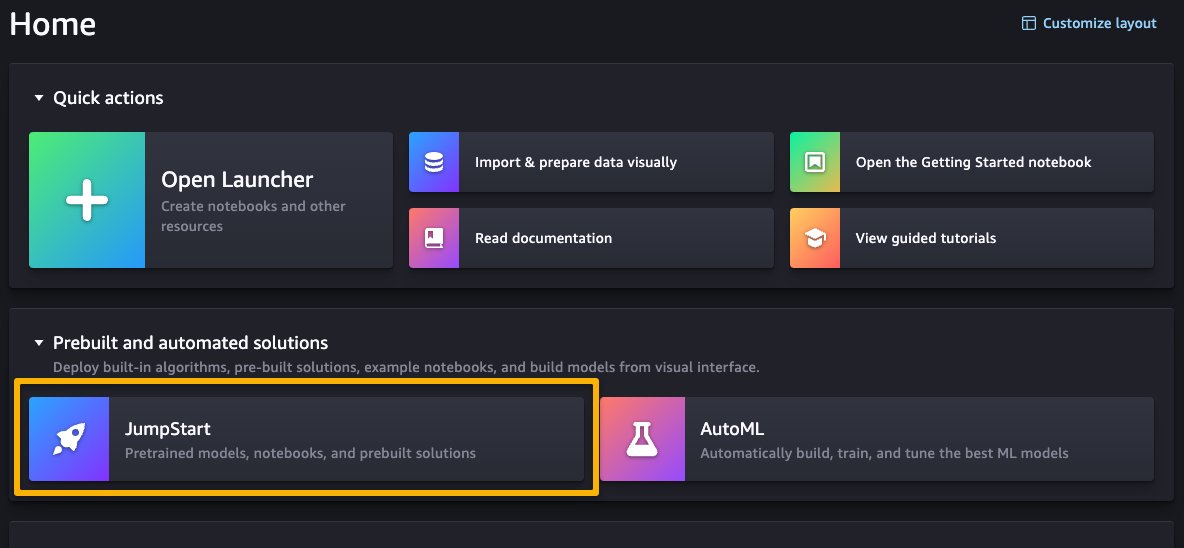
From the SageMaker JumpStart landing page, you can browse for solutions, models, notebooks, and other resources. The following screenshot shows an example of the landing page with solutions and foundation models listed.
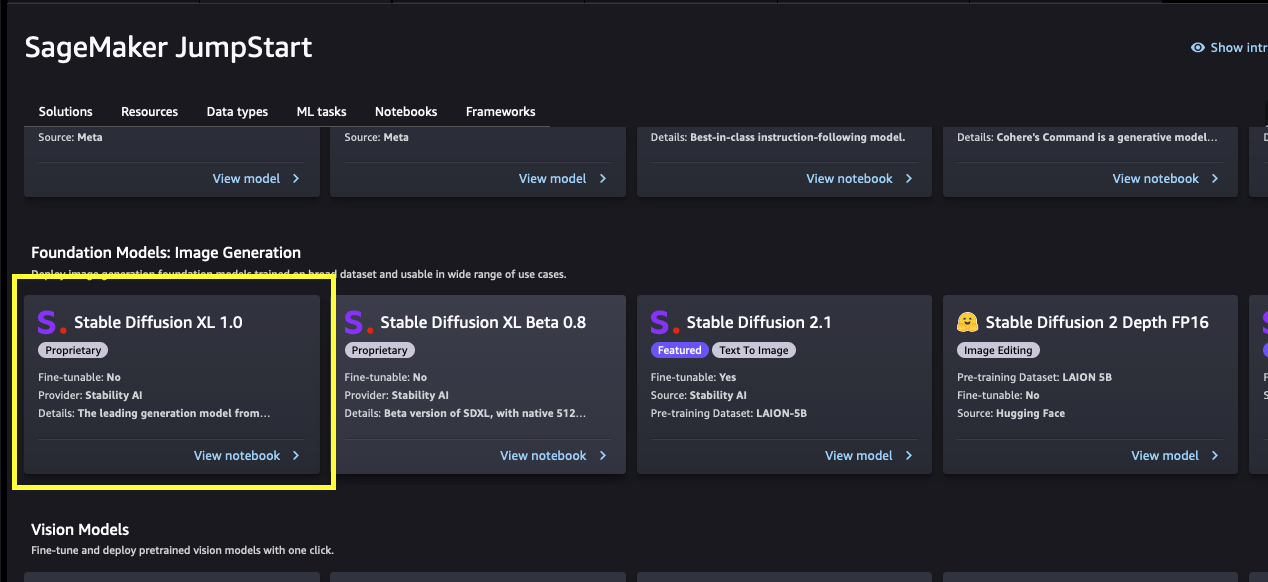
Each model has a model card, as shown in the following screenshot, which contains the model name, if it is fine-tunable or not, the provider name, and a short description about the model. You can find the Stable Diffusion XL 1.0 model in the Foundation Model: Image Generation carousel or search for it in the search box.
