Amazon SageMaker is a fully managed service that allows you to build, train, deploy, and monitor machine learning (ML) models. Its modular design allows you to pick and choose the features that suit your use cases at different stages of the ML lifecycle. Amazon SageMaker offers capabilities that abstract the heavy lifting of infrastructure management and provides the agility and scalability you desire for large-scale ML activities with different features and a pay-as-you-use pricing model.
In this post, we outline the pricing model for Amazon SageMaker and offer some best practices on how you can optimize your cost of using Amazon SageMaker resources to effectively and efficiently build, train, and deploy your ML models. In addition, the post offers programmatic approaches for automatically stopping or detecting idle resources that are incurring costs, allowing you to avoid unnecessary charges.
Amazon SageMaker pricing
Machine Learning is an iterative process with different computational needs for prototyping the code and exploring the dataset, processing, training, and hosting the model for real-time and offline predictions. In a traditional paradigm, estimating the right amount of computational resources to support different workloads is difficult, and often leads to over-provisioning resources. The modular design of Amazon SageMaker offers flexibility to optimize the scalability, performance, and costs for your ML workloads depending on each stage of the ML lifecycle. For more information about how Amazon SageMaker works, see the following resources:
- What Is Amazon SageMaker?
- Amazon SageMaker Studio
- Get Started with Amazon SageMaker
The following diagram is a simplified illustration of the modular design for each stage of the ML lifecycle. Each environment, called build, train (and tune), and deploy, use separate compute resources with different pricing.
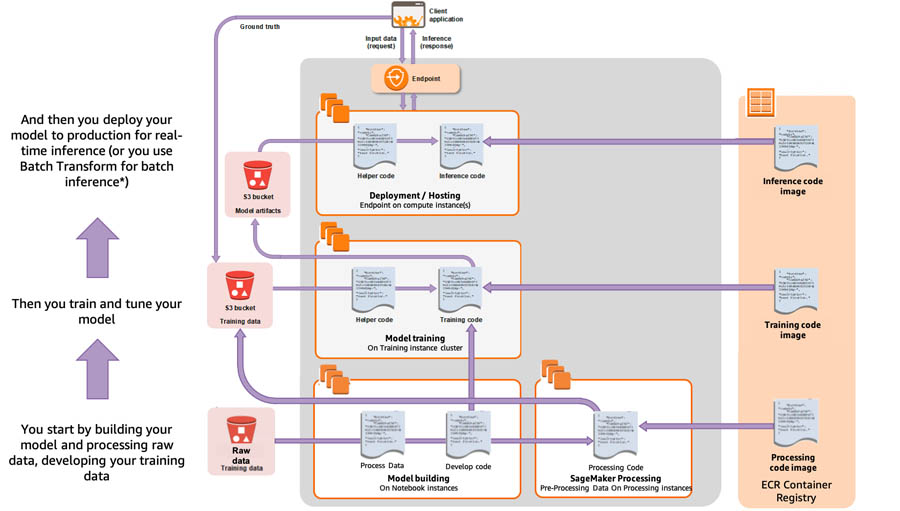
For more information about the costs involved in your ML journey on Amazon SageMaker, see Lowering total cost of ownership for machine learning and increasing productivity with Amazon SageMaker.
With Amazon SageMaker, you pay only for what you use. Pricing within Amazon SageMaker is broken down by the ML stage: building, processing, training, and model deployment (or hosting), and further explained in this section.
Build environment
Amazon SageMaker offers two environments for building your ML models: SageMaker Studio Notebooks and on-demand notebook instances. Amazon SageMaker Studio is a fully integrated development environment for ML, using a collaborative, flexible, and managed Jupyter notebook experience. You can now access Amazon SageMaker Studio, the first fully integrated development environment (IDE), for free, and you only pay for the AWS services that you use within Studio. For more information, see Amazon SageMaker Studio Tour.
Prices for compute instances are the same for both Studio and on-demand instances, as outlined in Amazon SageMaker Pricing. With Studio, your notebooks and associated artifacts such as data files and scripts are persisted on Amazon Elastic File System (Amazon EFS). For more information about storage charges, see Amazon EFS Pricing.
An Amazon SageMaker on-demand notebook instance is a fully managed compute instance running the Jupyter Notebook app. Amazon SageMaker manages creating the instance and related resources. Notebooks contain everything needed to run or recreate an ML workflow. You can use Jupyter notebooks in your notebook instance to prepare and process data, write code to train models, deploy models to Amazon SageMaker hosting, and test or validate your models.
