Posted by Huiyu Wang, Student Researcher and Liang-Chieh Chen, Research Scientist, Google Research
Panoptic segmentation is a computer vision task that unifies semantic segmentation (assigning a class label to each pixel) and instance segmentation (detecting and segmenting each object instance). A core task for real-world applications, panoptic segmentation predicts a set of non-overlapping masks along with their corresponding class labels (i.e., category of object, like “car”, “traffic light”, “road”, etc.) and is generally accomplished using multiple surrogate sub-tasks that approximate (e.g., by using box detection methods) the goals of panoptic segmentation.
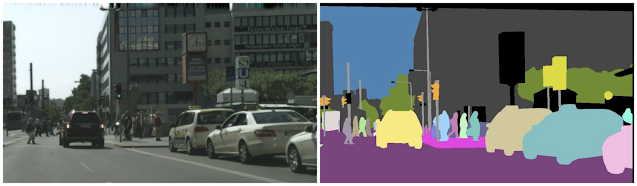 |
An example image and its panoptic segmentation masks from the Cityscapes dataset. |
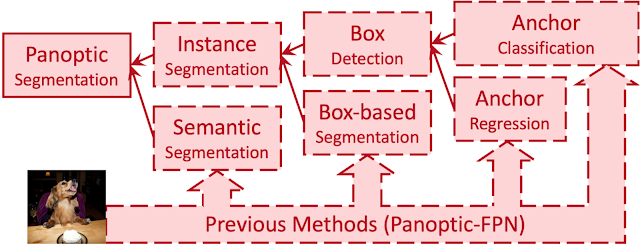 |
Previous methods approximate panoptic segmentation with a tree of surrogate sub-tasks. |
Each surrogate sub-task in this proxy tree introduces extra manually-designed modules, such as anchor design rules, box assignment rules, non-maximum suppression (NMS), thing-stuff merging, etc. Although there are good solutions to individual surrogate sub-tasks and modules, undesired artifacts are introduced when these sub-tasks come together in a pipeline for panoptic segmentation, especially in challenging conditions (e.g., two people with similar bounding boxes will trigger NMS, resulting in a missing mask).
Previous efforts, such as DETR, attempted to solve some of these issues by simplifying the box detection sub-task into an end-to-end operation, which is more computationally efficient and results in fewer undesired artifacts. However, the training process still relies heavily on box detection, which does not align with the mask-based definition of panoptic segmentation. Another line of work completely removes boxes from the pipeline, which has the benefit of removing an entire surrogate sub-task along with its associated modules and artifacts. For example, Axial-DeepLab predicts pixel-wise offsets to predefined instance centers, but the surrogate sub-task it uses encounters challenges with highly deformable objects, which have a large variety of shapes (e.g., a cat), or nearby objects with close centers in the image plane, e.g. the image below of a dog seated in a chair.
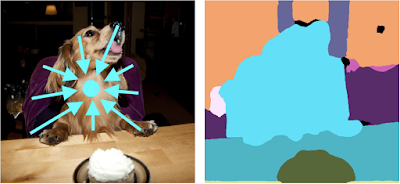 |
When the centers of the dog and the chair are close to each other, Axial-DeepLab merges them into one object. |
In “MaX-DeepLab: End-to-End Panoptic Segmentation with Mask Transformers”, to be presented at CVPR 2021, we propose the first fully end-to-end approach for the panoptic segmentation pipeline, directly predicting class-labeled masks by extending the Transformer architecture to this computer vision task. Dubbed MaX-DeepLab for extending Axial-DeepLab with a Mask Xformer, our method employs a dual-path architecture that introduces a global memory path, allowing for direct communication with any convolution layers. As a result, MaX-DeepLab shows a significant 7.1% panoptic quality (PQ) gain in the box-free regime on the challenging COCO dataset, closing the gap between box-based and box-free methods for the first time. MaX-DeepLab achieves the state-of-the-art 51.3% PQ on COCO test-dev set, without test time augmentation.
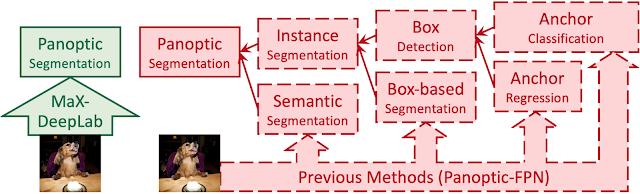 |
MaX-DeepLab is fully end-to-end: It predicts panoptic segmentation masks directly from images. |
End-to-End Panoptic Segmentation
Inspired by DETR, our model directly predicts a set of non-overlapping masks and their corresponding semantic labels, with output masks and classes that are optimized with a PQ-style objective. Specifically, inspired by the evaluation metric, PQ, which is defined as the recognition quality (whether or not the predicted class is correct) times the segmentation quality (whether the predicted mask is correct), we define a similarity metric between two class-labeled masks in the exact same way. The model is directly trained by maximizing this similarity between ground truth masks and predicted masks via one-to-one matching. This direct modeling of panoptic segmentation enables end-to-end training and inference, removing the hand-coded priors that are necessary in existing box-based and box-free methods.
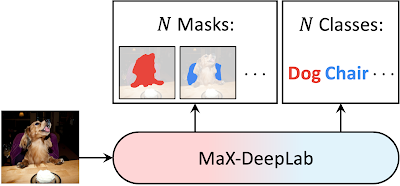 |
MaX-DeepLab directly predicts N masks and N classes with a CNN and a mask transformer. |
Dual-Path Transformer
Instead of stacking a traditional transformer on top of a convolutional neural network (CNN), we propose a dual-path framework for combining CNNs with transformers. Specifically, we enable any CNN layer to read and write to global memory by using a dual-path transformer block. This proposed block adopts all four types of attention between the CNN-path and the memory-path, and can be inserted anywhere in a CNN, enabling communication with the global memory at any layer. MaX-DeepLab also employs a stacked-hourglass-style decoder that aggregates multi-scale features into a high resolution output. The output is then multiplied with the global memory feature, to form the mask set prediction. The classes for the masks are predicted with another branch of the mask transformer.
