Polito is one of the featured speakers at the first virtual Amazon Web Services Machine Learning Summit on June 2.Read More
Hanging in the Balance: More Research Coordination, Collaboration Needed for AI to Reach Its Potential, Experts Say
As AI is increasingly established as a world-changing field, the U.S. has an opportunity not only to demonstrate global leadership, but to establish a solid economic foundation for the future of the technology.
A panel of experts convened last week at GTC to shed light on this topic, with the co-chairs of the Congressional AI Caucus, U.S. Reps. Jerry McNerney (D-CA) and Anthony Gonzalez (R-OH), leading a discussion that reflects Washington’s growing interest in the topic.
The panel also included Hodan Omaar, AI policy lead at the Center for Data Innovation; Russell Wald, director of policy at Stanford University’s Institute for Human-Centered AI and Damon Woodard, director of AI partnerships at University of Florida’s AI Initiative.
“AI is getting increased interest among my colleagues on both sides of the aisle, and this is going to continue for some time,” McNerney said. Given that momentum, Gonzalez said the U.S. should be on the bleeding edge of AI development “for both economic and geopolitical reasons.”
Along those lines, the first thing the pair wanted to learn was how panelists viewed the importance of legislative efforts to fund and support AI research and development.
Wald expressed enthusiasm over legislation Congress passed last year as part of the National Defense Authorization Act, which he said would have an expansive effect on the market for AI.
Wald also said he was surprised at the findings of Stanford’s “Government by Algorithm” report, which detailed the federal government’s use of AI to do things such as track suicide risk among veterans, support SEC insider trading investigations and identify Medicare fraud.
Woodard suggested that continued leadership and innovation coming from Washington is critical if AI is to deliver on its promise.
“AI can play a big role in the economy,” said Woodard. “Having this kind of input from the government is important before we can have the kind of advancements that we need.”
The Role of Universities
Woodard and UF are already doing their part. Woodard’s role at the school includes helping transform it into a so-called “AI university.” In response to a question from Gonzalez about what that transition looks like, he said it required establishing a world-class AI infrastructure, performing cutting-edge AI research and incorporating AI throughout the curriculum.
“We want to make sure every student has some exposure to AI as it relates to their field of study,” said Woodard.
He said the school has more than 200 faculty members engaged in AI-related research, and that it’s committed to hiring 100 more. And while Woodard believes the university’s efforts will lead to more qualified AI professionals and AI innovation around its campus in Gainesville, he also said that partnerships, especially those that encourage diversity, are critical to encouraging more widespread industry development.
Along those lines, UF has joined an engineering consortium and will provide 15 historically Black colleges and two Hispanic-serving schools with access to its prodigious AI resources.
Omaar said such efforts are especially important when considering how unequally the high performance computing resources needed to conduct AI research are distributed.
In response to a question from McNerney about a recent National Science Foundation report, Omaar noted the finding that the U.S. Department of Energy is only providing support to about a third of the researchers seeking access to HPC resources.
“Many universities are conducting AI research without the tools they need,” she said.
Omaar said she’d like to see the NSF focus its funding on supporting efforts in states where HPC resources are scarce but AI research activity is high.
McNerney announced that he would soon introduce legislation requiring NSF to determine what AI resources are necessary for significant research output.
Moving Toward National AI Research Resources
The myriad challenges points to the benefits that could come from a more coordinated national effort. To that end, Gonzalez asked about the potential of the National AI Research Resource Task Force Act, and the national AI research cloud that would result from it.
Wald called the legislation a “game-changing AI initiative,” noting that the limited number of universities with AI research computing resources has pushed AI research into the private sector, where the objectives are driven by shorter-term financial goals rather than long-term societal benefits.
“What we see is an imbalance in the AI research ecosystem,” Wald said. The federal legislation would establish a pathway for a national AI research hub, which “has the potential to unleash American AI innovation,” he said.
The way Omaar sees it, the nationwide collaboration that would likely result — among politicians, industry and academia — is necessary for AI to reach its potential.
“Since AI will impact us all,” she said, “it’s going to need everyone’s contribution.”
The post Hanging in the Balance: More Research Coordination, Collaboration Needed for AI to Reach Its Potential, Experts Say appeared first on The Official NVIDIA Blog.
It’s here! Join us for Amazon SageMaker Month, 30 days of content, discussion, and news
Want to accelerate machine learning (ML) innovation in your organization? Join us for 30 days of new Amazon SageMaker content designed to help you build, train, and deploy ML models faster. On April 20, we’re kicking off 30 days of hands-on workshops, Twitch sessions, Slack chats, and partner perspectives. Our goal is to connect you with AWS experts—including Greg Coquillio, the second-most influential speaker according to LinkedIn Top Voices 2020: Data Science & AI and Julien Simon, the number one AI evangelist according to AI magazine —to learn hints and tips for success with ML.
We built SageMaker from the ground up to provide every developer and data scientist with the ability to build, train, and deploy ML models quickly and at lower cost by providing the tools required for every step of the ML development lifecycle in one integrated, fully managed service. We have launched over 50 SageMaker capabilities in the past year alone, all aimed at making this process easier for our customers. The customer response to what we’re building has been incredible, making SageMaker one of the fastest growing services in AWS history.
