Machine learning (ML) is revolutionizing solutions across industries and driving new forms of insights and intelligence from data. Many ML algorithms train over large datasets, generalizing patterns it finds in the data and inferring results from those patterns as new unseen records are processed. Usually, if the dataset or model is too large to be trained on a single instance, distributed training allows for multiple instances within a cluster to be used and distribute either data or model partitions across those instances during the training process. Native support for distributed training is offered through the Amazon SageMaker SDK, along with example notebooks in popular frameworks.
However, sometimes due to security and privacy regulations within or across organizations, the data is decentralized across multiple accounts or in different Regions and it can’t be centralized into one account or across Regions. In this case, federated learning (FL) should be considered to get a generalized model on the whole data.
In this post, we discuss how to implement federated learning on Amazon SageMaker to run ML with decentralized training data.
What is federated learning?
Federated learning is an ML approach that allows for multiple separate training sessions running in parallel to run across large boundaries, for example geographically, and aggregate the results to build a generalized model (global model) in the process. More specifically, each training session uses its own dataset and gets its own local model. Local models in different training sessions will be aggregated (for example, model weight aggregation) into a global model during the training process. This approach stands in contrast to centralized ML techniques where datasets are merged for one training session.
Federated learning vs. distributed training on the cloud
When these two approaches are running on the cloud, distributed training happens in one Region on one account, and training data starts with a centralized training session or job. During distributed training process, the dataset gets split into smaller subsets and, depending on the strategy (data parallelism or model parallelism), subsets are sent to different training nodes or go through nodes in a training cluster, which means individual data doesn’t necessarily stay in one node of the cluster.
In contrast, with federated learning, training usually occurs in multiple separate accounts or across Regions. Each account or Region has its own training instances. The training data is decentralized across accounts or Regions from the beginning to the end, and individual data is only read by its respective training session or job between different accounts or Regions during the federated learning process.
Flower federated learning framework
Several open-source frameworks are available for federated learning, such as FATE, Flower, PySyft, OpenFL, FedML, NVFlare, and Tensorflow Federated. When choosing an FL framework, we usually consider its support for model category, ML framework, and device or operation system. We also need to consider the FL framework’s extensibility and package size so as to run it on the cloud efficiently. In this post, we choose an easily extensible, customizable, and lightweight framework, Flower, to do the FL implementation using SageMaker.
Flower is a comprehensive FL framework that distinguishes itself from existing frameworks by offering new facilities to run large-scale FL experiments, and enables richly heterogeneous FL device scenarios. FL solves challenges related to data privacy and scalability in scenarios where sharing data is not possible.
Design principles and implementation of Flower FL
Flower FL is language-agnostic and ML framework-agnostic by design, is fully extensible, and can incorporate emerging algorithms, training strategies, and communication protocols. Flower is open-sourced under Apache 2.0 License.
The conceptual architecture of the FL implementation is described in the paper Flower: A friendly Federated Learning Framework and is highlighted in the following figure.
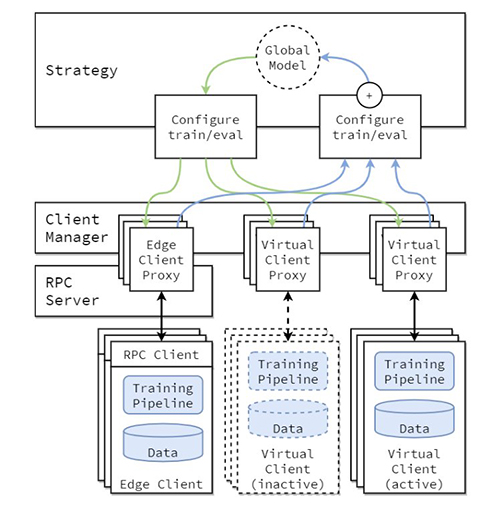
In this architecture, edge clients live on real edge devices and communicate with the server over RPC. Virtual clients, on the other hand, consume close to zero resources when inactive and only load model and data into memory when the client is being selected for training or evaluation.
The Flower server builds the strategy and configurations to be sent to the Flower clients. It serializes these configuration dictionaries (or config dict for short) to their ProtoBuf representation, transports them to the client using gRPC, and then deserializes them back to Python dictionaries.
Flower FL strategies
Flower allows customization of the learning process through the strategy abstraction. The strategy defines the entire federation process specifying parameter initialization (whether it’s server or client initialized), the minimum number of clients available required to initialize a run, the weight of the client’s contributions, and training and evaluation details.
