Amazon Lex is a service for building conversational interfaces into any application. The new Amazon Lex V2 console and APIs make it easier to build, deploy, and manage bots. The Amazon Lex V2 console and APIs provide a simple information architecture in which the bot intents and slot types are scoped to a specific language. Versioning is performed at the bot level so that resources such as intents and slot types don’t have to be versioned individually. All the resources associated with the bot (language, intents, and slot types) are archived as part of the created bot version. This new methodology makes it easier to manage your bots. You can find more information in the Amazon Lex V2 API reference.
The Amazon Lex V2 console and APIs introduce a new method to build and interact with your bots. This post illustrates different methods to interact with a Amazon Lex V2 chatbot programmatically using the:
- AWS Command Line Interface, (AWS CLI),
- AWS SDK for Python (Boto3), and the
- AWS SDK for DotNet.
For this post, we use the MakeAppointment bot, which simulates the workflow of setting up an appointment with a dentist.
Obtain ID values for your bot
To get started, you must collect the following information:
- Your bot ID
- Your alias ID
- Your locale ID (language code)
Obtain your bot ID
You can find your bot ID on the Amazon Lex V2 console.
- On the Amazon Lex V2 console, on the Bots page, choose the bot you want to interact with.
For this post, we use MakeAppointmentBot
.
- Choose the bot and in the Bot details section, you can find the ID.
For this post, our ID is UFIDGBA6DE
.
Obtain your alias ID
To obtain your bot’s alias ID, complete the following steps:
- On the Amazon Lex V2 console, navigate to your bot.
- In the navigation pane, under Bot versions, under Deployment, choose Aliases.
- Choose your alias.
For this post, we use TestBotAlias
.
- In the Details section, you can find the alias ID.
For this post, our alias ID is TSTALIASID
.
Obtain your locale ID
Your locale ID indicates which language your bot uses.
- On the Amazon Lex V2 console, in the navigation pane, choose Bot versions.
- Choose the version of your bot you want to use.
For this post, we use the version called Draft version
.
- In the navigation pane, under your bot version, choose All languages.
You can see a list of the available languages for your chatbot.
- Choose your preferred language.
For this post, we use English (US), which is en_US
.
Interacting via the AWS CLI
The AWS Command Line Interface (CLI) is a unified tool to manage your AWS services. With just one tool to download and configure, you can control multiple AWS services from the command line and automate them through scripts.
To demonstrate the AWS CLI, you can use AWS CloudShell. AWS CloudShell is a browser-based shell that makes it easy to securely manage, explore, and interact with your AWS resources. CloudShell is pre-authenticated with your console credentials.
There are some cases where AWS CloudShell don’t have the latest version of the AWS CLI installed. If you receive an error “Invalid choice: ‘lexv2-runtime’”, follow the instructions contained in this guide to update the AWS CLI on AWS CloudShell.
Invalid choice: 'lexv2-runtime', maybe you meant:
* lex-runtime
[cloudshell-user@ip-10-1-148-180 ~]$ aws --version
aws-cli/2.1.16 Python/3.7.3 Linux/4.14.209-160.339.amzn2.x86_64 exec-env/CloudShell exe/x86_64.amzn.2 prompt/off
In my case after updating, I got the following version:
[cloudshell-user@ip-10-1-148-180 ~]$ aws --version
aws-cli/2.1.28 Python/3.8.8 Linux/4.14.209-160.339.amzn2.x86_64 exec-env/CloudShell exe/x86_64.amzn.2 prompt/off
And this time when I run the “aws lexv2-runtime” command it is recognized:
[cloudshell-user@ip-10-1-148-180 ~]$ aws lexv2-runtime
usage: aws [options] <command> <subcommand> [<subcommand> ...] [parameters]
To see help text, you can run:
aws help
aws <command> help
aws <command> <subcommand> help
aws: error: the following arguments are required: operation
After collecting the required information, enter your bot’s parameters (please keep in mind your IDs will be different):
bot-id='UFIDGBA6DE'
bot-alias-id='TSTALIASID'
localeId='en_US'
session-id='test-session'
To get started, you will submit the text “I would like to see the dentist” by using the following command:
aws lexv2-runtime recognize-text --bot-id 'UFIDGBA6DE' --bot-alias-id 'TSTALIASID' --locale-id 'en_US' --session-id 'test_session' --text 'I would like to see the dentist'
You will receive the following response:
{
"messages": [
{
"content": "What type of appointment would you like to schedule?",
"contentType": "PlainText"
}
],
"sessionState": {
"dialogAction": {
"type": "ElicitSlot",
"slotToElicit": "AppointmentType"
},
"intent": {
"name": "MakeAppointment",
"slots": {
"AppointmentType": null,
"Date": null,
"Time": null
},
"state": "InProgress",
"confirmationState": "None"
},
"originatingRequestId": "85f22c97-b5d3-4a74-9e3d-95446768ecaa"
},
"interpretations": [
{
"nluConfidence": {
"score": 0.84
},
"intent": {
"name": "MakeAppointment",
"slots": {
"AppointmentType": null,
"Date": null,
"Time": null
},
"state": "InProgress",
"confirmationState": "None"
}
},
{
"intent": {
"name": "FallbackIntent",
"slots": {}
}
}
],
"sessionId": "test_session"
}
Diving deep into the response you will notice the dialog state is ElicitSlot, or in other words, the chatbot is requiring the user to provide a value for the slot type ‘AppointmentType’.
