Amazon-Illinois Center on Artificial Intelligence for Interactive Conversational Experiences also names first cohort of academic fellows.Read More
How AI-Based Cybersecurity Strengthens Business Resilience
The world’s 5 billion internet users and nearly 54 billion devices generate 3.4 petabytes of data per second, according to IDC. As digitalization accelerates, enterprise IT teams are under greater pressure to identify and block incoming cyber threats to ensure business operations and services are not interrupted — and AI-based cybersecurity provides a reliable way to do so.
Few industries appear immune to cyber threats. This year alone, international hotel chains, financial institutions, Fortune 100 retailers, air traffic-control systems and the U.S. government have all reported threats and intrusions.
Whether from insider error, cybercriminals, hacktivists or other threats, risks in the cyber landscape can damage an enterprise’s reputation and bottom line. A breach can paralyze operations, jeopardize proprietary and customer data, result in regulatory fines and destroy customer trust.
Using AI and accelerated computing, businesses can reduce the time and operational expenses required to detect and block cyber threats while freeing up resources to focus on core business value operations and revenue-generating activities.
Here’s a look at how industries are applying AI techniques to safeguard data, enable faster threat detection and mitigate attacks to ensure the consistent delivery of service to customers and partners.
Public Sector: Protecting Physical Security, Energy Security and Citizen Services
AI-powered analytics and automation tools are helping government agencies provide citizens with instant access to information and services, make data-driven decisions, model climate change, manage natural disasters, and more. But public entities managing digital tools and infrastructure face a complex cyber risk environment that includes regulatory compliance requirements, public scrutiny, large interconnected networks and the need to protect sensitive data and high-value targets.
Adversary nation-states may initiate cyberattacks to disrupt networks, steal intellectual property or swipe classified government documents. Internal misuse of digital tools and infrastructure combined with sophisticated external espionage places public organizations at high risk of data breach. Espionage actors have also been known to recruit inside help, with 16% of public administration breaches showing evidence of collusion. To protect critical infrastructure, citizen data, public records and other sensitive information, federal organizations are turning to AI.
The U.S. Department of Energy’s (DOE) Office of Cybersecurity, Energy Security and Emergency Response (CESER) is tasked with strengthening the resilience of the country’s energy sector by addressing emerging threats and improving energy infrastructure security. The DOE-CESER has invested more than $240 million in cybersecurity research, development and demonstration projects since 2010.
In one project, the department developed a tool that uses AI to automate and optimize security vulnerability and patch management in energy delivery systems. Another project for artificial diversity and defense security uses software-defined networks to enhance the situational awareness of energy delivery systems, helping ensure uninterrupted flows of energy.
The Defense Advanced Research Projects Agency (DARPA), which is charged with researching and investing in breakthrough technologies for national security, is using machine learning and AI in several areas. The DARPA CASTLE program trains AI to defend against advanced, persistent cyber threats. As part of the effort, researchers intend to accelerate cybersecurity assessments with approaches that are automated, repeatable and measurable. The DARPA GARD program builds platforms, libraries, datasets and training materials to help developers build AI models that are resistant to deception and adversarial attacks.
To keep up with an evolving threat landscape and ensure physical security, energy security and data security, public organizations must continue integrating AI to achieve a dynamic, proactive and far-reaching cyber defense posture.
Financial Services: Securing Digital Transactions, Payments and Portfolios
Banks, asset managers, insurers and other financial service organizations are using AI and machine learning to deliver superior performance in fraud detection, portfolio management, algorithmic trading and self-service banking.
With constant digital transactions, payments, loans and investment trades, financial service institutions manage some of the largest, most complex and most sensitive datasets of any industry. Behind only the healthcare industry, these organizations suffer the second highest cost of a data breach, at nearly $6 million per incident. This cost grows if regulators issue fines or if recovery includes legal fees and lawsuit settlements. Worse still, lost business may never be recovered if trust can’t be repaired.
Banks and financial institutions use AI to improve insider threat detection, detect phishing and ransomware, and keep sensitive information safe.
FinSec Innovation Lab, a joint venture by Mastercard and Enel X, is using AI to help its customers defend against ransomware. Prior to working with FinSec, one card-processing customer suffered a LockBit ransomware attack in which 200 company servers were infected in just 1.5 hours. The company was forced to shut down servers and suspend operations, resulting in an estimated $7 million in lost business.
FinSec replicated this attack in its lab but deployed the NVIDIA Morpheus cybersecurity framework, NVIDIA DOCA software framework for intrusion detection and NVIDIA BlueField DPU computing clusters. With this mix of AI and accelerated computing, FinSec was able to detect the ransomware attack in less than 12 seconds, quickly isolate virtual machines and recover 80% of the data on infected servers. This type of real-time response helps businesses avoid service downtime and lost business while maintaining customer trust.
With AI to help defend against cyberattacks, financial institutions can identify intrusions and anticipate future threats to keep financial records, accounts and transactions secure.
Retail: Keeping Sales Channels and Payment Credentials Safe
Retailers are using AI to power personalized product recommendations, dynamic pricing and customized marketing campaigns. Multichannel digital platforms have made in-store and online shopping more convenient: up to 48% of consumers save a card on file with a merchant, significantly boosting card-not-present transactions. While digitization has brought convenience, it has also made sensitive data more accessible to attackers.
Sitting on troves of digital payment credentials for millions of customers, retailers are a prime target for cybercriminals looking to take advantage of security gaps. According to a recent Data Breach Investigations Report from Verizon, 37% of confirmed data disclosures in the retail industry resulted in stolen payment card data.
Malware attacks, ransomware and distributed denial of service attacks are all on the rise, but phishing remains the favored vector for an initial attack. With a successful phishing intrusion, criminals can steal credentials, access systems and launch ransomware.
Best Buy manages a network of more than 1,000 stores across the U.S. and Canada. With multichannel digital sales across both countries, protecting consumer information and transactions is critical. To defend against phishing and other cyber threats, Best Buy began using customized machine learning and NVIDIA Morpheus to better secure their infrastructure and inform their security analysts.
After deploying this AI-based cyber defense, the retail giant improved the accuracy of phishing detection to 96% while reducing false-positive rates. With a proactive approach to cybersecurity, Best Buy is protecting its reputation as a tech expert focused on customer needs.
From complex supply chains to third-party vendors and multichannel point-of-sale networks, expect retailers to continue integrating AI to protect operations as well as critical proprietary and customer data.
Smart Cities and Spaces: Protecting Critical Infrastructure and Transit Networks
IoT devices and AI that analyze movement patterns, traffic and hazardous situations have great potential to improve the safety and efficiency of spaces and infrastructure. But as airports, shipping ports, transit networks and other smart spaces integrate IoT and use data, they also become more vulnerable to attack.
In the last couple of years, there have been distributed denial of service (DDoS) attacks on airports and air traffic control centers and ransomware attacks on seaports, city municipalities, police departments and more. Attacks can paralyze information systems, ground flights, disrupt the flow of cargo and traffic, and delay the delivery of goods to markets. Hostile attacks could have far more serious consequences, including physical harm or loss of life.
In connected spaces, AI-driven security can analyze vast amounts of data to predict threats, isolate attacks and provide rapid self-healing after an intrusion. AI algorithms trained on emails can halt threats in the inbox and block phishing attempts like those that delivered ransomware to seaports earlier this year. Machine learning can be trained to recognize DDoS attack patterns to prevent the type of incoming malicious traffic that brought down U.S. airport websites last year.
Organizations adopting smart space technology, such as the Port of Los Angeles, are making efforts to get ahead of the threat landscape. In 2014, the Port of LA established a cybersecurity operations center and hired a dedicated cybersecurity team. In 2021, the port followed up with a cyber resilience center to enhance early-warning detection for cyberattacks that have the potential to impact the flow of cargo.
The U.S. Federal Aviation Administration has developed an AI certification framework that assesses the trustworthiness of AI and ML applications. The FAA also implements a zero-trust cyber approach, enforces strict access control and runs continuous verification across its digital environment.
