The head of Amazon’s ML Solutions lab shares the lessons she learned leading a 200-year-old government agency — and why she’s excited about the future.Read More
AWS DeepRacer League announces 2020 Championship Cup winner Po-Chun Hsu of Taiwan
AWS DeepRacer is the fastest way to get rolling with machine learning (ML). It’s a fully autonomous 1/18th scale race car driven by reinforcement learning, a 3D racing simulator, and a global racing league. Throughout 2020, tens of thousands of developers honed their ML skills and competed in the League’s virtual circuit via the AWS DeepRacer console and 14 AWS Summit online events leading up to the Championship Cup at 2020 AWS re:Invent.
Developers from around the world tuned in via Twitch to watch the AWS DeepRacer Championship Cup during re:Invent. What started as a group of more than 100 developers in the knockout rounds, narrowed down to a field of 32 in the head-to-head races. The competition ultimately resulted in eight finalists facing off against each other in a Grand Prix style finale, broadcast live at AWS re:Invent. It was an exciting race all the way to the very last lap where Po-Chun Hsu from Team NCTU-CGI came from behind to take the checkered flag and the 2020 AWS DeepRacer Championship. As a grand prize, Po-Chun will receive $10,000 AWS promotional credits and an all-expenses-paid trip to an F1 Grand Prix. Congratulations, Po-Chun!
Watch all the action from the final race in the following video.
The final race was one of the most exciting we’ve seen this season and the first all-virtual Championship race. The starting grid featured JPMC-DriftKing in eighth, Karl-NAB in seventh, Robin-Castro in sixth, Condoriano in fifth, Jochem in fourth, Duckworth in third, Po-Chun-NCTU-CGI in second, and Kuei-NCTU-CGI on the pole. The action got underway at the drop of the green flag, with Po-Chun getting off to an early lead with a decisive and slick move to the inside curve on the first turn. As Po-Chun moved out to increase his lead in first place, a big pileup occurred on the first lap that gathered up the back-five racers, firmly establishing Po-Chun, Duckworth, and fellow NTCU-CGI teammate Kuei as the top three racers to watch for the remainder of the finale.
By Lap 3, Po-Chun really started to take a commanding lead, establishing a more than 7-second gap from second place Duckworth. At this point, the championship was clearly in sight for Po-Chun, while the most competitive racing was for second place between Duckworth, Karl-NAB, and Kuei with less than a second of time between them. But, just as the race looked as if it were well in hand, Po-Chun hit a snag. On Lap 4, Po-Chun spun out, giving the other racers a chance to catch up to the leader, with a second-place Duckworth eating into Po-Chun’s lead and now following by only 3 seconds.
Going into the final lap, we saw an intriguing mishap. Just as the lap began, Po-Chun spun off again. This time, Duckworth overtook him right at the lap line to take the lead for the first time. Although Po-Chun had been leading the whole race, he was now seeing the AWS DeepRacer League Championship slip through his fingers. It was neck and neck to the checkered flag with one lap to go. Po-Chun had a late opportunity to overtake Duckworth on the first turn of the last lap, but his tires got caught under Duckworth and he was forced to take a 5-second penalty. At this point, Duckworth had a clear shot to the finish line with a tremendous come-from-behind victory in his grasp. Then, the unbelievable happened. Rounding the corner to the finish line, Duckworth suddenly slid off the track!
Coming in fast, Po-Chun never missed a beat, rounding the final corner, crossing the line, and taking the Championship as he passed an idling Duckworth. Duckworth quickly restarted to cross over the line to take second. Po-Chun’s teammate Kuei rounded out the podium with a third place finish. The racers finishing from first through eighth were Po-Chun, Duckworth, Kuei, Karl-NAB, Robin-Castro, Jochem, Condoriano, and JPMC-DriftKing. Well done, Grand Prix finalists!
Final Grand Prix Results
Po-Chun was asked what was going through his mind on the last lap. “I thought I was about to lose,” said a stunned Po-Chun. “I never expected the other car would crash right in front of the finish line. I couldn’t believe it!”
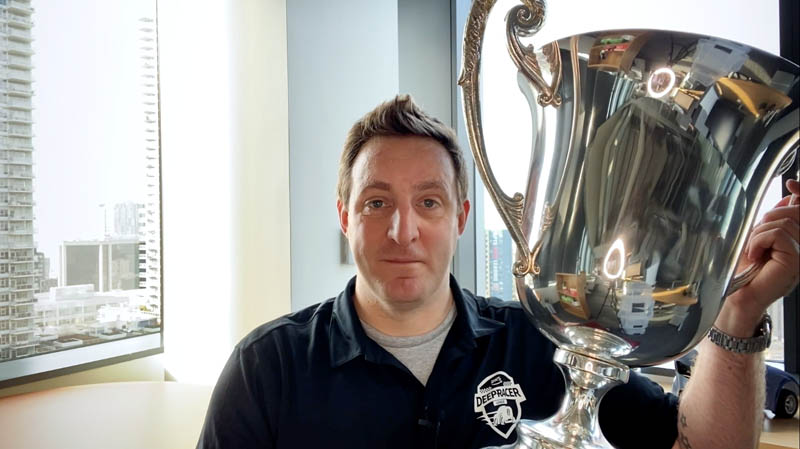
Matt Wood, AWS VP, Articifical Intelligence, presents Po-Chun of team NCTU-CGI the trophy for the 2020 AWS DeepRacer League Championship
Congratulations to Po-Chun Hsu for taking home the 2020 AWS DeepRacer Championship. And thanks to all of the developers who participated in this year’s AWS DeepRacer League Championship Cup.