"sessionState": {
"dialogAction": {
"type": "ElicitSlot",
"slotToElicit": "AppointmentType"
}
Also, you will notice the messages:
"messages": [
{
"content": "What type of appointment would you like to schedule?",
"contentType": "PlainText"
}
In this case you will state “Root canal” as the appointment type:
aws lexv2-runtime recognize-text --bot-id 'UFIDGBA6DE' --bot-alias-id 'TSTALIASID' --locale-id 'en_US' --session-id 'test_session' --text 'Root canal'
The response you will receive will look similar to this:
{
"messages": [
{
"content": "When should I schedule your appointment?",
"contentType": "PlainText"
}
],
"sessionState": {
"dialogAction": {
"type": "ElicitSlot",
"slotToElicit": "Date"
},
"intent": {
"name": "MakeAppointment",
"slots": {
"AppointmentType": {
"value": {
"originalValue": "Root canal",
"interpretedValue": "Root canal",
"resolvedValues": [
"root canal"
]
}
},
"Date": null,
"Time": null
},
"state": "InProgress",
"confirmationState": "None"
},
"originatingRequestId": "85f22c97-b5d3-4a74-9e3d-95446768ecaa"
},
"interpretations": [
{
"nluConfidence": {
"score": 1.0
},
"intent": {
"name": "MakeAppointment",
"slots": {
"AppointmentType": {
"value": {
"originalValue": "Root canal",
"interpretedValue": "Root canal",
"resolvedValues": [
"root canal"
]
}
},
"Date": null,
"Time": null
},
"state": "InProgress",
"confirmationState": "None"
}
},
{
"intent": {
"name": "FallbackIntent",
"slots": {}
}
}
],
"sessionId": "test_session"
}
Check the current dialogAction:
"sessionState": {
"dialogAction": {
"type": "ElicitSlot",
"slotToElicit": "Date"
}
Also, the messages:
"messages": [
{
"content": "When should I schedule your appointment?",
"contentType": "PlainText"
}
This time you will provide the pickup date:
aws lexv2-runtime recognize-text --bot-id 'UFIDGBA6DE' --bot-alias-id 'TSTALIASID' --locale-id 'en_US' --session-id 'test_session' --text 'Next Wednesday'
This time the response will look similar to this:
{
"messages": [
{
"content": "At what time should I schedule your appointment?",
"contentType": "PlainText"
}
],
"sessionState": {
"dialogAction": {
"type": "ElicitSlot",
"slotToElicit": "Time"
},
"intent": {
"name": "MakeAppointment",
"slots": {
"AppointmentType": {
"value": {
"originalValue": "Root canal",
"interpretedValue": "Root canal",
"resolvedValues": [
"root canal"
]
}
},
"Date": {
"value": {
"originalValue": "Next Wednesday",
"interpretedValue": "2021-03-17",
"resolvedValues": [
"2021-03-17"
]
}
},
"Time": null
},
"state": "InProgress",
"confirmationState": "None"
},
"originatingRequestId": "85f22c97-b5d3-4a74-9e3d-95446768ecaa"
},
"interpretations": [
{
"nluConfidence": {
"score": 1.0
},
"intent": {
"name": "MakeAppointment",
"slots": {
"AppointmentType": {
"value": {
"originalValue": "Root canal",
"interpretedValue": "Root canal",
"resolvedValues": [
"root canal"
]
}
},
"Date": {
"value": {
"originalValue": "Next Wednesday",
"interpretedValue": "2021-03-17",
"resolvedValues": [
"2021-03-17"
]
}
},
"Time": null
},
"state": "InProgress",
"confirmationState": "None"
}
},
{
"intent": {
"name": "FallbackIntent",
"slots": {}
}
}
],
"sessionId": "test_session"
}
At time of writing this blog “next Wednesday” is March 17, 2021, which is what your chatbot interpreted:
chatbot interpreted:
"Date": {
"value": {
"originalValue": "Next Wednesday",
"interpretedValue": "2021-03-17",
"resolvedValues": [
"2021-03-17"
]
}
And the messages:
"messages": [
{
"content": "At what time should I schedule your appointment?",
"contentType": "PlainText"
}
Next step is to define the Time:
aws lexv2-runtime recognize-text --bot-id 'UFIDGBA6DE' --bot-alias-id 'TSTALIASID' --locale-id 'en_US' --session-id 'test_session' --text '9 am'
The response will be similar to this:
{
"messages": [
{
"content": "09:00 is available, should I go ahead and book your appointment?",