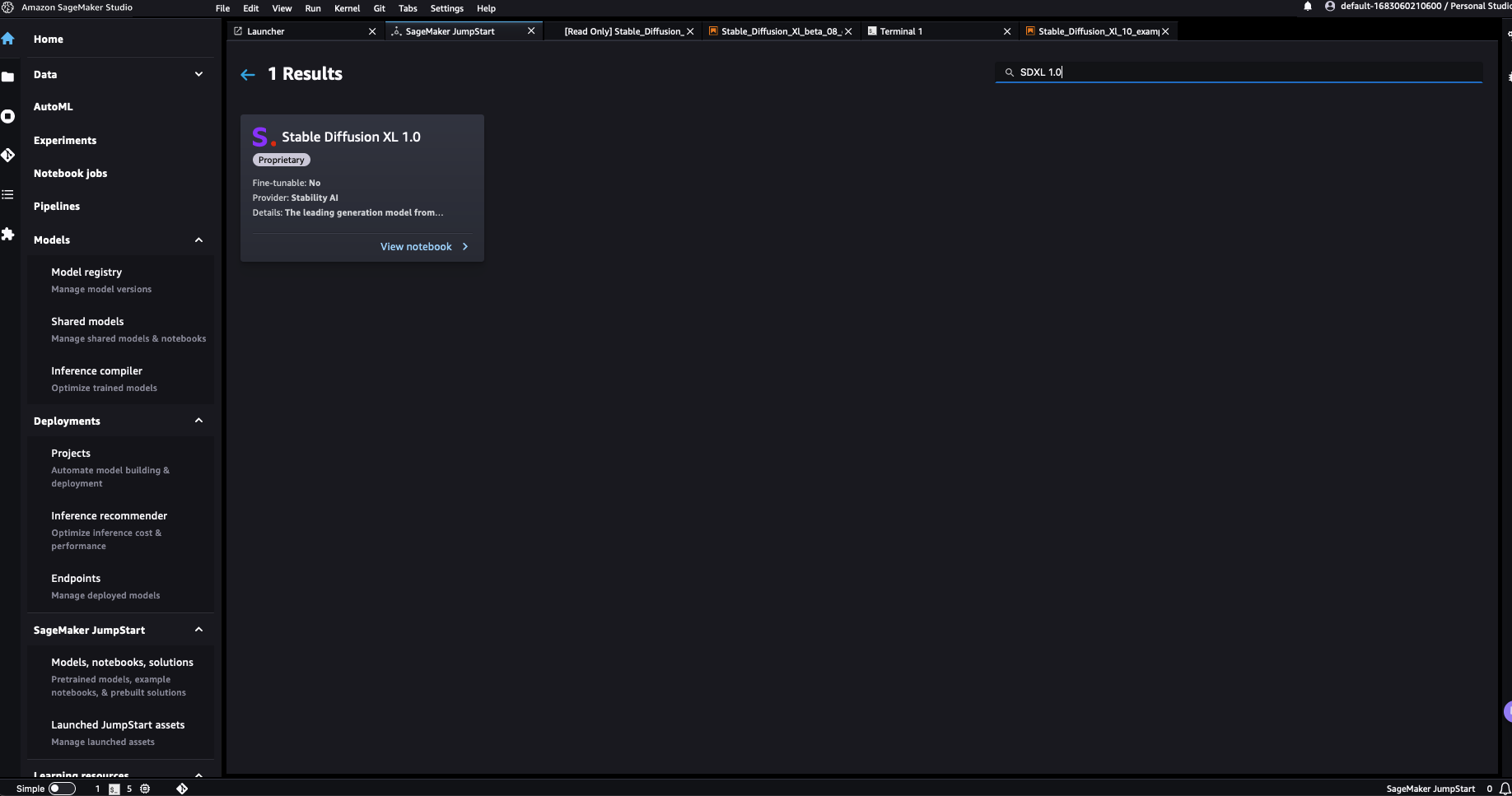
You can choose Stable Diffusion XL 1.0 to open an example notebook that walks you through how to use the SDXL 1.0 model. The example notebook opens as read-only mode; you need to choose Import notebook to run it.