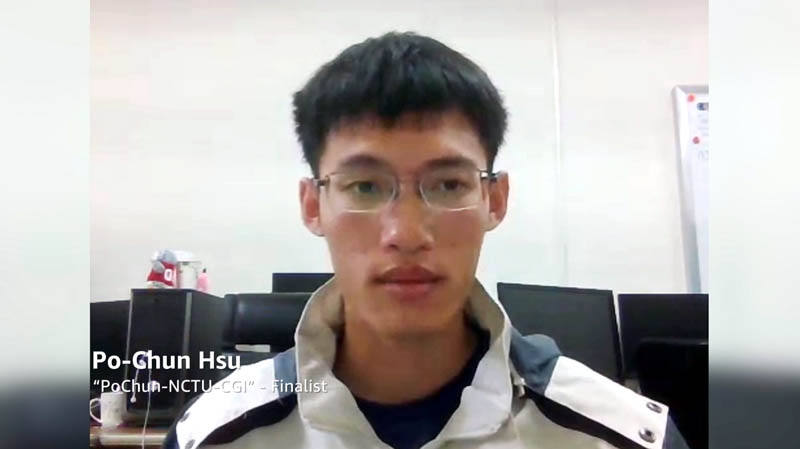
Po-Chun Hsu of Team NCTU-CGI, the 2020 AWS DeepRacer Champion
Don’t forget to start training your models early as the 2021 AWS DeepRacer season is just around the turn. The AWS DeepRacer League is introducing new skill-based Open and Pro racing divisions in March 2021, with five times as many opportunities for racers to win prizes, and recognition for participation and performance. Another exciting new feature coming in 2021 is the expansion of community races into community leagues, enabling organizations and racing enthusiasts to set up their own racing leagues and compete with their friends over multiple races.
It’s never too early to get ready to race. Be sure to take advantage of the December cost reductions for training and evaluation for AWS DeepRacer by over 70% (from $1–3.50 per hour) through December, 2020. Take advantage of these low rates today.
See you in 2021 and let’s get ready to race!
About the Author
Dan McCorriston is a Senior Product Marketing Manager for AWS Machine Learning. He is passionate about technology, collaborating with developers, and creating new methods of expanding technology education. Out of the office he likes to hike, cook and spend time with his family.
Privacy Considerations in Large Language Models
Posted by Nicholas Carlini, Research Scientist, Google Research
Machine learning-based language models trained to predict the next word in a sentence have become increasingly capable, common, and useful, leading to groundbreaking improvements in applications like question-answering, translation, and more. But as language models continue to advance, new and unexpected risks can be exposed, requiring the research community to proactively work to develop new ways to mitigate potential problems.
One such risk is the potential for models to leak details from the data on which they’re trained. While this may be a concern for all large language models, additional issues may arise if a model trained on private data were to be made publicly available. Because these datasets can be large (hundreds of gigabytes) and pull from a range of sources, they can sometimes contain sensitive data, including personally identifiable information (PII) — names, phone numbers, addresses, etc., even if trained on public data. This raises the possibility that a model trained using such data could reflect some of these private details in its output. It is therefore important to identify and minimize the risks of such leaks, and to develop strategies to address the issue for future models.
![]() |
If one prompts the GPT-2 language model with the prefix “East Stroudsburg Stroudsburg…”, it will autocomplete a long block of text that contains the full name, phone number, email address, and physical address of a particular person whose information was included in GPT-2’s training data. |
In “Extracting Training Data from Large Language Models”, a collaboration with OpenAI, Apple, Stanford, Berkeley, and Northeastern University, we demonstrate that, given only the ability to query a pre-trained language model, it is possible to extract specific pieces of training data that the model has memorized. As such, training data extraction attacks are realistic threats on state-of-the-art large language models. This research represents an early, critical step intended to inform researchers about this class of vulnerabilities, so that they may take steps to mitigate these weaknesses.
Ethics of Language Model Attacks
A training data extraction attack has the greatest potential for harm when applied to a model that is available to the public, but for which the dataset used in training is not. However, since conducting this research on such a dataset could have harmful consequences, we instead mount a proof of concept training data extraction attack on GPT-2, a large, publicly available language model developed by OpenAI, that was trained using only public data. While this work focuses on GPT-2 specifically, the results apply to understanding what privacy threats are possible on large language models generally.
As with other privacy- and security-related research, it is important to consider the ethics of such attacks before actually performing them. To minimize the potential risk of this work, the training data extraction attack in this work was developed using publicly available data. Furthermore, the GPT-2 model itself was made public by OpenAI in 2019, and the training data used to train GPT-2 was collected from the public internet, and is available for download by anyone who follows the data collection process documented in the GPT-2 paper.
Additionally, in accordance with responsible computer security disclosure norms, we followed up with individuals whose PII was extracted, and secured their permission before including references to this data in publication. Further, in all publications of this work, we have redacted any personally identifying information that may identify individuals. We have also worked closely with OpenAI in the analysis of GPT-2.
The Training Data Extraction Attack
By design, language models make it very easy to generate a large amount of output data. By seeding the model with random short phrases, the model can generate millions of continuations, i.e., probable phrases that complete the sentence. Most of the time, these continuations will be benign strings of sensible text. For example, when asked to predict the continuation of the string “Mary had a little…”, a language model will have high confidence that the next token is the word “lamb”. However, if one particular training document happened to repeat the string “Mary had a little wombat” many times, the model might predict that phrase instead.
The goal of a training data extraction attack is then to sift through the millions of output sequences from the language model and predict which text is memorized. To accomplish this, our approach leverages the fact that models tend to be more confident on results captured directly from their training data. These membership inference attacks enable us to predict if a result was used in the training data by checking the confidence of the model on a particular sequence.
The main technical contribution of this work is the development of a method for inferring membership with high accuracy along with techniques for sampling from models in a way that encourages the output of memorized content. We tested a number of different sampling strategies, the most successful of which generates text conditioned on a wide variety of input phrases. We then compare the output of two different language models. When one model has high confidence in a sequence, but the other (equally accurate) model has low confidence in a sequence, it’s likely that the first model has memorized the data.