"contentType": "PlainText"
}
],
"sessionState": {
"dialogAction": {
"type": "ConfirmIntent"
},
"intent": {
"name": "MakeAppointment",
"slots": {
"AppointmentType": {
"value": {
"originalValue": "Root canal",
"interpretedValue": "Root canal",
"resolvedValues": [
"root canal"
]
}
},
"Date": {
"value": {
"originalValue": "Next Wednesday",
"interpretedValue": "2021-03-17",
"resolvedValues": [
"2021-03-17"
]
}
},
"Time": {
"value": {
"originalValue": "9 am",
"interpretedValue": "09:00",
"resolvedValues": [
"09:00"
]
}
}
},
"state": "InProgress",
"confirmationState": "None"
},
"originatingRequestId": "85f22c97-b5d3-4a74-9e3d-95446768ecaa"
},
"interpretations": [
{
"nluConfidence": {
"score": 1.0
},
"intent": {
"name": "MakeAppointment",
"slots": {
"AppointmentType": {
"value": {
"originalValue": "Root canal",
"interpretedValue": "Root canal",
"resolvedValues": [
"root canal"
]
}
},
"Date": {
"value": {
"originalValue": "Next Wednesday",
"interpretedValue": "2021-03-17",
"resolvedValues": [
"2021-03-17"
]
}
},
"Time": {
"value": {
"originalValue": "9 am",
"interpretedValue": "09:00",
"resolvedValues": [
"09:00"
]
}
}
},
"state": "InProgress",
"confirmationState": "None"
}
},
{
"intent": {
"name": "FallbackIntent",
"slots": {}
}
}
],
"sessionId": "test_session"
}
This time your chatbot interpreted the value as 09:00 and used it as the value for the slot Time:
"Time": {
"value": {
"originalValue": "9 am",
"interpretedValue": "09:00",
"resolvedValues": [
"09:00"
]
}
}
Because the slots have been set now, the sessionState dialogAction is ConfirmIntent.
'sessionState': {
'dialogAction': {
'type': 'ConfirmIntent'
}
This time, messages contains the details contained in the slots.
"messages": [
{
"content": "09:00 is available, should I go ahead and book your appointment?",
"contentType": "PlainText"
}
]
You will submit the confirmation:
aws lexv2-runtime recognize-text --bot-id 'UFIDGBA6DE' --bot-alias-id 'TSTALIASID' --locale-id 'en_US' --session-id 'test_session' --text 'yes'
Response will be similar to:
{
"sessionState": {
"dialogAction": {
"type": "Close"
},
"intent": {
"name": "MakeAppointment",
"slots": {
"AppointmentType": {
"value": {
"originalValue": "Root canal",
"interpretedValue": "Root canal",
"resolvedValues": [
"root canal"
]
}
},
"Date": {
"value": {
"originalValue": "Next Wednesday",
"interpretedValue": "2021-03-17",
"resolvedValues": [
"2021-03-17"
]
}
},
"Time": {
"value": {
"originalValue": "9 am",
"interpretedValue": "09:00",
"resolvedValues": [
"09:00"
]
}
}
},
"state": "ReadyForFulfillment",
"confirmationState": "Confirmed"
},
"originatingRequestId": "85f22c97-b5d3-4a74-9e3d-95446768ecaa"
},
"interpretations": [
{
"nluConfidence": {
"score": 1.0
},
"intent": {
"name": "MakeAppointment",
"slots": {
"AppointmentType": {
"value": {
"originalValue": "Root canal",
"interpretedValue": "Root canal",
"resolvedValues": [
"root canal"
]
}
},
"Date": {
"value": {
"originalValue": "Next Wednesday",
"interpretedValue": "2021-03-17",
"resolvedValues": [
"2021-03-17"
]
}
},
"Time": {
"value": {
"originalValue": "9 am",
"interpretedValue": "09:00",
"resolvedValues": [
"09:00"
]
}
}
},
"state": "ReadyForFulfillment",
"confirmationState": "Confirmed"
}
},
{
"intent": {
"name": "FallbackIntent",
"slots": {}
}
}
],
"sessionId": "test_session"
}
Also the intent sate is “ReadyForFulfillment” and “Confirmed”
'state': 'ReadyForFulfillment',
'confirmationState': 'Confirmed'
Interact programmatically with Amazon Lex V2 via Boto3
Boto is the AWS SDK for Python. It enables Python developers to create, configure, and manage AWS services, such as Amazon Elastic Compute Cloud (Amazon EC2) and Amazon Simple Storage Service (Amazon S3). Boto provides an easy-to-use, object-oriented API, as well as low-level access to AWS services.