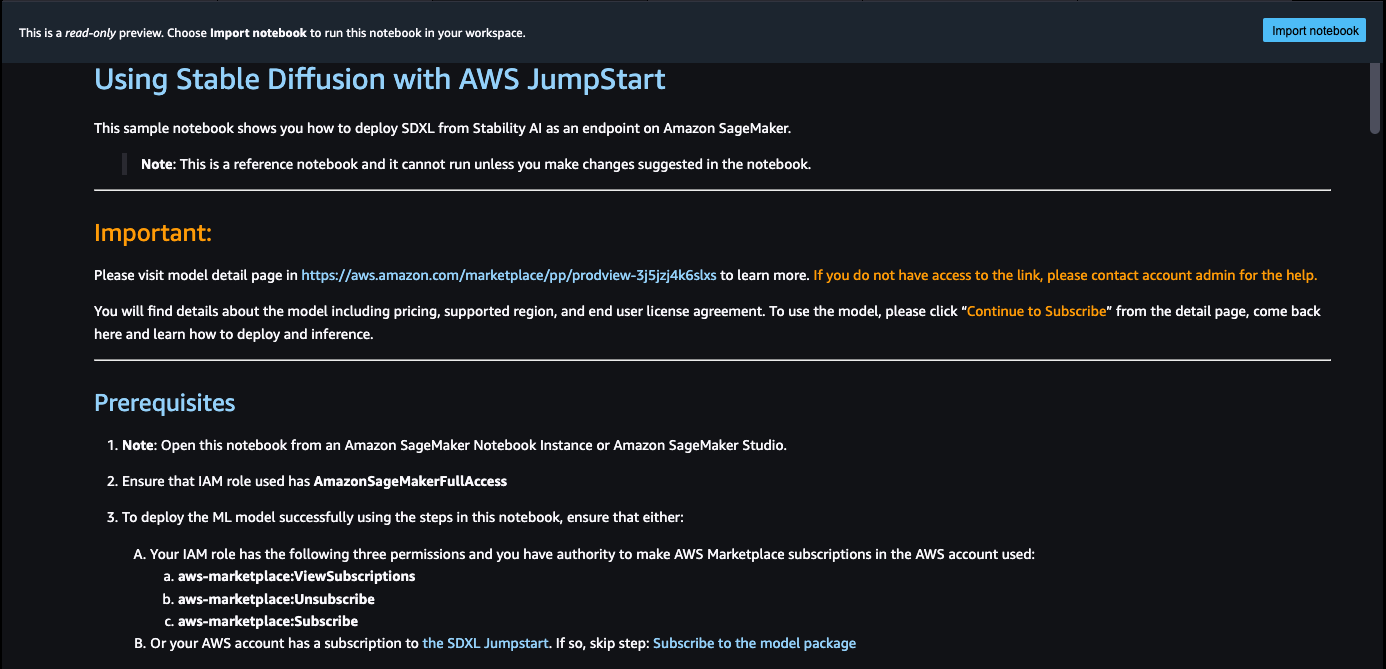
After importing the notebook, you need to select the appropriate notebook environment (image, kernel, instance type, and so on) before running the code.
Deploy SDXL 1.0 from SageMaker JumpStart
In this section, we walk through how to subscribe and deploy the model.