Results
Out of 1800 candidate sequences from the GPT-2 language model, we extracted over 600 that were memorized from the public training data, with the total number limited by the need for manual verification. The memorized examples cover a wide range of content, including news headlines, log messages, JavaScript code, PII, and more. Many of these examples are memorized even though they appear infrequently in the training dataset. For example, for many samples of PII we extract are found in only a single document in the dataset. However, in most of these cases, the originating document contains multiple instances of the PII, and as a result, the model still learns it as high likelihood text.
Finally, we also find that the larger the language model, the more easily it memorizes training data. For example, in one experiment we find that the 1.5 billion parameter GPT-2 XL model memorizes 10 times more information than the 124 million parameter GPT-2 Small model. Given that the research community has already trained models 10 to 100 times larger, this means that as time goes by, more work will be required to monitor and mitigate this problem in increasingly large language models.
Lessons
While we demonstrate these attacks on GPT-2 specifically, they show potential flaws in all large generative language models. The fact that these attacks are possible has important consequences for the future of machine learning research using these types of models.
Fortunately, there are several ways to mitigate this issue. The most straightforward solution is to ensure that models do not train on any potentially problematic data. But this can be difficult to do in practice.
The use of differential privacy, which allows training on a dataset without revealing any details of individual training examples, is one of the most principled techniques to train machine learning models with privacy. In TensorFlow, this can be achieved with the use of the tensorflow/privacy module (or similar for PyTorch or JAX) that is a drop-in replacement for existing optimizers. Even this can have limitations and won’t prevent memorization of content that is repeated often enough. If this is not possible, we recommend at least measuring how much memorization occurs so appropriate action can be taken.
Language models continue to demonstrate great utility and flexibility—yet, like all innovations, they can also pose risks. Developing them responsibly means proactively identifying those risks and developing ways to mitigate them. We hope that this effort to highlight current weaknesses in large language modeling will raise awareness of this challenge in the broader machine learning community and motivate researchers to continue to develop effective techniques to train models with reduced memorization.
Acknowledgements
This work was performed jointly with Florian Tramer, Eric Wallace, Matthew Jagielski, Ariel Herbert-Voss, Katherine Lee, Adam Roberts, Tom Brown, Dawn Song, Ulfar Erlingsson, Alina Oprea, and Colin Raffel.
How The Trevor Project assesses LGBTQ youth suicide risk with TensorFlow
Posted by Wilson Lee (Machine Learning Engineering Manager at The Trevor Project), Dan Fichter (Head of AI & Engineering at The Trevor Project), Amber Zhang, and Nick Hamatake (Software Engineers at Google)
The Trevor Project’s mission is to end suicide among LGBTQ youth. In addition to offering free crisis services through our original phone lifeline (started in 1998), we’ve since expanded to a digital platform, including SMS and web browser-based chat. Unfortunately, there are high-volume times when there are more youth reaching out on the digital platform than there are counselors, and youth have to wait for a counselor to become available. Ideally, youth would be connected with counselors based on their relative risk of attempting suicide, so that those who are at imminent risk of harm would be connected earlier.
As part of the Google AI Impact Challenge, Google.org provided us with a $1.5M grant, Cloud credits, and a Google.org Fellowship, a team of ML, product, and UX specialists who worked full-time pro bono with The Trevor Project for 6 months. The Googlers joined forces with The Trevor Project’s in-house engineering team to apply Natural Language Processing to the crisis contact intake process. As a result, Trevor is now able to connect youth with the help they need faster. And our work together is continuing with the support of a new $1.2M grant as well as a new cohort of Google.org Fellows, who are at The Trevor Project through December helping expand ML solutions.
ML Problem Framing
We framed the problem as a binary text classification problem. The inputs are answers to questions on the intake form that youth complete when they reach out:
- Have you attempted suicide before? Yes / No
- Do you have thoughts of suicide? Yes / No
- How upset are you? [multiple choice]
- What’s going on? [free text input]
The output is a binary classification: whether to place the youth in the standard queue or a priority queue. As counselors become available, they connect with youth from the priority queue before youth from the standard queue.
Data
Once a youth connects with a counselor, the counselor performs a clinical risk assessment and records the result. The risk assessment result can be mapped to whether the youth should have been placed in the standard queue or the priority queue. The full transcript of the (digital) conversation is also logged, as are the answers to the intake questions. Thus, the dataset used for training consisted of a mixture of free-form text, binary / multiple-choice features, and human-provided labels.
Fortunately, there are relatively few youth classified as high-risk compared to standard-risk. This resulted in a significant class imbalance which had to be accounted for during training. Another major challenge was low signal-to-noise ratio in the dataset. Different youth could provide very similar responses on the intake form and then be given opposite classifications by counselors after completing in-depth conversations. Various methods of dealing with these issues are detailed later.
Because of the extremely sensitive nature of the dataset, special measures were taken to limit its storage, access, and processing. We automatically scrubbed and replaced data with personally-identifiable information (PII) such as names and locations with placeholder strings such as “[PERSON_NAME]” or “[LOCATION]”. This means the models were not trained using PII. Access to the scrubbed dataset was limited to the small group of people working on the project, and the data and model were kept strictly within Trevor’s systems and are not accessible to Google.
Metrics
For a binary classification task, we would usually optimize for metrics like precision and recall, or derived measures like F1 score or AUC. For crisis contact classification, however, the metric we need to optimize most for is how long a high-risk youth (one who should be classified into the priority queue) has to wait before connecting with a counselor. To estimate this, we built a queue simulation system that can predict average wait times given a historical snapshot of class balance, quantitative flow of contacts over time, number of counselors available, and the precision and recall of the prediction model.
The simulation was too slow to run during the update step of gradient descent, so we optimized first for proxy metrics such as precision at 80% recall, and precision at 90% recall. We then ran simulations at all points on the precision-recall curve of the resulting model to determine the optimal spot on the curve for minimizing wait time for high-risk youth.