Support for Amazon Lex V2 APIs is introduced in version 1.16.59.
To interact with your Amazon Lex bot using the Boto3 lexv2-runtime
, you need to use the values that you collected earlier for botID
, AliasId
, and localeID
.
Boto3 code example
After I collected the required information, I have the following parameters (these will be different for your account):
botId='UFIDGBA6DE'
botAliasId='TSTALIASID'
localeId='en_US'
The first message you send to your bot is “I would like to see the dentist “:
import boto3
# LexV2 client uses 'lexv2-runtime'
client = boto3.client('lexv2-runtime')
# Submit the text 'I would like to see a dentist'
response = client.recognize_text(
botId='UFIDGBA6DE',
botAliasId='TSTALIASID',
localeId='en_US',
sessionId="test_session",
text='I would like to see the dentist')
You get a response similar to the following:
{
'ResponseMetadata': {
'RequestId': 'e998ce7f-3381-4501-9973-970b221504a5',
'HTTPStatusCode': 200,
'HTTPHeaders': {
'x-amzn-requestid': 'e998ce7f-3381-4501-9973-970b221504a5',
'date': 'Tue,
9Mar202123: 38: 01GMT',
'content-type': 'application/json',
'content-length': '666'
},
'RetryAttempts': 0
},
'messages': [
{
'content': 'What type of appointment would you like to schedule?',
'contentType': 'PlainText'
}
],
'sessionState': {
'dialogAction': {
'type': 'ElicitSlot',
'slotToElicit': 'AppointmentType'
},
'intent': {
'name': 'MakeAppointment',
'slots': {
'AppointmentType': None,
'Date': None,
'Time': None
},
'state': 'InProgress',
'confirmationState': 'None'
},
'originatingRequestId': '85f22c97-b5d3-4a74-9e3d-95446768ecaa'
},
'interpretations': [
{
'nluConfidence': {
'score': 0.82
},
'intent': {
'name': 'MakeAppointment',
'slots': {
'AppointmentType': None,
'Date': None,
'Time': None
},
'state': 'InProgress',
'confirmationState': 'None'
}
},
{
'intent': {
'name': 'FallbackIntent',
'slots': {
}
}
}
],
'sessionId': 'test_session'
}
We can dive deeper into the response to see the dialog state is ElicitSlot
, meaning the chatbot is requiring the user to provide a value for the slot type AppointmentType
:
'sessionState': {
'dialogAction': {
'type': 'ElicitSlot',
'slotToElicit': 'AppointmentType'
}
In this case, you enter Root Canal
:
response = client.recognize_text(
botId='UFIDGBA6DE',
botAliasId='TSTALIASID',
localeId='en_US',
sessionId="test_session",
text='Root Canal')
This time, your response is the following:
{
'ResponseMetadata': {
'RequestId': 'fae988d3-c956-4ea4-b5f8-2646d914adf4',
'HTTPStatusCode': 200,
'HTTPHeaders': {
'x-amzn-requestid': 'fae988d3-c956-4ea4-b5f8-2646d914adf4',
'date': 'Tue,
9Mar202123: 40: 03GMT',
'content-type': 'application/json',
'content-length': '842'
},
'RetryAttempts': 0
},
'messages': [
{
'content': 'When should I schedule your appointment?',
'contentType': 'PlainText'
}
],
'sessionState': {
'dialogAction': {
'type': 'ElicitSlot',
'slotToElicit': 'Date'
},
'intent': {
'name': 'MakeAppointment',
'slots': {
'AppointmentType': {
'value': {
'originalValue': 'RootCanal',
'interpretedValue': 'RootCanal',
'resolvedValues': [
'rootcanal'
]
}
},
'Date': None,
'Time': None
},
'state': 'InProgress',
'confirmationState': 'None'
},
'originatingRequestId': '85f22c97-b5d3-4a74-9e3d-95446768ecaa'
},
'interpretations': [
{
'nluConfidence': {
'score': 1.0
},
'intent': {
'name': 'MakeAppointment',
'slots': {
'AppointmentType': {
'value': {
'originalValue': 'RootCanal',
'interpretedValue': 'RootCanal',
'resolvedValues': [
'rootcanal'
]
}
},
'Date': None,
'Time': None
},
'state': 'InProgress',
'confirmationState': 'None'
}
},
{
'intent': {
'name': 'FallbackIntent',
'slots': {
}
}
}
],
'sessionId': 'test_session'
}
The chatbot interpreted that the value for the slot AppointmentType
is root canal
:
'slots': {
'AppointmentType': {
'value': {
'originalValue': 'Root Canal',
'interpretedValue': 'Root Canal',
'resolvedValues': [
'root canal'
]
}
},
'Date': None,