To help you dive deep into these SageMaker innovations, we’re dedicating April 20 – May 21, 2021 to SageMaker education. Here are some must dos to add to your calendar:
- April 23 – Introduction to SageMaker workshop
- April 30 – SageMaker Fridays Twitch session with Greg Coquillio and Julien Simon on cost-optimization
- May 12 – An end-to-end tutorial on SageMaker during the workshop at the AWS Summit
Besides these virtual hands-on opportunities, we will have regular blog posts from AWS experts and our partners, including Snowflake, Tableau, Genesys, and DOMO. Bookmark the SageMaker Month webpage or sign up to our weekly newsletters so you don’t miss any of the planned activities.
But we aren’t stopping there!
To coincide with SageMaker Month, we launched new Savings Plans. The SageMaker Savings Plans offer a flexible, usage-based pricing model for SageMaker. The goal of the savings plans is to offer you the flexibility to save up to 64% on SageMaker ML instance usage in exchange for a commitment of consistent usage for a 1 or 3-year term. For more information, read the launch blog. Further, to help you save even more, we also just announced a price drop on several instance families in SageMaker.
The SageMaker Savings Plans are on top of the productivity and cost-optimizing capabilities already available in SageMaker Studio. You can improve your data science team’s productivity up to 10 times using SageMaker Studio. SageMaker Studio provides a single web-based visual interface where you can perform all your ML development steps. SageMaker Studio gives you complete access, control, and visibility into each step required to build, train, and deploy models. You can quickly upload data, create new notebooks, train and tune models, move back and forth between steps to adjust experiments, compare results, and deploy models to production all in one place, which boosts productivity.
You can also optimize costs through capabilities such as Managed Spot Training, in which you use Amazon Elastic Compute Cloud (Amazon EC2) Spot Instances for your SageMaker training jobs (see Optimizing and Scaling Machine Learning Training with Managed Spot Training for Amazon SageMaker), and Amazon Elastic Inference, which allows you to attach just the right amount of GPU-powered inference acceleration to any SageMaker instance type.
We are also excited to see continued customer momentum with SageMaker. Just in the first quarter of 2021, we launched 15 new SageMaker case studies and references, spanning a wide range industries including SNCF, Mueller, Bundesliga, University of Oxford, and Latent Space. Some highlights include:
- The data science team at SNFC reduced model training time from 3 days to 10 hours.
- Mueller Water Products automated the daily collection of more than 5 GB of data and used ML to improve leak-detection performance.
- Latent Space scaled model training beyond 1 billion parameters.
We would love for you to join the thousands of customers who are seeing success with Amazon SageMaker. We want to add you to our customer reference list, and we can’t wait to work with you this month!
About the Author
Kimberly Madia is a Principal Product Marketing Manager with AWS Machine Learning. Her goal is to make it easy for customers to build, train, and deploy machine learning models using Amazon SageMaker. For fun outside work, Kimberly likes to cook, read, and run on the San Francisco Bay Trail.
NVIDIA Partners with Boys & Girls Clubs of Western Pennsylvania on AI Pathways Program
Meet Paige Frank: Avid hoopster, Python coder and robotics enthusiast.
Still in high school, the Pittsburgh sophomore is so hooked on AI and robotics, she’s already a mentor to other curious teens.
“Honestly, I never was that interested in STEM. I wanted to be a hair stylist as a kid, which is also cool, but AI is clearly important for our future!” said Paige. “Everything changed in my freshman year, when I heard about the AI Pathways Institute.”
The initiative, known as AIPI for short, began in 2019 as a three-week pilot program offered by Boys & Girls Clubs of Western Pennsylvania (BGCWPA). Paige was in the first cohort of 40 youth to attend AIPI, which also included Tomi Oalore (left) and Makiyah Carrington (right), shown above.
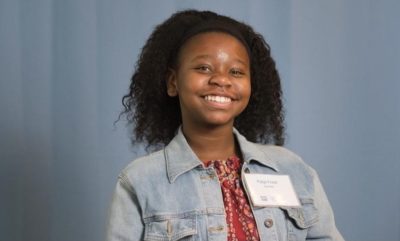
Building on the success of that program, NVIDIA and BGCWPA this week have entered into a three-year partnership with the goal of expanding access to AI education to more students, particularly those from underserved and underrepresented communities.
Core to the collaboration is the creation of an AI Pathways Toolkit to make it easy for Boys & Girls Clubs nationwide and other education-focused organizations to deliver the curriculum to their participants.
“At first it was hard. But once we understood AI fundamentals from the AIPI coursework that the staff at BGCWPA taught and by using the NVIDIA Jetson online videos, it all began to come together,” said Paige. “Learning robotics hands-on with the Jetson Nano made it much easier. And it was exciting to actually see our programming in action as the Jetbot robot navigated the maze we created for the project.”
New AI Pathways to the Future
AI is spreading rapidly. But a major challenge to developing AI skills is access to hands-on learning and adequate computing resources. The AI Pathways Toolkit aims to make AI and robotics curriculum accessible for all students, even those without previous coding experience. It’s meant to prepare — and inspire — more students, like Paige, to see themselves as builders of our AI future.
Another obstacle to AI skills development can be perception. “I wasn’t that excited at first — there’s this thing that it’s too nerdy,” commented Paige, who says most of her friends felt similarly. “But once you get into coding and see how things work on the Jetbot, it’s real fun.”
She sees this transformation in action at her new internship as a mentor with the BGCWPA, where she helps kids get started with AI and coding. “Even kids who aren’t that involved at first really get into it. It’s so inspiring,” she said.