By bolstering cybersecurity and integrating AI, smart space and transport infrastructure administrators can offer secure access to physical spaces and digital networks to protect the uninterrupted movement of people and goods.
Telecommunications: Ensure Network Resilience and Block Incoming Threats
Telecommunications companies are leaning into AI to power predictive maintenance and maximum network uptime, network optimization, equipment troubleshooting, call-routing and self-service systems.
The industry is responsible for critical national infrastructure in every country, supports over 5 billion customer endpoints and is expected to constantly deliver above 99% reliability. As reliance on cloud, IoT and edge computing expands and 5G becomes the norm, immense digital surface areas must be protected from misuse and malicious attack.
Telcos can deploy AI to ensure the security and resilience of networks. AI can monitor IoT devices and edge networks to detect anomalies and intrusions, identify fake users, mitigate attacks and quarantine infected devices. AI can continuously assess the trustworthiness of devices, users and applications, thereby shortening the time needed to identify fraudsters.
Pretrained AI models can be deployed to protect 5G networks from threats such as malware, data exfiltration and DOS attacks.
Using deep learning and NVIDIA BlueField DPUs, Palo Alto Networks has built a next-generation firewall addressing 5G needs, maximizing cybersecurity performance while maintaining a small infrastructure footprint. The DPU powers accelerated intelligent network filtering to parse, classify and steer traffic to improve performance and isolate threats. With more efficient computing that deploys fewer servers, telcos can maximize return on investment for compute investments and minimize digital attack surface areas.
By putting AI to work, telcos can build secure, encrypted networks to ensure network availability and data security for both individual and enterprise customers.
Automotive: Insulate Vehicle Software From Outside Influence and Attack
Modern cars rely on complex AI and ML software stacks running on in-vehicle computers to process data from cameras and other sensors. These vehicles are essentially giant, moving IoT devices — they perceive the environment, make decisions, advise drivers and even control the vehicle with autonomous driving features.
Like other connected devices, autonomous vehicles are susceptible to various types of cyberattacks. Bad actors can infiltrate and compromise AV software both on board and from third-party providers. Denial of service attacks can disrupt over-the-air software updates that vehicles rely on to operate safely. Unauthorized access to communications systems like onboard WiFi, Bluetooth or RFID can expose vehicle systems to the risk of remote manipulation and data theft. This can jeopardize geolocation and sensor data, operational data, driver and passenger data, all of which are crucial to functional safety and the driving experience.
AI-based cybersecurity can help monitor in-car and network activities in real time, allowing for rapid response to threats. AI can be deployed to secure and authenticate over-the-air updates to prevent tampering and ensure the authenticity of software updates. AI-driven encryption can protect data transmitted over WiFi, Bluetooth and RFID connections. AI can also probe vehicle systems for vulnerabilities and take remedial steps.
Ranging from AI-powered access control to unlock and start vehicles to detecting deviations in sensor performance and patching security vulnerabilities, AI will play a crucial role in the safe development and deployment of autonomous vehicles on our roads.
Keeping Operations Secure and Customers Happy With AI Cybersecurity
By deploying AI to protect valuable data and digital operations, industries can focus their resources on innovating better products, improving customer experiences and creating new business value.
NVIDIA offers a number of tools and frameworks to help enterprises swiftly adjust to the evolving cyber risk environment. The NVIDIA Morpheus cybersecurity framework provides developers and software vendors with optimized, easy-to-use tools to build solutions that can proactively detect and mitigate threats while drastically reducing the cost of cyber defense operations. To help defend against phishing attempts, the NVIDIA spear phishing detection AI workflow uses NVIDIA Morpheus and synthetic training data created with the NVIDIA NeMo generative AI framework to flag and halt inbox threats.
The Morpheus SDK also enables digital fingerprinting to collect and analyze behavior characteristics for every user, service, account and machine across a network to identify atypical behavior and alert network operators. With the NVIDIA DOCA software framework, developers can create software-defined, DPU-accelerated services, while leveraging zero trust to build more secure applications.
AI-based cybersecurity empowers developers across industries to build solutions that can identify, capture and act on threats and anomalies to ensure business continuity and uninterrupted service, keeping operations safe and customers happy.
Learn how AI can help your organization achieve a proactive cybersecurity posture to protect customer and proprietary data to the highest standards.
Bundesliga Match Facts Shot Speed – Who fires the hardest shots in the Bundesliga?
There’s a kind of magic that surrounds a soccer shot so powerful, it leaves spectators, players, and even commentators in a momentary state of awe. Think back to a moment when the sheer force of a strike left an entire Bundesliga stadium buzzing with energy. What exactly captures our imagination with such intensity? While there are many factors that contribute to an iconic goal, there’s a particular magnetism to shots that blaze through the air, especially those taken from a distance.
Over the years, the Bundesliga has witnessed players who’ve become legends, not just for their skill but for their uncanny ability to unleash thunderbolts. Bernd Nickel, a standout figure from Frankfurt’s illustrious squad in the 1970s and 1980s, earned the title “Dr. Hammer” from ardent fans. Over his illustrious career, he netted 141 times in 426 matches.
Beyond his shooting prowess, another feat of Nickel’s that stands out is his ability to directly score from corner kicks. In fact, he holds the unique distinction of having scored from all four corner positions at Frankfurt’s Waldstadion. An example was witnessed by Frankfurt’s fans in May 1971, during a high-stakes game against Kickers Offenbach when he unveiled a masterclass.
Nickel scored a stunning goal in the 17th minute, which eventually led Frankfurt to a 2:0 victory. What made this goal even more memorable was the manner in which it was executed—a spectacular sideways scissors-kick from the penalty spot, fitting perfectly into the top corner. This goal would later be recognized as the “Goal of the Month” for May 1971. Nickl’s impact on the field was undeniable, and during the time he represented Eintracht Frankfurt, the club won the DFB-Pokal three times (in 1974, 1975, and 1981) and the UEFA Cup once in 1980.
Similarly, Thomas “the Hammer” Hitzlsberger has etched his name into Bundesliga folklore with his stunning left-footed rockets. His 2009 strike against Leverkusen at a speed of 125 km/h is one that is vividly remembered because the sheer velocity of Hitzlsperger’s free-kick was enough to leave Germany’s number one goalkeeper, René Adler, seemingly petrified.
Struck during the fifty-first minute of the game from a distance of 18 meters, the ball soared past Adler, leaving him motionless, and bulged the net, making the score 2:0. This remarkable goal not only showcased Hitzlsperger’s striking ability but also demonstrated the awe-inspiring power that such high-velocity goals can have on a match.
Historical data has shown us a few instances where the ball’s velocity exceeded the 130 km/h mark in Bundesliga, with the all-time record being a jaw-dropping shot at 137 km/h by Bayern’s Roy Makaay.
With all this in mind, it becomes even clearer why the speed and technique behind every shot matters immensely. Although high shooting speed excites soccer fans, it has not been measured regularly in the Bundesliga until now. Recognizing this, we are excited to introduce the new Bundesliga Match Facts: Shot Speed. This new metric aims to shed light on the velocity behind these incredible goals, enhancing our understanding and appreciation of the game even further.
How it works
Have you ever wondered just how fast a shot from your favorite Bundesliga player travels? The newly introduced Bundesliga Match Facts (BMF) Shot Speed now allows fans to satisfy their curiosity by providing insights into the incredible power and speed behind shots. Shot speed is more than just a number; it’s a window into the awe-inspiring athleticism and skill of the Bundesliga players.
Shot speed has a captivating effect on fans, igniting debates about which player possesses the most potent shot in the league and who consistently delivers lightning-fast strikes. Shot speed data is the key to resolving these questions.
Besides that, the new BMF helps to highlight memorable moments. The fastest shots often result in spectacular goals that live long in the memory of fans. Shot speed helps immortalize these moments, allowing fans to relive the magic of those lightning-fast strikes.
But how does this work? Let’s delve into the details.
Data collection process
A foundation of shot speed calculation lies in an organized data collection process. This process comprises two key components: event data and optical tracking data.
Event data collection entails gathering the fundamental building blocks of the game. Shots, goals, assists, fouls, and substitutions provide vital context for understanding what happens on the pitch. In our specific case, we focus on shots, their variations, and the players responsible for them.