'Time': None
}
You can also check the current dialogAction
:
'sessionState': {
'dialogAction': {
'type': 'ElicitSlot',
'slotToElicit': 'Date'
}
In the messages
section, you can see the following:
'messages': [
{
'content': 'When should I schedule your appointment?',
'contentType': 'PlainText'
}
]
Provide appointment date:
response = client.recognize_text(
botId='UFIDGBA6DE',
botAliasId='TSTALIASID',
localeId='en_US',
sessionId="test_session",
text='Next Wednesday')
You get a response similar to the following:
{
'ResponseMetadata': {
'RequestId': '8639e4dd-bbfe-42a3-aa9e-96391fd821fd',
'HTTPStatusCode': 200,
'HTTPHeaders': {
'x-amzn-requestid': '8639e4dd-bbfe-42a3-aa9e-96391fd821fd',
'date': 'Tue,
9Mar202123: 41: 51GMT',
'content-type': 'application/json',
'content-length': '1058'
},
'RetryAttempts': 0
},
'messages': [
{
'content': 'At what time should I schedule your appointment?',
'contentType': 'PlainText'
}
],
'sessionState': {
'dialogAction': {
'type': 'ElicitSlot',
'slotToElicit': 'Time'
},
'intent': {
'name': 'MakeAppointment',
'slots': {
'AppointmentType': {
'value': {
'originalValue': 'Root Canal',
'interpretedValue': 'Root Canal',
'resolvedValues': [
'root canal'
]
}
},
'Date': {
'value': {
'originalValue': 'Next Wednesday',
'interpretedValue': '2021-03-17',
'resolvedValues': [
'2021-03-17'
]
}
},
'Time': None
},
'state': 'InProgress',
'confirmationState': 'None'
},
'originatingRequestId': '85f22c97-b5d3-4a74-9e3d-95446768ecaa'
},
'interpretations': [
{
'nluConfidence': {
'score': 1.0
},
'intent': {
'name': 'MakeAppointment',
'slots': {
'AppointmentType': {
'value': {
'originalValue': 'RootCanal',
'interpretedValue': 'RootCanal',
'resolvedValues': [
'rootcanal'
]
}
},
'Date': {
'value': {
'originalValue': 'Next Wednesday',
'interpretedValue': '2021-03-17',
'resolvedValues': [
'2021-03-17'
]
}
},
'Time': None
},
'state': 'InProgress',
'confirmationState': 'None'
}
},
{
'intent': {
'name': 'FallbackIntent',
'slots': {
}
}
}
],
'sessionId': 'test_session'
}
At the time of this writing, “next Wednesday” is March 17, 2021, which is exactly what your chatbot interpreted:
'Date': {
'value': {
'originalValue': 'Next Wednesday',
'interpretedValue': '2021-03-17',
'resolvedValues': [
'2021-03-17'
]
}
You can see the following in the messages
section:
'messages': [
{
'content': 'At what time should I schedule your appointment?',
'contentType': 'PlainText'
}
]
The next step is to define the PickupTime
:
'sessionState': {
'dialogAction': {
'type': 'ElicitSlot',
'slotToElicit': 'Time'
}
Use the following code:
response = client.recognize_text(
botId='UFIDGBA6DE',
botAliasId='TSTALIASID',
localeId='en_US',
sessionId="test_session",
text='9 am')
You receive a response similar to the following:
{
'ResponseMetadata': {
'RequestId': 'e8c06004-960b-442f-bf0d-0d995ec366bf',
'HTTPStatusCode': 200,
'HTTPHeaders': {
'x-amzn-requestid': 'e8c06004-960b-442f-bf0d-0d995ec366bf',
'date': 'Tue,
9Mar202123: 44: 39GMT',
'content-type': 'application/json',
'content-length': '1223'
},
'RetryAttempts': 0
},
'messages': [
{
'content': '09:00 is available, should I go ahead and book your appointment?',
'contentType': 'PlainText'
}
],
'sessionState': {
'dialogAction': {
'type': 'ConfirmIntent'
},
'intent': {
'name': 'MakeAppointment',
'slots': {
'AppointmentType': {
'value': {
'originalValue': 'Root Canal',
'interpretedValue': 'Root Canal',
'resolvedValues': [
'root canal'
]
}
},
'Date': {
'value': {
'originalValue': 'Next Wednesday',
'interpretedValue': '2021-03-17',
'resolvedValues': [
'2021-03-17'
]
}
},
'Time': {
'value': {
'originalValue': '9 am',
'interpretedValue': '09:00',
'resolvedValues': [
'09:00'
]
}
}
},
'state': 'InProgress',
'confirmationState': 'None'
},
'originatingRequestId': '85f22c97-b5d3-4a74-9e3d-95446768ecaa'
},
'interpretations': [
{
'nluConfidence': {
'score': 1.0
},
'intent': {
'name': 'MakeAppointment',