Flower has an extensive implementation of FL averaging algorithms and a robust communication stack. For a list of averaging algorithms implemented and associated research papers, refer to the following table, from Flower: A friendly Federated Learning Framework.
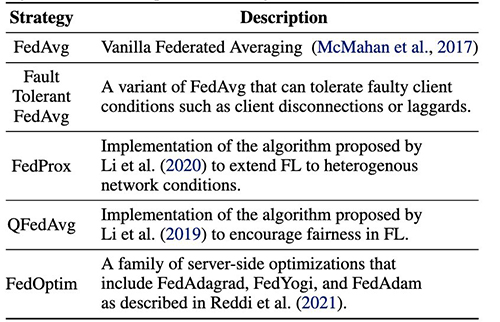
Federated learning with SageMaker: Solution architecture
A federated learning architecture using SageMaker with the Flower framework is implemented on top of bi-directional gRPC (foundation) streams. gRPC defines the types of messages exchanged and uses compilers to then generate efficient implementation for Python, but it can also generate the implementation for other languages, such as Java or C++.
The Flower clients receive instructions (messages) as raw byte arrays via the network. Then the clients deserialize and run the instruction (training on local data). The results (model parameters and weights) are then serialized and communicated back to the server.
The server/client architecture for Flower FL is defined in SageMaker using notebook instances in different accounts in the same Region as the Flower server and Flower client. The training and evaluation strategies are defined on the server as well as the global parameters, then the configuration is serialized and sent to the client over VPC peering.
The notebook instance client starts a SageMaker training job that runs a custom script to trigger the instantiation of the Flower client, which deserializes and reads the server configuration, triggers the training job, and sends the parameters response.
The last step occurs on the server when the evaluation of the newly aggregated parameters is triggered upon completion of the number of runs and clients stipulated on the server strategy. The evaluation takes place on a testing dataset existing only on the server, and the new improved accuracy metrics are produced.
The following diagram illustrates the architecture of the FL setup on SageMaker with the Flower package.
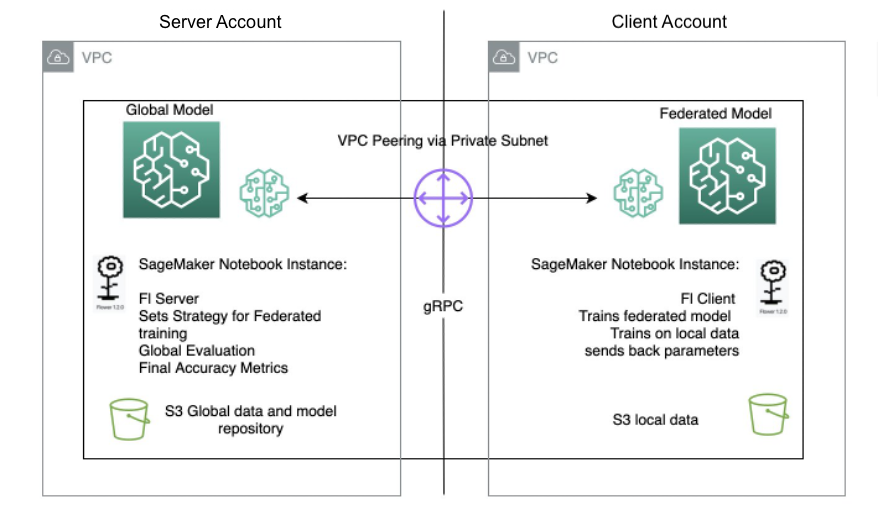
Implement federated learning using SageMaker
SageMaker is a fully managed ML service. With SageMaker, data scientists and developers can quickly build and train ML models, and then deploy them into a production-ready hosted environment.
In this post, we demonstrate how to use the managed ML platform to provide a notebook experience environment and perform federated learning across AWS accounts, using SageMaker training jobs. The raw training data never leaves the account that owns the data and only the derived weights are sent across the peered connection.
We highlight the following core components in this post:
- Networking – SageMaker allows for quick setup of default networking configuration while also allowing you to fully customize the networking depending on your organization’s requirements. We use a VPC peering configuration within the Region in this example.
- Cross-account access settings – In order to allow a user in the server account to start a model training job in the client account, we delegate access across accounts using AWS Identity and Access Management (IAM) roles. This way, a user in the server account doesn’t have to sign out of the account and sign in to the client account to perform actions on SageMaker. This setting is only for purposes of starting SageMaker training jobs, and it doesn’t have any cross-account data access permission or sharing.