It was also critical to quantify the fairness of the model with respect to the diverse range of demographic and intersectional groups that reach out to Trevor. For each finalized model, we computed false positive and false negative rates broken out by over 20 demographic categories, including intersectionality. We made sure that no demographic group was favored or disfavored by the model more often than the previous system.
Model Selection
We experimented with bi-LSTM and transformer-based models, as they have been shown to provide state-of-the-art results across a broad range of textual tasks. We tried embedding the textual inputs using Glove, Elmo, and Universal Sentence Encoder. For transformer-based models, we tried a single-layer transformer network and ALBERT (many transformer layers pre-trained with unlabeled text from the web).
We selected ALBERT for several reasons. It showed the best performance at the high-recall end of the curve where we were most interested. ALBERT allowed us not only to take advantage of massive amounts of pre-training, but also to leverage some of our own unlabeled data to do further pretraining (more on this later). Since ALBERT shares weights between its transformer layers, the model is cheaper to deploy (important for a non-profit organization) and less prone to overfitting (important given the noisiness of our data).
Training
We trained in a three-step process:
- Pre-training: ALBERT is already pre-trained with a large amount of data from the web. We simply loaded a pre-trained model using TF Hub.
Instructions available here for loading a pre-trained model for text classification,.
- Further pre-training: Since ALBERT’s language model is based on generic Web data, we pre-trained it further using our own in-domain, unlabeled data. This included anonymized text from chat transcripts as well as from forum posts on TrevorSpace, The Trevor Project’s safe-space social networking site for LGBTQ youth. Although the unlabeled data is not labeled for suicide risk, it comes from real youth in our target demographics and is therefore linguistically closer to our labeled dataset than ALBERT’s generic web corpora are. We found that this increased model performance significantly.
Instructions available here for checkpoint management strategies.
- Fine-tuning: We fine-tuned the model using our hand-labeled training data. We initially used ALBERT just to encode the textual response to “What’s going on” and used one-hot vectors to encode the responses to the binary and multiple-choice questions. We then tried converting everything to text and using ALBERT to encode everything. Specifically, instead of encoding the Yes / No answer to a question like “Do you have thoughts of suicide?” as a one-hot vector, we prepended something like “[ counselor] Do you have thoughts of suicide? [ youth] No” to the textual response to “What’s going on?” This yielded significant improvements in performance.
Instructions available here for encoding with BERT tokenizer.
Optimization
We did some coarse parameter selection (learning rate and batch size) using manual trials. We also used Keras Tuner to refine the parameter space further. Because Keras Tuner is model-agnostic, we were able to use a similar tuning script for each of our model classes. For the LSTM-based models, we also used Keras Tuner to decide which kind of embeddings to use.
Normally, we would train with as large of a batch size as would fit on a GPU, but in this case we found better performance with fairly small batch sizes (~8 examples). We theorize that this is because the data has so much noise that it tends to regularize itself. This self-regularization effect is more pronounced in small batches.
Instructions available here here for setting up hyperparameter trials.
Conclusion
We trained a text-based model to prioritize at-risk youth seeking crisis services. The model outperformed a baseline classifier that only used responses from several multiple-choice intake questions as features. The NLP model was also shown to have less bias than the baseline model. Some of the highest-impact ingredients to the final model were 1) Using in-domain unlabeled data to further pretrain an off-the-shelf ALBERT model, 2) encoding multiple-choice responses as full text, which is in turn encoded by ALBERT, and 3) tuning hyperparameters using intuition about our specific dataset in addition to standard search methods.
Despite the success of the model, there are some limitations. The intake questions that produced our dataset were not extremely well-correlated with the results of the expert risk assessments that made up our training labels. This resulted in a low signal-to-noise ratio in our training dataset. More non-ML work could be done in the future to elicit more high-signal responses from youth in the intake process.
We’d like to acknowledge all of the teams and individuals who contributed to this project: Google.org and the Google.org Fellows, The Trevor Project’s entire engineering and data science team, as well as many hours of review and input from Trevor’s crisis service and clinical staff.
You can support our work by donating at TheTrevorProject.org/Donate. Your life-saving gift can help us expand our advocacy efforts, train a record number of crisis counselors, and provide all of our crisis services 24/7.
Scotland’s Rural College Makes Moo-ves Against Bovine Tuberculosis with AI
Each morning millions of bleary-eyed people pour milk into their bowls of cereal or cups of coffee without a second thought as to where that beverage came from.
Few will consider the processes in place to maintain the health of the animals involved in milk production and to ensure that the final product is fit for consumption.
For cattle farmers, few things can sour their efforts like bovine tuberculosis (bTB), a chronic, slow-progressing and debilitating disease. bTB presents significant economic and welfare challenges to the worldwide cattle sector.
Applying GPU-accelerated AI and data science, Scotland’s Rural College (SRUC), headquartered in Edinburgh, recently spearheaded groundbreaking research into how bTB can be monitored and treated more effectively and efficiently.
Bovine Tuberculosis
Caused by bacteria, bTB is highly infectious among cattle and transmissible to other animals and humans.
It also causes substantial financial strain through involuntary culling, animal movement restrictions, and the cost of control and eradication programs. In countries where mandatory eradication programs are not in place for bTB carriers, the disease also carries considerable public health implications.
As bTB is a slow-developing disease, it’s rare for cattle to show any signs of infection until the disease has progressed to its later stages.
To monitor the health of herds, cattle need to receive regular diagnostic tests. Currently, the standard is a single intradermal comparative cervical tuberculin (SICCT) skin test. These tests are time consuming, labor intensive and only correctly identify an infected animal about 50-80 percent of the time.
Milking It
SRUC’s research brought to light a new method of monitoring bTB based on milk samples that were already being collected as part of regular quality control checks through what is called mid-infrared (MIR) analysis.