Boys & Girls on an AI Mission
Comprising 14 clubhouses and two Career Works Centers, BGCWPA offers programs, services and outreach that serve more than 12,000 youth ages 4-18 across the region. The AIPI is a part of its effort to provide young people with the tools needed to activate and advance their potential.
With support from NVIDIA, BGCWPA developed the initial three-week AIPI summer camp to introduce local high school students to AI and machine learning. Its curriculum was developed by BGCWPA Director of STEM Education Christine Nguyen and representatives from Carnegie Mellon University using NVIDIA’s educational materials, including the Jetson AI Specialist certification program.
The pilot in 2019 included two local summer camps with a focus on historically underrepresented communities encompassing six school districts. The camp attendees also created a hands-on project using the award-winning Jetson Nano developer kit and Jetbot robotics toolkit.
“We know how important it is to provide all students with opportunities to impact the future of technology,” said Nguyen. “We’re excited to utilize the NVIDIA Jetson AI certification materials with our students as they work toward being leaders in the fields of AI and robotics.”
Students earned a stipend in a work-based learning experience, and all of the participants demonstrated knowledge gained in the “Five Big Ideas in AI,” a framework created by AI4K12, a group working to develop guidelines for K-12 AI education. They also got to visit companies and see AI in action, learn human-centered design and present a capstone project that focused on a social problem they wanted to solve with AI.
“With the support of NVIDIA, we’re helping students from historically underrepresented communities build confidence and skills in the fields of AI, ML and robotics,” said Lisa Abel-Palmieri, Ph.D., president and CEO of BGCWPA. “Students are encouraged to develop personal and professional connections with a diverse group of peers who share similar passions. We also equip participants with the vital knowledge and tools to implement technology that addresses bias in AI and benefits society as a whole.”
From Summer Camp to Yearlong Program
Helping youth get started on a pathway to careers in AI and robotics has become an urgent need. Moreover, learning to develop AI applications requires real-world skills and resources that are often scarce in underserved and underrepresented communities.
NVIDIA’s partnership with BGCWPA includes a funding grant and access to technical resources, enabling the group to continue to develop a new AI Pathways Toolkit and open-source curriculum supported by staff tools and training.
The curriculum scales the summer camp model into a yearlong program that creates a pathway for students to gain AI literacy through hands-on development with the NVIDIA Jetson Nano and Jetbot kits. And the tools and training will make it easy for educators, including the Boys & Clubs’ Youth Development Professionals, to deliver the curriculum to their students.
The toolkit, when completed, will be made available to the network of Boys & Girls Clubs across the U.S., with the goal of implementing the program at 80 clubs by the middle of 2024. The open-source curriculum will also be available to other organizations interested in implementing AI education programs around the world.
As for Paige’s future plans: “I want to strengthen my coding skills and become a Python pro. I also would like to start a robotics club at my high school. And I definitely want to pursue computer science in college. I have a lot of goals,” she said.
Boys & Girls Club Joins AI Educators at GTC21
Abel-Palmieri was a featured panelist at a special event at GTC21 last week. With a record 1,600+ sessions this year, GTC offers a wealth of content — from getting started with AI for those new to the field, to advanced sessions for developers deploying real-world robotics applications. Register for free to view on-demand replays.
Joining Abel-Palmieri on the panel, “Are You Smarter Than a Fifth Grader Who Knows AI?” (SE2802), were Babak Mostaghimi, assistant superintendent of Curriculum, Instructional Support and Innovation for Gwinnett County Public Schools of Suwanee, Georgia; Jim Gibbs, CEO of Meter Feeder; Justin “Mr. Fascinate” Shaifer; and Maynard Okereke (a.k.a. Hip Hop MD) from STEM Success Summit.
Free GTC sessions to help students learn the basics of AI or brush up robotics skills include:
- Jetson 101: Learning Edge AI Fundamentals (S32700)
- Build Edge AI Projects with the Jetson Community (S32750)
- Optimizing for Edge AI on Jetson (S32354)
Many GTC hands-on sessions are designed to help educators learn and teach AI, including: “Duckietown on NVIDIA Jetson: Hands-On AI in the Classroom” with ETH Zurich (S32637) and “Hands-On Deep Learning Robotics Curriculum in High Schools with Jetson Nano” with CAVEDU Education (S32702).
NVIDIA has also launched the Jetson Nano 2GB Developer Kit Grant Program with a goal to further democratize AI and robotics. The new program offers limited quantities of Jetson Developer Kits to professors, educators and trainers across the globe.
The post NVIDIA Partners with Boys & Girls Clubs of Western Pennsylvania on AI Pathways Program appeared first on The Official NVIDIA Blog.
An EPIC way to evaluate reward functions
Cross-posted from the DeepMind Safety blog.
In many reinforcement learning problems the objective is too complex to be specified procedurally, and a reward function must instead be learned from user data. However, how can you tell if a learned reward function actually captures user preferences? Our method, Equivalent-Policy Invariant Comparison (EPIC), allows one to evaluate a reward function by computing how similar it is to other reward functions. EPIC can be used to benchmark reward learning algorithms by comparing learned reward functions to a ground-truth reward.
It can also be used to validate learned reward functions prior to deployment, by comparing them against reward functions learned via different techniques or data sources.
Figure 1: EPIC compares reward functions $R_a$ and $R_b$ by first mapping them to canonical representatives and then computing the Pearson distance between the canonical representatives on a coverage distribution $mathcal{D}$. Canonicalization removes the effect of potential shaping, and Pearson distance is invariant to positive affine transformations.
