The demand for audio and video media content is growing at an unprecedented rate. Organizations are using media to engage with their audiences like never before. Product documentation is increasingly published in video form, and podcasts are increasingly produced in place of blog posts. The recent explosion in the use of virtual workplaces has resulted in content that is encapsulated in the form of recorded meetings, calls, and voicemails. Contact centers also generate media content such as support calls, screen share recordings, or post-call surveys.
Amazon Machine Learning services help you find answers and extract valuable insights from the content of your audio and video files as well as your text files.
In this post, we introduce a new open-source solution, MediaSearch, built to make your media files searchable, and consumable in search results. It uses Amazon Transcribe to convert media audio tracks to text, and Amazon Kendra to provide intelligent search. Your users can find the content they’re looking for, even when it’s embedded in the sound track of your audio or video files. The solution also provides an enhanced Amazon Kendra query application that lets users play the relevant section of original media files, directly from the search results page.
Solution overview
MediaSearch is easy to install and try out! Use it to enable your customers to find answers to their questions from your podcast recordings and presentations, or for your students to find answers from your educational videos or lecture recordings, in addition to text documents.
The MediaSearch solution has two components, as illustrated in the following diagram.
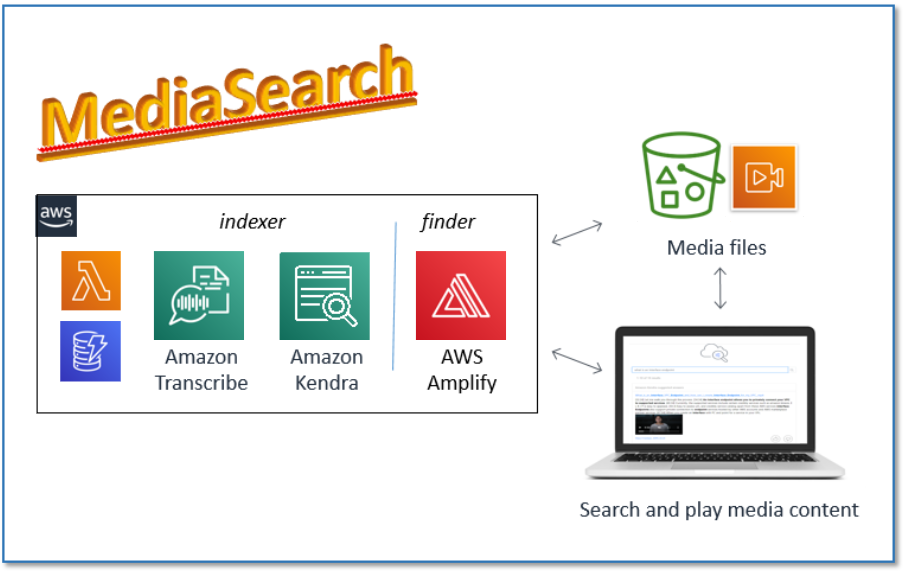
The first component, the MediaSearch indexer, finds and transcribes audio and video files stored in an Amazon Simple Storage Service (Amazon S3) bucket. It prepares the transcriptions by embedding time markers at the start of each sentence, and it indexes each prepared transcription in a new or existing Amazon Kendra index. It runs the first time when you install it, and subsequently runs on an interval that you specify, maintaining the index to reflect any new, modified, or deleted files.
The second component, the MediaSearch finder, is a sample web search client that you use to search for content in your Amazon Kendra index. It has all the features of a standard Amazon Kendra search page, but it also includes in-line embedded media players in the search result, so you can not only see the relevant section of the transcript, but also play the corresponding section from the original media without navigating away from the search page (see the following screenshot).