First, the bTB phenotype (the observable characteristics of an infected animal) was created using data relating to traditional SICCT skin-test results, culture status, whether a cow was slaughtered, and whether any bTB-caused lesions were observed. Information from each of these categories was combined to create a binary phenotype, with zero representing healthy cows and 1 representing bTB-affected cows.
Contemporaneous individual milk MIR data was collected as part of monthly routine milk recording, matched to bTB status of individual animals on the SICCT test date, and converted into 53×20-pixel images. These were used to train a deep convolutional neural network on an NVIDIA DGX Station that was able to identify particular high-level features indicative of bTB infection.
SRUC’s models were able to identify which cows would be expected to fail the SICCT skin test, with an accuracy of 95 percent and a corresponding sensitivity and specificity of 0.96 and 0.94, respectively.
To process the millions of data points used for training their bTB prediction models, the team at SRUC needed a computing system that was fast, stable and secure. Using an NVIDIA DGX Station, models that had previously needed months of work now could be developed in a matter of days. And with RAPIDS data science software on top, the team further accelerated their research and started developing deep learning models in just a few hours.
“By running our models on NVIDIA DGX Station with RAPIDS, we were able to speed up the time it took to develop models at least tenfold,” said Professor Mike Coffey, leader of the Animal Breeding Team and head of EGENES at SRUC. “Speeding up this process means that we’ll be able to get meaningful solutions for combating bTB into the hands of farmers faster and vastly improve how bTB is handled nationwide.”
Moo-ving Forward
Using routinely collected milk samples for the early identification of bTB-infected cows represents an innovative, low-cost and, importantly, noninvasive tool that has the potential to contribute substantially to the push to eradicate bTB in the U.K. and beyond.
Such a tool would enable farmers to get access to crucial information much faster than currently possible. And this would enable farmers to make more efficient and informed decisions that significantly increase the health and welfare of their animals, as well as reduce costs to the farm, government and taxpayer.
The success of predicting bTB status with deep learning also opens up the possibility to calibrate MIR analysis for other diseases, such as paratuberculosis (Johne’s disease), to help improve cattle welfare further.
The post Scotland’s Rural College Makes Moo-ves Against Bovine Tuberculosis with AI appeared first on The Official NVIDIA Blog.
AI helps protect Australian wildlife in fire-affected areas
Over the next six months, more than 600 sensor cameras will be deployed in bushfire-affected areas across Australia, monitoring and evaluating the surviving wildlife populations. This nationwide effort is part of An Eye on Recovery, a large-scale collaborative camera sensor project, run by the World Wide Fund for Nature (WWF) and Conservation International, with the support of a $1 million grant from Google.org. Using Wildlife Insights, a platform powered by Google’s Artificial Intelligence technology, researchers across the country will upload and share sensor camera photos to give a clearer picture of how Australian wildlife is coping after the devastating bushfires in the past year.
Why is this important?
For many Aussies, the horror of last summer’s fires is still very raw and real. Up to 19 million hectares were burned (more than 73,000 square miles), with 12.6 million hectares primarily forest and bushland. Thirty-three lives were lost and 3,094 homes destroyed. And the wildlife toll? A staggering three billion animals were estimated to have been impacted by the flames.

The scale of the damage is so severe that one year on—as we prepare for the next bushfire season—WWF and scientists are still in the field conducting ecological assessments. Our findings have been sobering. Nearly 61,000 koalas, Australia’s most beloved marsupial, are estimated to have been killed or impacted. Over 300 threatened species were affected, pushing more of our precious wildlife on the fast-track towards extinction.
Hope will prevail
In November, I travelled to Kangaroo Island in South Australia to place the first 100 of the sensor cameras in bushfire-ravaged areas. Though much of the native cover has been decimated by the flames, the island’s wildlife has shown signs of recovery.
One animal at risk from the flames is the Kangaroo Island dunnart, an adorable, grey-coloured, nocturnal marsupial so elusive that a researcher told WWF that she’d never seen one in the field. We were fortunate to capture this creature of the night on one of our cameras.
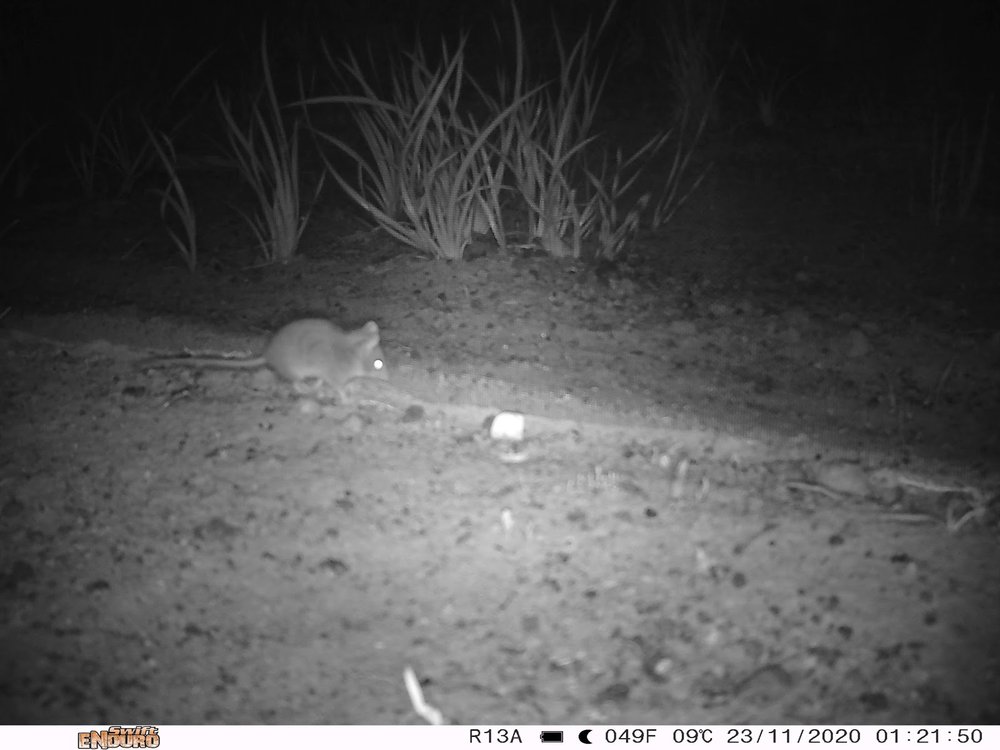
Thou art a dunnart.