Processing
Amazon SageMaker Processing lets you easily run your preprocessing, postprocessing, and model evaluation workloads on a fully managed infrastructure. Amazon SageMaker manages the instances on your behalf, and launches the instances for the job and terminates the instances when the job is done. For more information, see Amazon SageMaker Processing – Fully Managed Data Processing and Model Evaluation.
Training and tuning
Depending on the size of your training dataset and how quickly you need the results, you can use resources ranging from a single general-purpose instance to a distributed cluster of GPU instances. Amazon SageMaker manages these resources on your behalf, and provisions, launches, and then stops and terminates the compute resources automatically for the training jobs. With Amazon SageMaker training and tuning, you only pay for the time the instances were consumed for training. For more information, see Train and tune a deep learning model at scale.
Amazon SageMaker automatic model tuning, also known as hyperparameter tuning, finds the best version of a model by running many training jobs on your dataset using the algorithm and ranges of hyperparameters that you specify on a cluster of instances you define. Similar to training, you only pay for the resources consumed during the tuning time.
Deployment and hosting
You can perform model deployment for inference in two different ways:
- ML hosting for real-time inference – After you train your model, you can deploy it to get predictions in real time using a persistent endpoint with Amazon SageMaker hosting services
- Batch transform – You can use Amazon SageMaker batch transform to get predictions on an entire dataset offline
The Amazon SageMaker pricing model
The following table summarizing the pricing model for Amazon SageMaker.
|
ML Compute Instance |
Storage |
Data Processing In/Out |
Build (On-Demand Notebook Instances) |
Per instance-hour consumed while the notebook instance is running. |
Cost of GB-month of provisioned storage. |
No cost. |
Build (Stuio Notebookes) |
Per instance-hour consumed while the instance is running. |
See Amaon Elastic File System (EFS) pricing. |
No cost. |
Processing |
Per instance-hour consumed for each instance while the processing job is running. |
Cost of GB-month of provisioned storage. |
No cost. |
Training and Tuning |
On-Demand Instances: Per instance-hour consumed for each instance, from the time an instance is available for use until it is terminated or stopped. Each partial instance-hour consumed is billed per second.
Spot Training: Save up to 90% costs compared to on-demand instances by using managed spot training.
|
GB-month of provisioned storage. |
No cost. |
Batch Transform |
Per instance-hour consumed for each instance while the batch transform job is running. |
No cost. |
No cost |
Deployment (Hosting) |
Per instance-hour consumed for each instance while the endpoint is running. |
GB-month of provisioned storage. |
GB Data Processed IN and GB Data Processed OUT of the endpoint instance. |
You can also get started with Amazon SageMaker with the free tier. For more information about pricing, see Amazon SageMaker Pricing.
Right-sizing compute resources for Amazon SageMaker notebooks, processing jobs, training, and deployment
With the pricing broken down based on time and resources you use in each stage of an ML lifecycle, you can optimize the cost of Amazon SageMaker and only pay for what you really need. In this section, we discuss general guidelines to help you choose the right resources for your Amazon SageMaker ML lifecycle.
Amazon SageMaker currently offers ML compute instances on the following instance families:
- T – General-purpose burstable performance instances (when you don’t need consistently high levels of CPU, but benefit significantly from having full access to very fast CPUs when you need them)
- M – General-purpose instances
- C – Compute-optimized instances (ideal for compute bound applications)
- R – Memory-optimized instances (designed to deliver fast performance for workloads that process large datasets in memory)
- P, G and Inf – Accelerated compute instances (using hardware accelerators, or co-processors)
- EIA – Inference acceleration instances (used for Amazon Elastic Inference)
Instance type consideration for a computational workload running on an Amazon SageMaker ML compute instance is no different than running on an Amazon Elastic Compute Cloud (Amazon EC2) instance. For more information about instance specifications, such as number of virtual CPU and amount of memory, see Amazon SageMaker Pricing.
Build environment
The Amazon SageMaker notebook instance environment is suitable for interactive data exploration, script writing, and prototyping of feature engineering and modeling. We recommend using notebooks with instances that are smaller in compute for interactive building and leaving the heavy lifting to ephemeral training, tuning, and processing jobs with larger instances, as explained in the following sections. This way, you don’t keep a large instance (or a GPU) constantly running with your notebook. This can help you minimize your build costs by selecting the right instance.
For the building stage, the size of an Amazon SageMaker on-demand notebook instance depends on the amount of data you need to load in-memory for meaningful exploratory data analyses (EDA) and the amount of computation required. We recommend starting small with general-purpose instances (such as T or M families) and scale up as needed.