'slots': {
'AppointmentType': {
'value': {
'originalValue': 'Root Canal',
'interpretedValue': 'Root Canal',
'resolvedValues': [
'rootcanal'
]
}
},
'Date': {
'value': {
'originalValue': 'Next Wednesday',
'interpretedValue': '2021-03-17',
'resolvedValues': [
'2021-03-17'
]
}
},
'Time': {
'value': {
'originalValue': '9 am',
'interpretedValue': '09:00',
'resolvedValues': [
'09:00'
]
}
}
},
'state': 'InProgress',
'confirmationState': 'None'
}
},
{
'intent': {
'name': 'FallbackIntent',
'slots': {
}
}
}
],
'sessionId': 'test_session'
}
The chatbot set your appointment Time
for 9:00 AM:
'Time': {
'value': {
'originalValue': '9 am',
'interpretedValue': '09:00',
'resolvedValues': [
'09:00'
]
}
Because the slots have been set, the sessionState
dialogAction
is now ConfirmIntent
:
'sessionState': {
'dialogAction': {
'type': 'ConfirmIntent'
}
This time, the messages
section contains the details contained in the slots:
'messages': [
{
'content': '09:00 is available, should I go ahead and book your appointment?',
'contentType': 'PlainText'
}
You submit the confirmation:
response = client.recognize_text(
botId='4HKK09CVHD',
botAliasId='TSTALIASID',
localeId='en_US',
sessionId="test_session",
text='yes')
The response looks like the following:
{
'ResponseMetadata': {
'RequestId': '362d4478-caea-49cf-8b1b-b0ab035e324f',
'HTTPStatusCode': 200,
'HTTPHeaders': {
'x-amzn-requestid': '362d4478-caea-49cf-8b1b-b0ab035e324f',
'date': 'Tue,
9Mar202123: 46: 48GMT',
'content-type': 'application/json',
'content-length': '1125'
},
'RetryAttempts': 0
},
'sessionState': {
'dialogAction': {
'type': 'Close'
},
'intent': {
'name': 'MakeAppointment',
'slots': {
'AppointmentType': {
'value': {
'originalValue': 'Root Canal',
'interpretedValue': 'Root Canal',
'resolvedValues': [
'root canal'
]
}
},
'Date': {
'value': {
'originalValue': 'Next Wednesday',
'interpretedValue': '2021-03-17',
'resolvedValues': [
'2021-03-17'
]
}
},
'Time': {
'value': {
'originalValue': '9 am',
'interpretedValue': '09:00',
'resolvedValues': [
'09:00'
]
}
}
},
'state': 'ReadyForFulfillment',
'confirmationState': 'Confirmed'
},
'originatingRequestId': '85f22c97-b5d3-4a74-9e3d-95446768ecaa'
},
'interpretations': [
{
'nluConfidence': {
'score': 1.0
},
'intent': {
'name': 'MakeAppointment',
'slots': {
'AppointmentType': {
'value': {
'originalValue': 'Root Canal',
'interpretedValue': 'Root Canal',
'resolvedValues': [
'root canal'
]
}
},
'Date': {
'value': {
'originalValue': 'Next Wednesday',
'interpretedValue': '2021-03-17',
'resolvedValues': [
'2021-03-17'
]
}
},
'Time': {
'value': {
'originalValue': '9 am',
'interpretedValue': '09:00',
'resolvedValues': [
'09:00'
]
}
}
},
'state': 'ReadyForFulfillment',
'confirmationState': 'Confirmed'
}
},
{
'intent': {
'name': 'FallbackIntent',
'slots': {
}
}
}
],
'sessionId': 'test_session'
}
The sessionState
dialogAction
this time is Close
:
'sessionState': {
'dialogAction': {
'type': 'Close'
}
Also, the intent state is ReadyForFulfillment
and Confirmed
:
'state': 'ReadyForFulfillment',
'confirmationState': 'Confirmed'
About DotNet SDK
The AWS SDK for DotNet provides an interface that you can use from your DotNet applications to interact with your bots. You can use the SDK for DotNet to build client applications for users.
The following application interacts with the . It uses the AmazonLexRuntimeV2Client
from the SDK for DotNet to call the RecognizeText operation to conduct a conversation with the bot.
To run the application, you need the following values:
- botId –The identifier assigned to the bot when you created it. You can see the bot ID on the Amazon Lex console on the Bot details
- botAliasId – The identifier assigned to the bot alias when you created it. You can see the bot alias ID on the Amazon Lex console on the Aliases If you can’t see the alias ID in the list, choose the gear icon on the upper right and turn on Alias ID.