But if I hadn’t told you that was a dunnart, you might have thought it was a mouse. And as anyone with thousands of holiday photos will tell you, sorting and organizing heaps of camera pictures and footage can be labor-intensive and time-consuming. Analyzing camera sensor pictures traditionally requires expertise to determine the best pictures (and which ones you can just delete), and you can get hundreds of empty images before you strike gold.
How AI can help
With the Wildlife Insights platform, we can now identify over 700 species of wildlife in seconds and quickly discard empty images, taking the tedium out of the process and helping scientists and ecologists make better and more informed data assessments.
The platform will help us identify wildlife in landscapes impacted by last summer’s bushfires, including the Blue Mountains, East Gippsland, South East Queensland, and of course Kangaroo Island. We’re particularly keen to see species like the Hastings River Mouse, a native rodent that was already endangered before fire tore through its habitat in northern New South Wales, and the brush-tailed rock-wallaby, which lost vital habitat and food to blazes in the Blue Mountains.
These images will help us to understand what species have survived in bushfire zones and determine where recovery actions are needed most.
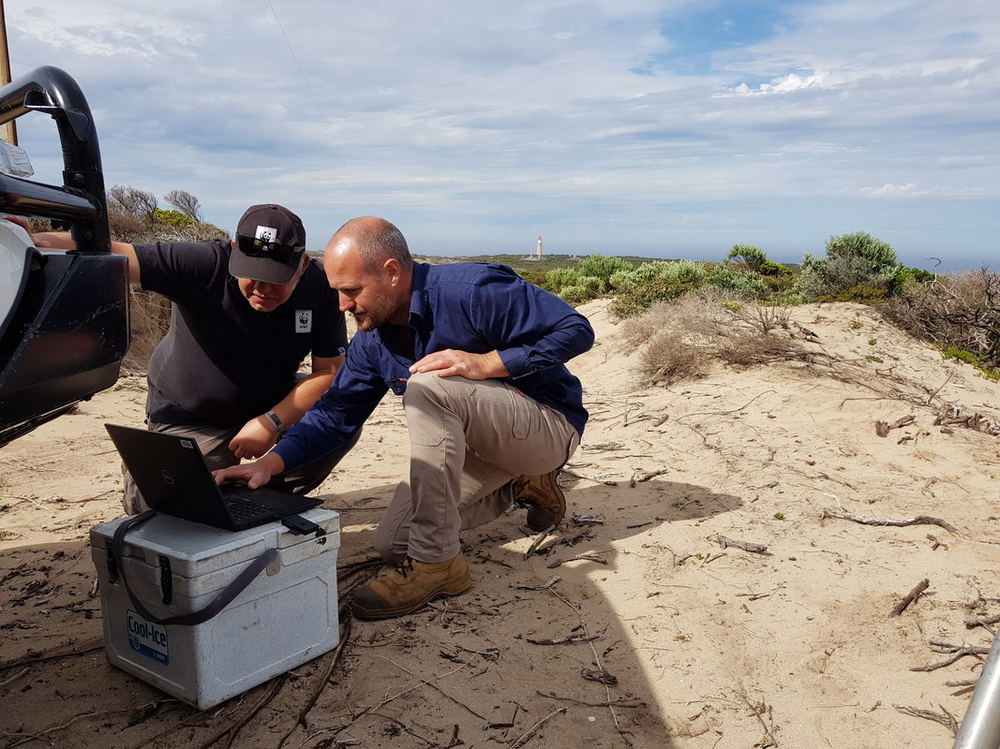
WWF-Australia / Slavica Miskovich
Join us to safeguard species
The platform is still growing, and the more images we feed it, the better it will get at recognizing different types of animals. While we’re already rolling out hundreds of sensor cameras across the country, we are calling for more images—and asking Australians to help. If you have any sensor camera footage, please get in touch with us. We’re looking for images specifically from sensor cameras placed in animal’s habitats, rather than wildlife photography (as beautiful as these pictures may be).
With your help, we can help safeguard species such as the Kangaroo Island dunnart, marvel at their bright beaming eyes on film, and protect their environment on the ground–so future generations can continue to enjoy the richness of Australia’s wildlife.
The Metaverse Begins: NVIDIA Omniverse Open Beta Now Available
Explore virtual collaboration and photorealistic simulation with NVIDIA Omniverse open beta, available now.
NVIDIA Omniverse is an open, cloud-native platform that makes it easy to accelerate design workflows and collaborate in real time. Omniverse allows creators, engineers and researchers to collaborate in virtual worlds that are all connected — the beginnings of the term Neal Stephenson coined, “Metaverse.”
The platform enhances efficiency, productivity and flexibility, as teams from across the room or across the globe can enter Omniverse and simultaneously work together on projects with real-time photorealistic rendering.
As part of the open beta, we’ve released several Omniverse applications that can be used within the Omniverse software, including Omniverse View for architecture, engineering and construction professionals; Omniverse Create for designers, creators and specialists in media and entertainment and manufacturing/product design; and Omniverse Kaolin for 3D deep learning researchers.
Early next year, we’ll release Omniverse Audio2Face, an AI-powered facial animation app; Omniverse Machinima for GeForce RTX gamers; and Isaac Sim 2021.1 for robotics development.
A fundamental breakthrough of Omniverse is the ability to easily work concurrently between software applications. Creators, designers and engineers can access several Omniverse Connectors to leading industry software applications like Autodesk Maya and Revit, Adobe Photoshop, Substance Designer, Substance Painter, McNeel Rhino, Trimble SketchUp and Epic Unreal Engine. Many more are in development, including those for Blender, Houdini, 3ds Max and Motion Builder.