Broadening the Reach of Contrastive Learning with Viewmaker Networks
The Benefits and Bounds of Self-Supervised Pretraining
Deep learning is data hungry. Neural networks sometimes require millions of human-labeled data points to perform well, making it hard for the average person or company to train these models. This constraint keeps many important applications out of reach, including for rare diseases, low-resource languages, or even developers who want to train models on their own custom datasets.
Fortunately, self-supervised pretraining has recently come to the rescue. These algorithms teach models to learn from large amounts of raw data without requiring humans to label each data point. The resulting models need drastically fewer labeled examples to achieve the same performance on a particular task.
But currently, the pretraining methods used for different kinds of data are all distinct. Since new domains require new algorithms, pretraining is still underexplored in many high-impact domains, including healthcare, astronomy, and remote sensing, as well as multimodal settings that involve learning the relationships between different modalities, like language and vision.
Learning Views for Contrastive Learning
In our ICLR 2021 paper, we make progress on this problem by developing viewmaker networks, a single algorithm which enables competitive or superior pretraining performance on three diverse modalities: natural images, speech recordings, and wearable sensor data.
At its core, our method extends a number of view-based pretraining methods in computer vision. In this family of methods, depicted below, the network’s goal is to tell whether two distorted examples—known as views—were produced from the same original data point. These methods include contrastive learning methods such as SimCLR, MoCo, and InstDisc, along with non-contrastive algorithms like BYOL and SwAV.
A key challenge for these methods is determining what kinds of views to produce from an input—since this determines how hard the task will be for the network, along with what capabilities the network will need to learn as it solves it. In computer vision, for example, the views are carefully-chosen combinations of image-specific data augmentation functions—such as cropping, blurring, and changes in hue, saturation, brightness, and contrast. Selecting views is currently more an art than a science, and requires both domain expertise and trial and error.
In our work, we train a new generative model—called a viewmaker network—to learn good views, without extensive hand tuning or domain knowledge. Viewmaker networks enable pretraining on a wide range of different modalities, including ones where what makes a good view is still unknown. Remarkably, even without the benefits of domain knowledge or carefully-curated transformation functions, our method produces models with comparable or superior transfer learning accuracy to handcrafted views on the three diverse domains we consider! This suggests that viewmaker networks may be an important step towards general pretraining methods that work across modalities.
A Stochastic Bounded Adversary
At its core, a viewmaker network is a stochastic bounded adversary. Let’s break these terms down, one at a time:
Stochastic: Viewmaker networks accept a training example and a random noise vector, and output a perturbed input. Stochasticity enables the network to learn an infinite number of different views to apply to the input during pretraining.
Bounded: The perturbations applied to an input shouldn’t be too large in magnitude—otherwise the pretraining task would be impossible. Because of this, the viewmaker network perturbations are bounded in strength.
But how can we control the strength of a perturbation in a domain-agnostic way? We use a simple L1-norm bound on the input—this gives the viewmaker the flexibility to make either strong changes to a small part of an input, or weaker transformations to a larger part. In practice, we train the viewmaker to directly output a delta—the difference to the eventual perturbation—which is added to the input after being scaled to an L1 radius.
This radius, or “distortion budget,” is tuned as a hyperparameter, but we found a single setting to work well across the three different modalities we considered.
Adversary: What objective function should the viewmaker have? We train it adversarially—in other words, the viewmaker tries to increase the contrastive loss of the encoder network (e.g. SimCLR or InstDisc) as much as possible given the bounded constraint. Unlike GANs, which are known to suffer from training instability, we find viewmakers to be easier to train—perhaps because perturbing the data is a less challenging task than generating it.
Visualizing the Learned Views
Here are example perturbations for two of the different modalities we consider: natural images (left) and speech recordings (right). The center square shows a training example, while the outer images show the scaled perturbation generated by the viewmaker. The images show the diversity of views learned for a single input, as well as how the viewmaker network tailors the perturbations to the input image.
Performance on Transfer Tasks
Remarkably, despite not requiring domain-specific assumptions, viewmaker networks match the performance of expert-tuned views used for images, as measured by performance on a range of transfer tasks. Furthermore, they outperform common views used for spectrograms (e.g. SpecAugment) when pretraining on speech and sensor data—improving transfer accuracy by +9% and +17% points, resp. on average. This suggests viewmakers may be an important ingredient for developing pretraining methods that work across modalities. The tables below show linear evaluation accuracies on image, audio, and wearable sensor datasets (in that order). See the paper for more details.
Conclusion & Future Work
Viewmaker networks untether contrastive learning from a particular set of domain-specific augmentations, resulting in a more general pretraining method. Our results show that viewmakers enable strong pretraining performance on three diverse modalities, without requiring handcrafted expertise or domain knowledge for each domain.
In terms of future work, we’re excited to see viewmakers applied to other domains, either by themselves or as a way to supplement existing handcrafted views. We’re also excited by potential applications of viewmakers to supervised learning and robustness research. Please check out our repo for code and the paper for more details!
Asia’s Rising Star: VinAI Advances Innovation with Vietnam’s Most Powerful AI Supercomputer
A rising technology star in Southeast Asia just put a sparkle in its AI.
Vingroup, Vietnam’s largest conglomerate, is installing the most powerful AI supercomputer in the region. The NVIDIA DGX SuperPOD will power VinAI Research, Vingroup’s machine-learning lab, in global initiatives that span autonomous vehicles, healthcare and consumer services.
One of the lab’s most important missions is to develop the AI smarts for an upcoming fleet of autonomous electric cars from VinFast, the group’s automotive division, driving its way to global markets.