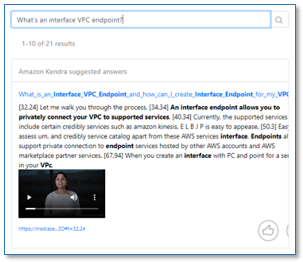
In the sections that follow, we discuss several topics:
- How to deploy the solution to your AWS account
- How to use it to index and search sample media files
- How to use the solution with your own media files
- How the solution works under the hood
- The costs involved
- How to monitor usage and troubleshoot problems
- Options to customize and tune the solution
- How to uninstall and clean up when you’re done experimenting
Deploy the MediaSearch solution
In this section, we walk through deploying the two solution components: the indexer and the finder. We use an AWS CloudFormation stack to deploy the necessary resources in the us-east-1
(N. Virginia) AWS Region.
The source code is available in our GitHub repository. Follow the directions in the README to deploy MediaSearch to additional Regions supported by Amazon Kendra.
Deploy the indexer component
To deploy the indexer component, complete the following steps:
- Choose Launch Stack:

- Change the stack name if required to ensure that it’s unique.
- For ExistingIndexId, leave blank to create a new Amazon Kendra index (Developer Edition), otherwise provide the
IndexId
(not the index name) for an existing index in your account and Region (Amazon Kendra Enterprise Edition should be used for production workloads).
- For MediaBucket and MediaFolderPrefix, use the defaults initially to transcribe and index sample audio and video files.
- For now, use the default values for the other parameters.
- Select the acknowledgement check boxes, and choose Create stack.
- When the stack is created (after approximately 15 minutes), choose the Outputs tab, and copy the value of
IndexId
—you need it to deploy the finder component in the next step.
The newly installed indexer runs automatically to find, transcribe, and index the sample audio and video files. Later you can provide a different bucket name and prefix to index your own media files. If you have media files in multiple buckets, you can deploy multiple instances of the indexer, each with a unique stack name.
Deploy the finder component
To deploy the finder web application component, complete the following steps:
- Choose Launch Stack:

- For IndexId, use the Amazon Kendra index copied from the MediaSearch indexer stack outputs.
- For MediaBucketNames, use the default initially to allow the search page to access media files from the sample file bucket.
- When the stack is created (after approximately 5 minutes), choose the Outputs tab and use the link for
MediaSearchFinderURL
to open the new media search application page in your browser.
If the application isn’t ready when you first open the page, don’t worry! The initial application build and deployment (using AWS Amplify) takes about 10 minutes, so it will work when you try again a little later. If for any reason the application still doesn’t open, refer to the README in the GitHub repo for troubleshooting steps.
And that’s all there is to the deployment! Next, let’s run some search queries to see it in action.
Test with the sample media files
By the time the MediaSearch finder application is deployed and ready to use, the indexer should have completed processing the sample media files (selected AWS Podcast episodes and AWS Knowledge center videos). You can now run your first MediaSearch query.
- Open the MediaSearch finder application in your browser as described in the previous section.
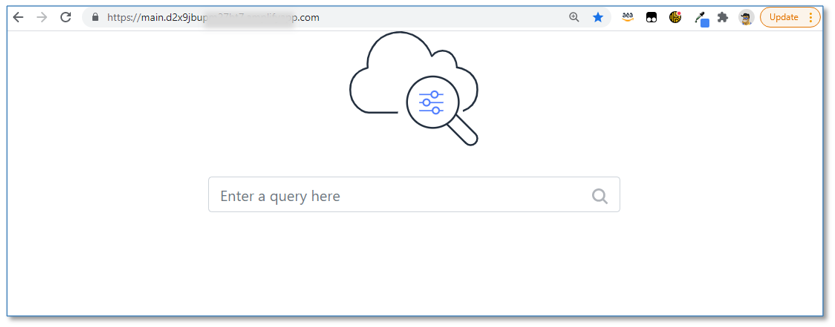
- In the query box, enter
What’s an interface VPC Endpoint?
The query returns multiple results, sourced from the transcripts of the sample media files.
- Observe the time markers at the beginning of each sentence in the answer text. This indicates where the answer is to be found in the original media file.