- Open the model listing page in AWS Marketplace using the link available from the example notebook in SageMaker JumpStart.
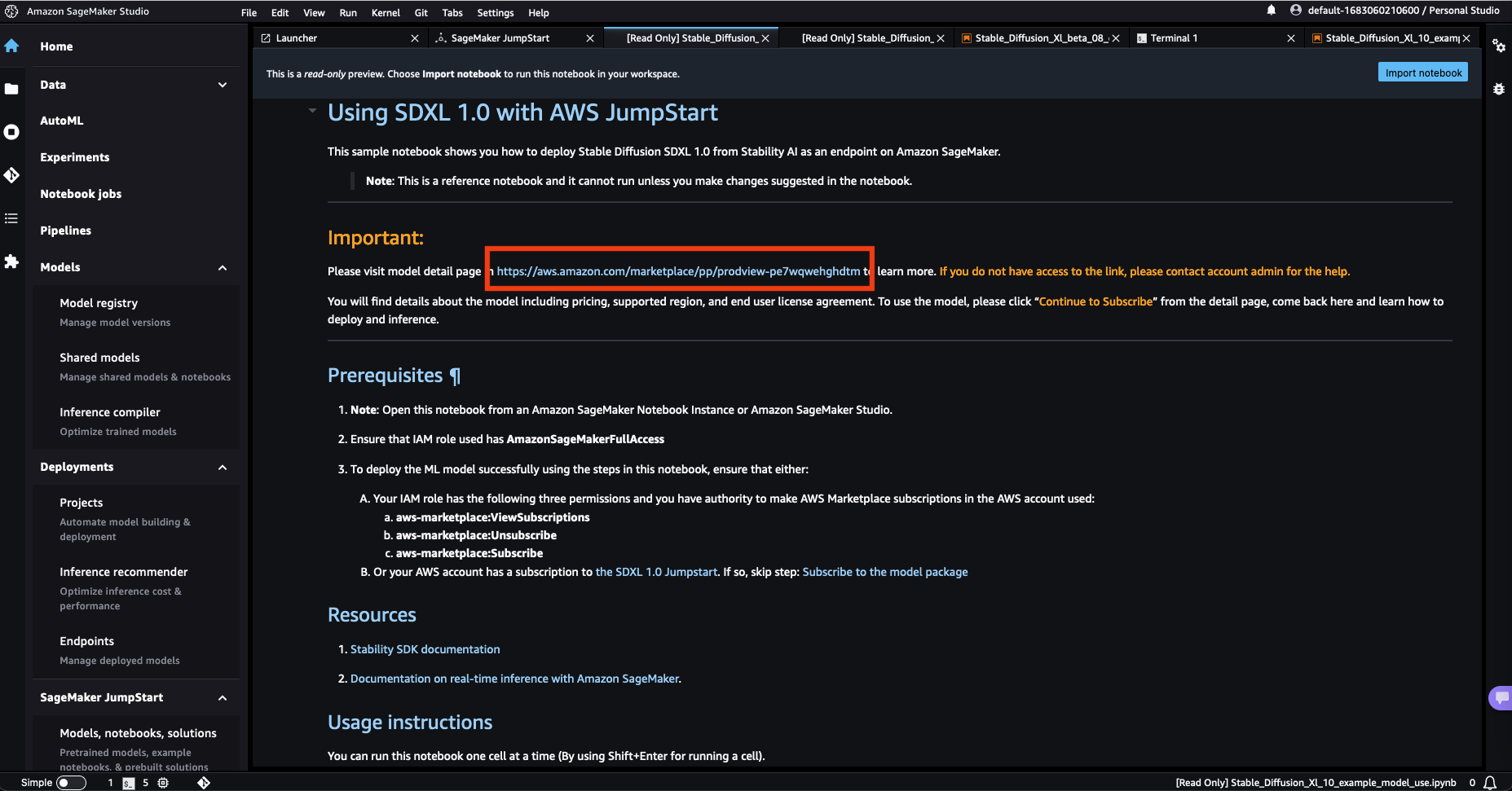
- On the AWS Marketplace listing, choose Continue to subscribe.