Customers Reach New Heights with Omniverse
Over 500 creators and professionals across industries like architecture, manufacturing, product design, and media and entertainment have tested Omniverse through our early access program.
Global architectural firm Kohn Pedersen Fox used the platform to bring together its worldwide offices and have its people work simultaneously on projects.
“The future of architectural design will rely on the accessibility of all design data in one accurate visualization and simulation application,” said Cobus Bothma, director of applied research at KPF. “We’ve been testing NVIDIA Omniverse and it shows great potential to allow our entire design team to use a variety of applications to collaborate in real time — wherever they’re working.”
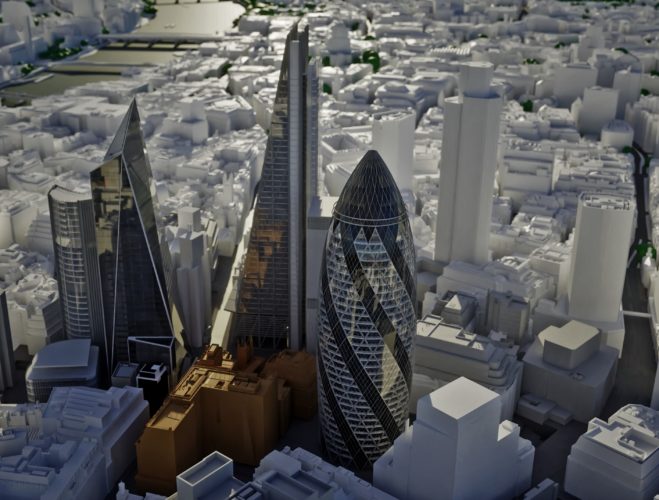
Ecoplants, a member of NVIDIA Inception, focuses on bringing real-world experience into the virtual world in an innovative way, offering high-quality real 3D models and materials.
“Omniverse innovatively fuses development and production workflows of different software packages and across multiple industries,” said Peng Cheng, CEO of Ecoplants. “We are testing how we will use Omniverse to be widely adopted and intensively applied across our company, delivering long-term business value and enabling us to improve efficiency and reduce rendering time from hours to seconds.”
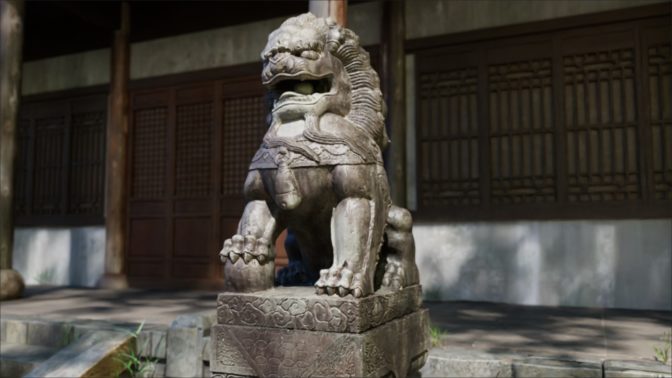
Many industry software leaders are also integrating Omniverse into their applications so users can collaborate and work through graphics workflows.
One early partner for Omniverse is Adobe with its Substance by Adobe suite for texturing and material authoring. At the forefront of physically based rendering, and an early supporter of NVIDIA Material Definition Language, Substance has revolutionized the texturing workflow for real-time rendering.
“From its inception, we’ve been a strong believer in Omniverse and the vision behind application interoperability. We’re proud to be among the first to work with NVIDIA to integrate Substance by Adobe into early versions of the platform,” said Sébastien Deguy, vice president of 3D and Immersive at Adobe. “We can’t wait for our users to experience the power of real-time collaboration and unlock new, more powerful workflows.”
Reallusion is a software developer of animation tools, pipelines and assets for real-time production. Specializing in digital human character creation and animation, Reallusion software provides users with a rapid development solution for virtual characters.
“Since our early understanding of the platform, we have been working to connect our iClone character and animation capabilities through a live link to Omniverse. We believe this open platform will enable artist, designers, movie and game makers to collaborate and design across multiple workflows in real-time with amazing fidelity,” said Charles Chen, CEO of Reallusion. “The ability for our users to move from world to world and leverage the power of our tools combined with other software tools in their workflows seamlessly and quickly will enable world building and real-world simulation.”
The Metaverse Begins
NVIDIA Omniverse has played a critical role in physically accurate virtual world simulation.
At GTC in October, we showed how DRIVE Sim leveraged the platform’s real-time, photoreal simulation capabilities for end-to-end, physically accurate autonomous vehicle virtual validation.
NVIDIA Isaac Sim 2021.1, releasing in February, is built entirely on Omniverse and meets the demand for accurate, reliable, easy-to-use simulation tools in robotics. Researchers and developers around the world can use the app within Omniverse to enhance their robotics simulation and training.
And in research, Omniverse is combining scientific visualization tools with high-quality computer graphics. NVIDIA showcased a simulation of COVID-19, visualized in Omniverse, where each spike on a coronavirus protein is represented with more than 1.8 million triangles, rendered by NVIDIA RTX GPUs.
NVIDIA Omniverse isn’t just a breakthrough in graphics — it’s a platform that’s setting the new standard for design and real-time collaboration across all industries.
Users can download the platform directly from NVIDIA and run on any NVIDIA RTX-enabled GPU. Learn more about NVIDIA Omniverse and download the open beta today at www.nvidia.com/omniverse.
The post The Metaverse Begins: NVIDIA Omniverse Open Beta Now Available appeared first on The Official NVIDIA Blog.