- localeId – The identifier of the locale that you used for your bot. For a list of locales and language codes, see Languages and locales supported by Amazon Lex.
- accessKey and secretKey – The authentication keys for your account. If you don’t have a set of keys, create them using the AWS Identity and Access Management (IAM) console.
- sessionId – An identifier for the session with the Amazon Lex bot. In this case, the code uses a random UUID.
Additionally, if your bot isn’t in the US East (N. Virginia) AWS Region, make sure that you change the region
value.
Let’s look at the following sample code to understand how we can easily interact with Amazon Lex V2 from the DotNet framework.
First, we create an AmazonLexRuntimeV2Client
object with the access key and secret:
// create a LexV2 runtime client
AmazonLexRuntimeV2Client client =
new AmazonLexRuntimeV2Client(access_key
, secret_key
, Amazon.RegionEndpoint.GetBySystemName(preferred_region));
Provide the following information:
// provide the parameters
// Randomly generated GUID unique per session
string sessionId = Guid.NewGuid().ToString();
// provide the unique BotId
string botId = "XXXXX";
//provide the unique BotAliasId
string botAliasId = "XXXXX";
// refer to the list of supported locales
string localeId = "en_US";
The request uses the required URI parameters botAliasId
, botId
, localeId
, and sessionId
.
The user initiates the conversation by providing the intent:
string userInput1 = ""
Next, we call the GetRecongizeTextV2Response
method by passing the required parameters and the AmazonLexRuntimeV2Client
client object:
var response1 = GetRecongizeTextV2Response(client, botId, botAliasId, localeId, sessionId, userInput1)
Inside the GetRecongizeTextV2Response
method, we create a RecognizeTextRequest
object, containing the parameters to the RecognizeText
operation:
RecognizeTextRequest request = new RecognizeTextRequest
{
BotAliasId = botAliasId,
BotId = botId,
LocaleId = localeId,
SessionId = sessionId,
Text = userInput
};
The RecognizeTextRequest
in JSON format looks like the following:
{
"BotAliasId": "YOURBOTALIASID",
"BotId": "YOURBOTID",
"LocaleId": "en_US",
"RequestAttributes": {
},
"SessionId": "cb447544-1920-432d-aa41-4ff2237a0a62",
"SessionStateValue": null,
"Text": "I would like to see the dentist"
}
Then we send the user input to Amazon Lex. Client applications can use this API to send requests to Amazon Lex at runtime. You can send requests synchronously or asynchronously. For this post, we send the request using the RecognizeTextAsync
method asynchronously. Amazon Lex interprets the user input using the machine learning model that it built for the bot. See the following code:
response = client.RecognizeTextAsync(request).GetAwaiter().GetResult();
In response, Amazon Lex returns the next message to convey to the user and an optional response card to display:
{
"Interpretations": [
{
"Intent": {
"ConfirmationState": {
"Value": "None"
},
"Name": "MakeAppointment",
"Slots": {
"AppointmentType": null,
"Date": null,
"Time": null
},
"State": {
"Value": "InProgress"
}
},
"NluConfidence": {
"Score": 0.84
},
"SentimentResponse": null
},
{
"Intent": {
"ConfirmationState": null,
"Name": "FallbackIntent",
"Slots": {
},
"State": null
},
"NluConfidence": null,
"SentimentResponse": null
}
],
"Messages": [
{
"Content": "What type of appointment would you like to schedule?",
"ContentType": {
"Value": "PlainText"
},
"ImageResponseCard": null
}
],
"RequestAttributes": {
},
"SessionId": "cb447544-1920-432d-aa41-4ff2237a0a62",
"SessionStateValue": {
"ActiveContexts": [
],
"DialogAction": {
"SlotToElicit": "AppointmentType",
"Type": {
"Value": "ElicitSlot"
}
},
"Intent": {
"ConfirmationState": {
"Value": "None"
},
"Name": "MakeAppointment",
"Slots": {
"AppointmentType": null,
"Date": null,
"Time": null
},
"State": {
"Value": "InProgress"
}
},
"OriginatingRequestId": "36e1cb54-1861-45c9-8d67-898e632daf31",
"SessionAttributes": {
}
},
"ResponseMetadata": {
"RequestId": "36e1cb54-1861-45c9-8d67-898e632daf31",
"Metadata": {
}
},
"ContentLength": 690,
"HttpStatusCode": 200
}
As you can see, Amazon Lex returns the next message inside the Content
as a plain text, with HttpStatusCode
as 200
.