If you don’t have the necessary permissions to view or subscribe to the model, reach out to your AWS administrator or procurement point of contact. Many enterprises may limit AWS Marketplace permissions to control the actions that someone can take in the AWS Marketplace Management Portal.
- Choose Continue to Subscribe.
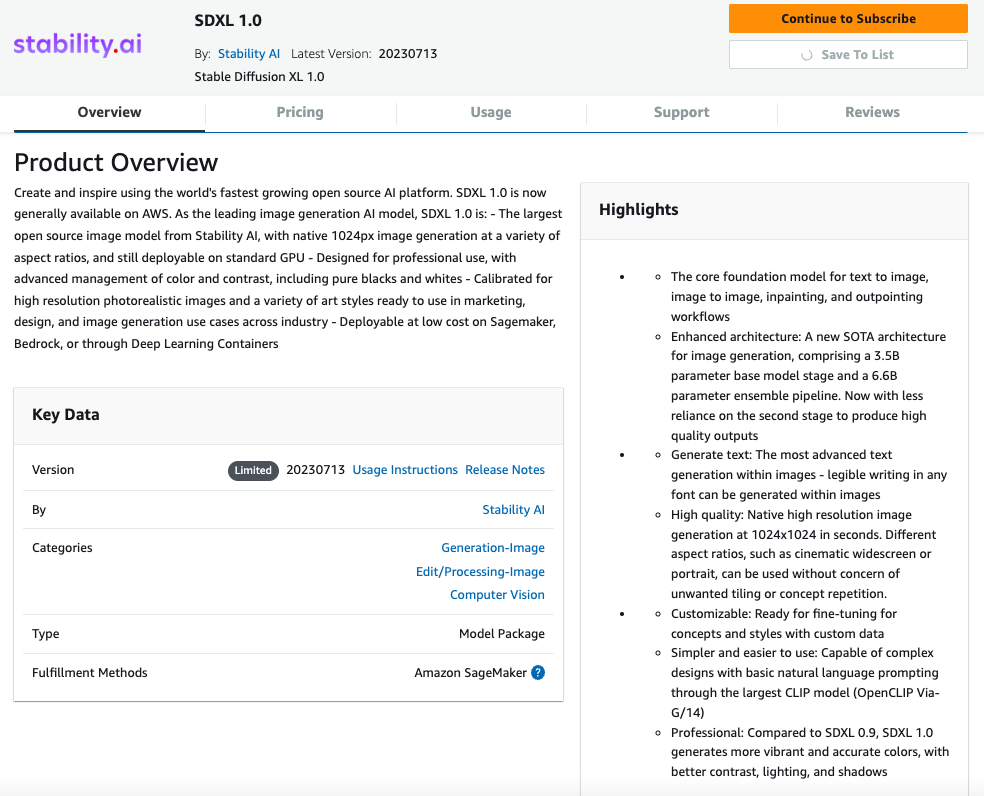
- On the Subscribe to this software page, review the pricing details and End User Licensing Agreement (EULA). If agreeable, choose Accept offer.
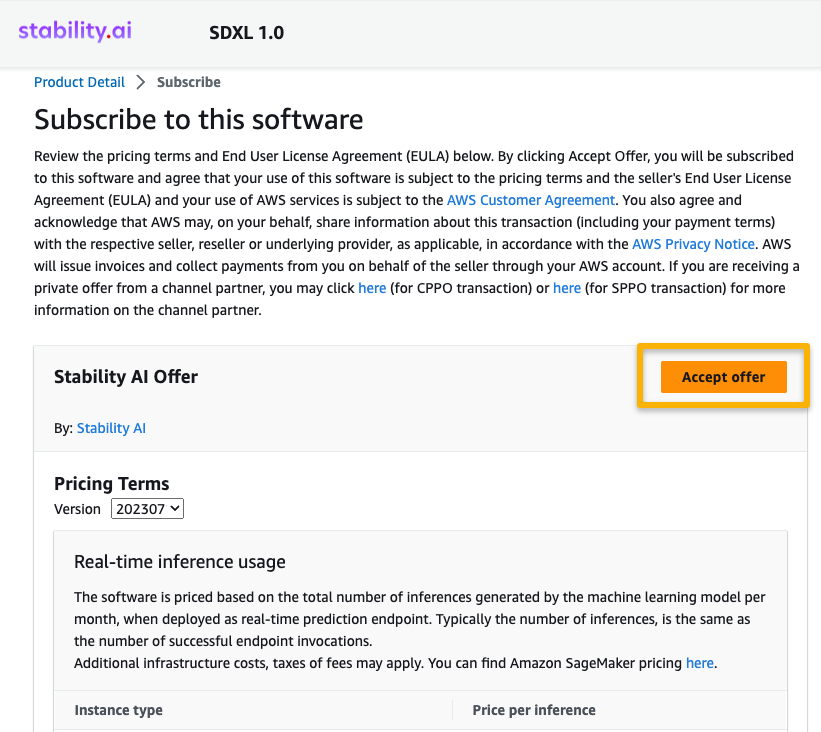
- Choose Continue to configuration to start configuring your model.