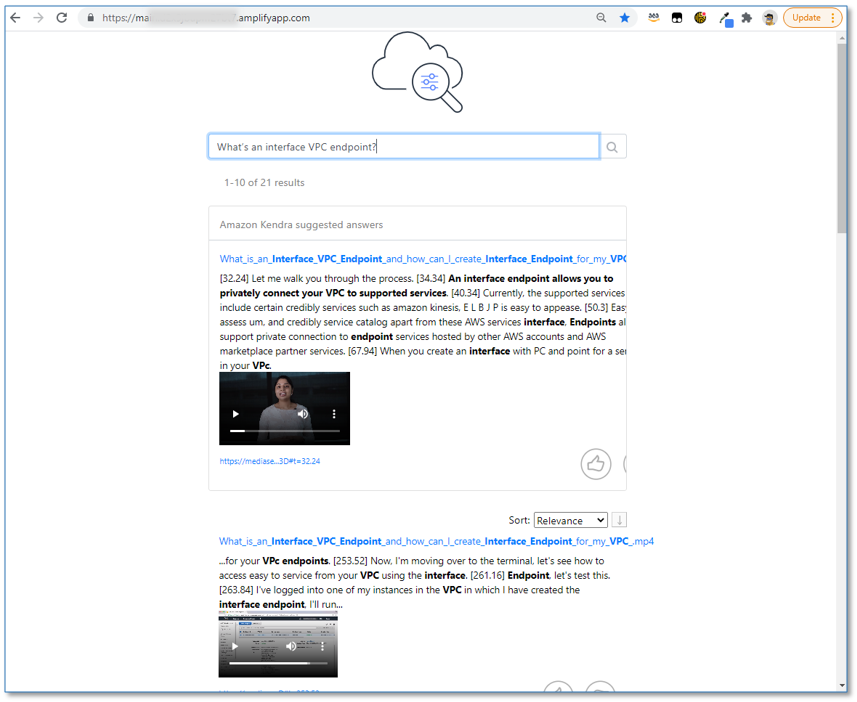
- Use the embedded video player to play the original video inline. Observe that the media playback starts at the relevant section of the video based on the time marker.
- To play the video full screen in a new browser tab, use the Fullscreen menu option in the player, or choose the media file hyperlink shown above the answer text.
- Choose the video file hyperlink (right-click), copy the URL, and paste it into a text editor. It looks something like the following:
https://mediasearchtest.s3.amazonaws.com/mediasamples/What_is_an_Interface_VPC_Endpoint_and_how_can_I_create_Interface_Endpoint_for_my_VPC_.mp4?AWSAccessKeyId=ASIAXMBGHMGZLSYWJHGD&Expires=1625526197&Signature=BYeOXOzT585ntoXLDoftkfS4dBU%3D&x-amz-security-token=.... #t=253.52
This is a presigned S3 URL that provides your browser with temporary read access to the media file referenced in the search result. Using presigned URLs means you don’t need to provide permanent public access to all of your indexed media files.
- Scroll down the page, and observe that some search results are from audio (MP3) files, and some are from video (MP4) files.
You can mix and match media types in the same index. You could include other data source types as well, such as documents, webpages, and other file types supported by available Amazon Kendra data sources, and search across them all, allowing Amazon Kendra to find the best content to answer your query.
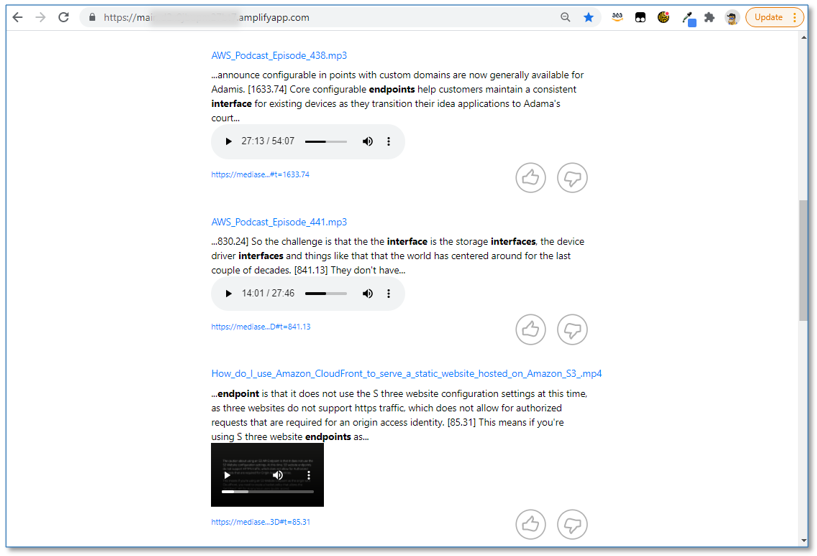
- Experiment with additional queries, such as
What does a solutions architect do?