On the flip side, optical tracking data is collected using advanced camera systems. These systems record player movements and ball positions, offering a high level of precision. This data serves as the bedrock for comprehensive analysis of player performance, tactical intricacies, and team strategies. When it comes to calculating shot speed, this data is essential for tracking the velocity of the ball.
These two streams of data originate from distinct sources, and their synchronization in time is not guaranteed. For the precision needed in shot speed calculations, we must ensure that the ball’s position aligns precisely with the moment of the event. This eliminates any discrepancies that might arise from the manual collection of event data. To achieve this, our process uses a synchronization algorithm that is trained on a labeled dataset. This algorithm robustly associates each shot with its corresponding tracking data.
Shot speed calculation
The heart of determining shot speed lies in a precise timestamp given by our synchronization algorithm. Imagine a player getting ready to take a shot. Our event gatherers are ready to record the moment, and cameras closely track the ball’s movement. The magic happens exactly when the player decides to pull the trigger.
An accurate timestamp measurement helps us figure out how fast the shot was right from the start. We measure shot speed for shots that end up as goals, those that hit the post, or get saved. To make sure we’re consistent, we don’t include headers or shots that get blocked. These can get a bit tricky due to deflections.
Let’s break down how we transform the collected data into the shot speed you see:
- Extracting shot trajectory – After recording the event and tracking the ball’s movement, we extract the trajectory of the shot. This means we map out the path the ball takes from the moment it leaves the player’s foot.
- Smoothing velocity curve – The data we get is detailed but can sometimes have tiny variations due to factors like camera sensitivity. To ensure accuracy, we smooth out the velocity curve. This means we remove any minor bumps or irregularities in the data to get a more reliable speed measurement.
- Calculating maximum speed – With a clean velocity curve in hand, we then calculate the maximum speed the ball reaches during its flight. This is the key number that represents the shot’s speed and power.
We analyzed around 215 matches from the Bundesliga 2022–2023 season. The following plot shows the number of fast shots (>100 km/h) by player. The 263 players with at least one fast shot (>100 km/h) have, on average, 3.47 fast shots. As the graph shows, some players have a frequency way above average, with around 20 fast shots.
Let’s look at some examples from the current season (2023–2024)
The following videos show examples of measured shots that achieved top-speed values.
Example 1
Measured with top shot speed 118.43 km/h with a distance to goal of 20.61 m
Example 2
Measured with top shot speed 123.32 km/h with a distance to goal of 21.19 m
Example 3
Measured with top shot speed 121.22 km/h with a distance to goal of 25.44 m
Example 4
Measured with top shot speed 113.14 km/h with a distance to goal of 24.46 m
How it’s implemented
In our quest to accurately determine shot speed during live matches, we’ve implemented a cutting-edge solution using Amazon Managed Streaming for Apache Kafka (Amazon MSK). This robust platform serves as the backbone for seamlessly streaming positional data at a rapid 25 Hz sampling rate, enabling real-time updates of shot speed. Through Amazon MSK, we’ve established a centralized hub for data streaming and messaging, facilitating seamless communication between containers for sharing a wealth of Bundesliga Match Facts.
The following diagram outlines the entire workflow for measuring shot speed from start to finish.
Match-related data is gathered and brought into the system via DFL’s DataHub. To process match metadata, we use an AWS Lambda function called MetaDataIngestion
, while positional data is brought in using an AWS Fargate container known as MatchLink
. These Lambda functions and Fargate containers then make this data available for further use in the appropriate MSK topics.
At the heart of the BMF Shot Speed lies a dedicated Fargate container named BMF ShotSpeed
. This container is active throughout the duration of the match, continuously pulling in all the necessary data from Amazon MSK. Its algorithm responds instantly to every shot taken during the game, calculating the shot speed in real time. Moreover, we have the capability to recompute shot speed should any underlying data undergo updates.
Once the shot speeds have undergone their calculations, the next phase in our data journey is the distribution. The shot speed metrics are transmitted back to DataHub, where they are made available to various consumers of Bundesliga Match Facts.
Simultaneously, the shot speed data finds its way to a designated topic within our MSK cluster. This allows other components of Bundesliga Match Facts to access and take advantage of this metric. We’ve implemented an AWS Lambda function with the specific task of retrieving the calculated shot speed from the relevant Kafka topic. Once the Lambda function is triggered, it stores the data in an Amazon Aurora Serverless database. This database houses the shot speed data, which we then use to create interactive, near real-time visualizations using Amazon QuickSight.
Beyond this, we have a dedicated component specifically designed to calculate a seasonal ranking of shot speeds. This allows us to keep track of the fastest shots throughout the season, ensuring that we always have up-to-date information about the fastest shots and their respective rankings after each shot is taken.
Summary
In this blog post, we’re excited to introduce the all-new Bundesliga Match Facts: Shot Speed, a metric that allows us to quantify and objectively compare the velocity of shots taken by different Bundesliga players. This statistic will provide commentators and fans with valuable insights into the power and speed of shots on goal.
The development of the Bundesliga Match Facts is the result of extensive analysis conducted by a collaborative team of soccer experts and data scientists from the Bundesliga and AWS. Notable shot speeds will be displayed in real time on the live ticker during matches, accessible through the official Bundesliga app and website. Additionally, this data will be made readily available to commentators via the Data Story Finder and visually presented to fans at key moments during broadcasts.
We’re confident that the introduction of this brand-new Bundesliga Match Fact will enhance your understanding of the game and add a new dimension to your viewing experience. To delve deeper into the partnership between AWS and Bundesliga, please visit Bundesliga on AWS!
We’re eagerly looking forward to the insights you uncover with this new Shot Speed metric. Share your findings with us on X: @AWScloud, using the hashtag #BundesligaMatchFacts.
About the Authors
Tareq Haschemi is a consultant within AWS Professional Services. His skills and areas of expertise include application development, data science, and machine learning (ML). He supports customers in developing data-driven applications within the AWS Cloud. Prior to joining AWS, he was also a consultant in various industries, such as aviation and telecommunications. He is passionate about enabling customers on their data and artificial intelligence (AI) journey to the cloud.
Jean-Michel Lourier is a Senior Data Scientist within AWS Professional Services. He leads teams implementing data-driven applications side-by-side with AWS customers to generate business value out of their data. He’s passionate about diving into tech and learning about AI, ML, and their business applications. He is also an enthusiastic cyclist, taking long bike-packing trips.
Luc Eluère is a Data Scientist within Sportec Solutions AG. His mission is to develop and provide valuable KPIs to the soccer industry. At university, he learned the statistical theory with a goal: to apply its concepts to the beautiful game. Even though he was promised a nice career in table soccer, his passion for data science took over, and he chose computers as a career path.
Javier Poveda-Panter is a Senior Data and Machine Learning Engineer for EMEA sports customers within the AWS Professional Services team. He enables customers in the area of spectator sports to innovate and capitalize on their data, delivering high-quality user and fan experiences through ML, data science, and analytics. He follows his passion for a broad range of sports, music, and AI in his spare time.
Zero-shot adaptive prompting of large language models
Recent advances in large language models (LLMs) are very promising as reflected in their capability for general problem-solving in few-shot and zero-shot setups, even without explicit training on these tasks. This is impressive because in the few-shot setup, LLMs are presented with only a few question-answer demonstrations prior to being given a test question. Even more challenging is the zero-shot setup, where the LLM is directly prompted with the test question only.
Even though the few-shot setup has dramatically reduced the amount of data required to adapt a model for a specific use-case, there are still cases where generating sample prompts can be challenging. For example, handcrafting even a small number of demos for the broad range of tasks covered by general-purpose models can be difficult or, for unseen tasks, impossible. For example, for tasks like summarization of long articles or those that require domain knowledge (e.g., medical question answering), it can be challenging to generate sample answers. In such situations, models with high zero-shot performance are useful since no manual prompt generation is required. However, zero-shot performance is typically weaker as the LLM is not presented with guidance and thus is prone to spurious output.
