This is a guest post by Mark Wood at Pulselive. In their own words, “Pulselive, based out of the UK, is the proud digital partner to some of the biggest names in sports.”
At Pulselive, we create experiences sports fans can’t live without; whether that’s the official Cricket World Cup website or the English Premier League’s iOS and Android apps.
One of the key things our customers measure us on is fan engagement with digital content such as videos. But until recently, the videos each fan saw were based on a most recently published list, which wasn’t personalized.
Sports organizations are trying to understand who their fans are and what they want. The wealth of digital behavioral data that can be collected for each fan tells a story of how unique they are and how they engage with our content. Based on the increase of available data and the increasing presence of machine learning (ML), Pulselive was asked by customers to provide tailored content recommendations.
In this post, we share our experience of adding Amazon Personalize to our platform as our new recommendation engine and how we increased video consumption by 20%.
Implementing Amazon Personalize
Before we could start, Pulselive had two main challenges: we didn’t have any data scientists on staff and we needed to find a solution that our engineers with minimal ML experience would understand and would still produce measurable results. We considered using external companies to assist (expensive), using tools such as Amazon SageMaker (still quite the learning curve), or Amazon Personalize.
We ultimately chose to use Amazon Personalize for several reasons:
- The barrier to entry was low, both technically and financially.
- We could quickly conduct an A/B test to demonstrate the value of a recommendation engine.
- We could create a simple proof of concept (PoC) with minimal disruption to the existing site.
- We were more concerned about the impact and improving the results than having a clear understanding of what was going on under the hood of Amazon Personalize.
Like any other business, we couldn’t afford to have an adverse impact on our daily operations, but still needed the confidence that the solution would work for our environment. Therefore, we started out with A/B testing in a PoC that we could spin up and execute in a matter of days.
Working with the Amazon Prototyping team, we narrowed down a range of options for our first integration to one that would require minimal changes to the website and be easily A/B tested. After examining all locations where a user is presented with a list of videos, we decided that re-ranking the list of videos to watch next would be the quickest to implement personalized content. For this prototype, we used an AWS Lambda function and Amazon API Gateway to provide a new API that would intercept the request for more videos and re-rank them using the Amazon Personalize GetPersonalizedRanking API.
To be considered successful, the experiment needed to demonstrate that statistically significant improvements had been made to either total video views or completion percentage. To make this possible, we needed to test across a sufficiently long enough period of time to make sure that we covered days with multiple sporting events and quieter days with no matches. We hoped to eliminate any behavior that would be dependent on the time of day or whether a match had recently been played by testing across different usage patterns. We set a time frame of 2 weeks to gather initial data. All users were part of the experiment and randomly assigned to either the control group or the test group. To keep the experiment as simple as possible, all videos were part of the experiment. The following diagram illustrates the architecture of our solution.
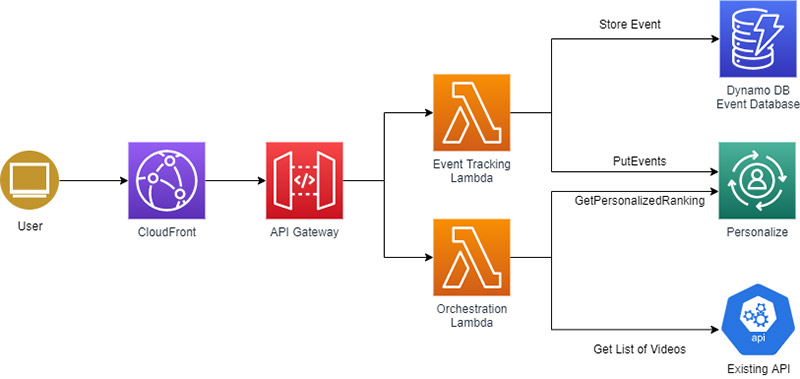
To get started, we needed to build an Amazon Personalize solution that provided us with the starting point for the experiment. Amazon Personalize requires a user-item interactions dataset to be able to define a solution and create a campaign to recommend videos to a user. We satisfied these requirements by creating a CSV file that contains a timestamp, user ID, and video ID for each video view across several weeks of usage. Uploading the interaction history to Amazon Personalize was a simple process, and we could immediately test the recommendations on the AWS Management Console. To train the model, we used a dataset of 30,000 recent interactions.
To compare metrics for total videos viewed and video completion percentage, we built a second API to record all video interactions in Amazon DynamoDB. This second API solved the problem of telling Amazon Personalize about new interactions via the PutEvents API, which helped keep the ML model up to date.
We tracked all video views and what prompted video views for all users in the experiment. Video prompts included direct linking (for example, from social media), linking from another part of the website, and linking from a list of videos. Each time a user viewed a video page, they were presented with the current list of videos or the new re-ranked list, depending on whether they were in the control or test group. We started our experiment with 5% of total users in the test group. When our approach showed no problems (no obvious drop in video consumption or increase in API errors), we increased this to 50%, with the remaining users acting as the control group, and started to collect data.