The burstable T family of instances is ideal for notebook activity because computation only comes when you run a cell but you want full power from CPU. For example, ml.t2.medium
is sufficient for most of basic data processing, feature engineering, and EDA that deal with small datasets that can be held within 4 GB memory. You can select an instance with larger memory capacity, such as ml.m5.12xlarge
(192 GB memory), if you need to load significantly more data into the memory for feature engineering. If your feature engineering involves heavy computational work (such as image processing), you can use one of the compute-optimized C family instances, such as ml.c5.xlarge
.
The benefit of Studio notebooks over on-demand notebook instances is that with Studio, the underlying compute resources are fully elastic and you can change the instance on the fly, allowing you to scale the compute up and down as your compute demand changes, for example from ml.t3.medium
to ml.g4dn.xlarge
as your build compute demand increases, without interrupting your work or managing infrastructure. Moving from one instance to another is seamless, and you can continue working while the instance launches. With on-demand notebook instances, you need to stop the instance, update the setting, and restart with the new instance type.
To keep your build costs down, we recommend stopping your on-demand notebook instances or shutting down your Studio instances when you don’t need them. In addition, you can use AWS Identity and Access Management (IAM) condition keys as an effective way to restrict certain instance types, such as GPU instances, for specific users, thereby controlling costs. We go into more detail in the section Recommendations for avoiding unnecessary costs.
Processing environment
After you complete data exploration and prototyping with a subset of your data and are ready to apply the preprocessing and transformation on the entire data, you can launch an Amazon SageMaker Processing job with your processing script that you authored during the EDA phase without scaling up the relatively small notebook instance you have been using. Amazon SageMaker Processing dispatches all things needed for processing the entire dataset, such as code, container, and data, to a compute infrastructure separate from the Amazon SageMaker notebook instance. Amazon SageMaker Processing takes care of the resource provisioning, data and artifact transfer, and shutdown of the infrastructure once the job finishes.
The benefit of using Amazon SageMaker Processing is that you only pay for the processing instances while the job is running. Therefore, you can take advantage of powerful instances without worrying too much about the cost. For example, as a general recommendation, you can use an ml.m5.4xlarge
for medium jobs (MBs of GB of data), ml.c5.18xlarge
for workloads requiring heavy computational capacity, or ml.r5.8xlarge
when you want to load multiple GBs of data in memory for processing, and only pay for the time of the processing job. Sometimes, you may consider using a larger instance to get the job done quicker, and end up paying less in total cost of the job.
Alternatively, for distributed processing, you can use a cluster of smaller instances by increasing the instance count. For this purpose, you could shard input objects by Amazon Simple Storage Service (Amazon S3) key by setting s3_data_distribution_type='ShardedByS3Key'
inside a ProcessingInput so that each instance receives about the same number of more manageable input objects, and instead you can use smaller instances in the cluster, leading to potential cost savings. Furthermore, you could perform the processing job asynchronously with .run(…, wait = False), as if you submit the job and get your notebook cell back immediately for other activities, leading to a more efficient use of your build compute instance time.
Training and tuning environment
The same compute paradigm and benefits for Amazon SageMaker Processing apply to Amazon SageMaker Training and Tuning. When you use fully managed Amazon SageMaker Training, it dispatches all things needed for a training job, such as code, container, and data, to a compute infrastructure separate from the Amazon SageMaker notebook instance. Therefore, your training jobs aren’t limited by the compute resource of the notebook instance. The Amazon SageMaker Training Python SDK also supports asynchronous training when you call .fit(…, wait = False)
. You get your notebook cell back immediately for other activities, such as calling .fit()
again for another training job with a different ML compute instance for profiling purposes or a variation of the hyperparameter settings for experimentation purposes. Because ML training can often be a compute-intensive and time-consuming part of the ML lifecycle, with training jobs happening asynchronously in a remote compute infrastructure, you can safely shut down the notebook instance for cost-optimizing purposes if starting a training job is the last task of your day. We discuss how to automatically shut down unused, idle on-demand notebook instances in the section Recommendations for avoiding unnecessary costs.
Cost-optimization factors that you need to consider when selecting instances for training include the following:
- Instance family – What type of instance is suitable for the training? You need to optimize for overall cost of training, and sometimes selecting a larger instance can lead to much faster training and thus less total cost; can the algorithm even utilize a GPU instance?
- Instance size – What is the minimum compute and memory capacity your algorithm requires to run the training? Can you use distributed training?