or What is Kendra?
, or try your own questions.
Index and search your own media files
To index media files stored in your own S3 bucket, replace the MediaBucket
and MediaFolderPrefix
parameters with your own bucket name and prefix when you install or update the indexer component stack, and modify the MediaBucketNames
parameter with your own bucket name when you install or update the finder component stack.
- Create a new MediaSearch indexer stack using an existing Amazon Kendra
IndexId
to add files stored in the new location. To deploy a new indexer, follow the directions in the Deploy the indexer component section in this post, but this time replace the defaults to specify the media bucket name and prefix for your own media files.
- Alternatively, update an existing MediaSearch indexer stack to replace the previously indexed files with files from the new location:
- Select the stack on the CloudFormation console, choose Update, then Use current template, then Next.
- Modify the media bucket name and prefix parameter values as needed.
- Choose Next twice, select the acknowledgement check box, and choose Update stack.
- Update an existing MediaSearch finder stack to change bucket names or add additional bucket names to the
MediaBucketNames
parameter.
When the MediaSearch indexer stack is successfully created or updated, the indexer automatically finds, transcribes, and indexes the media files stored in your S3 bucket. When it’s complete, you can submit queries and find answers from the audio tracks of your own audio and video files.
You have the option to provide metadata for any or all of your media files. Use metadata to assign values to index attributes for sorting, filtering, and faceting your search results, or to specify access control lists to govern access to the files. Metadata files can be in the same S3 folder as your media files (default), or in a parallel folder structure specified by the optional indexer parameter MetadataFolderPrefix
. For more information about how to create metadata files, see S3 document metadata.
You can also provide customized transcription options for any or all of your media files. This allows you to take full advantage of Amazon Transcribe features such as custom vocabularies, automatic content redaction, and custom language models. For more information, refer to the README in the GitHub repo.
How the MediaSearch solution works
Let’s take a quick look under the hood to see how the solution works, as illustrated in the following diagram.
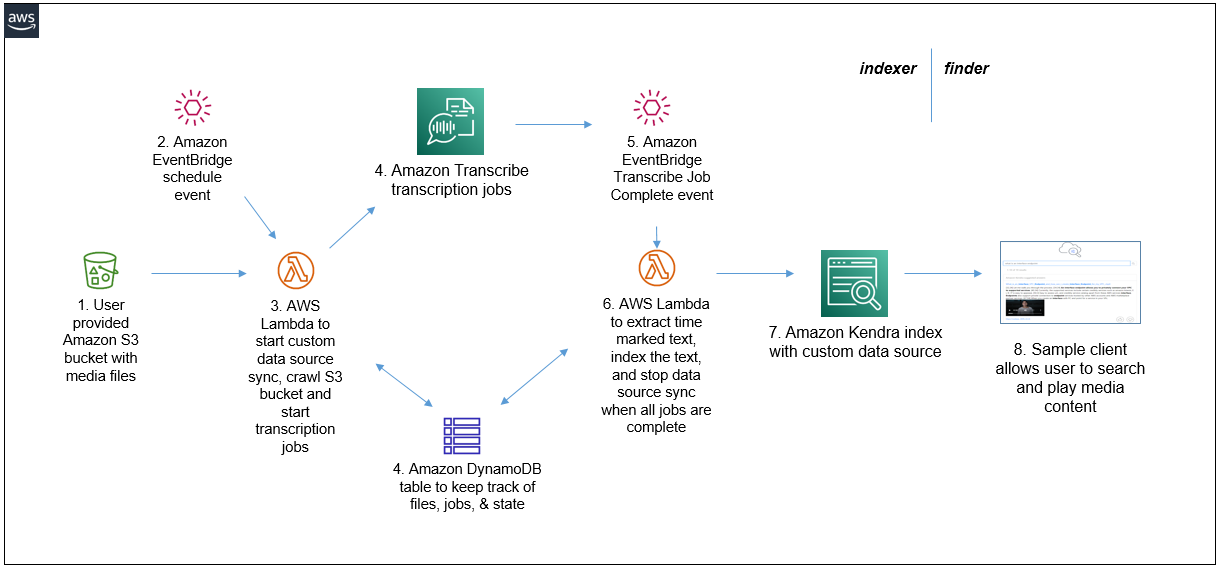
The MediaSearch solution has an event-driven serverless computing architecture with the following steps:
- You provide an S3 bucket containing the audio and video files you want to index and search.