In “Better Zero-shot Reasoning with Self-Adaptive Prompting”, published at ACL 2023, we propose Consistency-Based Self-Adaptive Prompting (COSP) to address this dilemma. COSP is a zero-shot automatic prompting method for reasoning problems that carefully selects and constructs pseudo-demonstrations for LLMs using only unlabeled samples (that are typically easy to obtain) and the models’ own predictions. With COSP, we largely close the performance gap between zero-shot and few-shot while retaining the desirable generality of zero-shot prompting. We follow this with “Universal Self-Adaptive Prompting“ (USP), accepted at EMNLP 2023, in which we extend the idea to a wide range of general natural language understanding (NLU) and natural language generation (NLG) tasks and demonstrate its effectiveness.
Prompting LLMs with their own outputs
Knowing that LLMs benefit from demonstrations and have at least some zero-shot abilities, we wondered whether the model’s zero-shot outputs could serve as demonstrations for the model to prompt itself. The challenge is that zero-shot solutions are imperfect, and we risk giving LLMs poor quality demonstrations, which could be worse than no demonstrations at all. Indeed, the figure below shows that adding a correct demonstration to a question can lead to a correct solution of the test question (Demo1 with question), whereas adding an incorrect demonstration (Demo 2 + questions, Demo 3 with questions) leads to incorrect answers. Therefore, we need to select reliable self-generated demonstrations.
![]() |
Example inputs & outputs for reasoning tasks, which illustrates the need for carefully designed selection procedure for in-context demonstrations (MultiArith dataset & PaLM-62B model): (1) zero-shot chain-of-thought with no demo: correct logic but wrong answer; (2) correct demo (Demo1) and correct answer; (3) correct but repetitive demo (Demo2) leads to repetitive outputs; (4) erroneous demo (Demo3) leads to a wrong answer; but (5) combining Demo3 and Demo1 again leads to a correct answer. |
COSP leverages a key observation of LLMs: that confident and consistent predictions are more likely correct. This observation, of course, depends on how good the uncertainty estimate of the LLM is. Luckily, in large models, previous works suggest that the uncertainty estimates are robust. Since measuring confidence requires only model predictions, not labels, we propose to use this as a zero-shot proxy of correctness. The high-confidence outputs and their inputs are then used as pseudo-demonstrations.
With this as our starting premise, we estimate the model’s confidence in its output based on its self-consistency and use this measure to select robust self-generated demonstrations. We ask LLMs the same question multiple times with zero-shot chain-of-thought (CoT) prompting. To guide the model to generate a range of possible rationales and final answers, we include randomness controlled by a “temperature” hyperparameter. In an extreme case, if the model is 100% certain, it should output identical final answers each time. We then compute the entropy of the answers to gauge the uncertainty — the answers that have high self-consistency and for which the LLM is more certain, are likely to be correct and will be selected.
Assuming that we are presented with a collection of unlabeled questions, the COSP method is:
- Input each unlabeled question into an LLM, obtaining multiple rationales and answers by sampling the model multiple times. The most frequent answers are highlighted, followed by a score that measures consistency of answers across multiple sampled outputs (higher is better). In addition to favoring more consistent answers, we also penalize repetition within a response (i.e., with repeated words or phrases) and encourage diversity of selected demonstrations. We encode the preference towards consistent, un-repetitive and diverse outputs in the form of a scoring function that consists of a weighted sum of the three scores for selection of the self-generated pseudo-demonstrations.
- We concatenate the pseudo-demonstrations into test questions, feed them to the LLM, and obtain a final predicted answer.
COSP focuses on question-answering tasks with CoT prompting for which it is easy to measure self-consistency since the questions have unique correct answers. But this can be difficult for other tasks, such as open-ended question-answering or generative tasks that don’t have unique answers (e.g., text summarization). To address this limitation, we introduce USP in which we generalize our approach to other general NLP tasks:
- Classification (CLS): Problems where we can compute the probability of each class using the neural network output logits of each class. In this way, we can measure the uncertainty without multiple sampling by computing the entropy of the logit distribution.
- Short-form generation (SFG): Problems like question answering where we can use the same procedure mentioned above for COSP, but, if necessary, without the rationale-generating step.
- Long-form generation (LFG): Problems like summarization and translation, where the questions are often open-ended and the outputs are unlikely to be identical, even if the LLM is certain. In this case, we use an overlap metric in which we compute the average of the pairwise ROUGE score between the different outputs to the same query.
We compute the relevant confidence scores depending on the type of task on the aforementioned set of unlabeled test samples. After scoring, similar to COSP, we pick the confident, diverse and less repetitive answers to form a model-generated pseudo-demonstration set. We finally query the LLM again in a few-shot format with these pseudo-demonstrations to obtain the final predictions on the entire test set.
Key Results
For COSP, we focus on a set of six arithmetic and commonsense reasoning problems, and we compare against 0-shot-CoT (i.e., “Let’s think step by step“ only). We use self-consistency in all baselines so that they use roughly the same amount of computational resources as COSP. Compared across three LLMs, we see that zero-shot COSP significantly outperforms the standard zero-shot baseline.
![]() |
Key results of COSP in six arithmetic (MultiArith, GSM-8K, AddSub, SingleEq) and commonsense (CommonsenseQA, StrategyQA) reasoning tasks using PaLM-62B, PaLM-540B and GPT-3 (code-davinci-001) models. |
For USP, we expand our analysis to a much wider range of tasks, including more than 25 classifications, short-form generation, and long-form generation tasks. Using the state-of-the-art PaLM 2 models, we also test against the BIG-Bench Hard suite of tasks where LLMs have previously underperformed compared to people. We show that in all cases, USP again outperforms the baselines and is competitive to prompting with golden examples.
![]() |
Accuracy on BIG-Bench Hard tasks with PaLM 2-M (each line represents a task of the suite). The gain/loss of USP (green stars) over standard 0-shot (green triangles) is shown in percentages. “Human” refers to average human performance; “AutoCoT” and “Random demo” are baselines we compared against in the paper; and “3-shot” is the few-shot performance for three handcrafted demos in CoT format. |
We also analyze the working mechanism of USP by validating the key observation above on the relation between confidence and correctness, and we found that in an overwhelming majority of the cases, USP picks confident predictions that are more likely better in all task types considered, as shown in the figure below.
Conclusion
Zero-shot inference is a highly sought-after capability of modern LLMs, yet the success in which poses unique challenges. We propose COSP and USP, a family of versatile, zero-shot automatic prompting techniques applicable to a wide range of tasks. We show large improvement over the state-of-the-art baselines over numerous task and model combinations.
Acknowledgements
This work was conducted by Xingchen Wan, Ruoxi Sun, Hootan Nakhost, Hanjun Dai, Julian Martin Eisenschlos, Sercan Ö. Arık, and Tomas Pfister. We would like to thank Jinsung Yoon Xuezhi Wang for providing helpful reviews, and other colleagues at Google Cloud AI Research for their discussion and feedback.
Using teacher knowledge at inference time to enhance student model
New method improves the state of the art in knowledge distillation by leveraging a knowledge base of teacher predictions.Read More
Deploy ML models built in Amazon SageMaker Canvas to Amazon SageMaker real-time endpoints
Amazon SageMaker Canvas now supports deploying machine learning (ML) models to real-time inferencing endpoints, allowing you take your ML models to production and drive action based on ML-powered insights. SageMaker Canvas is a no-code workspace that enables analysts and citizen data scientists to generate accurate ML predictions for their business needs.
Until now, SageMaker Canvas provided the ability to evaluate an ML model, generate bulk predictions, and run what-if analyses within its interactive workspace. But now you can also deploy the models to Amazon SageMaker endpoints for real-time inferencing, making it effortless to consume model predictions and drive actions outside the SageMaker Canvas workspace. Having the ability to directly deploy ML models from SageMaker Canvas eliminates the need to manually export, configure, test, and deploy ML models into production, thereby saving reducing complexity and saving time. It also makes operationalizing ML models more accessible to individuals, without the need to write code.
In this post, we walk you through the process to deploy a model in SageMaker Canvas to a real-time endpoint.