- Instance count – If you can use distributed training, what instance type (CPU or GPU) can you use in the cluster, and how many?
As for the choice of instance type, you could base your decision on what algorithms or frameworks you use for the workload. If you use the Amazon SageMaker built-in algorithms, which give you a head start without any sophisticated programming, see Instance types for built-in algorithms for detailed guidelines. For example, XGBoost currently only trains using CPUs. It is a memory-bound (as opposed to compute-bound) algorithm. So, a general-purpose compute instance (for example, M5
) is a better choice than a compute-optimized instance (for example, C4
).
Furthermore, we recommend having enough total memory in selected instances to hold the training data. Although it supports the use of disk space to handle data that doesn’t fit into main memory (the out-of-core feature available with the libsvm input mode), writing cache files onto disk slows the algorithm processing time. For the object detection algorithm, we support the following GPU instances for training:
ml.p2.xlarge
ml.p2.8xlarge
ml.p2.16xlarge
ml.p3.2xlarge
ml.p3.8xlarge
ml.p3.16xlarge
We recommend using GPU instances with more memory for training with large batch sizes. You can also run the algorithm on multi-GPU and multi-machine settings for distributed training.
If you’re bringing your own algorithms with script mode or with custom containers, you need to first clarify whether the framework or algorithm supports CPU, GPU, or both to decide the instance type to run the workload. For example, scikit-learn doesn’t support GPU, meaning that training with accelerated compute instances doesn’t result in any material gain in runtime but leads to overpaying for the instance. To determine which instance type and number of instances, if training in distributed fashion, for your workload, it’s highly recommended to profile your jobs to find the sweet spot between number of instance and runtime, which translates to cost. For more information, see Amazon Web Services achieves fasted training times for BERT and Mask R-CNN. You should also find the balance between instance type, number of instances, and runtime. For more information, see Train ALBERT for natural language processing with TensorFlow on Amazon SageMaker.
When it comes to GPU-powered P
and G
families of instances, you need to consider the differences. For example, P3
GPU compute instances are designed to handle large distributed training jobs for fastest time to train, whereas G4 instances are suitable for cost-effective, small-scale training jobs.
Another factor to consider in training is that you can select from either On-Demand Instances or Spot Instances. On-demand ML instances for training let you pay for ML compute capacity based on the time the instance is consumed, at on-demand rates. However, for jobs that can be interrupted or don’t need to start and stop at specific times, you can choose managed Spot Instances (Managed Spot Training). Amazon SageMaker can reduce the cost of training models by up to 90% over On-Demand Instances, and manages the Spot interruptions on your behalf.
Deployment/hosting environment
In many cases, up to 90% of the infrastructure spend for developing and running an ML application is on inference, making the need for high-performance, cost-effective ML inference infrastructure critical. This is mainly because the build and training jobs aren’t frequent and you only pay for the duration of build and training, but an endpoint instance is running all the time (while the instance is in service). Therefore, selecting the right way to host and the right type of instance can have a large impact on the total cost of ML projects.
For model deployment, it’s important to work backwards from your use case. What is the frequency of the prediction? Do you expect live traffic to your application and real-time response to your clients? Do you have many models trained for different subsets of data for the same use case? Does the prediction traffic fluctuate? Is latency of inference a concern?
There are hosting options from Amazon SageMaker for each of these situations. If your inference data comes in batches, Amazon SageMaker batch transform is a cost-effective way to obtain predictions with fully managed infrastructure provisioning and tear-down. If you have trained multiple models for one single use case, a multi-modal endpoint is a great way to save cost on hosting ML models that are trained on a per-user or segment basis. For more information, see Save on inference costs by using Amazon SageMaker multi-model endpoints.
After you decide how to host your models, load testing is the best practice to determine the appropriate instance type and fleet size, with or without autoscaling for your live endpoint to avoid over-provisioning and paying extra for capacity you don’t need. Algorithms that train most efficiently on GPUs might not benefit from GPUs for efficient inference. It’s important to load test to determine the most cost-effective solution. The following flowchart summarizes the decision process.