- Amazon EventBridge generates events on a repeating interval (such as every 2 hours, every 6 hours, and so on)
- These events invoke an AWS Lambda function. The function is invoked initially when the CloudFormation stack is first deployed, and then subsequently by the scheduled events from EventBridge. An Amazon Kendra data source sync job is started. The Lambda function lists all the supported media files (FLAC, MP3, MP4, Ogg, WebM, AMR, or WAV) and associated metadata and Transcribe options stored in the user-provided S3 bucket.
- Each new file is added to the Amazon DynamoDB tracking table and submitted to be transcribed by a Transcribe job. Any file that has been previously transcribed is submitted for transcription again only if it has been modified since it was previously transcribed, or if associated Transcribe options have been updated. The DynamoDB table is updated to reflect the transcription status and last modified timestamp of each file. Any tracked files that no longer exist in the S3 bucket are removed from the DynamoDB table and from the Amazon Kendra index. If no new or updated files are discovered, the Amazon Kendra data source sync job is immediately stopped. The DynamoDB table holds a record for each media file with attributes to track transcription job names and status, and last modified timestamps.
- As each Transcribe job completes, EventBridge generates a Job Complete event, which invokes an instance of another Lambda function.
- The Lambda function processes the transcription job output, generating a modified transcription that has a time marker inserted at the start of each sentence. This modified transcription is indexed in Amazon Kendra, and the job status for the file is updated in the DynamoDB table. When the last file has been transcribed and indexed, the Amazon Kendra data source sync job is stopped.
- The index is populated and kept in sync with the transcriptions of all the media files in the S3 bucket monitored by the MediaSearch indexer component, integrated with any additional content from any other provisioned data sources. The media transcriptions are used by Amazon Kendra’s intelligent query processing, which allows users to find content and answers to their questions.
- The sample finder client application enhances users’ search experience by embedding an inline media player with each Amazon Kendra answer that is based on a transcribed media file. The client uses the time markers embedded in the transcript to start media playback at the relevant section of the original media file.
Estimate costs
In addition to Amazon S3 costs associated with storing your media, the MediaSearch solution incurs usage costs from Amazon Kendra and Transcribe. Additional minor (usually not significant) costs are incurred by the other services mentioned after free tier allowances have been used. For more information, see the pricing documentation for Amazon Kendra, Transcribe, Lambda, DynamoDB, and EventBridge.
Pricing example: Index the sample media files
The sample dataset has 25 media files—13 audio podcast and 12 video files—containing a total of around 480 minutes or 29,000 seconds of audio.
If you don’t provide an existing Amazon Kendra IndexId
when you install MediaSearch, a new Amazon Kendra Developer Edition index is automatically created for you so you can test the solution. After you use your free tier allowance (up to 750 hours in the first 30 days), the index costs $1.125 per hour.