- Implementing federated learning client code in the client account and server code in the server account – We implement federated learning client code in the client account by using the Flower package and SageMaker managed training. Meanwhile, we implement server code in the server account by using the Flower package.
Set up VPC peering
A VPC peering connection is a networking connection between two VPCs that enables you to route traffic between them using private IPv4 addresses or IPv6 addresses. Instances in either VPC can communicate with each other as if they are within the same network.
To set up a VPC peering connection, first create a request to peer with another VPC. You can request a VPC peering connection with another VPC in the same account, or in our use case, connect with a VPC in a different AWS account. To activate the request, the owner of the VPC must accept the request. For more details about VPC peering, refer to Create a VPC peering connection.
Launch SageMaker notebook instances in VPCs
A SageMaker notebook instance provides a Jupyter notebook app through a fully managed ML Amazon Elastic Compute Cloud (Amazon EC2) instance. SageMaker Jupyter notebooks are used to perform advanced data exploration, create training jobs, deploy models to SageMaker hosting, and test or validate your models.
The notebook instance has a variety of networking configurations available to it. In this setup, we have the notebook instance run within a private subnet of the VPC and don’t have direct internet access.
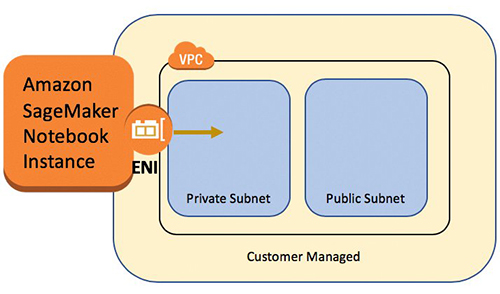
Configure cross-account access settings
Cross-account access settings include two steps to delegate access from the server account to client account by using IAM roles:
- Create an IAM role in the client account.
- Grant access to the role in the server account.
For detailed steps to set up a similar scenario, refer to Delegate access across AWS accounts using IAM roles.
In the client account, we create an IAM role called FL-kickoff-client-job
with the policy FL-sagemaker-actions
attached to the role. The FL-sagemaker-actions
policy has JSON content as follows:
{
"Version": "2012-10-17",
"Statement": [
{
"Effect": "Allow",
"Action": [
"sagemaker:CreateTrainingJob",
"sagemaker:DescribeTrainingJob",
"sagemaker:StopTrainingJob",
"sagemaker:UpdateTrainingJob"
],
"Resource": "*"
},
{
"Effect": "Allow",
"Action": [
"ec2:DescribeSubnets",
"ec2:DescribeVpcs",
"ec2:DescribeNetworkInterfaces"
],
"Resource": "*"
},
{
"Effect": "Allow",
"Action": [
"iam:GetRole",
"iam:PassRole"
],
"Resource": "arn:aws:iam::<client-account-number>:role/service-role/AmazonSageMaker-ExecutionRole-<xxxxxxxxxxxxxxx>"
}
]
}
We then modify the trust policy in the trust relationships of the FL-kickoff-client-job
role:
{
"Version": "2012-10-17",
"Statement": [
{
"Effect": "Allow",
"Principal": {
"AWS": "arn:aws:iam::<server-account-number>:root"
},
"Action": "sts:AssumeRole",
"Condition": {}
}
]
}
In the server account, permissions are added to an existing user (for example, developer
) to allow switching to the FL-kickoff-client-job
role in client account. To do this, we create an inline policy called FL-allow-kickoff-client-job
and attach it to the user. The following is the policy JSON content:
{
"Version": "2012-10-17",
"Statement": {
"Effect": "Allow",
"Action": "sts:AssumeRole",
"Resource": "arn:aws:iam::<client-account-number>:role/FL-kickoff-client-job"
}
}
Sample dataset and data preparation
In this post, we use a curated dataset for fraud detection in Medicare providers’ data released by the Centers for Medicare & Medicaid Services (CMS). Data is split into a training dataset and a testing dataset. Because the majority of the data is non-fraud, we apply SMOTE to balance the training dataset, and further split the training dataset into training and validation parts. Both the training and validation data are uploaded to an Amazon Simple Storage Service (Amazon S3) bucket for model training in the client account, and the testing dataset is used in the server account for testing purposes only. Details of the data preparation code are in the following notebook.
With the SageMaker pre-built Docker images for the scikit-learn framework and SageMaker managed training process, we train a logistic regression model on this dataset using federated learning.
Implement a federated learning client in the client account
In the client account’s SageMaker notebook instance, we prepare a client.py script and a utils.py script. The client.py
file contains code for the client, and the utils.py
file contains code for some of the utility functions that will be needed for our training. We use the scikit-learn package to build the logistic regression model.