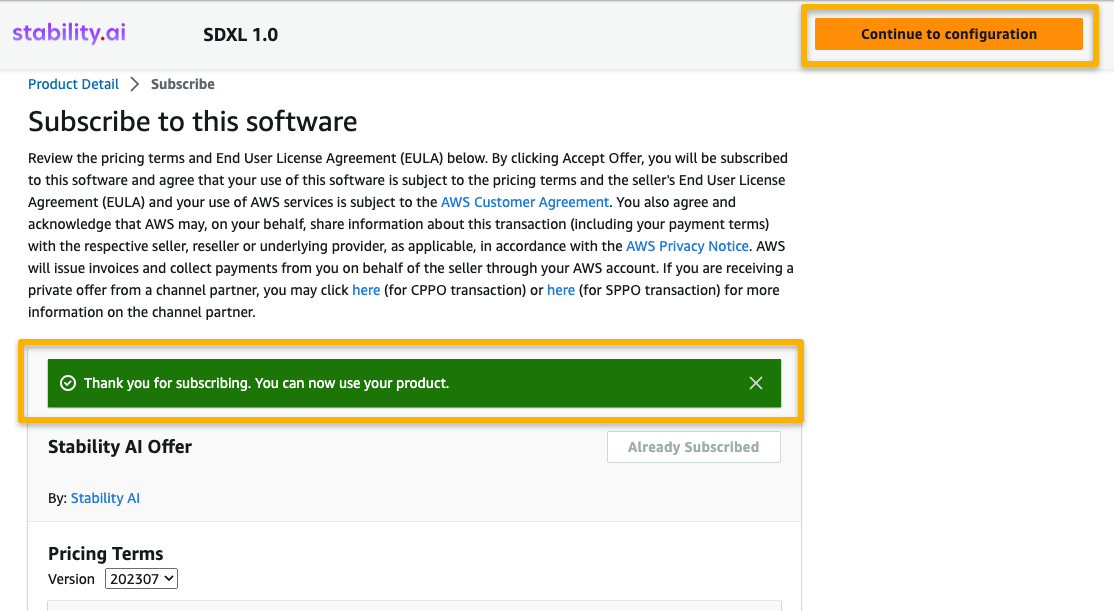
- Choose a supported Region.
You will see a product ARN displayed. This is the model package ARN that you need to specify while creating a deployable model using Boto3.
- Copy the ARN corresponding to your Region and specify the same in the notebook’s cell instruction.
ARN information may be already available in the example notebook.