Transcribe pricing is based on the number of seconds of audio transcribed, with a free tier allowance of 60 minutes of audio per month for the first 12 months. After the free tier is used, the cost is $0.00040 for each second of audio transcribed. If you’re no longer free tier eligible, the cost to transcribe the sample files is as follows:
- Total seconds of audio = 29,000
- Transcription price per second = $0.00040
- Total cost for Transcribe = [number of seconds] x [cost per second] = 29,000 x $0.00040 = $11.60
Monitor and troubleshoot
To see the details of each media file transcript job, navigate to the Transcription jobs page on the Transcribe console.
Each media file is transcribed only one time, unless the file is modified. Modified files are re-transcribed and re-indexed to reflect the changes.
Choose any transcription job to review the transcription and examine additional job details.
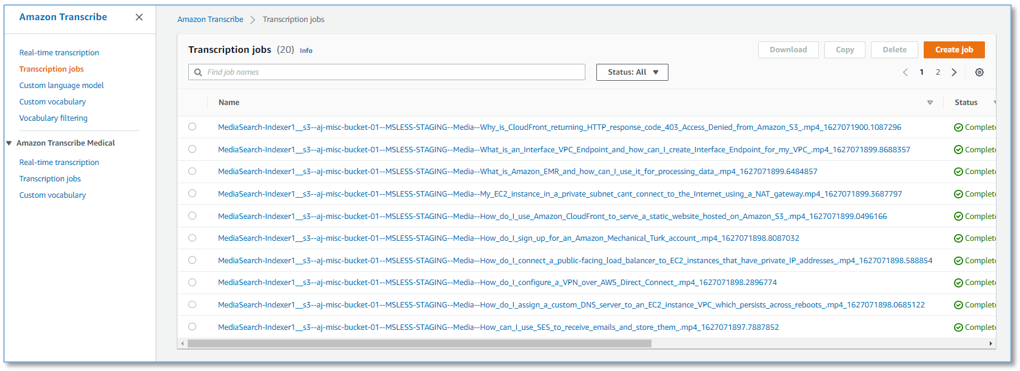
On the Indexes page of the Amazon Kendra console, choose the index used by MediaSearch to examine the index details.
Choose Data sources in the navigation pane to examine the MediaSearch indexer data source, and observe the data source sync run history. The data source syncs when the indexer runs every interval specified in the CloudFormation stack parameters when you deployed or last updated the solution.
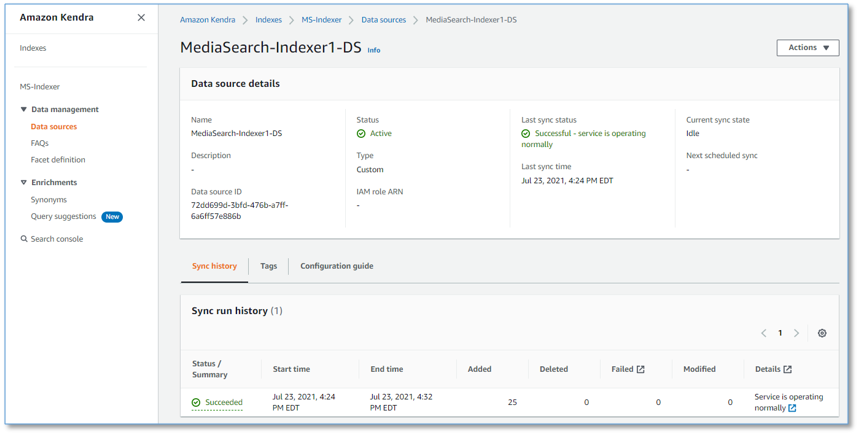
On the DynamoDB console, choose Tables in the navigation pane. Use your MediaSearch stack name as a filter to display the MediaSearch DynamoDB table, and examine the items showing each indexed media file and corresponding status. The table has one record for each media file, and contains attributes with information about the file and its processing status.