In client.py
, we define a Flower client. The client is derived from the class fl.client.NumPyClient. It needs to define the following three methods:
- get_parameters – It returns the current local model parameters. The utility function
get_model_parameters
will do this.
- fit – It defines the steps to train the model on the training data in client’s account. It also receives global model parameters and other configuration information from the server. We update the local model’s parameters using the received global parameters and continue training it on the dataset in the client account. This method also sends the local model’s parameters after training, the size of the training set, and a dictionary communicating arbitrary values back to the server.
- evaluate – It evaluates the provided parameters using the validation data in the client account. It returns the loss together with other details such as the size of the validation set and accuracy back to the server.
The following is a code snippet for the Flower client definition:
"""Client interface"""
class FlowerClient(fl.client.NumPyClient):
def get_parameters(self, config):
return utils.get_model_parameters(model)
def fit(self, parameters, config):
utils.set_model_params(model, parameters)
with warnings.catch_warnings():
warnings.simplefilter("ignore")
model.fit(X_train, y_train)
return utils.get_model_parameters(model), len(X_train), {}
def evaluate(self, parameters, config):
utils.set_model_params(model, parameters)
loss = log_loss(y_test, model.predict_proba(X_test))
accuracy = model.score(X_test, y_test)
return loss, len(X_test), {"accuracy": accuracy}
We then use SageMaker script mode to prepare the rest of the client.py
file. This includes defining parameters that will be passed to SageMaker training, loading training and validation data, initializing and training the model on the client, setting up the Flower client to communicate with the server, and finally saving the trained model.
utils.py
includes a few utility functions that are called in client.py
:
- get_model_parameters – It returns the scikit-learn LogisticRegression model parameters.
- set_model_params – It sets the model’s parameters.
- set_initial_params – It initializes the parameters of the model as zeros. This is required because the server asks for initial model parameters from the client at launch. However, in the scikit-learn framework,
LogisticRegression
model parameters are not initialized until model.fit()
is called.
- load_data – It loads the training and testing data.
- save_model – It saves model as a
.joblib
file.
Because Flower is not a package installed in the SageMaker pre-built scikit-learn Docker container, we list flwr==1.3.0
in a requirements.txt
file.
We put all three files (client.py
, utils.py
, and requirements.txt
) under a folder and tar zip it. The .tar.gz file (named source.tar.gz
in this post) is then uploaded to an S3 bucket in the client account.
Implement a federated learning server in the server account
In the server account, we prepare code on a Jupyter notebook. This includes two parts: the server first assumes a role to start a training job in the client account, then the server federates the model using Flower.
Assume a role to run the training job in the client account
We use the Boto3 Python SDK to set up an AWS Security Token Service (AWS STS) client to assume the FL-kickoff-client-job
role and set up a SageMaker client so as to run a training job in the client account by using the SageMaker managed training process:
sts_client = boto3.client('sts')
assumed_role_object = sts_client.assume_role(
RoleArn = "arn:aws:iam::<client-account-number>:role/FL-kickoff-client-job",
RoleSessionName = "AssumeRoleSession1"
)
credentials = assumed_role_object['Credentials']
sagemaker_client = boto3.client(
'sagemaker',
aws_access_key_id = credentials['AccessKeyId'],
aws_secret_access_key = credentials['SecretAccessKey'],
aws_session_token = credentials['SessionToken'],
)
Using the assumed role, we create a SageMaker training job in client account. The training job uses the SageMaker built-in scikit-learn framework. Note that all S3 buckets and the SageMaker IAM role in the following code snippet are related to the client account:
sagemaker_client.create_training_job(
TrainingJobName = training_job_name,
HyperParameters = {
"penalty": "l2",
"max-iter": "10",
"server-address":"<server-ip-address>:8080",
"sagemaker_program": "client.py",
"sagemaker_submit_directory": "s3://<client-account-s3-code-bucket>/client_code/source.tar.gz",
},
AlgorithmSpecification = {
"TrainingImage": training_image,
"TrainingInputMode": "File",
},
RoleArn = "arn:aws:iam::<client-account-number>:role/service-role/AmazonSageMaker-ExecutionRole-<xxxxxxxxxxxxxxx>",
InputDataConfig=[
{
"ChannelName": "train",
"DataSource": {
"S3DataSource": {
"S3DataType": "S3Prefix",
"S3Uri": "s3://<client-account-s3-data-bucket>/data_prep/",
"S3DataDistributionType": "FullyReplicated",