New Hub on the AI Map
It’s a world-class challenge for the team led by Hung Bui. As a top-tier AI researcher and alum of Google’s DeepMind unit with nearly 6,000 citations from more than 200 papers and the winner of an International Math Olympiad in his youth, he’s up for a heady challenge.
In barely two years, Hung’s built a team that now includes 200 researchers. Last year, as a warm-up, they published as many as 20 papers at top conferences, pushing the boundaries of AI while driving new capabilities into the sprawling group’s many products.
“By July, a fleet of cars will start sending us their data from operating 24/7 in real traffic conditions over millions of miles on roads in the U.S. and Europe, and that’s just the start — the volume of data will only increase,” said Hung.
The team will harness the data to design and refine at least a dozen AI models to enable level 3 autonomous driving capabilities for VinFast’s cars.
DGX SuperPOD Behind the Wheel
Hung foresees a need to retrain those models on a daily basis as new data arrives. He believes the DGX SuperPOD can accelerate by at least 10x the AI work of the NVIDIA DGX A100 system VinAI currently uses, letting engineers update their models every 24 hours.
“That’s the goal, it will save a lot of engineering time, but we will need a lot of help from NVIDIA,” said Hung, who hopes to have in May the new cluster of 20 DGX A100 systems linked together with an NVIDIA Mellanox HDR 200Gb/s InfiniBand network.
Developing World-Class Talent
With a DGX SuperPOD in place, Hung hopes to attract and develop more world-class AI talent in Vietnam. It’s a goal shared widely at Vingroup.
In October, the company hosted a ceremony to mark the end of the initial year of studies for the first 260 students at its VinUniversity. Vietnam’s first private, nonprofit college — founded and funded by Vingroup — it so far offers programs in business, engineering, computer science and health sciences.
It’s a kind of beacon pointing to a better future, like the Landmark81 (pictured above), the 81-story skyscraper, the country’s largest, that the group built and operates on the banks of the Saigon River.
“AI technology is a way to move the company forward, and it can make a lot of impact on the lives of people in Vietnam,” he said, noting other group divisions use DGX systems to advance medical imaging and diagnosis.
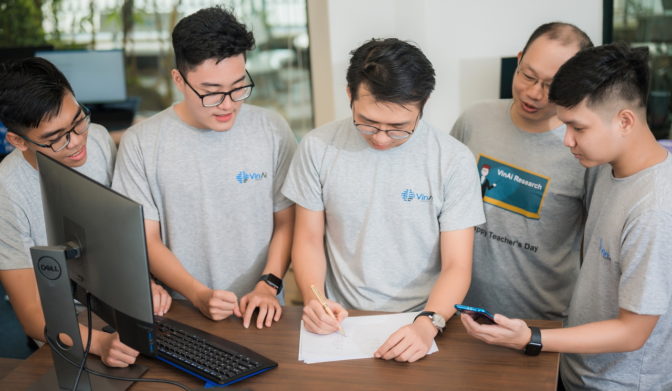
Making Life Better with AI
Hung has seen AI’s impact firsthand. His early work in the field at SRI International, in Silicon Valley, helped spawn the technology that powers the Siri assistant in Apple’s iPhone.
More recently, VinAI developed an AI model that lets users of VinSmart handsets unlock their phones using facial recognition — even if they’re wearing a COVID mask. At the same time, core AI researchers on his team developed Pho-BERT, a version for Vietnamese of the giant Transformer model used for natural-language processing.
It’s the kind of world-class work that two years ago Vingroup’s chairman and Vietnam’s first billionaire, Pham Nhat Vuong, wanted from VinAI Research. He personally convinced Hung to leave a position as research scientist in the DeepMind team and join Vingroup.
Navigating the AI Future
Last year to help power its efforts, VinAI became the first company in Southeast Asia to install a DGX A100 system.
“We’ve been using the latest hardware and software from NVIDIA quite successfully in speech recognition, NLP and computer vision, and now we’re taking our work to the next level with a perception system for driving,” he said.
It’s a challenge Hung gets to gauge daily amid a rising tide of pedestrians, bicycles, scooters and cars on his way to his office in Hanoi.
“When I came back to Vietnam, I had to relearn how to drive here — the traffic conditions are very different from the U.S.” he said.
“After a while I got the hang of it, but it got me thinking a machine probably will do an even better job — Vietnam’s driving conditions provide the ultimate challenge for systems trying to reach level 5 autonomy,” he added.
The post Asia’s Rising Star: VinAI Advances Innovation with Vietnam’s Most Powerful AI Supercomputer appeared first on The Official NVIDIA Blog.
Enforce VPC rules for Amazon Comprehend jobs and CMK encryption for custom models
You can now control the Amazon Virtual Private Cloud (Amazon VPC) and encryption settings for your Amazon Comprehend APIs using AWS Identity and Access Management (IAM) condition keys, and encrypt your Amazon Comprehend custom models using customer managed keys (CMK) via AWS Key Management Service (AWS KMS). IAM condition keys enable you to further refine the conditions under which an IAM policy statement applies. You can use the new condition keys in IAM policies when granting permissions to create asynchronous jobs and creating custom classification or custom entity training jobs.