Overview of solution
For our use case, we are assuming the role of a business user in the marketing department of a mobile phone operator, and we have successfully created an ML model in SageMaker Canvas to identify customers with the potential risk of churn. Thanks to the predictions generated by our model, we now want to move this from our development environment to production. To streamline the process of deploying our model endpoint for inference, we directly deploy ML models from SageMaker Canvas, thereby eliminating the need to manually export, configure, test, and deploy ML models into production. This helps reduce complexity, saves time, and also makes operationalizing ML models more accessible to individuals, without the need to write code.
The workflow steps are as follows:
- Upload a new dataset with the current customer population into SageMaker Canvas. For the full list of supported data sources, refer to Import data into Canvas.
- Build ML models and analyze their performance metrics. For instructions, refer to Build a custom model and Evaluate Your Model’s Performance in Amazon SageMaker Canvas.
- Deploy the approved model version as an endpoint for real-time inferencing.
You can perform these steps in SageMaker Canvas without writing a single line of code.
Prerequisites
For this walkthrough, make sure that the following prerequisites are met:
- To deploy model versions to SageMaker endpoints, the SageMaker Canvas admin must give the necessary permissions to the SageMaker Canvas user, which you can manage in the SageMaker domain that hosts your SageMaker Canvas application. For more information, refer to Permissions Management in Canvas.
- Implement the prerequisites mentioned in Predict customer churn with no-code machine learning using Amazon SageMaker Canvas.
You should now have three model versions trained on historical churn prediction data in Canvas:
- V1 trained with all 21 features and quick build configuration with a model score of 96.903%
- V2 trained with all 19 features (removed phone and state features) and quick build configuration and improved accuracy of 97.403%
- V3 trained with standard build configuration with 97.103% model score
Use the customer churn prediction model
Enable Show advanced metrics on the model details page and review the objective metrics associated with each model version so that you can select the best-performing model for deploying to SageMaker as an endpoint.
Based on the performance metrics, we select version 2 to be deployed.
Configure the model deployment settings—deployment name, instance type, and instance count.
As a starting point, Canvas will automatically recommend the best instance type and the number of instances for your model deployment. You can change it as per your workload needs.
You can test the deployed SageMaker inference endpoint directly from within SageMaker Canvas.
You can change input values using the SageMaker Canvas user interface to infer additional churn prediction.
Now let’s navigate to Amazon SageMaker Studio and check out the deployed endpoint.
Open a notebook in SageMaker Studio and run the following code to infer the deployed model endpoint. Replace the model endpoint name with your own model endpoint name.
Our original model endpoint is using an ml.m5.xlarge instance and 1 instance count. Now, let’s assume you expect the number of end-users inferencing your model endpoint will increase and you want to provision more compute capacity. You can accomplish this directly from within SageMaker Canvas by choosing Update configuration.
Clean up
To avoid incurring future charges, delete the resources you created while following this post. This includes logging out of SageMaker Canvas and deleting the deployed SageMaker endpoint. SageMaker Canvas bills you for the duration of the session, and we recommend logging out of SageMaker Canvas when you’re not using it. Refer to Logging out of Amazon SageMaker Canvas for more details.
Conclusion
In this post, we discussed how SageMaker Canvas can deploy ML models to real-time inferencing endpoints, allowing you take your ML models to production and drive action based on ML-powered insights. In our example, we showed how an analyst can quickly build a highly accurate predictive ML model without writing any code, deploy it on SageMaker as an endpoint, and test the model endpoint from SageMaker Canvas, as well as from a SageMaker Studio notebook.
To start your low-code/no-code ML journey, refer to Amazon SageMaker Canvas.
Special thanks to everyone who contributed to the launch: Prashanth Kurumaddali, Abishek Kumar, Allen Liu, Sean Lester, Richa Sundrani, and Alicia Qi.
About the Authors
Janisha Anand is a Senior Product Manager in the Amazon SageMaker Low/No Code ML team, which includes SageMaker Canvas and SageMaker Autopilot. She enjoys coffee, staying active, and spending time with her family.
Indy Sawhney is a Senior Customer Solutions Leader with Amazon Web Services. Always working backward from customer problems, Indy advises AWS enterprise customer executives through their unique cloud transformation journey. He has over 25 years of experience helping enterprise organizations adopt emerging technologies and business solutions. Indy is an area of depth specialist with AWS’s Technical Field Community for AI/ML, with specialization in generative AI and low-code/no-code Amazon SageMaker solutions.
Develop generative AI applications to improve teaching and learning experiences
Recently, teachers and institutions have looked for different ways to incorporate artificial intelligence (AI) into their curriculums, whether it be teaching about machine learning (ML) or incorporating it into creating lesson plans, grading, or other educational applications. Generative AI models, in particular large language models (LLMs), have dramatically sped up AI’s impact on education. Generative AI and natural language programming (NLP) models have great potential to enhance teaching and learning by generating personalized learning content and providing engaging learning experiences for students.
In this post, we create a generative AI solution for teachers to create course materials and for students to learn English words and sentences. When students provide answers, the solution provides real-time assessments and offers personalized feedback and guidance for students to improve their answers.
Specifically, teachers can use the solution to do the following:
- Create an assignment for students by generating questions and answers from a prompt
- Create an image from the prompt to represent the assignment
- Save the new assignment to a database
- Browse existing assignments from the database
Students can use the solution to do the following:
- Select and review an assignment from the assignment database
- Answer the questions of the selected assignment
- Check the grading scores of the answers in real time
- Review the suggested grammatical improvements to their answers
- Review the suggested sentence improvements to their answers
- Read the recommended answers
We walk you through the steps of creating the solution using Amazon Bedrock, Amazon Elastic Container Service (Amazon ECS), Amazon CloudFront, Elastic Load Balancing (ELB), Amazon DynamoDB, Amazon Simple Storage Service (Amazon S3), and AWS Cloud Development Kit (AWS CDK).
Solution overview
The following diagram shows the resources and services used in the solution.
The solution runs as a scalable service. Teachers and students use their browsers to access the application. The content is served through an Amazon CloudFront distribution with an Application Load Balancer as its origin. It saves the generated images to an S3 bucket, and saves the teacher’s assignments and the students’ answers and scores to separate DynamoDB tables.
The solution uses Amazon Bedrock to generate questions, answers, assignment images and to grade students’ answers. Amazon Bedrock is a fully managed service that makes foundation models from leading AI startups and Amazon available via easy-to-use API interfaces. The solution also uses the grammatical error correction API and the paraphrase API from AI21 to recommend word and sentence corrections.
You can find the implementation details in the following sections. The source code is available in the GitHub repository.
Prerequisites
You should have some knowledge of generative AI, ML, and the services used in this solution, including Amazon Bedrock, Amazon ECS, Amazon CloudFront, Elastic Load Balancing, Amazon DynamoDB and Amazon S3
We use AWS CDK to build and deploy the solution. You can find the setup instructions in the readme file.
Create assignments
Teachers can create an assignment from an input text using the following GUI page. An assignment comprises an input text, the questions and answers generated from the text, and an image generated from the input text to represent the assignment.
For our example, a teacher inputs the Kids and Bicycle Safety guidelines from the United States Department of Transportation. For the input text, we use the file bike.safe.riding.tips.txt.
The following is the generated image output.
The following are the generated questions and answers:
"question": "What should you always wear when riding a bicycle?",
"answer": "You should always wear a properly fitted bicycle helmet when riding a bicycle. A helmet protects your brain and can save your life in a crash."
"question": "How can you make sure drivers can see you when you are bicycling?",
"answer": "To make sure drivers can see you, wear bright neon or fluorescent colors. Also use reflective tape, markings or flashing lights so you are visible."
"question": "What should you do before riding your bicycle?",
"answer": "Before riding, you should inspect your bicycle to make sure all parts are secure and working properly. Check that tires are inflated, brakes work properly, and reflectors are in place."
"question": "Why is it more dangerous to ride a bicycle at night?",
"answer": "It is more dangerous to ride at night because it is harder for other people in vehicles to see you in the dark."
