Structured Query Language (SQL) is a complex language that requires an understanding of databases and metadata. Today, generative AI can enable people without SQL knowledge. This generative AI task is called text-to-SQL, which generates SQL queries from natural language processing (NLP) and converts text into semantically correct SQL. The solution in this post aims to bring enterprise analytics operations to the next level by shortening the path to your data using natural language.
With the emergence of large language models (LLMs), NLP-based SQL generation has undergone a significant transformation. Demonstrating exceptional performance, LLMs are now capable of generating accurate SQL queries from natural language descriptions. However, challenges still remain. First, human language is inherently ambiguous and context-dependent, whereas SQL is precise, mathematical, and structured. This gap may result in inaccurate conversion of the user’s needs into the SQL that’s generated. Second, you might need to build text-to-SQL features for every database because data is often not stored in a single target. You may have to recreate the capability for every database to enable users with NLP-based SQL generation. Third, despite the larger adoption of centralized analytics solutions like data lakes and warehouses, complexity rises with different table names and other metadata that is required to create the SQL for the desired sources. Therefore, collecting comprehensive and high-quality metadata also remains a challenge. To learn more about text-to-SQL best practices and design patterns, see Generating value from enterprise data: Best practices for Text2SQL and generative AI.
Our solution aims to address those challenges using Amazon Bedrock and AWS Analytics Services. We use Anthropic Claude v2.1 on Amazon Bedrock as our LLM. To address the challenges, our solution first incorporates the metadata of the data sources within the AWS Glue Data Catalog to increase the accuracy of the generated SQL query. The workflow also includes a final evaluation and correction loop, in case any SQL issues are identified by Amazon Athena, which is used downstream as the SQL engine. Athena also allows us to use a multitude of supported endpoints and connectors to cover a large set of data sources.
After we walk through the steps to build the solution, we present the results of some test scenarios with varying SQL complexity levels. Finally, we discuss how it is straightforward to incorporate different data sources to your SQL queries.
Solution overview
There are three critical components in our architecture: Retrieval Augmented Generation (RAG) with database metadata, a multi-step self-correction loop, and Athena as our SQL engine.
We use the RAG method to retrieve the table descriptions and schema descriptions (columns) from the AWS Glue metastore to ensure that the request is related to the right table and datasets. In our solution, we built the individual steps to run a RAG framework with the AWS Glue Data Catalog for demonstration purposes. However, you can also use knowledge bases in Amazon Bedrock to build RAG solutions quickly.
The multi-step component allows the LLM to correct the generated SQL query for accuracy. Here, the generated SQL is sent for syntax errors. We use Athena error messages to enrich our prompt for the LLM for more accurate and effective corrections in the generated SQL.
You can consider the error messages occasionally coming from Athena like feedback. The cost implications of an error correction step are negligible compared to the value delivered. You can even include these corrective steps as supervised reinforced learning examples to fine-tune your LLMs. However, we did not cover this flow in our post for simplicity purposes.
Note that there is always inherent risk of having inaccuracies, which naturally comes with generative AI solutions. Even if Athena error messages are highly effective to mitigate this risk, you can add more controls and views, such as human feedback or example queries for fine-tuning, to further minimize such risks.
Athena not only allows us to correct the SQL queries, but it also simplifies the overall problem for us because it serves as the hub, where the spokes are multiple data sources. Access management, SQL syntax, and more are all handled via Athena.
The following diagram illustrates the solution architecture.