Amazon Comprehend now supports five new condition keys:
comprehend:VolumeKmsKey
comprehend:OutputKmsKey
comprehend:ModelKmsKey
comprehend:VpcSecurityGroupIds
comprehend:VpcSubnets
The keys allow you to ensure that users can only create jobs that meet your organization’s security posture, such as jobs that are connected to the allowed VPC subnets and security groups. You can also use these keys to enforce encryption settings for the storage volumes where the data is pulled down for computation and on the Amazon Simple Storage Service (Amazon S3) bucket where the output of the operation is stored. If users try to use an API with VPC settings or encryption parameters that aren’t allowed, Amazon Comprehend rejects the operation synchronously with a 403 Access Denied exception.
Solution overview
The following diagram illustrates the architecture of our solution.
We want to enforce a policy to do the following:
- Make sure that all custom classification training jobs are specified with VPC settings
- Have encryption enabled for the classifier training job, the classifier output, and the Amazon Comprehend model
This way, when someone starts a custom classification training job, the training data that is pulled in from Amazon S3 is copied to the storage volumes in your specified VPC subnets and is encrypted with the specified VolumeKmsKey
. The solution also makes sure that the results of the model training are encrypted with the specified OutputKmsKey
. Finally, the Amazon Comprehend model itself is encrypted with the AWS KMS key specified by the user when it’s stored within the VPC. The solution uses three different keys for the data, output, and the model, respectively, but you can choose to use the same key for all three tasks.
Additionally, this new functionality enables you to audit model usage in AWS CloudTrail by tracking the model encryption key usage.
Encryption with IAM policies
The following policy makes sure that users must specify VPC subnets and security groups for VPC settings and AWS KMS keys for both the classifier and output:
{
"Version": "2012-10-17",
"Statement": [{
"Action": ["comprehend:CreateDocumentClassifier"],
"Effect": "Allow",
"Resource": "*",
"Condition": {
"Null": {
"comprehend:VolumeKmsKey": "false",
"comprehend:OutputKmsKey": "false",
"comprehend:ModelKmsKey": "false",
"comprehend:VpcSecurityGroupIds": "false",
"comprehend:VpcSubnets": "false"
}
}
}]
}
For example, in the following code, User 1 provides both the VPC settings and the encryption keys, and can successfully complete the operation:
aws comprehend create-document-classifier
--region region
--document-classifier-name testModel
--language-code en
--input-data-config S3Uri=s3://S3Bucket/docclass/filename
--data-access-role-arn arn:aws:iam::[your account number]:role/testDataAccessRole
--volume-kms-key-id arn:aws:kms:region:[your account number]:alias/ExampleAlias
--output-data-config S3Uri=s3://S3Bucket/output/file name,KmsKeyId=arn:aws:kms:region:[your account number]:alias/ExampleAlias
--vpc-config SecurityGroupIds=sg-11a111111a1exmaple,Subnets=subnet-11aaa111111example
User 2, on the other hand, doesn’t provide any of these required settings and isn’t allowed to complete the operation:
aws comprehend create-document-classifier
--region region
--document-classifier-name testModel
--language-code en
--input-data-config S3Uri=s3://S3Bucket/docclass/filename
--data-access-role-arn arn:aws:iam::[your account number]:role/testDataAccessRole
--output-data-config S3Uri=s3://S3Bucket/output/file name
In the preceding code examples, as long as the VPC settings and the encryption keys are set, you can run the custom classifier training job. Leaving the VPC and encryption settings in their default state results in a 403 Access Denied exception.
In the next example, we enforce an even stricter policy, in which we have to set the VPC and encryption settings to also include specific subnets, security groups, and KMS keys. This policy applies these rules for all Amazon Comprehend APIs that start new asynchronous jobs, create custom classifiers, and create custom entity recognizers. See the following code:
{
"Version": "2012-10-17",
"Statement": [{
"Action":
[
"comprehend:CreateDocumentClassifier",
"comprehend:CreateEntityRecognizer",
"comprehend:Start*Job"
],
"Effect": "Allow",
"Resource": "*",
"Condition": {
"ArnEquals": {
"comprehend:VolumeKmsKey": "arn:aws:kms:region:[your account number]:key/key_id",
"comprehend:ModelKmsKey": "arn:aws:kms:region:[your account number]:key/key_id1",
"comprehend:OutputKmsKey": "arn:aws:kms:region:[your account number]:key/key_id2"
},
"ForAllValues:StringLike": {
"comprehend:VpcSecurityGroupIds": [
"sg-11a111111a1exmaple"
],
"comprehend:VpcSubnets": [
"subnet-11aaa111111example"
]
}
}
}]
}
In the next example, we first create a custom classifier on the Amazon Comprehend console without specifying the encryption option. Because we have the IAM conditions specified in the policy, the operation is denied.
When you enable classifier encryption, Amazon Comprehend encrypts the data in the storage volume while your job is being processed. You can either use an AWS KMS customer managed key from your account or a different account. You can specify the encryption settings for the custom classifier job as in the following screenshot.
Output encryption enables Amazon Comprehend to encrypt the output results from your analysis. Similar to Amazon Comprehend job encryption, you can either use an AWS KMS customer managed key from your account or another account.
Because our policy also enforces the jobs to be launched with VPC and security group access enabled, you can specify these settings in the VPC settings section.
Amazon Comprehend API operations and IAM condition keys
The following table lists the Amazon Comprehend API operations and the IAM condition keys that are supported as of this writing. For more information, see Actions, resources, and condition keys for Amazon Comprehend.
Model encryption with a CMK
Along with encrypting your training data, you can now encrypt your custom models in Amazon Comprehend using a CMK. In this section, we go into more detail about this feature.