"question": "How can you avoid hazards while bicycling?",
"answer": "Look ahead for hazards like potholes, broken glass, and dogs. Point out and yell about hazards to bicyclists behind you. Avoid riding at night when it is harder to see hazards."
The teacher expects the students to complete the assignment by reading the input text and then answering the generated questions.
The portal uses Amazon Bedrock to create questions, answers, and images. Amazon Bedrock speeds up the development of generative AI solutions by exposing the foundation models through API interfaces. You can find the source code in the file 1_Create_Assignments.py.
The portal invokes two foundation models:
- Stable Diffusion XL to generate images using the function
query_generate_image_endpoint
- Anthropic Claude v2 to generate questions and answers using the function
query_generate_questions_answers_endpoint
The portal saves generated images to an S3 bucket using the function load_file_to_s3. It creates an assignment based on the input text, the teacher ID, the generated questions and answers, and the S3 bucket link for the loaded image. It saves the assignment to the DynamoDB table assignments using the function insert_record_to_dynamodb
.
You can find the AWS CDK code that creates the DynamoDB table in the file cdk_stack.py.
Show assignments
Teachers can browse assignments and the generated artifacts using the following GUI page.
The portal uses the function get_records_from_dynamodb
to retrieve the assignments from the DynamoDB table assignments. It uses the function download_image
to download an image from the S3 bucket. You can find the source code in the file 2_Show_Assignments.py.
Answer questions
A student selects and reads a teacher’s assignment and then answers the questions of the assignment.
The portal provides an engaging learning experience. For example, when the student provides the answer “I should waer hat protect brain in crash” the portal grades the answer in real time by comparing the answer with the correct answer. The portal also ranks all students’ answers to the same question and shows the top three scores. You can find the source code in the file 3_Complete_Assignments.py.
The portal saves the student’s answers to a DynamoDB table called answers. You can find the AWS CDK code that creates the DynamoDB table in the file cdk_stack.py.
To grade a student’s answer, the portal invokes the Amazon Titan Embeddings model to translate the student’s answer and the correct answer into numerical representations and then compute their similarity as a score. You can find the solution in the file 3_Complete_Assignments.py.
The portal generates suggested grammatical corrections and sentence improvements for the student’s answer. Finally, the portal shows the correct answer to the question.
The portal uses the grammatical error correction API and the paraphrase API from AI21 to generate the recommended grammatical and sentence improvements. The AI21 paraphrase model is available as a foundation model in SageMaker. You can deploy the AI21 paraphrase model as an inference point in SageMaker and invoke the inference point to generate sentence improvements.
The functions generate_suggestions_sentence_improvements
and generate_suggestions_word_improvements
in the file 3_Complete_Assignments.py show an alternative way of using the AI21 REST API endpoints. You need to create an AI21 account and find the API key associated with your account to invoke the APIs. You will have to pay for the invocations after the trial period.
Conclusion
This post showed you how to use an AI-assisted solution to improve the teaching and learning experience by using multiple generative AI and NLP models. You can use the same approach to develop other generative AI prototypes and applications.
If you’re interested in the fundamentals of generative AI and how to work with foundation models, including advanced prompting techniques, check out the hands-on course Generative AI with LLMs. It’s an on-demand, 3-week course for data scientists and engineers who want to learn how to build generative AI applications with LLMs. It’s a good foundation to start building with Amazon Bedrock. Visit the Amazon Bedrock Features page and sign up to learn more about Amazon Bedrock.
About the Authors
Jeff Li is a Senior Cloud Application Architect with the Professional Services team at AWS. He is passionate about diving deep with customers to create solutions and modernize applications that support business innovations. In his spare time, he enjoys playing tennis, listening to music, and reading.
Isaac Privitera is a Senior Data Scientist at the Generative AI Innovation Center, where he develops bespoke generative AI based solutions to address customers’ business problems. He works primarily on building responsible AI systems using retrieval augmented generation (RAG) and chain of thought reasoning. In his spare time he enjoys golf, football, and walking with his dog Barry.
Harish Vaswani is a Principal Cloud Application Architect at Amazon Web Services. He specializes in architecting and building cloud native applications and enables customers with best practices in their cloud transformation journey. Outside of work, Harish and his wife, Simin, are award-winning independent short film producers and love spending their time with their 5-year old son, Karan.
How Are Foundation Models Used in Gaming?
AI technologies are having a massive impact across industries, including media and entertainment, automotive, customer service and more. For game developers, these advances are paving the way for creating more realistic and immersive in-game experiences.
From creating lifelike characters that convey emotions to transforming simple text into captivating imagery, foundation models are becoming essential in accelerating developer workflows while reducing overall costs. These powerful AI models have unlocked a realm of possibilities, empowering designers and game developers to build higher-quality gaming experiences.
What Are Foundation Models?
A foundation model is a neural network that’s trained on massive amounts of data — and then adapted to tackle a wide variety of tasks. They’re capable of enabling a range of general tasks, such as text, image and audio generation. Over the last year, the popularity and use of foundation models has rapidly increased, with hundreds now available.
For example, GPT-4 is a large multimodal model developed by OpenAI that can generate human-like text based on context and past conversations. Another, DALL-E 3, can create realistic images and artwork from a description written in natural language.
Powerful foundation models like NVIDIA NeMo and Edify model in NVIDIA Picasso make it easy for companies and developers to inject AI into their existing workflows. For example, using the NVIDIA NeMo framework, organizations can quickly train, customize and deploy generative AI models at scale. And using NVIDIA Picasso, teams can fine-tune pretrained Edify models with their own enterprise data to build custom products and services for generative AI images, videos, 3D assets, texture materials and 360 HDRi.
How Are Foundation Models Built?
Foundation models can be used as a base for AI systems that can perform multiple tasks. Organizations can easily and quickly use a large amount of unlabeled data to create their own foundation models.
The dataset should be as large and diverse as possible, as too little data or poor-quality data can lead to inaccuracies — sometimes called hallucinations — or cause finer details to go missing in generated outputs.
Next, the dataset must be prepared. This includes cleaning the data, removing errors and formatting it in such a way that the model can understand it. Bias is a pervasive issue when preparing a dataset, so it’s important to measure, reduce and tackle these inconsistencies and inaccuracies.
Training a foundational model can be time-consuming, especially given the size of the model and the amount of data required. Hardware like NVIDIA A100 or H100 Tensor Core GPUs, along with high-performance data systems like the NVIDIA DGX SuperPOD, can accelerate training. For example, ChatGPT-3 was trained on over 1,000 NVIDIA A100 GPUs over about 34 days.
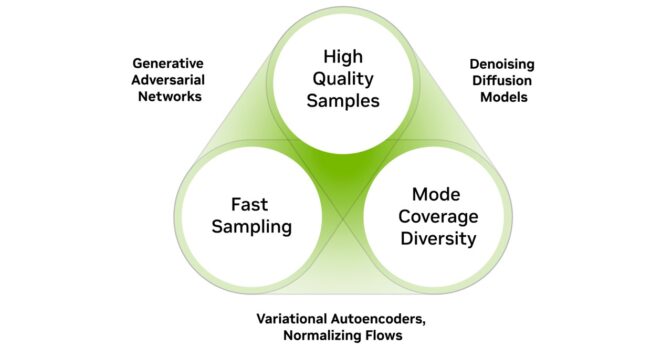
After training, the foundation model is evaluated on quality, diversity and speed. There are several methods for evaluating performance, for example:
- Tools and frameworks that quantify how well the model predicts a sample of text
- Metrics that compare generated outputs with one or more references and measure the similarities between them
- Human evaluators who assess the quality of the generated output on various criteria
Once the model passes the relevant tests and evaluations, it can then be deployed for production.
Exploring Foundation Models in Games
Pretrained foundation models can be leveraged by middleware, tools and game developers both during production and at run-time. To train a base model, resources and time are necessary — alongside a certain level of expertise. Currently, many developers within the gaming industry are exploring off-the-shelf models, but need custom solutions that fit their specific use cases. They need models that are trained on commercially safe data and optimized for real-time performance — without exorbitant costs of deployment. The difficulty of meeting these requirements has slowed adoption of foundation models.