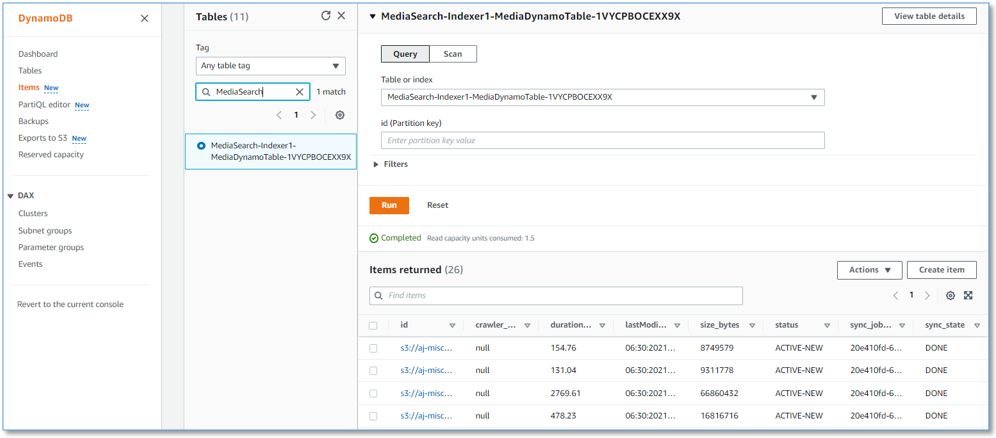
On the Functions page of the Lambda console, use your MediaSearch stack name as a filter to list the two MediaSearch indexer functions described earlier.
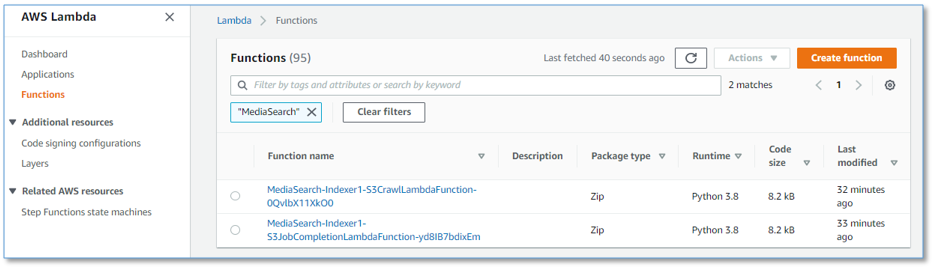
Choose either of the functions to examine the function details, including environment variables, source code, and more. Choose Monitor & View logs in CloudWatch to examine the output of each function invocation and troubleshoot any issues.
Customize and enhance the solution
You can fork the MediaSearch GitHub repository, enhance the code, and send us pull requests so we can incorporate and share your improvements!
The following are a few suggestions for features you might want to implement:
Clean up
When you’re finished experimenting with this solution, clean up your resources by using the AWS CloudFormation console to delete the indexer and finder stacks that you deployed. This deletes all the resources, including any Amazon Kendra indexes that were created by deploying the solution. Pre-existing indexes aren’t deleted. However, media files that were indexed by the solution are removed from the pre-existing index when you delete the indexer stack.
Conclusion
The combination of Amazon Transcribe and Amazon Kendra enable a scalable, cost-effective solution to make your media files discoverable. You can use the content of your media files to find accurate answers to your users’ questions, whether they’re from text documents or media files, and consume them in their native format. In other words, this solution is a leap in bringing media files on par with text documents as containers of information.
The sample MediaSearch application is provided as open source—use it as a starting point for your own solution, and help us make it better by contributing back fixes and features via GitHub pull requests. For expert assistance, AWS Professional Services and other Amazon partners are here to help.
We’d love to hear from you. Let us know what you think in the comments section, or using the issues forum in the MediaSearch GitHub repository.
About the Authors
Bob Strahan is a Principal Solutions Architect in the AWS Language AI Services team.
Abhinav Jawadekar is a Senior Partner Solutions Architect at Amazon Web Services. Abhinav works with AWS Partners to help them in their cloud journey.
Read More