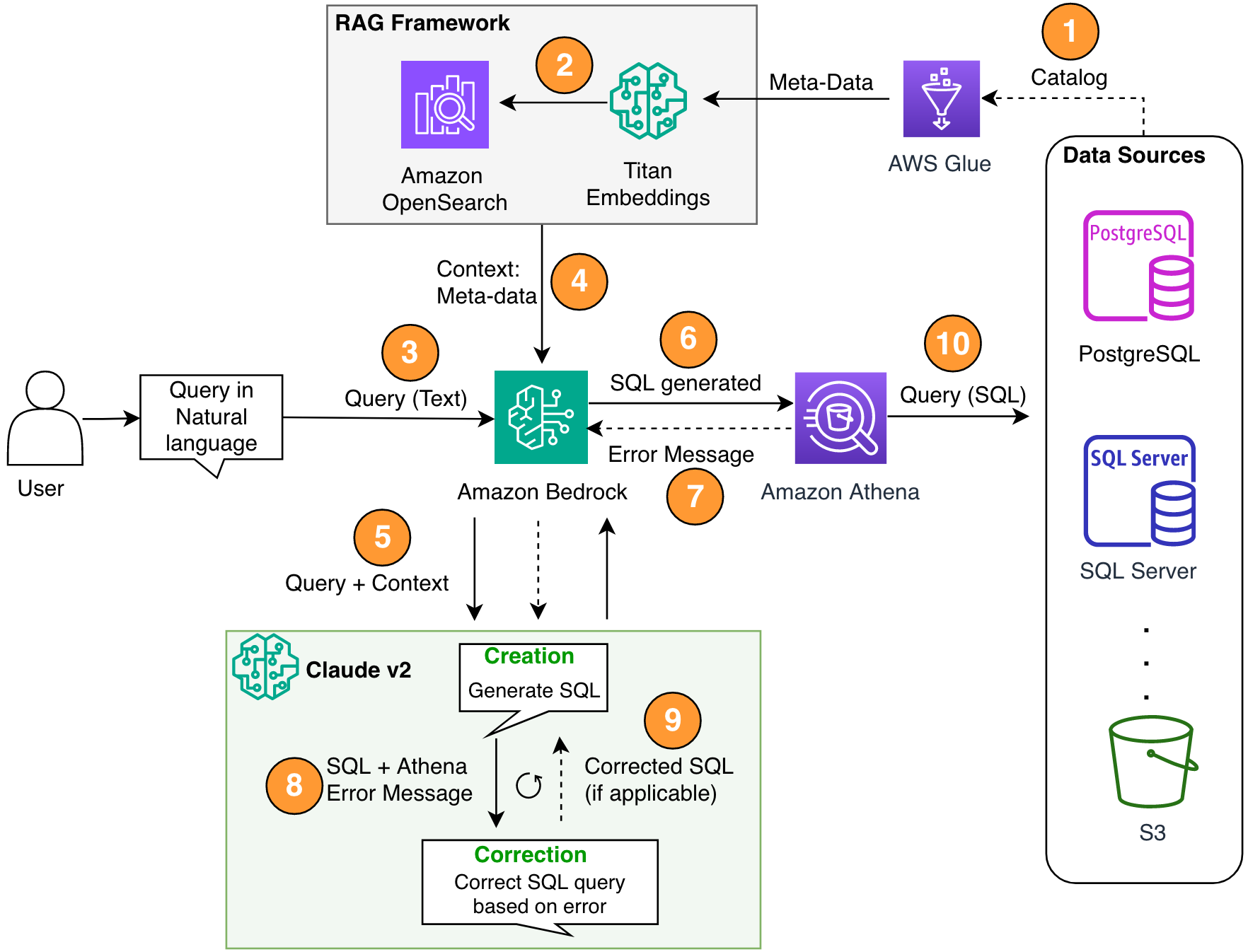
Figure 1. The solution architecture and process flow.
The process flow includes the following steps:
- Create the AWS Glue Data Catalog using an AWS Glue crawler (or a different method).
- Using the Titan-Text-Embeddings model on Amazon Bedrock, convert the metadata into embeddings and store it in an Amazon OpenSearch Serverless vector store, which serves as our knowledge base in our RAG framework.
At this stage, the process is ready to receive the query in natural language. Steps 7–9 represent a correction loop, if applicable.
- The user enters their query in natural language. You can use any web application to provide the chat UI. Therefore, we did not cover the UI details in our post.
- The solution applies a RAG framework via similarity search, which adds the extra context from the metadata from the vector database. This table is used for finding the correct table, database, and attributes.
- The query is merged with the context and sent to Anthropic Claude v2.1 on Amazon Bedrock.
- The model gets the generated SQL query and connects to Athena to validate the syntax.
- If Athena provides an error message that mentions the syntax is incorrect, the model uses the error text from Athena’s response.
- The new prompt adds Athena’s response.
- The model creates the corrected SQL and continues the process. This iteration can be performed multiple times.
- Finally, we run the SQL using Athena and generate output. Here, the output is presented to the user. For the sake of architectural simplicity, we did not show this step.
Prerequisites
For this post, you should complete the following prerequisites:
- Have an AWS account.
- Install the AWS Command Line Interface (AWS CLI).
- Set up the SDK for Python (Boto3).
- Create the AWS Glue Data Catalog using an AWS Glue crawler (or a different method).
- Using the Titan-Text-Embeddings model on Amazon Bedrock, convert the metadata into embeddings and store it in an OpenSearch Serverless vector store.