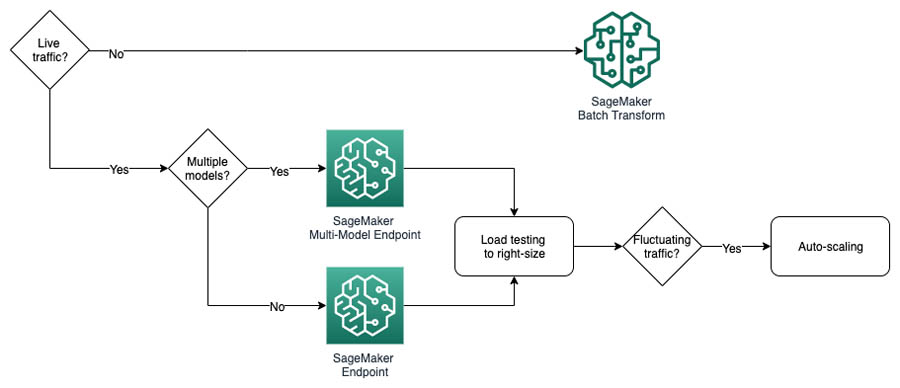
Amazon SageMaker offers different options for instance families that you can use for inference, from general-purpose instances to compute-optimized and GPU-powered instances. Each family is optimized for a different application, and not all instance types are suitable for inference jobs. For example, Amazon Inf1 instances offer high throughput and low latency and have the lowest cost per inference in the cloud. G4
instances for inference G4 have the lowest cost per inference for GPU instances and offer greater performance, lower latency, and reduced cost per inference for GPU instances. And P3
instances are optimized for training, and are designed to handle large distributed training jobs for fastest time to train, and thus not fully utilized for inference.
Another way to lower inference cost is to use Elastic Inference for cost savings of up to 75% on inference jobs. Picking an instance type and size for inference may not be easy, given the many factors involved. For example, for larger models, the inference latency of CPUs may not meet the needs of online applications, while the cost of a full-fledged GPU may not be justified. In addition, resources like RAM and CPU may be more important to the overall performance of your application than raw inference speed. With Elastic Inference, you attach just the right amount of GPU-powered inference acceleration to any Amazon compute instance. This is also available for Amazon SageMaker notebook instances and endpoints, bringing acceleration to built-in algorithms and to deep learning environments. This lets you select the best price/performance ratio for your application. For example, an ml.c5.large
instance configured with eia1.medium
acceleration costs you about 75% less than an ml.p2.xlarge
, but with only 10–15% slower performance. For more information, see Amazon Elastic Inference – GPU-Powered Deep Learning Inference Acceleration.
In addition, you can use Auto Scaling for Amazon SageMaker to add and remove capacity or accelerated instances to your endpoints automatically, whenever needed. With this feature, instead of having to closely monitor inference volume and change the endpoint configuration in response, your endpoint automatically adjusts the number of instances up or down in response to actual workloads, determined by using Amazon CloudWatch metrics and target values defined in the policy. For more information, see AWS Auto Scaling.
Recommendations for avoiding unnecessary costs
Certain Amazon SageMaker resources (such as processing, training, tuning, and batch transform instances) are ephemeral, and Amazon SageMaker automatically launches the instance and terminates them when the job is done. However, other resources (such as build compute resources or hosting endpoints) aren’t ephemeral, and the user has control over when these resources should be stopped or terminated. Therefore, knowing how to identify idle resources and stopping them can lead to better cost-optimization. This section outlines some useful methods for automating these processes.
Build environment: Automatically stopping idle on-demand notebook instances
One way to avoid the cost of idle notebook instances is to automatically stop idle instances using lifecycle configurations. With lifecycle configuration in Amazon SageMaker, you can customize your notebook environment by installing packages or sample notebooks on your notebook instance, configuring networking and security for it, or otherwise use a shell script to customize it. Such flexibility allows you to have more control over how your notebook environment is set up and run.
AWS maintains a public repository of notebook lifecycle configuration scripts that address common use cases for customizing notebook instances, including a sample bash script for stopping idle notebooks.
You can configure your notebook instance using a lifecycle configuration to automatically stop itself if it’s idle for a certain period of time (a parameter that you set). The idle state for a Jupyter notebook is defined in the following GitHub issue. To create a new lifecycle configuration for this purpose, follow these steps:
- On the Amazon SageMaker console, choose Lifecycle configurations.
- Choose Create a new lifecycle configuration (if you are creating a new one).
- For Name, enter a name using alphanumeric characters and
-
, but no spaces. The name can have a maximum of 63 characters. For example, Stop-Idle-Instance
.
- To create a script that runs when you create the notebook and every time you start it, choose Start notebook.
- In the Start notebook editor, enter the script.
- Choose Create configuration.
The bash script to use for this purpose can be found on AWS Samples repository for lifecycle configuration samples. This script is basically running a cron job at a specific period of idle time, as defined with parameter IDLE_TIME
in the script. You can change this time to your preference and change the script as needed on the Lifecycle configuration page.