However, innovation within the generative AI space is swift, and once major hurdles are addressed, developers of all sizes — from startups to AAA studios — will use foundation models to gain new efficiencies in game development and accelerate content creation. Additionally, these models can help create completely new gameplay experiences.
The top industry use cases are centered around intelligent agents and AI-powered animation and asset creation. For example, many creators today are exploring models for creating intelligent non-playable characters, or NPCs.
Custom LLMs fine-tuned with the lingo and lore of specific games can generate human-like text, understand context and respond to prompts in a coherent manner. They’re designed to learn patterns and language structures and understand game state changes — evolving and progressing alongside the player in the game.
As NPCs become increasingly dynamic,real-time animation and audio that sync with their responses will be needed. Developers are using NVIDIA Riva to create expressive character voices using speech and translation AI. And designers are tapping NVIDIA Audio2Face for AI-powered facial animations.
Foundation models are also being used for asset and animation generation. Asset creation during the pre-production and production phases of game development can be time-consuming, tedious and expensive.
With state-of-the-art diffusion models, developers can iterate more quickly, freeing up time to spend on the most important aspects of the content pipeline, such as developing higher-quality assets and iterating. The ability to fine-tune these models from a studio’s own repository of data ensures the outputs generated are similar to the art styles and designs of their previous games.
Foundation models are readily available, and the gaming industry is only in the beginning phases of understanding their full capabilities. Various solutions have been built for real-time experiences, but the use cases are limited. Fortunately, developers can easily access models and microservices through cloud APIs today and explore how AI can affect their games and scale their solutions to more customers and devices than ever before.
The Future of Foundation Models in Gaming
Foundation models are poised to help developers realize the future of gaming. Diffusion models and large language models are becoming much more lightweight as developers look to run them natively on a range of hardware power profiles, including PCs, consoles and mobile devices.
The accuracy and quality of these models will only continue to improve as developers look to generate high-quality assets that need little to no touching up before being dropped into an AAA gaming experience.
Foundation models will also be used in areas that have been challenging for developers to overcome with traditional technology. For example, autonomous agents can help analyze and detect world space during game development, which will accelerate processes for quality assurance.
The rise of multimodal foundation models, which can ingest a mix of text, image, audio and other inputs simultaneously, will further enhance player interactions with intelligent NPCs and other game systems. Also, developers can use additional input types to improve creativity and enhance the quality of generated assets during production.
Multimodal models also show great promise in improving the animation of real-time characters, one of the most time-intensive and expensive processes of game development. They may be able to help make characters’ locomotion identical to real-life actors, infuse style and feel from a range of inputs, and ease the rigging process.
Learn More About Foundation Models in Gaming
From enhancing dialogue and generating 3D content to creating interactive gameplay, foundation models have opened up new opportunities for developers to forge the future of gaming experiences.
Learn more about foundation models and other technologies powering game development workflows.
GeForce NOW-vember Brings Over 50 New Games to Stream In the Cloud
Gear up with gratitude for more gaming time. GeForce NOW brings members a cornucopia of 15 newly supported games to the cloud this week. That’s just the start — there are a total of 54 titles coming in the month of November.
Members can also join thousands of esports fans in the cloud with the addition of Virtex Stadium to the GeForce NOW library for a ‘League of Legends’ world championship viewing party.
Esports Like Never Before
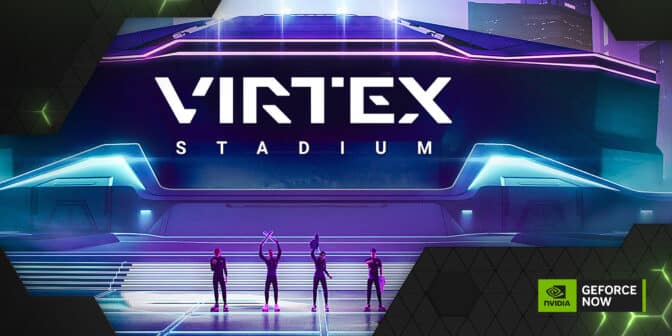
This year’s League of Legends world championship finals are coming to Virtex Stadium — an online virtual stadium now streaming on NVIDIA’s cloud gaming infrastructure.
In Virtex Stadium, esports fans can hang out with friends from across the world, create and personalize avatars, and watch live competitions together — all from the comfort of their homes.
Starting on Thursday, Nov. 2, watch League of Legends Worlds 2023 in Virtex Stadium with thousands of others. Use props and emotes to cheer players on together via chat.
GeForce NOW members and League of Legends fans can drop into Virtex Stadium without needing to create a new login. Within the Virtex Stadium app, members can choose to create a “Ready Player Me” avatar and account to save their digital characters for future visits. Members can even link their Twitch accounts to chat and emote with other viewers while in the stadium.
Catch all the action on the following dates:
- Quarterfinal 1: Nov. 2 at 9 a.m. CET
- Quarterfinal 2: Nov. 3 at 9 a.m. CET
- Quarterfinal 3: Nov. 4 at 9 a.m. CET
- Quarterfinal 4: Nov. 5 at 9 a.m. CET
- Semifinal 1: Nov. 11 at 9 a.m. CET
- Semifinal 2: Nov. 12 at 9 a.m. CET
- Final: Nov. 19 at 9 a.m. CET
Time to Shine
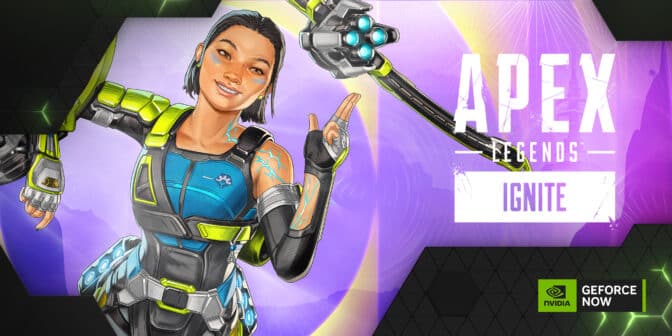
Electronic Arts’ and Respawn Entertainment’s Apex Legends: Ignite, the newest season for the battle royale first-person shooter, is now available to stream from the cloud. Light the way with Conduit, the new support Legend with shield-based abilities. Plus, check out a faster and deadlier Storm Point map, a new Battle Pass with rewards, and more to help ignite Apex Legends players’ ways to victory.
Members can start their adventures now, along with 15 other games newly supported in the cloud this week:
- Headbangers: Rhythm Royale (New release on Steam, Xbox and available on PC Game Pass, Oct. 31)
- Jusant (New release on Steam, Xbox and available on PC Game Pass, Oct. 31)
- RoboCop: Rogue City (New release on Steam, Nov. 2)
- The Talos Principle 2 (New release on Steam, Nov. 2)
- StrangerZ (New release on Steam, Nov. 3)
- Curse of the Dead Gods (Xbox, available on Microsoft Store)
- Daymare 1994: Sandcastle (Steam)
- ENDLESS Dungeon (Steam)
- F1 Manager 2023 (Xbox, available on PC Game Pass)
- Heretic’s Fork (Steam)
- HOT WHEELS UNLEASHED 2 – Turbocharged (Epic Games Store)
- Kingdoms Reborn (Steam)
- Q.U.B.E. 2 (Epic Games Store)
- Soulstice (Epic Games Store)
- Virtex Stadium (Free)
Then check out the plentiful games for the rest of November:
- The Invincible (New release on Steam, Nov 6.)