Prerequisites
You need to add an IAM policy to allow a principal to use or manage CMKs. CMKs are specified in the Resource element of the policy statement. When writing your policy statements, it’s a best practice to limit CMKs to those that the principals need to use, rather than give the principals access to all CMKs.
In the following example, we use an AWS KMS key (1234abcd-12ab-34cd-56ef-1234567890ab
) to encrypt an Amazon Comprehend custom model.
When you use AWS KMS encryption, kms:CreateGrant and kms:RetireGrant permissions are required for model encryption.
For example, the following IAM policy statement in your dataAccessRole provided to Amazon Comprehend allows the principal to call the create operations only on the CMKs listed in the Resource element of the policy statement:
{"Version": "2012-10-17",
"Statement": {"Effect": "Allow",
"Action": [
"kms:CreateGrant",
"kms:RetireGrant",
"kms:GenerateDataKey",
"kms:Decrypt"
],
"Resource": [
"arn:aws:kms:us-west-2:[your account number]:key/1234abcd-12ab-34cd-56ef-1234567890ab"
]
}
}
Specifying CMKs by key ARN, which is a best practice, makes sure that the permissions are limited only to the specified CMKs.
Enable model encryption
As of this writing, custom model encryption is available only via the AWS Command Line Interface (AWS CLI). The following example creates a custom classifier with model encryption:
aws comprehend create-document-classifier
--document-classifier-name my-document-classifier
--data-access-role-arn arn:aws:iam::[your account number]:role/mydataaccessrole
--language-code en --region us-west-2
--model-kms-key-id arn:aws:kms:us-west-2:[your account number]:key/[your key Id]
--input-data-config S3Uri=s3://path-to-data/multiclass_train.csv
The next example trains a custom entity recognizer with model encryption:
aws comprehend create-entity-recognizer
--recognizer-name my-entity-recognizer
--data-access-role-arn arn:aws:iam::[your account number]:role/mydataaccessrole
--language-code "en" --region us-west-2
--input-data-config '{
"EntityTypes": [{"Type": "PERSON"}, {"Type": "LOCATION"}],
"Documents": {
"S3Uri": "s3://path-to-data/documents"
},
"Annotations": {
"S3Uri": "s3://path-to-data/annotations"
}
}'
Finally, you can also create an endpoint for your custom model with encryption enabled:
aws comprehend create-endpoint
--endpoint-name myendpoint
--model-arn arn:aws:comprehend:us-west-2:[your account number]:document-classifier/my-document-classifier
--data-access-role-arn arn:aws:iam::[your account number]:role/mydataaccessrole
--desired-inference-units 1 --region us-west-2
Conclusion
You can now enforce security settings like enabling encryption and VPC settings for your Amazon Comprehend jobs using IAM condition keys. The IAM condition keys are available in all AWS Regions where Amazon Comprehend is available. You can also encrypt the Amazon Comprehend custom models using customer managed keys.
To learn more about the new condition keys and view policy examples, see Using IAM condition keys for VPC settings and Resource and Conditions for Amazon Comprehend APIs. To learn more about using IAM condition keys, see IAM JSON policy elements: Condition.
About the Authors
Sam Palani is an AI/ML Specialist Solutions Architect at AWS. He enjoys working with customers to help them architect machine learning solutions at scale. When not helping customers, he enjoys reading and exploring the outdoors.
Shanthan Kesharaju is a Senior Architect in the AWS ProServe team. He helps our customers with AI/ML strategy, architecture, and developing products with a purpose. Shanthan has an MBA in Marketing from Duke University and an MS in Management Information Systems from Oklahoma State University.
Facebook invites telecommunications experts to register for the 2021 Connectivity Research Workshop
Facebook Connectivity invites telecommunications experts from around the world to attend the 2021 Connectivity Research Workshop on May 18. Those interested can register at the link below by 5:00 pm PDT on Monday, May 17.
Internet access has become more important than ever, and the COVID-19 pandemic has underscored the need for internet inclusion. It gives us an important channel to connect with our friends and family, prepares us for the technology-intensive jobs of tomorrow, and enables us to participate in the broader economy. Further, according to the recent World Bank report on 4G/5G universal broadband, internet access is increasingly seen as a cornerstone for sustainable development.
However, many people around the world still do not have access to mobile connectivity, and technology innovations are needed to help close this gap. To provide connectivity in rural regions, for example, we have to overcome many challenges, including lack of infrastructure, high development costs, and lack of tailored technical solutions. In urban areas, affordability is a persistent challenge in many areas. In addition, rapid increase of data traffic means telecommunication networks are stressed and future internet performance is at risk.
These complex challenges require innovative business and tech solutions. At Facebook Connectivity, our mission is to bring more people online to a faster internet. To ensure relevance and impact, we need input from thought leaders in industry and academia to identify the most relevant challenges and the most promising solution elements. We need to work together to ensure that our research and development efforts contribute to impactful solution sets.
Over the past several years, we’ve used input from experts to guide our research activities, including the V-band mmWave Channel Sounder program and the diffractive non-line-of-sight wireless backhaul project.
To continue our progress in rural connectivity, Facebook Connectivity is inviting some of our key partners to share their thoughts on our collaborative research activities. The workshop will feature presentations from the following attendees:
- Alex Aimé, Director of Network Investments, Facebook
- Renan Ruiz, CTO, Internet para Todos de Peru
- Omar Tupayachi Calderón, CEO, Mayu Telecomunicaciones
- Roberto Nogueira, CEO, Brisanet
- Grace Chen, Product Manager, Facebook
To register for the workshop, click the link below. Registration closes at 5:00 pm PDT on Monday, May 17.