Fault-tolerant quantum circuits with much lower overhead
New approach reduces the number of ancillary qubits required to implement the crucial T gate by at least an order of magnitude.Read More
NVIDIA Chief Scientist Highlights New AI Research in GTC Keynote
NVIDIA researchers are defining ways to make faster AI chips in systems with greater bandwidth that are easier to program, said Bill Dally, NVIDIA’s chief scientist, in a keynote released today for a virtual GTC China event.
He described three projects as examples of how the 200-person research team he leads is working to stoke Huang’s Law — the prediction named for NVIDIA CEO Jensen Huang that GPUs will double AI performance every year.
“If we really want to improve computer performance, Huang’s Law is the metric that matters, and I expect it to continue for the foreseeable future,” said Dally, who helped direct research at NVIDIA in AI, ray tracing and fast interconnects.
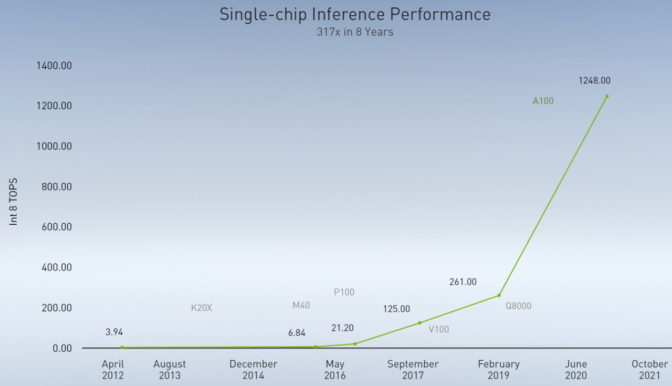
An Ultra-Efficient Accelerator
Toward that end, NVIDIA researchers created a tool called MAGNet that generated an AI inference accelerator that hit 100 tera-operations per watt in a simulation. That’s more than an order of magnitude greater efficiency than today’s commercial chips.
MAGNet uses new techniques to orchestrate the flow of information through a device in ways that minimize the data movement that burns most of the energy in today’s chips. The research prototype is implemented as a modular set of tiles so it can scale flexibly.
A separate effort seeks to replace today’s electrical links inside systems with faster optical ones.
Firing on All Photons
“We can see our way to doubling the speed of our NVLink [that connects GPUs] and maybe doubling it again, but eventually electrical signaling runs out of gas,” said Dally, who holds more than 120 patents and chaired the computer science department at Stanford before joining NVIDIA in 2009.
The team is collaborating with researchers at Columbia University on ways to harness techniques telecom providers use in their core networks to merge dozens of signals onto a single optical fiber.
Called dense wavelength division multiplexing, it holds the potential to pack multiple terabits per second into links that fit into a single millimeter of space on the side of a chip, more than 10x the density of today’s interconnects.
Besides faster throughput, the optical links enable denser systems. For example, Dally showed a mockup (below) of a future NVIDIA DGX system with more than 160 GPUs.
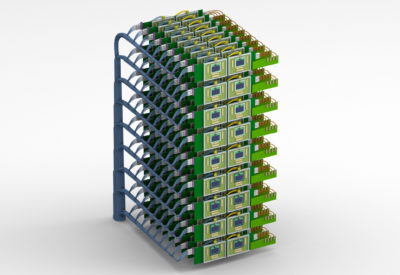
In software, NVIDIA’s researchers have prototyped a new programming system called Legate. It lets developers take a program written for a single GPU and run it on a system of any size — even a giant supercomputer like Selene that packs thousands of GPUs.
Legate couples a new form of programming shorthand with accelerated software libraries and an advanced runtime environment called Legion. It’s already being put to the test at U.S. national labs.
Rendering a Vivid Future
The three research projects make up just one part of Dally’s keynote, which describes NVIDIA’s domain-specific platforms for a variety of industries such as healthcare, self-driving cars and robotics. He also delves into data science, AI and graphics.
“In a few generations our products will produce amazing images in real time using path tracing with physically based rendering, and we’ll be able to generate whole scenes with AI,” said Dally.
He showed the first public demonstration (below) that combines NVIDIA’s conversational AI framework called Jarvis with GauGAN, a tool that uses generative adversarial networks to create beautiful landscapes from simple sketches. The demo lets users instantly generate photorealistic landscapes using simple voice commands.
In an interview between recording sessions for the keynote, Dally expressed particular pride for the team’s pioneering work in several areas.
“All our current ray tracing started in NVIDIA Research with prototypes that got our product teams excited. And in 2011, I assigned [NVIDIA researcher] Bryan Catanzaro to work with [Stanford professor] Andrew Ng on a project that became CuDNN, software that kicked off much of our work in deep learning,” he said.
A First Foothold in Networking
Dally also spearheaded a collaboration that led to the first prototypes of NVLink and NVSwitch, interconnects that link GPUs running inside some of the world’s largest supercomputers today.
“The product teams grabbed the work out of our hands before we were ready to let go of it, and now we’re considered one of the most advanced networking companies,” he said.
With his passion for technology, Dally said he often feels like a kid in a candy store. He may hop from helping a group with an AI accelerator one day to helping another team sort through a complex problem in robotics the next.
“I have one of the most fun jobs in the company if not in the world because I get to help shape the future,” he said.
The keynote is just one of more than 220 sessions at GTC China. All the sessions are free and most are conducted in Mandarin.
Panel, Startup Showcase at GTC China
Following the keynote, a panel of senior NVIDIA executives will discuss how the company’s technologies in AI, data science, healthcare and other fields are being adopted in China.
The event also includes a showcase of a dozen top startups in China, hosted by NVIDIA Inception, an acceleration program for AI and data science startups.
Companies participating in GTC China include Alibaba, AWS, Baidu, ByteDance, China Telecom, Dell Technologies, Didi, H3C, Inspur, Kuaishou, Lenovo, Microsoft, Ping An, Tencent, Tsinghua University and Xiaomi.
The post NVIDIA Chief Scientist Highlights New AI Research in GTC Keynote appeared first on The Official NVIDIA Blog.