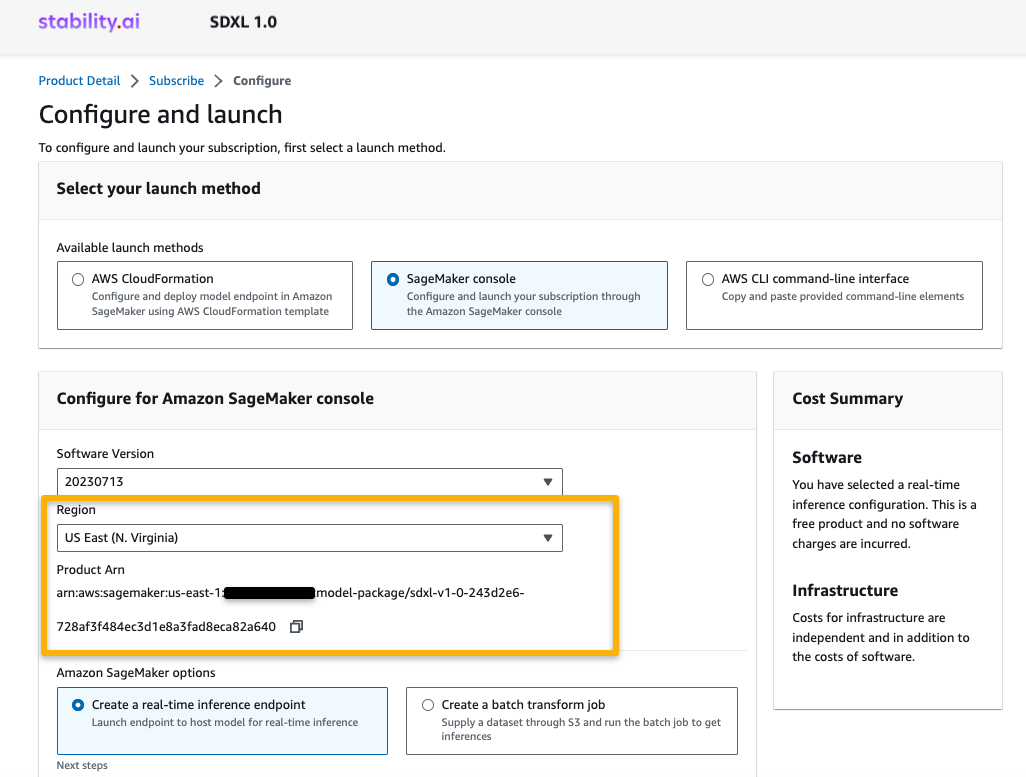
- Now you’re ready to start following the example notebook.
You can also continue from AWS Marketplace, but we recommend following the example notebook in SageMaker Studio to better understand how deployment works.
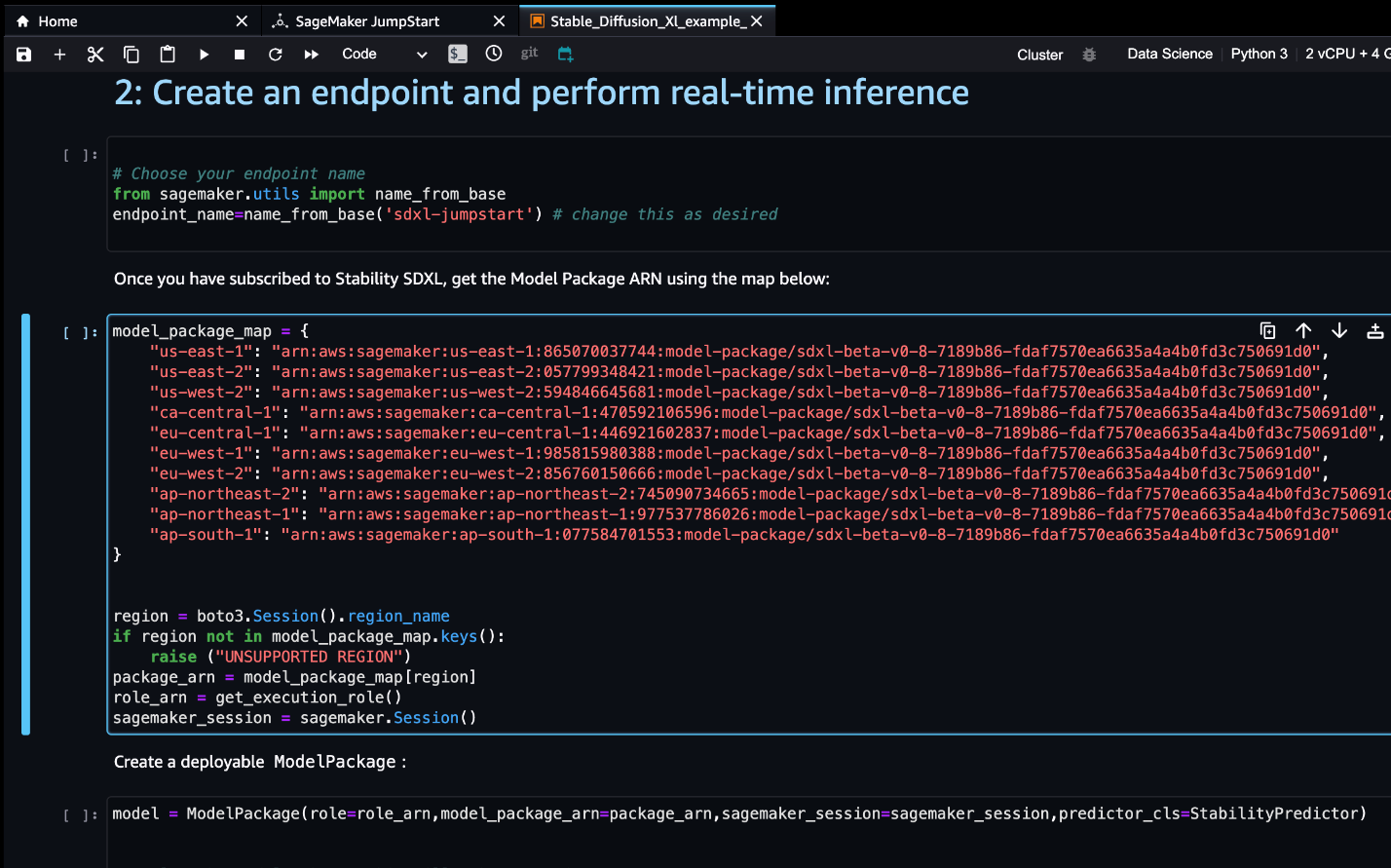
Clean up
When you’ve finished working, you can delete the endpoint to release the Amazon Elastic Compute Cloud (Amazon EC2) instances associated with it and stop billing.
Get your list of SageMaker endpoints using the AWS CLI as follows:
!aws sagemaker list-endpoints
Then delete the endpoints:
deployed_model.sagemaker_session.delete_endpoint(endpoint_name)
Conclusion
In this post, we showed you how to get started with the new SDXL 1.0 model in SageMaker Studio. With this model, you can take advantage of the different features offered by SDXL to create realistic images. Because foundation models are pre-trained, they can also help lower training and infrastructure costs and enable customization for your use case.
Resources
About the authors
June Won is a product manager with SageMaker JumpStart. He focuses on making foundation models easily discoverable and usable to help customers build generative AI applications.
Mani Khanuja is an Artificial Intelligence and Machine Learning Specialist SA at Amazon Web Services (AWS). She helps customers using machine learning to solve their business challenges using the AWS. She spends most of her time diving deep and teaching customers on AI/ML projects related to computer vision, natural language processing, forecasting, ML at the edge, and more. She is passionate about ML at edge, therefore, she has created her own lab with self-driving kit and prototype manufacturing production line, where she spends lot of her free time.
Nitin Eusebius is a Sr. Enterprise Solutions Architect at AWS with experience in Software Engineering , Enterprise Architecture and AI/ML. He works with customers on helping them build well-architected applications on the AWS platform. He is passionate about solving technology challenges and helping customers with their cloud journey.
Suleman Patel is a Senior Solutions Architect at Amazon Web Services (AWS), with a special focus on Machine Learning and Modernization. Leveraging his expertise in both business and technology, Suleman helps customers design and build solutions that tackle real-world business problems. When he’s not immersed in his work, Suleman loves exploring the outdoors, taking road trips, and cooking up delicious dishes in the kitchen.
Dr. Vivek Madan is an Applied Scientist with the Amazon SageMaker JumpStart team. He got his PhD from University of Illinois at Urbana-Champaign and was a Post Doctoral Researcher at Georgia Tech. He is an active researcher in machine learning and algorithm design and has published papers in EMNLP, ICLR, COLT, FOCS, and SODA conferences.
Read More