Implement the solution
You can use the following Jupyter notebook, which includes all the code snippets provided in this section, to build the solution. We recommend using Amazon SageMaker Studio to open this notebook with an ml.t3.medium instance with the Python 3 (Data Science) kernel. For instructions, refer to Train a Machine Learning Model. Complete the following steps to set up the solution:
- Create the knowledge base in OpenSearch Service for the RAG framework:
- Build the prompt (
final_question
) by combining the user input in natural language (user_query
), the relevant metadata from the vector store (vector_search_match
), and our instructions (details
): - Invoke Amazon Bedrock for the LLM (Claude v2) and prompt it to generate the SQL query. In the following code, it makes multiple attempts in order to illustrate the self-correction step:x
- If any issues are received with the generated SQL query (
{sqlgenerated}
) from the Athena response ({syntaxcheckmsg}
), the new prompt (prompt
) is generated based on the response and the model tries again to generate the new SQL: - After the SQL is generated, the Athena client is invoked to run and generate the output:
Test the solution
In this section, we run our solution with different example scenarios to test different complexity levels of SQL queries.
To test our text-to-SQL, we use two datasets available from IMDB. Subsets of IMDb data are available for personal and non-commercial use. You can download the datasets and store them in Amazon Simple Storage Service (Amazon S3). You can use the following Spark SQL snippet to create tables in AWS Glue. For this example, we use title_ratings
and title
:
Store data in Amazon S3 and metadata in AWS Glue
In this scenario, our dataset is stored in an S3 bucket. Athena has an S3 connector that allows you to use Amazon S3 as a data source that can be queried.
For our first query, we provide the input “I am new to this. Can you help me see all the tables and columns in imdb schema?”
The following is the generated query:
The following screenshot and code show our output.
For our second query, we ask “Show me all the title and details in US region whose rating is more than 9.5.”
The following is our generated query:
The response is as follows.
For our third query, we enter “Great Response! Now show me all the original type titles having ratings more than 7.5 and not in the US region.”
The following query is generated:
We get the following results.
Generate self-corrected SQL
This scenario simulates a SQL query that has syntax issues. Here, the generated SQL will be self-corrected based on the response from Athena. In the following response, Athena gave a COLUMN_NOT_FOUND
error and mentioned that table_description
can’t be resolved:
Using the solution with other data sources
To use the solution with other data sources, Athena handles the job for you. To do this, Athena uses data source connectors that can be used with federated queries. You can consider a connector as an extension of the Athena query engine. Pre-built Athena data source connectors exist for data sources like Amazon CloudWatch Logs, Amazon DynamoDB, Amazon DocumentDB (with MongoDB compatibility), and Amazon Relational Database Service (Amazon RDS), and JDBC-compliant relational data sources such MySQL, and PostgreSQL under the Apache 2.0 license. After you set up a connection to any data source, you can use the preceding code base to extend the solution. For more information, refer to Query any data source with Amazon Athena’s new federated query.
Clean up
To clean up the resources, you can start by cleaning up your S3 bucket where the data resides. Unless your application invokes Amazon Bedrock, it will not incur any cost. For the sake of infrastructure management best practices, we recommend deleting the resources created in this demonstration.
Conclusion
In this post, we presented a solution that allows you to use NLP to generate complex SQL queries with a variety of resources enabled by Athena. We also increased the accuracy of the generated SQL queries via a multi-step evaluation loop based on error messages from downstream processes. Additionally, we used the metadata in the AWS Glue Data Catalog to consider the table names asked in the query through the RAG framework. We then tested the solution in various realistic scenarios with different query complexity levels. Finally, we discussed how to apply this solution to different data sources supported by Athena.
Amazon Bedrock is at the center of this solution. Amazon Bedrock can help you build many generative AI applications. To get started with Amazon Bedrock, we recommend following the quick start in the following GitHub repo and familiarizing yourself with building generative AI applications. You can also try knowledge bases in Amazon Bedrock to build such RAG solutions quickly.
About the Authors
Sanjeeb Panda is a Data and ML engineer at Amazon. With the background in AI/ML, Data Science and Big Data, Sanjeeb design and develop innovative data and ML solutions that solve complex technical challenges and achieve strategic goals for global 3P sellers managing their businesses on Amazon. Outside of his work as a Data and ML engineer at Amazon, Sanjeeb Panda is an avid foodie and music enthusiast.
Burak Gozluklu is a Principal AI/ML Specialist Solutions Architect located in Boston, MA. He helps strategic customers adopt AWS technologies and specifically Generative AI solutions to achieve their business objectives. Burak has a PhD in Aerospace Engineering from METU, an MS in Systems Engineering, and a post-doc in system dynamics from MIT in Cambridge, MA. Burak is still a research affiliate in MIT. Burak is passionate about yoga and meditation.