The post Facebook invites telecommunications experts to register for the 2021 Connectivity Research Workshop appeared first on Facebook Research.
Universal Scene Description Key to Shared Metaverse, GTC Panelists Say
Artists and engineers, architects, and automakers are coming together around a new standard — born in the digital animation industry — that promises to weave all our virtual worlds together.
That’s the conclusion of a group of panelists from a wide range of industries who gathered at NVIDIA GTC21 this week to talk about Pixar’s Universal Scene Description standard, or USD.
“You have people from automotive, advertising, engineering, gaming, and software and we’re all having this rich conversation about USD,” said Perry Nightingale, head of creative at WPP, one of the world’s largest advertising and communications companies. “We’re basically experiencing this live in our work.”
Born at Pixar
Conceived at Pixar more than a decade ago and released as open-source in 2016, USD provides a rich, common language for defining, packaging, assembling and editing 3D data for a growing array of industries and applications.
For more, see “Plumbing the Metaverse with USD” by Michael Kass
Most recently, the technology has been adopted by NVIDIA to build Omniverse — a platform that creates a real-time, shared 3D world to speed collaboration among far-flung workers and even train robots, so they can more safely and efficiently work alongside humans.
The panel — moderated by veteran gaming journalist Dean Takahashi — included Martha Tsigkari, a partner at architects Foster + Partners; Mattias Wikenmalm, a senior visualization expert at Volvo Cars; WPP’s Nightingale; Lori Hufford, vice president of applications integration at engineering software company Bentley Systems; Susanna Holt, vice president at 3D software company Autodesk; and Ivar Dahlberg, a technical artist with Stockholm-based gaming studio Embark Studios.
It also featured two of the engineers who helped create the USD standard at Pixar — F. Sebastian Grass, project lead for USD at Pixar, and Guido Quaroni, now senior director of engineering of 3D and immersive at Adobe.
Joining them was NVIDIA Distinguished Engineer Michael Kass, who, along with NVIDIA’s Rev Lebaredian, helped lead the effort to build NVIDIA Omniverse.
A Sci-Fi Metaverse Come to Life
Omniverse was made to create and experience shared virtual 3D worlds, ones not unlike the science-fiction metaverse described by Neal Stephenson in his early 1990s novel “Snow Crash.” Of course, the full vision of the fictional metaverse remains in the future, but judging by the panel, it’s a future that’s rapidly approaching.
A central goal of Omniverse was to seamlessly connect together as many tools, applications and technologies as possible. To do this, Kass and Lebaredian knew they needed to represent the data using a powerful, expressive and battle-tested open standard. USD exactly fit the bill.
“The fact that you’ve built something so general and extensible that it addresses very nicely the needs of all the participants on this call — that’s an extraordinary achievement,” Kass told USD pioneers Grassia and Quaroni.
One of NVIDIA’s key additions to the USD ecosystem is a replication system. An application programmer can use the standard USD API to query a scene and alter it at will. With no special effort on the part of the programmer, the system keeps track of everything that changes.
In real time, the changes can be published to NVIDIA’s Omniverse Nucleus server, which sends them along to all subscribers. As a result, different teams in different places using different tools can work together and see each other’s changes without noticeable delay.
That technology has become invaluable in architecture, engineering and construction, where large teams from many different disciplines can now collaborate far more easily.
“You need a way for the creative people to do things that can be passed directly to the engineers and consultants in a seamless way,” Tsigkari said. “The structural engineer doesn’t care about my windows, doesn’t care about my doors.”
USD allows the structural engineer to see what they do care about.
USD and NVIDIA Omniverse provide a way to link a wide variety of specialized tools — for creatives, engineers and others — in real time.
“We do see the different industries converging and that’s not going to work if they can’t talk to one another,” said Autodesk’s Holt.
One valuable application is the ability to create product mockups in real time. For too long, Nightingale said, creative teams would have to present clients with 2D mockups of their designs because the tools used by the design teams were incompatible with those of the marketing team. Now those mockups can be in 3D and updated instantly as the design team makes changes.
Virtual Worlds Where AI, Robots and Autonomous Vehicles Can Learn
Capabilities like these aren’t just critical for humans. USD also promises to be the foundation for virtual worlds where new products can be simulated and rigorously tested.
USD and Omniverse are at the center of NVIDIA’s DRIVE simulation platform, Kass explained, which gives automakers a sandbox where they can test new autonomous vehicles. Nothing should go out into the real world until it’s thoroughly tested in simulation, he said.
“We want all of our mistakes to happen in the virtual world, and we based that entire virtual world on USD,” Kass said.
There’s also potential for technologies like USD to allow participants in the kind of virtual worlds game makers are building to play a larger role in shaping those words in real time.
“One of the interesting things we’re seeing is how players can be part of creating a world,” Dahlberg said.
“Now there are a lot more opportunities where you create something together with the inhabitants of that world,” he added.
The first steps, however, have already been taken — thanks to USD — making it easier to exchange data about shared 3D worlds.
“If we can actually get that out of the way, when that’s easy to do, we can start building a proper metaverse,” Volvo’s Wikenmalm said.
For more, see “Plumbing the Metaverse with USD,” by Michael Kass
The post Universal Scene Description Key to Shared Metaverse, GTC Panelists Say appeared first on The Official NVIDIA Blog.