- Roboquest (New release on Steam, Nov. 7)
- Stronghold: Definitive Edition (New release on Steam, Nov. 7)
- Dungeons 4 (New release on Steam, Xbox and available on PC Game Pass, Nov. 9)
- Space Trash Scavenger (New release on Steam, Nov. 9)
- Spirittea (New release on Steam, Xbox and available on PC Game Pass, Nov 13)
- Naheulbeuk’s Dungeon Master (New release on Steam, Nov 15)
- Last Train Home (New release on Steam, Nov. 28)
- Gangs of Sherwood (New release on Steam, Nov. 30)
- Airport CEO (Steam)
- Arcana of Paradise —The Tower (Steam)
- Blazing Sails: Pirate Battle Royale (Epic Games Store)
- Breathedge (Xbox, available on Microsoft Store)
- Bridge Constructor: The Walking Dead (Xbox, available on Microsoft Store)
- Bus Simulator 21 (Xbox, available on Microsoft Store)
- Farming Simulator 19 (Xbox, available on Microsoft Store)
- GoNNER (Xbox, available on Microsoft Store)
- GoNNER2 (Xbox, available on Microsoft Store)
- Hearts of Iron IV (Xbox, available on Microsoft Store)
- Hexarchy (Steam)
- I Am Future (Epic Games Store)
- Imagine Earth (Xbox, available on Microsoft Store)
- Jurassic World Evolution 2 (Xbox, available on PC Game Pass)
- Land of the Vikings (Steam)
- Onimusha: Warlords (Steam)
- Overcooked! 2 (Xbox, available on Microsoft Store)
- Saints Row IV (Xbox, available on Microsoft Store)
- Settlement Survival (Steam)
- SHENZHEN I/O (Xbox, available on Microsoft Store)
- SOULVARS (Steam)
- The Surge 2 (Xbox, available on Microsoft Store)
- Thymesia (Xbox, available on Microsoft Store)
- Trailmakers (Xbox, available on PC Game Pass)
- Tropico 6 (Xbox, available on Microsoft Store)
- Wartales (Xbox, available on PC Game Pass)
- The Wonderful One: After School Hero (Steam)
- Warhammer Age of Sigmar: Realms of Ruin (Steam)
- West of Dead (Xbox, available on Microsoft Store)
- Wolfenstein: The New Order (Xbox, available on PC Game Pass)
- Wolfenstein: The Old Blood (Steam, Epic Games Store, Xbox and available on PC Game Pass)
Outstanding October
On top of the 60 games announced in October, an additional 48 joined the cloud last month, including several from this week’s additions, Curse of the Dead Gods, ENDLESS Dungeon, Farming Simulator 19, Hearts of Iron IV, Kingdoms Reborn, RoboCop: Rogue City, StrangerZ, The Talos Principle 2, Thymesia, Tropico 6 and Virtex Stadium:
- AirportSim (New release on Steam, Oct. 19)
- Battle Chasers: Nightwar (Xbox, available on Microsoft Store)
- Black Skylands (Xbox, available on Microsoft Store)
- Blair Witch (Xbox, available on Microsoft Store)
- Call of the Sea (Xbox, available on Microsoft Store
- Chicory: A Colorful Tale (Xbox and available on PC Game Pass)
- Cricket 22 (Xbox and available on PC Game Pass)
- Dead by Daylight (Xbox and available on PC Game Pass)
- Deceive Inc. (Epic Games Store)
- Dishonored (Steam)
- Dishonored: Death of the Outsider (Steam, Epic Games Store, Xbox and available on PC Game Pass)
- Dishonored Definitive Edition (Epic Games Store, Xbox and available on PC Game Pass)
- Dishonored 2 (Steam, Epic Games Store, Xbox and available on PC Game Pass)
- Dune: Spice Wars (Xbox and available on PC Game Pass)
- Eternal Threads (New release on Epic Games Store, Oct. 19)
- Everspace 2 (Xbox and available on PC Game Pass)
- EXAPUNKS (Xbox and available on PC Game Pass)
- From Space (New release on Xbox, available on PC Game Pass, Oct. 12)
- Ghostrunner 2 (New release on Steam, Oct. 26)
- Ghostwire: Tokyo (Steam, Epic Games Store, Xbox and available on PC Game Pass)
- Golf With Your Friends (Xbox, available on PC Game Pass)
- Gungrave G.O.R.E (Xbox and available on PC Game Pass)
- The Gunk (Xbox and available on PC Game Pass)
- Hotel: A Resort Simulator (New release on Steam, Oct. 12)
- Kill It With Fire (Xbox and available on PC Game Pass)
- Railway Empire 2 (Xbox and available on PC Game Pass)
- Rubber Bandits (Xbox, available on PC Game Pass)Saints Row IV (Xbox, available on Microsoft Store)
- Saltsea Chronicles (New release on Steam, Oct. 12)
- Soulstice (Epic Games Store)
- State of Decay 2: Juggernaut Edition (Steam, Epic Games Store, Xbox and available on PC Game Pass)
- Supraland Six Inches Under (Epic Games Store)
- Techtonica (Xbox and available on PC Game Pass)
- Teenage Mutant Ninja Turtles: Shredder’s Revenge (Xbox and available on PC Game Pass)
- Torchlight III (Xbox and available on PC Game Pass)
- Totally Accurate Battle Simulator (Xbox and available on PC Game Pass)
- Tribe: Primitive Builder (New release on Steam, Oct. 12)
- Trine 5: A Clockwork Conspiracy (Epic Games Store)
War Hospital didn’t make it in October due to a delay of its launch date. StalCraft and VEILED EXPERTS also didn’t make it in October due to technical issues. Stay tuned to GFN Thursday for more updates.
What are you looking forward to streaming this month? Let us know on Twitter or in the comments below.
Where is the most unusual place you’ve played a game on GFN?
—
NVIDIA GeForce NOW (@NVIDIAGFN) November 1, 2023
Accelerating Inference on x86-64 Machines with oneDNN Graph
Supported in PyTorch 2.0 as a beta feature, oneDNN Graph leverages aggressive fusion patterns to accelerate inference on x86-64 machines, especially Intel® Xeon® Scalable processors.
oneDNN Graph API extends oneDNN with a flexible graph API to maximize the optimization opportunity for generating efficient code on AI hardware. It automatically identifies the graph partitions to be accelerated via fusion. The fusion patterns focus on fusing compute-intensive operations such as convolution, matmul, and their neighbor operations for both inference and training use cases.
In PyTorch 2.0 and beyond, oneDNN Graph can help accelerate inference on x86-64 CPUs (primarily, Intel Xeon processor-based machines) with Float32 and BFloat16 (with PyTorch’s Automatic Mixed Precision support) datatypes. With BFloat16, speedup is limited to machines that support AVX512_BF16 ISA (Instruction Set Architecture), as well as machines that also support AMX_BF16 ISA.
oneDNN Graph Usage
From a user’s perspective, the usage is quite simple and intuitive, with the only change in code being an API invocation. To leverage oneDNN Graph with JIT-tracing, a model is profiled with an example input as shown below in Figure 1.
Fig. 1: A code-snippet that demonstrates using oneDNN Graph
oneDNN Graph receives the model’s graph and identifies candidates for operator-fusion with respect to the input shape of the example input. Currently, only static shapes are supported. This means that any other input shape would neither be supported nor receive any performance-benefit.
Measurements
To ensure reproducibility of results, we used a fork of TorchBench to measure inference speed-up of some Vision models on an AWS m7i.16xlarge instance, which uses 4th Gen Intel® Xeon® Scalable processors.
The baseline for comparison was torch.jit.optimize_for_inference which only supports Float32 datatype. The batch-size for each model was based on the respective batch size being used for them in TorchBench.
In Figure 2, we depict the inference speedup of using oneDNN Graph over PyTorch alone. The geomean speedup with oneDNN Graph for Float32 datatype was 1.24x, and the geomean speedup for BFloat16 datatype was 3.31x1.
Fig. 2: Inference speedup with oneDNN Graph over default CPU JIT Fuser (which only uses Float32 datatype)
Future work
oneDNN Graph is currently supported in PyTorch through TorchScript, but work is already underway by Intel to integrate it with the Inductor-CPU backend as a prototype feature in a future PyTorch release and Dynamo make supporting dynamic shapes easier with PyTorch, and we would like to introduce Dynamic shape support with Inductor-CPU. We also plan to add int8 quantization support.
Acknowledgements
The results presented in this blog are a joint effort between Meta and the Intel PyTorch team. Special thanks to Elias Ellison from Meta who spent precious time thoroughly reviewing the PRs and gave us helpful feedback.