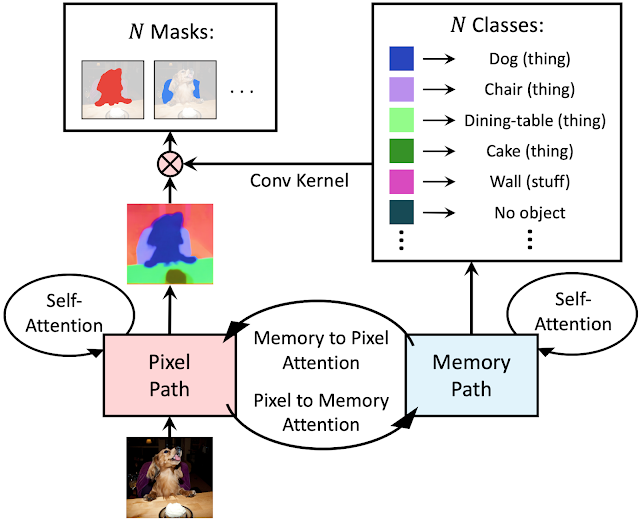 |
An overview of the dual-path transformer architecture. |
Results
We evaluate MaX-DeepLab on one of the most challenging panoptic segmentation datasets, COCO, against both of the state-of-the-art box-free (Axial-DeepLab) and box-based (DetectoRS) methods. MaX-DeepLab, without test time augmentation, achieves the state-of-the-art result of 51.3% PQ on the test-dev set.
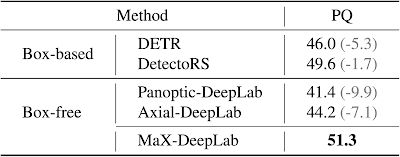 |
Comparison on COCO test-dev set. |
This result surpasses Axial-DeepLab by 7.1% PQ in the box-free regime and DetectoRS by 1.7% PQ, bridging the gap between box-based and box-free methods for the first time. For a consistent comparison with DETR, we also evaluated a lightweight version of MaX-DeepLab that matches the number of parameters and computations of DETR. The lightweight MaX-DeepLab outperforms DETR by 3.3% PQ on the val set and 3.0% PQ on the test-dev set. In addition, we performed extensive ablation studies and analyses on our end-to-end formulation, model scaling, dual-path architectures, and loss functions. Also the extra-long training schedule of DETR is not necessary for MaX-DeepLab.
As an example in the figure below, MaX-DeepLab correctly segments a dog sitting on a chair. Axial-DeepLab relies on a surrogate sub-task of regressing object center offsets. It fails because the centers of the dog and the chair are close to each other. DetectoRS classifies object bounding boxes, instead of masks, as a surrogate sub-task. It filters out the chair mask because the chair bounding box has a low confidence.
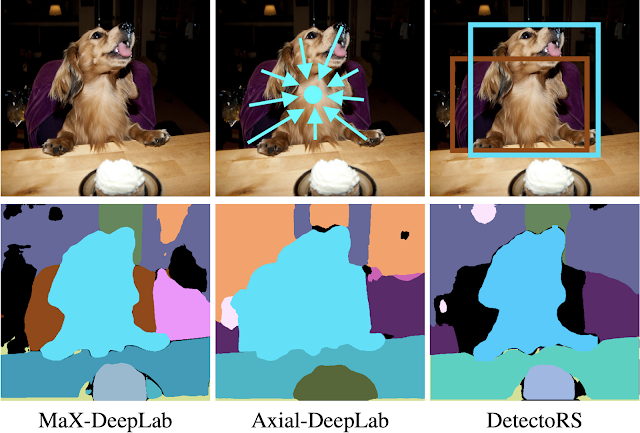 |
A case study for MaX-DeepLab and state-of-the-art box-free and box-based methods. |
Another example shows how MaX-DeepLab correctly segments images with challenging conditions.
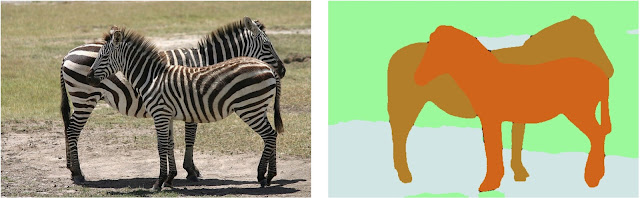 |
MaX-DeepLab correctly segments the overlapping zebras. This case is also challenging for other methods since the zebras have similar bounding boxes and nearby object centers. (credit & license) |
Conclusion
We have shown for the first time that panoptic segmentation can be trained end-to-end. MaX-DeepLab directly predicts masks and classes with a mask transformer, removing the need for many hand-designed priors such as object bounding boxes, thing-stuff merging, etc. Equipped with a PQ-style loss and a dual-path transformer, MaX-DeepLab achieves the state-of-the-art result on the challenging COCO dataset, closing the gap between box-based and box-free methods.
Acknowledgements
We are thankful to our co-authors, Yukun Zhu, Hartwig Adam, and Alan Yuille. We also thank Maxwell Collins, Sergey Ioffe, Jiquan Ngiam, Siyuan Qiao, Chen Wei, Jieneng Chen, and the Mobile Vision team for the support and valuable discussions.
Read More