DotNet SDK code example
The following is the full source code:
using System;
using Amazon.LexModelsV2;
using Amazon.LexRuntimeV2;
using Amazon.LexRuntimeV2.Model;
// This is a sample application to interact with a bot using RecognizeText API using DotNet.
namespace OrderFlowerV2
{
class OrderFlowers
{
const string access_key = "XXXXXXXXXX"; // provide you access key
const string secret_key = "XXXXXXXXXX"; // provide the secret
const string preferred_region = "XXXXX"; // provide an appropriate region
static void Main(string[] args)
{
// create a LexV2 runtime client
AmazonLexRuntimeV2Client client = new AmazonLexRuntimeV2Client(access_key,
secret_key, Amazon.RegionEndpoint.GetBySystemName(preferred_region));
string sessionId = Guid.NewGuid().ToString(); // Randomly generated GUID unique per session.
string botId = "XXXXX"; // provide the unique BotId
string botAliasId = "XXXXX"; //provide the unique BotAliasId
string localeId = "en_US"; // refer to the list of supported locales
// utterance 1
string userInput1 = "I would like to order flowers";
var response1 = GetRecongizeTextV2Response(client
, botId, botAliasId, localeId, sessionId, userInput1);
Console.WriteLine("User - "+userInput1);
foreach (var message in response1.Messages)
{
Console.WriteLine("Bot - "+message.Content);
}
// utterance 2
string userInput2 = "1 rose";
var response2 = GetRecongizeTextV2Response(client
, botId, botAliasId, localeId, sessionId, userInput2);
Console.WriteLine("User - "+userInput2);
foreach (var message in response2.Messages)
{
Console.WriteLine("Bot - "+message.Content);
}
// utterance 3
string userInput3 = "next Monday";
var response3 = GetRecongizeTextV2Response(client, botId, botAliasId, localeId, sessionId, userInput3);
Console.WriteLine("User - "+userInput3);
foreach (var message in response3.Messages)
{
Console.WriteLine("Bot - "+message.Content);
}
// utterance 4
string userInput4 = "7 in the evening";
var response4 = GetRecongizeTextV2Response(client, botId, botAliasId, localeId, sessionId, userInput4);
Console.WriteLine("User - "+userInput4);
foreach (var message in response4.Messages)
{
Console.WriteLine("Bot - "+message.Content);
}
// utterance 5
string userInput5 = "Yes";
var response5 = GetRecongizeTextV2Response(client, botId, botAliasId, localeId, sessionId, userInput5);
Console.WriteLine("User - "+userInput5);
foreach (var message in response5.Messages)
{
Console.WriteLine("Bot - "+message.Content);
}
}
/// <summary>
/// Call Amazon LexV2 RecognizeTextAsync method to get the response from the Bot
/// </summary>
/// <param name="client"></param>
/// <param name="botId"></param>
/// <param name="botAliasId"></param>
/// <param name="localeId"></param>
/// <param name="sessionId"></param>
/// <param name="userInput"></param>
/// <returns></returns>
private static RecognizeTextResponse GetRecongizeTextV2Response(AmazonLexRuntimeV2Client client, string botId, string botAliasId, string localeId, string sessionId, string userInput)
{
// create a RecognizeTextResponse object
RecognizeTextResponse response = new RecognizeTextResponse();
try
{
// create RecognizeTextRequest object with the required parameters
RecognizeTextRequest request = new RecognizeTextRequest
{
BotAliasId = botAliasId,
BotId = botId,
LocaleId = localeId,
SessionId = sessionId,
Text = userInput
};
// call the RecognizeText async method to get the response
response = client.RecognizeTextAsync(request).GetAwaiter().GetResult();
}catch(AmazonLexModelsV2Exception exception)
{
Console.WriteLine("AmazonLexModelsV2Exception - "+exception.Message);
}
catch(AmazonLexRuntimeV2Exception exception)
{
Console.WriteLine("AmazonLexRuntimeV2Exception - "+exception.Message);
}
catch(Exception exception)
{
Console.WriteLine("Exception - "+exception.Message);
}
return response;
}
}
}
Conclusion
Now that you have learned how to interact with your Amazon Lex v2 chatbot programmatically with the AWS CLI, Boto3, and, the AWS SDK for DotNet, you can integrate your bots with your applications or with different platforms. If you want to learn more about Amazon Lex v2, you can explore building a banking bot with support for English and Spanish. You can also dive deep into Amazon Lex through the Developer Guide.
About the Authors
Juan Bustos is an AI Services Specialist Solutions Architect at Amazon Web Services, based in Dallas, TX. Outside of work, he loves spending time writing and playing music as well as trying random restaurants with his family.
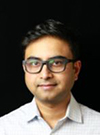
Esther Lee is a Product Manager for AWS Language AI Services. She is passionate about the intersection of technology and education. Out of the office, Esther enjoys long walks along the beach, dinners with friends and friendly rounds of Mahjong.