For this script to work, the notebook should meet these two criteria:
- The notebook instance has internet connectivity to fetch the example config Python script (
autostop.py
) from the public repository
- The notebook instance execution role permissions to
SageMaker:StopNotebookInstance
to stop the notebook and SageMaker:DescribeNotebookInstance
to describe the notebook
If you create notebook instances in a VPC that doesn’t allow internet connectivity, you need to add the Python script inline in the bash script. The script is available on the GitHub repo. Enter it in your bash script as follows, and use this for lifecycle configuration instead:
#!/bin/bash
set -e
# PARAMETERS
IDLE_TIME=3600
echo "Creating the autostop.py"
cat << EOF > autostop.py
##
## [PASTE PYTHON SCRIPT FROM GIT REPO HERE]
##
EOF
echo "Starting the SageMaker autostop script in cron"
(crontab -l 2>/dev/null; echo "*/5 * * * * /usr/bin/python $PWD/autostop.py --time $IDLE_TIME --ignore-connections") | crontab -
The following screenshot shows how to choose the lifecycle configuration on the Amazon SageMaker console.
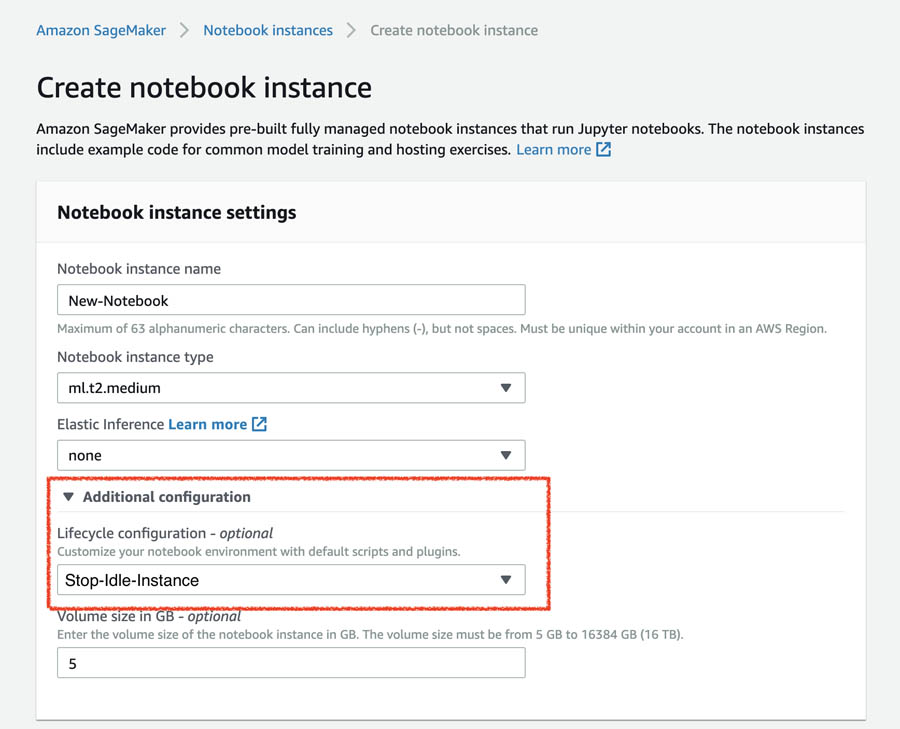
Alternatively, you can store the script on Amazon S3 and connect to the script through a VPC endpoint. For more information, see New – VPC Endpoint for Amazon S3.
Now that you have created the lifecycle configuration, you can assign it to your on-demand notebook instance when creating a new one or when updating existing notebooks. To create a notebook with your lifecycle configuration (for this post, Stop-Idle-Instance
), you need to assign the script to the notebook under the Additional Configuration section. All other steps are the same as outlined in Create a On-Demand Notebook Instance. To attach the lifecycle configuration to an existing notebook, you first need to stop the on-demand notebook instance, and choose Update settings to make changes to the instance. You attach the lifecycle configuration in the Additional configuration section.
Build environment: Scheduling start and stop of on-demand notebook instances
Another approach is to schedule your notebooks to start and stop at specific times. For example, if you want to start your notebooks (such as notebooks of specific groups or all notebooks in your account) at 7:00 AM and stop all of them at 9:00 PM during weekdays (Monday through Friday), you can accomplish this by using Amazon CloudWatch Events and AWS Lambda functions. For more information about configuring your Lambda functions, see Configuring functions in the AWS Lambda console. To build the schedule for this use case, you can follow the steps in the following sections.