Learning from our experiment
After two weeks of A/B testing, we pulled the KPIs we collected from DynamoDB and compared the two variants we tested across several KPIs. We opted to use a few simple KPIs for this initial experiment, but other organizations’ KPIs may vary.
Our first KPI was the number of video views per user per session. Our initial hypothesis was that we wouldn’t see meaningful change given that we were re-ranking a list of videos; however, we measured an increase in views per user by 20%. The following graph summarizes our video views for each group.
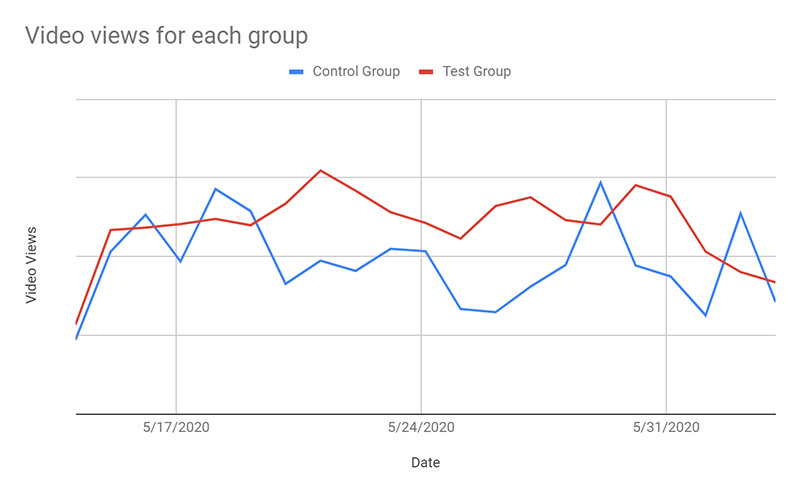
In addition to measuring total view count, we wanted to make sure that users were watching videos in full. We tracked this by sending an event for each 25% of the video a user viewed. For each video, we found that the average completion percentage didn’t change very much based on whether the video was recommended by Amazon Personalize or by the original list view. In combination with the number of videos viewed, we concluded that overall viewing time had increased for each user when presented with a personalized list of recommended videos.
We also tracked the position of each video in users’ “recommended video” bar and which item they selected. This allowed us to compare the ranking of a personalized list vs. a publication ordered list. We found that this didn’t make much difference between the two variants, which suggested that our users would most likely select a video that was visible on their screen rather than scrolling to see the entire list.
After we analyzed the results of the experiment, we presented them to the customer with the recommendation that we enable Amazon Personalize as the default method of ranking videos in the future.
Lessons learned
We learned the following lessons on our journey, which may help you when implementing your own solution:
- Gather your historical data of user-item interactions; we used about 30,000 interactions.
- Focus on recent historical data. Although your immediate position is to get as much historical data as you can, recent interactions are more valuable than older interactions. If you have a very large dataset of historical interactions, you can filter out older interactions to reduce the size of the dataset and training time.
- Make sure you can give all users a consistent and unique ID, either by using your SSO solution or by generating session IDs.
- Find a spot in your site or app where you can run an A/B test either re-ranking an existing list or displaying a list of recommended items.
- Update your API to call Amazon Personalize and fetch the new list of items.
- Deploy the A/B test and gradually increase the percentage of users in the experiment.
- Instrument and measure so that you can understand the outcome of your experiment.
Conclusion and future steps
We were thrilled by our first foray into the world of ML with Amazon Personalize. We found the entire process of integrating a trained model into our workflow was incredibly simple; and we spent far more time making sure that we had the right KPIs and data capture to prove the usefulness of the experiment than we did implementing Amazon Personalize.
In the future, we will be developing the following enhancements:
- Integrating Amazon Personalize throughout our workflow much more frequently by providing our development teams the opportunity to use Amazon Personalize everywhere a list of content is provided.
- Expanding the use cases beyond re-ranking to include recommended items. This should allow us to surface older items that are likely to be more popular with each user.
- Experiment with how often the model should be retrained—inserting new interactions into the model in real time is a great way to keep things fresh, but the models still needs daily retraining to be most effective.
- Exploring options for how we can use Amazon Personalize with all of our customers to help improve fan engagement by recommending the most relevant content in all forms.
- Using recommendation filters to expand the range of parameters available for each request. We will soon be targeting additional options such as filtering to include videos of your favorite players.
About the Author
Mark Wood is the Product Solutions Director at Pulselive. Mark has been at Pulselive for over 6 years and has held both Technical Director as well as Software Engineer roles during his tenure with the company. Prior to Pulselive, Mark was a Senior Engineer at Roke and a Developer at Querix. Mark is a graduate from the University of Southampton with a degree in Mathematics with Computer Science.
Read More