}
},
},
],
OutputDataConfig = {
"S3OutputPath": "s3://<client-account-s3-bucket-for-model-artifact>/client_artifact/"
},
ResourceConfig = {
"InstanceType": "ml.m5.xlarge",
"InstanceCount": 1,
"VolumeSizeInGB": 10,
},
VpcConfig={
'SecurityGroupIds': [
"<client-account-notebook-instance-security-group>",
],
'Subnets': [
"<client-account-notebook-instance-sunbet>",
]
},
StoppingCondition = {
"MaxRuntimeInSeconds": 86400
},
)
Aggregate local models into a global model using Flower
We prepare code to federate the model on the server. This includes defining the strategy for federation and its initialization parameters. We use utility functions in the utils.py
script described earlier to initialize and set model parameters. Flower allows you to define your own callback functions to customize an existing strategy. We use the FedAvg strategy with custom callbacks for evaluation and fit configuration. See the following code:
"""Initialize the model and federation strategy, then start the server"""
model = LogisticRegression()
utils.set_initial_params(model)
strategy = fl.server.strategy.FedAvg(
min_available_clients = 1, # Minimum number of clients that need to be connected to the server before a training round can start
min_fit_clients = 1, # Minimum number of clients to be sampled for the next round
min_evaluate_clients = 1,
evaluate_fn = get_evaluate_fn(model, X_test, y_test),
on_fit_config_fn = fit_round,
)
fl.server.start_server(
server_address = args.server_address,
strategy = strategy,
config = fl.server.ServerConfig(num_rounds=3) # run for 3 rounds
)
utils.save_model(args.model_dir, model)
The following two functions are mentioned in the preceding code snippet:
- fit_round – It’s used to send the round number to the client. We pass this callback as the
on_fit_config_fn
parameter of the strategy. We do this simply to demonstrate the use of the on_fit_config_fn
parameter.
- get_evaluate_fn – It’s used for model evaluation on the server.
For demo purposes, we use the testing dataset that we set aside in data preparation to evaluate the model federated from the client’s account and communicate the result back to the client. However, it’s worth noting that in almost all real use cases, the data used in the server account is not split from the dataset used in the client account.
After the federated learning process is finished, a model.tar.gz
file is saved by SageMaker as a model artifact in an S3 bucket in the client account. Meanwhile, a model.joblib
file is saved on the SageMaker notebook instance in the server account. Lastly, we use the testing dataset to test the final model (model.joblib
) on the server. Testing output of the final model is as follows:
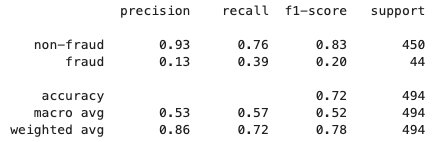
Clean up
After you are done, clean up the resources in both the server account and client account to avoid additional charges:
- Stop the SageMaker notebook instances.
- Delete VPC peering connections and corresponding VPCs.
- Empty and delete the S3 bucket you created for data storage.
Conclusion
In this post, we walked through how to implement federated learning on SageMaker by using the Flower package. We showed how to configure VPC peering, set up cross-account access, and implement the FL client and server. This post is useful for those who need to train ML models on SageMaker using decentralized data across accounts with restricted data sharing. Because the FL in this post is implemented using SageMaker, it’s worth noting that a lot more features in SageMaker can be brought into the process.
Implementing federated learning on SageMaker can take advantage of all the advanced features that SageMaker provides through the ML lifecycle. There are other ways to achieve or apply federated learning on the AWS Cloud, such as using EC2 instances or on the edge. For details about these alternative approaches, refer to Federated Learning on AWS with FedML and Applying Federated Learning for ML at the Edge.
About the authors
Sherry Ding is a senior AI/ML specialist solutions architect at Amazon Web Services (AWS). She has extensive experience in machine learning with a PhD degree in computer science. She mainly works with public sector customers on various AI/ML-related business challenges, helping them accelerate their machine learning journey on the AWS Cloud. When not helping customers, she enjoys outdoor activities.
Lorea Arrizabalaga is a Solutions Architect aligned to the UK Public Sector, where she helps customers design ML solutions with Amazon SageMaker. She is also part of the Technical Field Community dedicated to hardware acceleration and helps with testing and benchmarking AWS Inferentia and AWS Trainium workloads.
Ben Snively is an AWS Public Sector Senior Principal Specialist Solutions Architect. He works with government, non-profit, and education customers on big data, analytical, and AI/ML projects, helping them build solutions using AWS.
Read More