Starting notebooks with a Lambda function
To start your notebooks with a Lambda function, complete the following steps:
- On the Lambda console, create a Lambda function for starting on-demand notebook instances with specific keywords in their name. For this post, our development team’s on-demand notebook instances have names starting with
dev-
.
- Use Python as the runtime for the function, and name the function
start-dev-notebooks
.
Your Lambda function should have the SageMakerFullAccess
policy attached to its execution IAM role.
- Enter the following script into the Function code editing area:
# Code to start InService Notebooks that contain specific keywords in their name
# Change "dev-" in NameContains to your specific use case
import boto3
client = boto3.client('sagemaker')
def lambda_handler(event, context):
try:
response_nb_list = client.list_notebook_instances(
NameContains='dev-', # Change this to your specific use case
StatusEquals= 'Stopped'
)
for nb in response_nb_list['NotebookInstances']:
response_nb_stop = client.start_notebook_instance(
NotebookInstanceName = nb['NotebookInstanceName'])
return {"Status": "Success"}
except:
return {"Status": "Failure"}
- Under Basic Settings, change Timeout to 15 minutes (max).
This step makes sure the function has the maximum allowable timeout range during stopping and starting multiple notebooks.
- Save your function.
Stopping notebooks with a Lambda function
To stop your notebooks with a Lambda function, follow the same steps, use the following script, and name the function stop-dev-notebooks
:
# Code to stop InService Notebooks that contain specific keywords in their name
# Change "dev-" in NameContains to your specific use case
import boto3
client = boto3.client('sagemaker')
def lambda_handler(event, context):
try:
response_nb_list = client.list_notebook_instances(
NameContains='dev-', # Change this to your specific use case
StatusEquals= 'InService'
)
for nb in response_nb_list['NotebookInstances']:
response_nb_stop = client.stop_notebook_instance(
NotebookInstanceName = nb['NotebookInstanceName'])
return {"Status": "Success"}
except:
return {"Status": "Failure"}
Creating a CloudWatch event
Now that you have created the functions, you need to create an event to trigger these functions on a specific schedule.
We use cron expression format for the schedule. For more information about creating your custom cron expression, see Schedule Expressions for Rules. All scheduled events use UTC time zone, and the minimum precision for schedules is 1 minute.
For example, the cron expression for 7:00 AM, Monday through Friday throughout the year, is 0 7 ? * MON-FRI *
, and for 9:00 PM on the same days is 0 21 ? * MON-FRI *
.
To create the event for stopping your instances on a specific schedule, complete the following steps:
- On the CloudWatch console, under Events, choose Rules.
- Choose Create rule.
- Under Event Source, select Schedule, and then select Cron expression.
- Enter your cron expression (for example,
21 ? * MON-FRI *
for 9:00 PM Monday through Friday).
- Under Targets, choose Lambda function.
- Choose your function from the list (for this post,
stop-dev-notebooks
).
- Choose Configure details
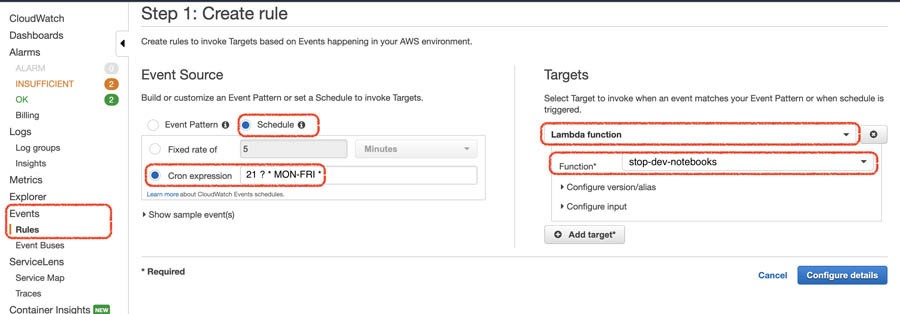
- Add a name for your event, such as
Stop-Notebooks-Event
, and a description.
- Leave Enabled
- Choose Create.
You can follow the same steps to create scheduled event to start your notebooks on a schedule, such as 7:00 AM on weekdays, so when your staff start their day, the notebooks are ready and in service.
Hosting environment: Automatically detecting idle Amazon SageMaker endpoints
You can deploy your ML models as endpoints to test the model for real-time inference. Sometimes these endpoints are accidentally left in service, leading to ongoing charges on the account. You can automatically detect these endpoints and take corrective actions (such as deleting them) by using CloudWatch Events and Lambda functions. For example, you can detect if endpoints have been idle for the past number of hours (with no invocations over a certain period, such as 24 hours). The function script we provide in this section detects idle endpoints and publishes a message to an Amazon Simple Notification Service (Amazon SNS) topic with the list of idle endpoints. You can subscribe the account admins to this topic, and they receive emails with the list of idle endpoints when detected. To create this scheduled event, follow these steps:
- Create an SNS topic and subscribe your email or phone number to it.
- Create a Lambda function with the following script.
- Your Lambda function should have the following policies attached to its IAM execution role:
CloudWatchReadOnlyAccess
, AmazonSNSFullAccess
, and AmazonSageMakerReadOnly
.
import boto3
from datetime import datetime
from datetime import timedelta
def lambda_handler(event, context):
idle_threshold_hr = 24 # Change this to your threshold in hours
cw = boto3.client('cloudwatch')
sm = boto3.client('sagemaker')
sns = boto3.client('sns')
try:
inservice_endpoints = sm.list_endpoints(
SortBy='CreationTime',
SortOrder='Ascending',
MaxResults=100,
# NameContains='string', # for example 'dev-'
StatusEquals='InService'
)
idle_endpoints = []
for ep in inservice_endpoints['Endpoints']:
ep_describe = sm.describe_endpoint(
EndpointName=ep['EndpointName']
)
metric_response = cw.get_metric_statistics(
Namespace='AWS/SageMaker',
MetricName='Invocations',
Dimensions=[
{
'Name': 'EndpointName',
'Value': ep['EndpointName']
},
{
'Name': 'VariantName',
'Value': ep_describe['ProductionVariants'][0]['VariantName']
}
],
StartTime=datetime.utcnow()-timedelta(hours=idle_threshold_hr),
EndTime=datetime.utcnow(),
Period=int(idle_threshold_hr*60*60),
Statistics=['Sum'],
Unit='None'
)
if len(metric_response['Datapoints'])==0:
idle_endpoints.append(ep['EndpointName'])
if len(idle_endpoints) > 0:
response_sns = sns.publish(
TopicArn='YOUR SNS TOPIC ARN HERE',
Message="The following endpoints have been idle for over {} hrs. Log on to Amazon SageMaker console to take actions.nn{}".format(idle_threshold_hr, 'n'.join(idle_endpoints)),
Subject='Automated Notification: Idle Endpoints Detected',
MessageStructure='string'
)
return {'Status': 'Success'}
except:
return {'Status': 'Fail'}
You can also revise this code to filter the endpoints based on resource tags. For more information, see AWS Python SDK Boto3 documentation.
Investigating endpoints
This script sends an email (or text message, depending on how the SNS topic is configured) with the list of detected idle endpoints. You can then sign in to the Amazon SageMaker console and investigate those endpoints, and delete them if you find them to be unused stray endpoints. To do so, complete the following steps:
- On the Amazon SageMaker console, under Inference, choose Endpoints.
You can see the list of all endpoints on your account in that Region.
- Select the endpoint that you want to investigate, and under Monitor, choose View invocation metrics.
- Under All metrics, select Invocations
You can see the invocation activities on the endpoint. If you notice no invocation event (or activity) for the duration of your interest, it means the endpoint isn’t in use and you can delete it.
- When you’re confident you want to delete the endpoint, go back to the list of endpoints, select the endpoint you want to delete, and under the Actions menu, choose
Conclusion
This post walked you through how Amazon SageMaker pricing works, best practices for right-sizing Amazon SageMaker compute resources for different stages of an ML project, and best practices for avoiding unnecessary costs of unused resources by either automatically stopping idle on-demand notebook instances or automatically detecting idle Amazon SageMaker endpoints so you can take corrective actions.
By understanding how Amazon SageMaker works and the pricing model for Amazon SageMaker resources, you can take steps in optimizing your total cost of ML projects even further.
About the authors
Nick Minaie is an Artificial Intelligence and Machine Learning (AI/ML) Specialist Solution Architect, helping customers on their journey to well-architected machine learning solutions at scale. In his spare time, Nick enjoys family time, abstract painting, and exploring nature.
Michael Hsieh is a Senior AI/ML Specialist Solutions Architect. He works with customers to advance their ML journey with a combination of AWS ML offerings and his ML domain knowledge. As a Seattle transplant, he loves exploring the great mother nature the city has to offer such as the hiking trails, scenic kayaking in the SLU, and the sunset at the Shilshole Bay.
Read More